- Department of Respiratory and Critical Care Medicine, First Hospital of Jilin University, Changchun, Jilin, China
Background: The pan-immune-inflammation value (PIV) is an emerging biomarker quantitatively reflecting the systemic immune-inflammatory status. The predictive value of PIV has been well-established across various clinical settings. However, its role in chronic obstructive pulmonary disease (COPD) remains unclear and necessitates further investigation.
Methods: Data from NHANES 1999–2018 were filtered. Logistic regression analyses were used to assess the correlation between COPD prevalence and PIV in all participants. COX regression analyses and Kaplan-Meier survival curves were used to investigate the relationship between COPD all-cause mortality and PIV in COPD patients. Restricted cubic spline (RCS) analyses and piecewise linear regression analyses were additionally employed to explore the correlation between PIV and COPD. Subgroup analyses were performed to further clarify the effects of other covariates on the associations. Sensitivity analyses were employed to assess the robustness of the results.
Results: A total of 28,485 participants aged 40 years and older were recruited for this study. After fully adjusting for covariates, higher PIV levels were independently associated with increased COPD prevalence (OR = 1.67; 95% CI: 1.39–2.01) and all-cause mortality (HR = 2.04; 95% CI: 1.41–2.95). The COPD prevalence curve exhibited an inflection point at Log10-PIV of 2.24, showing no significant correlation on the left side (OR = 0.86; 95% CI: 0.45–1.64) but a positive correlation on the right side (OR = 2.00; 95% CI: 1.57–2.55). The COPD all-cause mortality curve displayed an inflection point at Log10-PIV of 2.38, indicating a negative correlation on the left side (HR = 0.23; 95% CI: 0.12–0.44) and a positive correlation on the right side (HR = 4.12; 95% CI: 2.62–6.48). Subgroup analyses with interaction tests showed that the strength of the correlation between PIV and COPD prevalence was influenced by race, smoking status, and BMI (all p for interaction <0.05). The relationship between PIV and COPD all-cause mortality was unaffected by any covariates (all p for interaction >0.05).
Conclusion: Elevated PIV levels are associated with increased COPD prevalence. COPD patients with either elevated or reduced PIV levels experience higher all-cause mortality. Further large-scale, longitudinal studies are required to corroborate these findings.
1 Introduction
Chronic obstructive pulmonary disease (COPD) is a prevalent chronic airway disease characterized by persistent airway inflammation leading to irreversible airflow limitation (Christenson et al., 2022; Rao et al., 2020). According to the World Health Organization, COPD is the third leading cause of death globally, causing 3.23 million deaths in 2019 (World Health Organization, 2024). It is estimated that between 2020 and 2050, the direct and indirect costs of COPD worldwide will reach 4.326 trillion US dollars, imposing a significant burden on the global economy and society (Chen et al., 2023). Although COPD can affect individuals of all age groups, it is most common in those over 40 years old (Agustí et al., 2020). The pathogenesis of COPD is complex and heterogeneous, influenced by various factors, including environmental and genetic factors (Christenson et al., 2022). Novel and improved biomarkers for airway diseases may help address the clinical and biological complexities of COPD and facilitate precision medicine (Agusti et al., 2016). Further research is needed to discover reliable, measurable, and clinically relevant biomarkers to enhance COPD prevention and improve the prognosis of COPD patients.
The pan-immune-inflammation value (PIV), also known as the aggregate index of systemic inflammation (AISI), is a recently developed biomarker that relies on peripheral blood counts, calculated by using the counts of neutrophils, platelets, monocytes, and lymphocytes, which is believed to be indicative of systemic inflammation (Guven et al., 2022; Yang et al., 2022). Previous studies have demonstrated an association between PIV or AISI and the severity as well as clinical outcomes of numerous immune and inflammation-related diseases, including esophageal cancer (Feng et al., 2023), non-small cell lung cancer (Zhai et al., 2023), abdominal aortic calcification (Jin et al., 2024), and myocardial infarction (Murat et al., 2023). It is noteworthy that a recent study has investigated the prognostic role of AISI in COVID-19 patients with COPD(13). Large-scale studies examining the association between PIV and COPD, however, remain absent.
To address this knowledge gap, we utilized a substantial sample of individuals aged 40 and older from the National Health and Nutrition Examination Survey (NHANES) to assess the association between PIV levels and the COPD prevalence and all-cause mortality. We also employed restricted cubic spline (RCS) curves and segmented linear regression to explore their nonlinear relationships and applied subgroup analyses to identify specific populations.
2 Methods
2.1 Study design and participants
The National Health and Nutrition Examination Survey (NHANES) is a nationwide survey conducted by the National Center for Health Statistics (NCHS) under the Centers for Disease Control and Prevention to collect data on the health of the population. It has been conducted biennially since 1999. We used publicly available NHANES data from 1999 to 2018 for this study. Each NHANES survey cycle underwent rigorous evaluation and approval by the NCHS Research Ethics Review Board, and all participants provided written informed consent (CDC, 2024a). The dataset is available publicly on the NHANES website (CDC, 2024b).
Our study initially screened 101,316 participants from NHANES 1999–2018, excluding the following: 1) individuals with missing COPD data; 2) individuals younger than 40 or equal to 80 or older than 80; 3) individuals with missing PIV data; 4) individuals with incomplete covariate data (e.g., BMI, CVD, hypertension, diabetes). Ultimately, a total of 28,485 participants were included in this study (Figure 1).
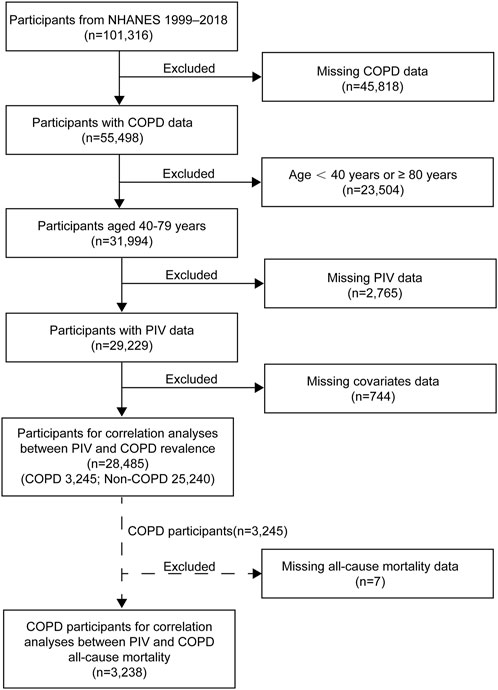
Figure 1. Flowchart of participant selection for this study. NHANES, National Health and Nutrition Examination Survey; COPD, Chronic Obstructive Pulmonary Disease.
2.2 Assessment of COPD
In accordance with the 2024 Global Initiative for Chronic Obstructive Lung Disease (GOLD) guidelines (GOLD, 2024) and the methodology used in previous studies (Huang et al., 2023a; Liu et al., 2024), we defined COPD as the presence of any of the following: (Christenson et al., 2022) forced expiratory volume in 1 s (FEV1)/forced vital capacity (FVC) <0.7 after bronchodilator inhalation; (Rao et al., 2020) individuals aged 40 or older with a history of smoking or chronic bronchitis who were currently undergoing COPD treatment, including inhaled corticosteroids, mast cell stabilizers, leukotriene modifiers, and selective phosphodiesterase-4 inhibitors; (World Health Organization, 2024) a positive response to any of the questions, “Has a doctor or other health professional ever told you that you had COPD?” “Has a doctor or other health professional ever told you that you had chronic bronchitis?” or “Has a doctor or other health professional ever told you that you had emphysema?”.
2.3 Assessment of PIV
The PIV was calculated as neutrophil count (10^9/L) × platelet count (10^9/L) × monocyte count (10^9/L)/lymphocyte count (10^9/L). Log10-PIV represents the logarithmic transformation of PIV with base 10, applied to address the skewed distribution of PIV.
2.4 Mortality data collection
We utilized NHANES data from 1999 to 2018 and prospectively correlated it with National Death Index (NDI) mortality data, with follow-up extending December 31, 2019. The primary outcome assessed in this study was all-cause mortality, defined as deaths from any cause.
2.5 Covariates
Based on the published studies, we selected several significant potential covariates that might influence the results, including age, sex, race, marriage, educational attainment, smoking status, body mass index (BMI, kg/m2), diabetes, hypertension, and cardiovascular disease (CVD). Age, sex, race, marriage, and educational attainment were obtained from self-reports by the participants. Smoking status was categorized into three groups: former, current, and never smokers. Former smokers were defined as individuals who had smoked at least 100 cigarettes in their lifetime but were not currently smoking. Current smokers were those who had smoked at least 100 cigarettes in their lifetime and were currently smoking either daily or occasionally. Never smokers were defined as individuals who had smoked no more than 100 cigarettes in their lifetime. BMI was grouped as follows: underweight (<18.5 kg/m2), normal (18.5–24.9 kg/m2), overweight (25–29.9 kg/m2), and obese (≥30 kg/m2). Diabetes was diagnosed based on a self-reported physician diagnosis or the application of diabetes medications. Hypertension was defined by a systolic blood pressure ≥130 mmHg, a diastolic blood pressure ≥80 mmHg, self-reported hypertension, or the use of antihypertensive medications. CVD was identified based on an affirmative response to the question, “Has a doctor or other health professional ever told you that you had congestive heart failure (CHF), coronary heart disease (CHD), angina pectoris, heart attack, or stroke?”.
2.6 Statistical analyses
All statistical analyses used appropriate sampling weights in accordance with the NHANES Analytic Guidelines to ensure that the sample data accurately represent the entire U.S. population, given the complex multistage probability sampling design. Continuous variables were presented as means (standard error), while categorical variables were expressed as counts (percentages). Due to the skewed distribution of PIV, it was logarithmically transformed for use in regression and subgroup analyses. We applied the weighted Student's t test for continuous variables and the chi-squared test for categorical variables.
Multiple logistic regression analyses were used to examine the associations between PIV and COPD prevalence. Three multivariate-adjusted models were constructed: the crude model without adjusting for any covariates, model 1 adjusting for age, sex, race, marriage, educational attainment, smoking status, and BMI, and model 2 adjusting for all covariates. The results were reported as odds ratios (ORs) and 95% confidence intervals (CIs).
Multiple COX regression analyses and Kaplan-Meier survival curves were utilized to assess the relationships between the PIV and all-cause mortality in COPD participants. Three models were similarly developed following the previous section. The results were indicated as hazard ratios (HRs) and 95% CIs.
We used restricted cubic spline (RCS) analyses to explore the potential nonlinear relationships between PIV and both COPD prevalence and all-cause mortality. Additionally, two-piecewise linear regression analysis was applied to investigate the association between PIV and COPD in more detail. In addition, subgroup analyses were conducted to evaluate the consistency of these relationships across subgroups, and interaction tests were performed to identify the interaction effects among covariates. Furthermore, data from COPD participants with less than 24 months of follow-up were excluded for sensitivity analyses to assess the robustness of the results regarding the relationship between PIV and all-cause mortality in COPD.
All data were analyzed using R software (version 4.3.2). A two-sided p-value <0.05 was considered statistically significant.
3 Results
3.1 Basic characteristics of participants
This study encompassed a total of 28,485 participants with a mean age of 55.80 years, comprising 14,007 males (47.80%) and 14,478 females (52.20%), including 3,245 COPD participants and 25,240 non-COPD participants. COPD participants may be older than non-COPD participants. Additionally, the two groups differed significantly in sex, race, marriage, educational attainment, smoking status, BMI, diabetes, hypertension, and CVD. Furthermore, the PIV of the COPD group was significantly higher than that of the non-COPD group (all p < 0.0001) (Table 1).
3.2 Associations between PIV and COPD prevalence
Among the 23,844 participants included, logistic regression analyses were applied to explore the relationship between PIV and the COPD prevalence, as detailed in Table 2. The results indicated a positive correlation between Log10-PIV and COPD, which remained statistically significant in the crude model (OR = 2.52, 95% CI: 2.13–2.99, p < 0.0001), model 1 (OR = 1.77, 95% CI: 1.48–2.12, p < 0.0001), and model 2 (OR = 1.67, 95% CI: 1.39–2.01, p < 0.0001). When Log10-PIV was transformed into a categorical variable by tertiles, the first tertile group (Q1, 0.07≤Log10-PIV≤2.27, n = 9,505), the second tertile group (Q2, 2.27<Log10-PIV≤2.51, n = 9,506), and the third tertile group (Q3, 2.51≤Log10-PIV≤4.36, n = 9,476) were analyzed. The Q2 group (OR = 1.24, 95% CI: 1.10–1.41, p < 0.0001) and Q3 group (OR = 1.79, 95% CI: 1.59–2.03, p < 0.0001) exhibited higher risks of COPD in the crude model. In the model adjusting for selected covariates (model 1), the Q3 group (OR = 1.41, 95% CI: 1.25–1.61, p < 0.0001) had an increased risk of COPD. Similarly, in the model adjusting for all covariates (model 2), only the Q3 group (OR = 1.36, 95% CI: 1.19–1.56, p < 0.0001) demonstrated a higher risk of COPD. Across all models, compared to the reference group (Q1), individuals in the highest tertile (Q3) consistently maintained a positive association with COPD.
3.3 Associations between PIV and COPD all-cause mortality
Among the 3,238 available death data of COPD participants, during a median follow-up period of 94 months, 852 cases (26%) of all-cause mortality occurred. The final cohort of COPD participants was categorized into three groups based on Log10-PIV tertiles: the first tertile group (Q1, 1.09≤Log10-PIV≤2.35, n = 1,080), the second tertile group (Q2, 2.35<Log10-PIV≤2.60, n = 1,075), and the third tertile group (Q3, 2.60≤Log10-PIV≤3.80, n = 1,083). Baseline characteristics of COPD participants according to Log10-PIV tertiles are detailed in Table 3. The results indicated that individuals with higher Log10-PIV were more likely to be non-Hispanic white, have a higher likelihood of smoking, have a higher tendency towards obesity, and have a greater likelihood with CVD (all p < 0.05).
We generated Kaplan-Meier survival curves with accompanying risk tables, using weighted survival rates at a follow-up duration of 249 months as the endpoint. In the Kaplan-Meier survival curves, the Q3 group exhibited the highest all-cause mortality (p < 0.0001, Figure 2). COX regression analyses showed a significant positive correlation between Log10-PIV and the COPD all-cause mortality in the crude model (HR = 2.86, 95% CI: 1.94–4.21, p < 0.0001), model 1 (HR = 2.12, 95% CI: 1.49–3.03, p < 0.0001), and model 2 (HR = 2.04, 95% CI: 1.41–2.95, p < 0.001). In all models, compared to the Q1 group, the Q3 group had a higher risk of all-cause mortality (crude model: HR = 1.78, 95% CI: 1.40–2.27, p < 0.0001; model 1: HR = 1.54, 95% CI: 1.22–1.94, p < 0.001; model 2: HR = 1.49, 95% CI: 1.17–1.89, p = 0.001) (Table 4).
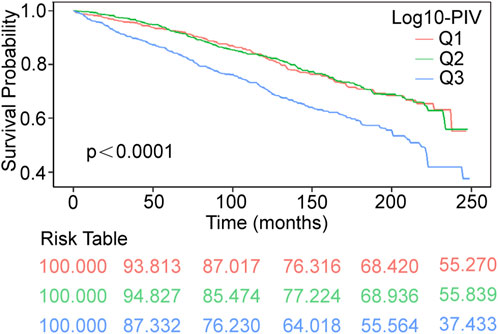
Figure 2. Kaplan–Meier survival curves of COPD all-cause mortality during 249 months follow–up duration. COPD, Chronic Obstructive Pulmonary Disease; PIV, pan-immune-inflammation value; Q1-3 respectively represent the groups divided according to the tertiles; Q1 [1.09, 2.35]; Q2 (2.35, 2.60]; Q3 (2.60, 3.80].
3.4 Identification of nonlinear relationship
To identify the potential nonlinear relationships between PIV and COPD, RCS analyses were applied after adjusting for all covariates. The results are presented in Figure 3. Log10-PIV showed a nonlinear correlation with COPD, exhibiting a J-shaped curve for COPD prevalence (p for nonlinear <0.05) and a U-shaped curve for COPD all-cause mortality (p for nonlinear <0.0001). The RCS curves exhibited distinct inflection points, prompting the execution of segmented linear regression analyses, as detailed in Table 5. The results revealed a significant inflection point (K = 2.24) between Log10-PIV and COPD prevalence. When Log10-PIV was below 2.24, the association between Log10-PIV and COPD prevalence was not statistically significant (OR = 0.86, 95% CI: 0.45–1.64, p = 0.65), whereas it was significantly positive when Log10-PIV exceeded 2.24 (OR = 2.00, 95% CI: 1.57–2.55, p < 0.0001). A distinct inflection point was also identified for Log10-PIV and COPD all-cause mortality (K = 2.38). On the left side of the inflection point, Log10-PIV had a strong negative correlation with all-cause mortality (HR = 0.23, 95% CI: 0.12–0.44, p < 0.0001). On the right side of the inflection point, there was a significant positive correlation between Log10-PIV and all-cause mortality (HR = 4.12, 95% CI: 2.62–6.48, p < 0.0001). These findings indicate that PIV levels have varying effects on COPD.
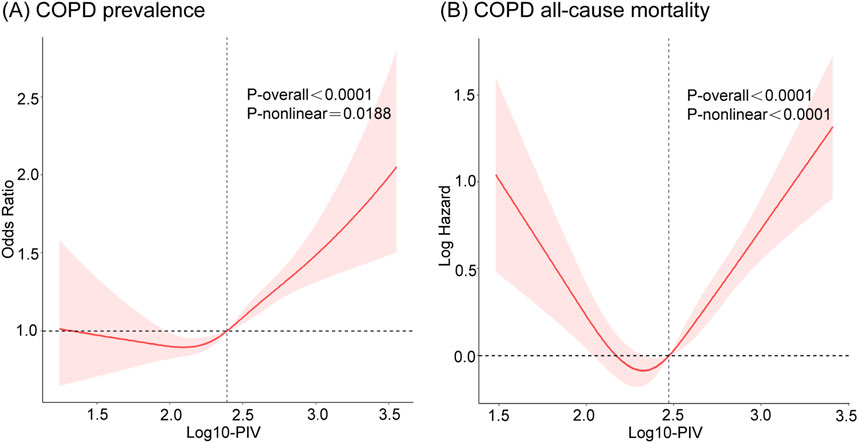
Figure 3. The RCS curves of the associations of PIV with COPD prevalence and COPD all-cause mortality after adjusting all covariates. (A) COPD prevalence; (B) COPD all-cause mortality. COPD, Chronic Obstructive Pulmonary Disease; PIV, pan-immune-inflammation value.
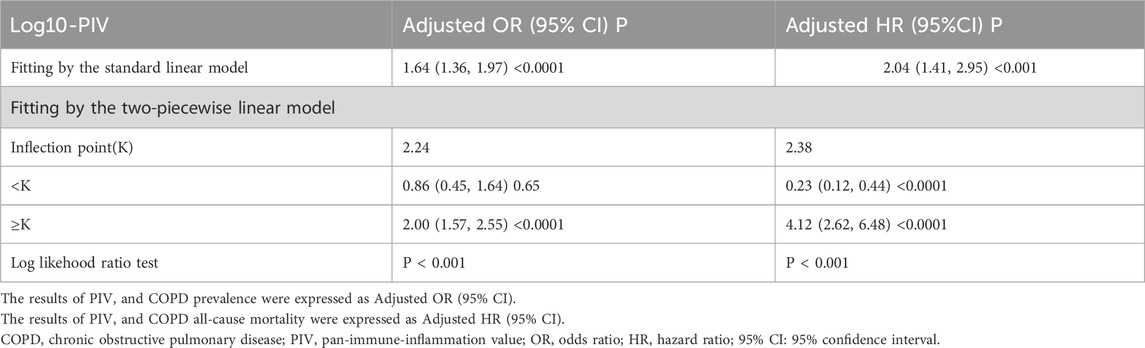
Table 5. Two-piecewise linear regression analyses of PIV and COPD prevalence and COPD all-cause mortality after adjusting all covariates.
3.5 Subgroup analyses and sensitivity analyses
Subgroup analyses and interaction tests were conducted after adjusting for all covariates to further validate the consistency of the association between PIV and COPD and to identify potential differences in specific subgroups. The results showed that the association between PIV and COPD prevalence remained unchanged after stratifying the participants by age, sex, marriage, educational attainment, diabetes, hypertension, and CVD (p for interaction >0.05). However, there were significant interactions between PIV and race, smoking status, and BMI. PIV and the risk of COPD prevalence were not strongly associated among non-Hispanic Black (OR = 1.41, 95% CI: 0.99–2.02), but showed stronger associations among Mexican Americans (OR = 4.12, 95% CI: 2.32–7.32), former smokers (OR = 2.80, 95% CI: 2.01–3.89), and those with a BMI classified as underweight (OR = 6.01, 95% CI: 2.14–16.91) (Figure 4). In addition, the results demonstrated no interaction in the association between PIV and COPD all-cause mortality across all subgroups (all p for interaction >0.05), indicating consistent results across subgroups (Figure 5).
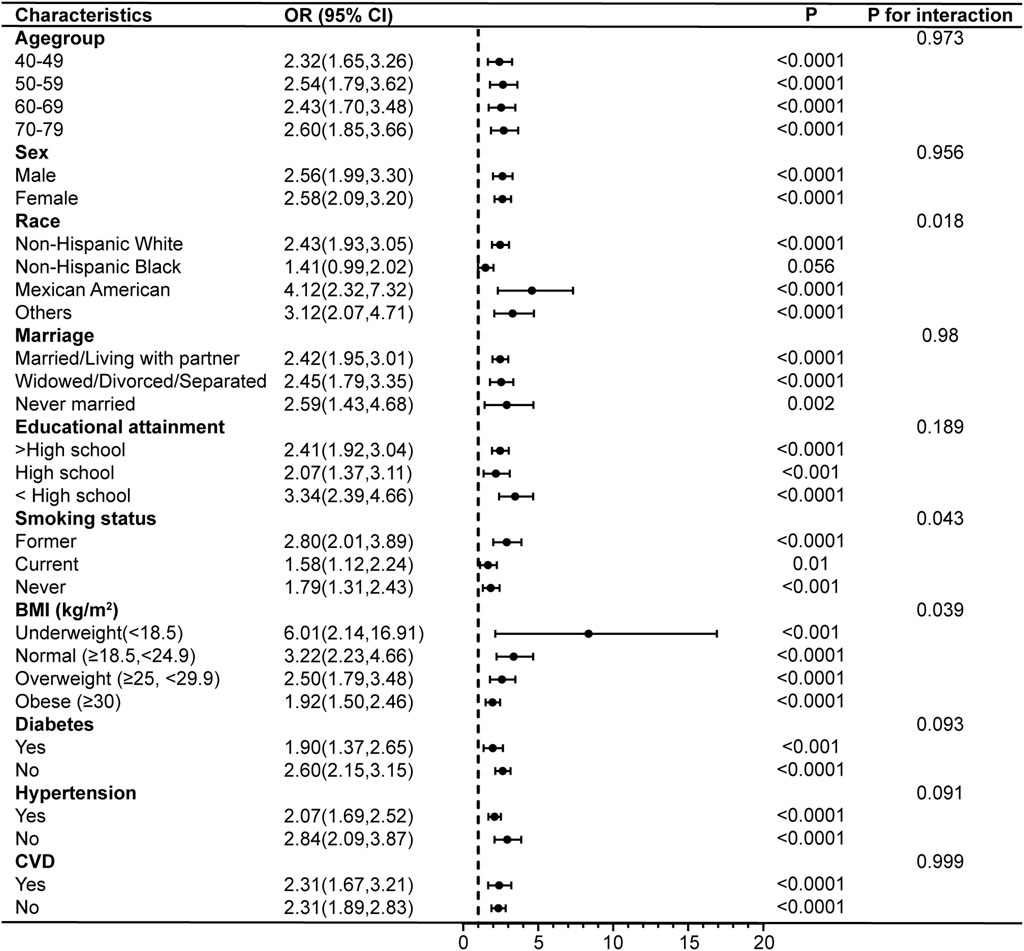
Figure 4. Subgroup analyses of the associations between PIV and COPD prevalence. COPD, Chronic Obstructive Pulmonary Disease; PIV, pan-immune-inflammation value; BMI, body mass index; CVD, cardiovascular disease; OR, odds ratio; 95% CI: 95% confidence interval.
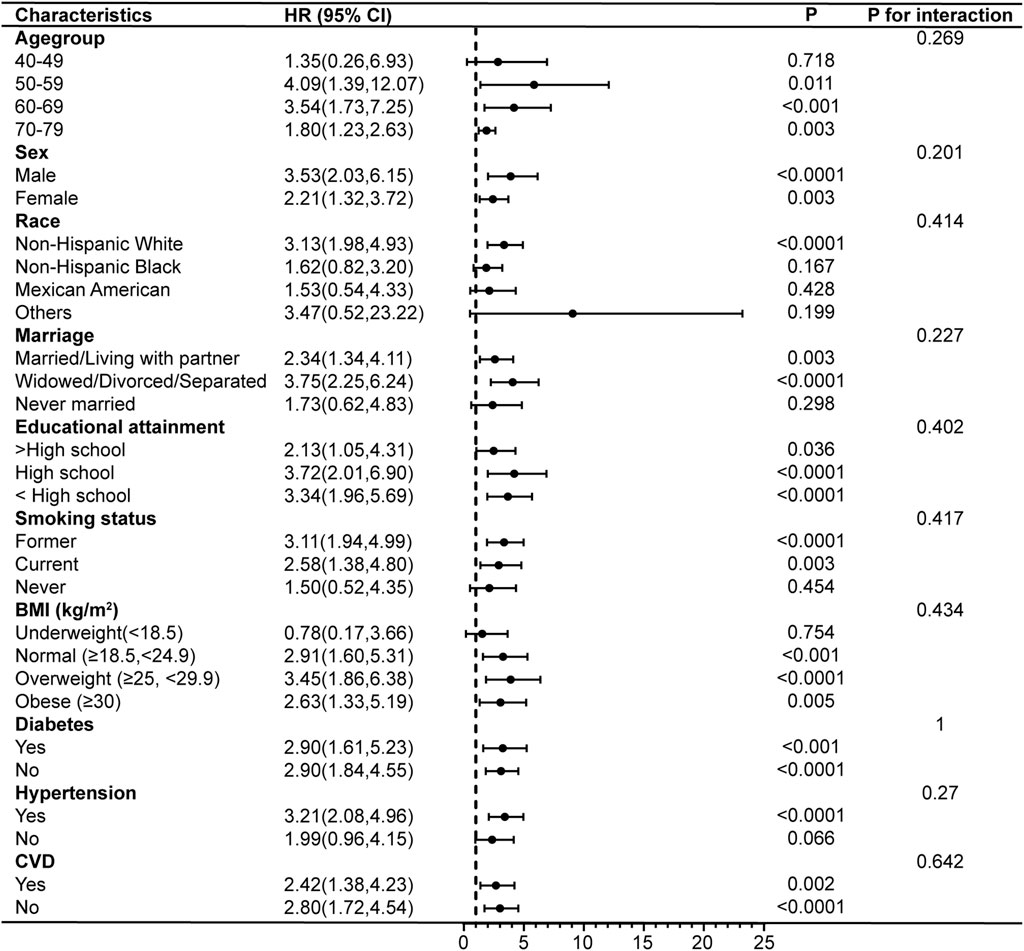
Figure 5. Subgroup analyses of the associations between PIV and COPD all-cause mortality. COPD, Chronic Obstructive Pulmonary Disease; PIV, pan-immune-inflammation value; BMI, body mass index; CVD, cardiovascular disease; HR, hazard ratio; 95% CI: 95% confidence interval.
Sensitivity analyses were performed by excluding individuals with follow-up times of less than 24 months (Supplementary Table S1), and the results of COX regression analyses remained consistent, thereby validating the robustness of our findings.
4 Discussion
To the best of our knowledge, this study is the first to elucidate the associations between PIV and COPD prevalence and all-cause mortality in a representative national sample. We included eligible participants from 1999 to 2018 in the NHANES database, and the study yielded the following main findings: 1) PIV was positively correlated with both COPD prevalence and all-cause mortality, with this relationship remaining significant in fully adjusted models. 2) PIV exhibited a J-shaped correlation with COPD prevalence and a U-shaped correlation with all-cause mortality. This suggests that an increase in PIV is significantly associated with higher COPD prevalence within certain ranges, whereas COPD all-cause mortality initially decreased and subsequently increased with rising PIV levels. 3) Subgroup analyses demonstrated that the association between PIV and COPD prevalence was influenced by race, smoking status, and BMI. This association was not significant among non-Hispanic Black individuals but was stronger in Mexican Americans, former smokers, and those with underweight BMI. However, the association between PIV and COPD all-cause mortality remained consistent across all subgroups and was unaffected by common demographic factors, lifestyle, and underlying conditions. These results could facilitate the clinical development of PIV as a more comprehensive composite indicator for assessing COPD diagnosis, prognosis, and the identification of high-risk COPD populations.
PIV combines four major peripheral blood immune cell types: neutrophils, platelets, monocytes, and lymphocytes, and is considered a novel and more comprehensive indicator of systemic inflammation. Previous research has primarily focused on cancer patients. For instance, a systematic review and meta-analysis of 15 studies involving 4,942 patients demonstrated that PIV serves as a prognostic biomarker for both overall survival and progression-free survival in cancer patients (Guven et al., 2022). Similar results were reported in another systematic review and meta-analysis focusing on colon cancer (Yang et al., 2022). In pulmonary tumors, PIV has served as a predictive biomarker for non-small cell lung cancer (Putzu et al., 2018) and as an indicator of pathological complete response and clinical prognosis in patients undergoing neoadjuvant immunotherapy for non-small cell lung cancer (Zhai et al., 2023). Recently, the prognostic value of PIV in non-cancerous diseases has been recognized. For example, PIV has been regarded as a prognostic indicator for outcomes in patients with ST-segment elevation myocardial infarction and non-traumatic subarachnoid hemorrhage (Murat et al., 2023; Huang et al., 2023b). In pulmonary diseases, PIV has been viewed both as an indicator for assessing the severity of COVID-19 and the necessity of ICU admission (Hamad et al., 2022), and as a prognostic indicator for adverse outcomes in patients with idiopathic pulmonary fibrosis (Zinellu et al., 2020). Recently, a study indicated that AISI upon admission serves as a reliable predictor of mortality in COVID-19 patients with COPD, with higher AISI values correlating with lower survival rates (Hosseninia et al., 2023). In our study, COPD participants exhibited higher PIV levels compared to non-COPD participants. The highest tertile of PIV was associated with the highest prevalence and all-cause mortality. Logistic regression and COX regression revealed that high PIV levels are independent risk factors for both COPD prevalence and all-cause mortality. These findings are consistent with previous studies, further confirming the clinical utility of PIV in COPD.
Our research confirmed a positive correlation between PIV and the risk of COPD prevalence and all-cause mortality. However, the underlying pathological and physiological mechanisms remain unclear. Current consensus suggests that the pathogenesis of COPD primarily involves inflammatory mechanisms, protease-antiprotease imbalance, and oxidative stress from various sources, collectively contributing to the development of small airway and emphysematous lesions, which result in the characteristic persistent airflow limitation of COPD (Mirza et al., 2018). From the perspective of PIV, neutrophils are the most abundant cells in circulation and are the first to be recruited to the site of inflammation (Sadiku et al., 2021). The activation and degranulation of neutrophils release various destructive enzymes, including neutrophil elastase (NE), matrix metalloproteinase (MMP), proteinase 3 (PR3), and cathepsin G (Cat G), leading to lung tissue damage (Chalmers et al., 2023; Jasper et al., 2019). NE stimulates toll-like receptors (TLRs) on epithelial cells, resulting in the upregulation and release of cytokines, increased production and secretion of airway mucoproteins, excessive mucus secretion, and subsequent airway obstruction (Voynow et al., 2020; Hao et al., 2020). Additionally, NE upregulates neutrophil chemotactic factors, such as interleukin (IL)-8, thereby amplifying inflammation (Clancy et al., 2018). Compared to NE, PR3 may experience fewer pulmonary inhibitory effects and have a broader radius of activity (Crisford et al., 2018). Platelets are not only important in hemostasis and thrombosis but also play a crucial role in immune and inflammatory regulation (Mallah et al., 2020). Activated platelets produce and release inflammatory mediators, such as IL-1β, recruiting inflammatory cells and driving oxidative stress and chronic inflammation in COPD (Koenen, 2016). Studies have shown that impaired repair and remodeling following lung injury are closely associated with COPD, where hemostatic disorders and chronic inflammation play major roles (Agustí and Hogg, 2019). In COPD patients, however, platelet activation disorders leading to impaired hemostasis hinder lung tissue repair (Liu et al., 2020), thereby promoting the development of COPD. Platelets can also mediate the formation of pulmonary microthrombi. Red blood cells in COPD patients have been observed to undergo deformation, facilitating the translocation of platelets to the vascular wall, which results in adhesion, aggregation, and activation on the vascular wall (Zouaoui Boudjeltia et al., 2021). Furthermore, hypoxia stress and elevated expression of hypoxia-inducible factor (HIF) due to hypoxemia in COPD can stimulate platelets to synthesize plasminogen activator inhibitor (PAI)-1, inducing a prothrombotic state associated with hypoxia (Chaurasia et al., 2019), which further promotes the development of COPD. Monocytes are recruited to the lungs and contribute to the macrophage pool during inflammation, increasing the macrophage population in the airways of COPD patients (Barnes, 2017). Macrophages secrete numerous inflammatory mediators, including IL-1β, tumor necrosis factor-α (TNF-α), IL-8, MMPs, and reactive oxygen species (ROS), which directly or indirectly affect airway structural cells and participate in airway remodeling (Wang et al., 2018a). Studies have found that monocyte-derived macrophages (MDMs) in COPD exhibit defects in phagocytosis and engulfment, leading to excessive cellular inflammation, an ineffective immune response, and ultimately lung injury, potentially associating with the frequency of acute exacerbations of COPD (Bewley et al., 2017; Ryan et al., 2023). Lymphocytes contribute to lung tissue damage in COPD patients (Wang et al., 2018a). Subtypes of CD4+ T cells, specifically Th17 cells, secrete IL-17, which stimulates airway epithelial cells to produce chemokines and other mediators, recruiting and activating neutrophils and macrophages (Pridgeon et al., 2011). Activated CD8+ T cells release cytotoxic mediators, such as perforin, which damage lung tissue structure and accelerate the progression of emphysema (Kobayashi et al., 2013). In addition, CD8+ T cells produce various inflammatory mediators, including interferon-γ (IFN-γ), TNF-α, and IL-4, which may be linked to exacerbations of COPD (Barczyk et al., 2006). These studies demonstrate the intricate biological relationship between neutrophils, platelets, monocytes, and lymphocytes and the pathogenesis of COPD. From an epidemiological perspective, our findings reveal a clear connection between PIV and COPD.
Using RCS analyses, we elucidated the nonlinear relationships between PIV and COPD prevalence and all-cause mortality. The prevalence curve exhibited a J-shaped correlation, and the all-cause mortality curve showed a U-shaped correlation, suggesting that elevated PIV increased the risk of COPD prevalence, while both elevated and reduced PIV increased the risk of all-cause mortality in COPD patients. Currently, there is no precise research elucidating the reasons for these outcomes. We speculated that this might be due to the fact that a low PIV level reflects an individual’s immune deficiency state, whereas a high PIV level indicates an excessive state. Both conditions might lead to immune imbalance in COPD patients and subsequent increase the risk of mortality. This suggests a potential beneficial effect of PIV within a specific range in reducing the risk of COPD prevalence and all-cause mortality.
After conducting subgroup analyses that adjusted for potential confounding factors, the associations between PIV and all-cause mortality in COPD patients remained consistent across different subgroups. However, our results revealed specific differences in the associations between PIV and COPD prevalence among various populations. PIV did not affect the prevalence risk in non-Hispanic Black individuals but showed a stronger correlation in Mexican Americans who were former smokers and had a BMI classified as underweight. These suggest that these individuals might constitute a high-risk population for COPD and require more intensive prevention strategies. Previous studies consistently demonstrate an increased risk of COPD associated with smoking and underweight (Wang et al., 2018b; Li et al., 2020), which aligns with our findings. Research indicates that smoking is a significant etiological factor for COPD, leading to increased production of reactive oxygen species and persistent inflammation even after smoking cessation (Rao et al., 2020). The increased risk of COPD associated with underweight may be related to malnutrition and muscle weakness (Benz et al., 2019; Shaheen and Barker, 1994). Although the interaction mechanisms between race and PIV remain unclear, a recent cohort study showed a lower likelihood of COPD in Black individuals compared to other races (Ejike et al., 2021), which is consistent with our results. However, this study also reported that Black individuals were associated with higher disease severity and acute exacerbation risks (Ejike et al., 2021), suggesting the need for further research to explore potential influencing factors such as race-specific genetics, physical function, and individual socioeconomic status.
Our study has several key strengths. Firstly, this study represents the inaugural investigation into the association between PIV and both COPD prevalence and all-cause mortality, utilizing a nationally representative sample from the United States. This approach enhances the generalizability and applicability of our findings. Secondly, we controlled for numerous potential confounding factors, including demographic information, lifestyle, and comorbidities, thereby enhancing the reliability and representativeness of our results. Thirdly, RCS analyses were applied to identify potential nonlinear relationships and cut-off values, while segmented linear regression analyses were used to more precisely describe the relationship between PIV and COPD. Finally, subgroup analyses with interaction verification and sensitivity analyses validated the robustness of our results and highlighted potential heterogeneity.
However, this study also has limitations. Firstly, our study population comprised individuals aged 40–79 from the United States. This was due to the fact that COPD patients are predominantly over the age of 40 (5). In addition, it was to exclude deaths caused by various factors, such as aging, among individuals older than 80. This may limit the generalizability of the findings to other populations. Secondly, some participants defined as having COPD were prescribed medication, which may have influenced PIV levels. Future research employing more in-depth and refined methodologies is necessary. Thirdly, although we adjusted for various confounding factors, other potential confounders could not be entirely accounted for because of many constraints, including space limitations. Fourthly, we are unable to infer causality between PIV and COPD because of the cross-sectional design of NHANES; therefore, further prospective studies and randomized trials are required to confirm these associations. Finally, the correlation between PIV and COPD prevalence is influenced by race, smoking status, and BMI, requiring further research into these factors to explore potential mechanisms and enhance precision medicine.
In summary, this study found that elevated PIV levels were associated with increased COPD prevalence, particularly among Mexican Americans, former smokers, and individuals with a BMI classified as underweight. This finding may facilitate the identification of at-risk populations and guide more effective management strategies. Additionally, the study revealed that COPD patients with either elevated or reduced PIV levels faced a higher risk of all-cause mortality. These findings provide valuable clues for targeted interventions aimed at reducing COPD prevalence and all-cause mortality and emphasize the potential of PIV as a biomarker.
5 Conclusion
Elevated PIV levels are associated with increased COPD prevalence. COPD patients with either elevated or reduced PIV levels experience higher all-cause mortality. Further large-scale, longitudinal studies are required to corroborate these findings.
Data availability statement
Publicly available datasets were analyzed in this study. This data can be found here: https://www.cdc.gov/nchs/nhanes/index.htm.
Ethics statement
The studies involving humans were approved by National Center for Health Statistics Research Ethics Review Board. The studies were conducted in accordance with the local legislation and institutional requirements. The participants provided their written informed consent to participate in this study.
Author contributions
SQ: Methodology, Writing–original draft, Project administration, Data curation, Conceptualization. QJ: Visualization, Writing–original draft, Investigation, Formal Analysis, Data curation. YL: Writing–review and editing, Resources, Project administration, Funding acquisition.
Funding
The author(s) declare that financial support was received for the research, authorship, and/or publication of this article. YL was supported by the Natural Science Foundation of Jilin Province of China (task number YDZJ202401172ZYTS).
Acknowledgments
The authors thank all the participants and staff of the National Health and Nutrition Examination Survey and the National Center for Environmental Health for their great contributions.
Conflict of interest
The authors declare that the research was conducted in the absence of any commercial or financial relationships that could be construed as a potential conflict of interest.
Publisher’s note
All claims expressed in this article are solely those of the authors and do not necessarily represent those of their affiliated organizations, or those of the publisher, the editors and the reviewers. Any product that may be evaluated in this article, or claim that may be made by its manufacturer, is not guaranteed or endorsed by the publisher.
Supplementary material
The Supplementary Material for this article can be found online at: https://www.frontiersin.org/articles/10.3389/fphys.2024.1440264/full#supplementary-material
References
Agusti A., Bel E., Thomas M., Vogelmeier C., Brusselle G., Holgate S., et al. (2016). Treatable traits: toward precision medicine of chronic airway diseases. Eur. Respir. J. 47 (2), 410–419. Epub 2016/02/02. doi:10.1183/13993003.01359-2015
Agustí A., Hogg J. C. (2019). Update on the pathogenesis of chronic obstructive pulmonary disease. N. Engl. J. Med. 381 (13), 1248–1256. Epub 2019/09/26. doi:10.1056/NEJMra1900475
Agustí A., Vogelmeier C., Faner R. (2020). Copd 2020: changes and challenges. Am. J. physiology Lung Cell. Mol. physiology 319 (5), L879-L883–l83. Epub 2020/09/24. doi:10.1152/ajplung.00429.2020
Barczyk A., Pierzchała W., Kon O. M., Cosio B., Adcock I. M., Barnes P. J. (2006). Cytokine production by bronchoalveolar lavage T lymphocytes in chronic obstructive pulmonary disease. J. allergy Clin. Immunol. 117 (6), 1484–1492. Epub 2006/06/06. doi:10.1016/j.jaci.2006.02.013
Barnes P. J. (2017). Cellular and molecular mechanisms of asthma and copd. Clin. Sci. Lond. Engl. 1979 131 (13), 1541–1558. Epub 2017/07/01. doi:10.1042/cs20160487
Benz E., Trajanoska K., Lahousse L., Schoufour J. D., Terzikhan N., De Roos E., et al. (2019). Sarcopenia in copd: a systematic review and meta-analysis. Eur. Respir. Rev. official J. Eur. Respir. Soc. 28 (154), 190049. Epub 2019/11/15. doi:10.1183/16000617.0049-2019
Bewley M. A., Preston J. A., Mohasin M., Marriott H. M., Budd R. C., Swales J., et al. (2017). Impaired mitochondrial microbicidal responses in chronic obstructive pulmonary disease macrophages. Am. J. Respir. Crit. care Med. 196 (7), 845–855. Epub 2017/05/31. doi:10.1164/rccm.201608-1714OC
CDC (2024a). National health and nutrition examination survey: nchs research Ethics review board (erb) approval. Available at: https://www.cdc.gov/nchs/nhanes/irba98.htm (Accessed April 22, 2024).
CDC (2024b). National health and nutrition examination survey. Available at: https://www.cdc.gov/nchs/nhanes/index.htm (Accessed April 22, 2024).
Chalmers J. D., Kettritz R., Korkmaz B. (2023). Dipeptidyl peptidase 1 inhibition as a potential therapeutic approach in neutrophil-mediated inflammatory disease. Front. Immunol. 14, 1239151. doi:10.3389/fimmu.2023.1239151
Chaurasia S. N., Kushwaha G., Kulkarni P. P., Mallick R. L., Latheef N. A., Mishra J. K., et al. (2019). Platelet hif-2α promotes thrombogenicity through pai-1 synthesis and extracellular vesicle release. Haematologica 104 (12), 2482–2492. Epub 2019/04/21. doi:10.3324/haematol.2019.217463
Chen S., Kuhn M., Prettner K., Yu F., Yang T., Bärnighausen T., et al. (2023). The global economic burden of chronic obstructive pulmonary disease for 204 countries and territories in 2020-50: a health-augmented macroeconomic modelling study. Lancet Glob. health 11 (8), e1183–e1193. Epub 2023/07/21. doi:10.1016/s2214-109x(23)00217-6
Christenson S. A., Smith B. M., Bafadhel M., Putcha N. (2022). Chronic obstructive pulmonary disease. Lancet London, Engl. 399 (10342), 2227–2242. Epub 2022/05/10. doi:10.1016/s0140-6736(22)00470-6
Clancy D. M., Sullivan G. P., Moran H. B. T., Henry C. M., Reeves E. P., McElvaney N. G., et al. (2018). Extracellular neutrophil proteases are efficient regulators of il-1, il-33, and il-36 cytokine activity but poor effectors of microbial killing. Cell. Rep. 22 (11), 2937–2950. Epub 2018/03/15. doi:10.1016/j.celrep.2018.02.062
Crisford H., Sapey E., Stockley R. A. (2018). Proteinase 3; a potential target in chronic obstructive pulmonary disease and other chronic inflammatory diseases. Respir. Res. 19 (1), 180. Epub 2018/09/22. doi:10.1186/s12931-018-0883-z
Ejike C. O., Woo H., Galiatsatos P., Paulin L. M., Krishnan J. A., Cooper C. B., et al. (2021). Contribution of individual and neighborhood factors to racial disparities in respiratory outcomes. Am. J. Respir. Crit. care Med. 203 (8), 987–997. Epub 2020/10/03. doi:10.1164/rccm.202002-0253OC
Feng J., Wang L., Yang X., Chen Q., Cheng X. (2023). Clinical utility of preoperative pan-immune-inflammation value (piv) for prognostication in patients with esophageal squamous cell carcinoma. Int. Immunopharmacol. 123, 110805. Epub 2023/08/18. doi:10.1016/j.intimp.2023.110805
GOLD (2024). Global strategy for prevention. Diagnosis Manag. Copd 2024 Rep. Available at: https://goldcopd.org/2024-gold-report/ (Accessed April 22, 2024).
Guven D. C., Sahin T. K., Erul E., Kilickap S., Gambichler T., Aksoy S. (2022). The association between the pan-immune-inflammation value and cancer prognosis: a systematic review and meta-analysis. Cancers 14 (11), 2675. Epub 2022/06/11. doi:10.3390/cancers14112675
Hamad D. A., Aly M. M., Abdelhameid M. A., Ahmed S. A., Shaltout A. S., Abdel-Moniem A. E., et al. (2022). Combined blood indexes of systemic inflammation as a mirror to admission to intensive care unit in covid-19 patients: a multicentric study. J. Epidemiol. Glob. health 12 (1), 64–73. Epub 2021/12/15. doi:10.1007/s44197-021-00021-5
Hao W., Li M., Zhang Y., Zhang C., Wang P. (2020). Severity of chronic obstructive pulmonary disease with 'exacerbator with emphysema phenotype' is associated with potential biomarkers. Postgrad. Med. J. 96 (1131), 28–32. Epub 2019/08/04. doi:10.1136/postgradmedj-2019-136599
Hosseninia S., Ghobadi H., Garjani K., Hosseini S. A. H., Aslani M. R. (2023). Aggregate index of systemic inflammation (aisi) in admission as a reliable predictor of mortality in copd patients with covid-19. BMC Pulm. Med. 23 (1), 107. Epub 2023/04/03. doi:10.1186/s12890-023-02397-5
Huang Q., Li S., Wan J., Nan W., He B. (2023a). Association between ethylene oxide exposure and prevalence of copd: evidence from nhanes 2013-2016. Sci. total Environ. 885, 163871. Epub 2023/05/07. doi:10.1016/j.scitotenv.2023.163871
Huang Y. W., Zhang Y., Li Z. P., Yin X. S. (2023b). Association between a four-parameter inflammatory index and all-cause mortality in critical ill patients with non-traumatic subarachnoid hemorrhage: a retrospective analysis of the mimic-iv database (2012-2019). Front. Immunol. 14, 1235266. Epub 2023/11/08. doi:10.3389/fimmu.2023.1235266
Jasper A. E., McIver W. J., Sapey E., Walton G. M. (2019). Understanding the role of neutrophils in chronic inflammatory airway disease. F1000Research 8, F1000 Faculty Rev-557. Epub 2019/05/10. doi:10.12688/f1000research.18411.1
Jin C., Li X., Luo Y., Zhang C., Zuo D. (2024). Associations between pan-immune-inflammation value and abdominal aortic calcification: a cross-sectional study. Front. Immunol. 15, 1370516. Epub 2024/04/12. doi:10.3389/fimmu.2024.1370516
Kobayashi S., Fujinawa R., Ota F., Kobayashi S., Angata T., Ueno M., et al. (2013). A single dose of lipopolysaccharide into mice with emphysema mimics human chronic obstructive pulmonary disease exacerbation as assessed by micro-computed tomography. Am. J. Respir. Cell. Mol. Biol. 49 (6), 971–977. Epub 2013/07/05. doi:10.1165/rcmb.2013-0074OC
Koenen R. R. (2016). The prowess of platelets in immunity and inflammation. Thrombosis haemostasis 116 (4), 605–612. Epub 2016/07/08. doi:10.1160/th16-04-0300
Li J., Zhu L., Wei Y., Lv J., Guo Y., Bian Z., et al. (2020). Association between adiposity measures and copd risk in Chinese adults. Eur. Respir. J. 55 (4), 1901899. Epub 2020/01/26. doi:10.1183/13993003.01899-2019
Liu Y., Liu H., Li C., Ma C., Ge W. (2020). Proteome profiling of lung tissues in chronic obstructive pulmonary disease (copd): platelet and macrophage dysfunction contribute to the pathogenesis of copd. Int. J. chronic Obstr. Pulm. Dis. 15, 973–980. Epub 2020/05/23. doi:10.2147/copd.s246845
Liu Z., Zeng H., Zhang H. (2024). Association of the oxidation balance score with the prevalence of chronic obstructive pulmonary disease from the nhanes 2007-2012: a large-scale cross-sectional study. Heart and lung J. Crit. care 65, 84–92. Epub 2024/03/07. doi:10.1016/j.hrtlng.2024.02.005
Mallah H., Ball S., Sekhon J., Parmar K., Nugent K. (2020). Platelets in chronic obstructive pulmonary disease: an update on pathophysiology and implications for antiplatelet therapy. Respir. Med. 171, 106098. Epub 2020/08/11. doi:10.1016/j.rmed.2020.106098
Mirza S., Clay R. D., Koslow M. A., Scanlon P. D. (2018). Copd guidelines: a review of the 2018 gold report. Mayo Clin. Proc. 93 (10), 1488–1502. Epub 2018/10/06. doi:10.1016/j.mayocp.2018.05.026
Murat B., Murat S., Ozgeyik M., Bilgin M. (2023). Comparison of pan-immune-inflammation value with other inflammation markers of long-term survival after st-segment elevation myocardial infarction. Eur. J. Clin. investigation 53 (1), e13872. Epub 2022/09/14. doi:10.1111/eci.13872
Pridgeon C., Bugeon L., Donnelly L., Straschil U., Tudhope S. J., Fenwick P., et al. (2011). Regulation of il-17 in chronic inflammation in the human lung. Clin. Sci. Lond. Engl. 1979 120 (12), 515–524. Epub 2011/01/07. doi:10.1042/cs20100417
Putzu C., Cortinovis D. L., Colonese F., Canova S., Carru C., Zinellu A., et al. (2018). Blood cell count indexes as predictors of outcomes in advanced non-small-cell lung cancer patients treated with nivolumab. Cancer Immunol. Immunother. CII 67 (9), 1349–1353. Epub 2018/06/28. doi:10.1007/s00262-018-2182-4
Rao W., Wang S., Duleba M., Niroula S., Goller K., Xie J., et al. (2020). Regenerative metaplastic clones in copd lung drive inflammation and fibrosis. Cell. 181 (4), 848–864. Epub 2020/04/17. doi:10.1016/j.cell.2020.03.047
Ryan E. M., Sadiku P., Coelho P., Watts E. R., Zhang A., Howden A. J. M., et al. (2023). Nrf2 activation reprograms defects in oxidative metabolism to restore macrophage function in chronic obstructive pulmonary disease. Am. J. Respir. Crit. care Med. 207 (8), 998–1011. Epub 2023/02/02. doi:10.1164/rccm.202203-0482OC
Sadiku P., Willson J. A., Ryan E. M., Sammut D., Coelho P., Watts E. R., et al. (2021). Neutrophils fuel effective immune responses through gluconeogenesis and glycogenesis. Cell. metab. 33 (2), 411–423.e4. Epub 2020/12/12. doi:10.1016/j.cmet.2020.11.016
Shaheen S., Barker D. J. (1994). Early lung growth and chronic airflow obstruction. Thorax 49 (6), 533–536. Epub 1994/06/01. doi:10.1136/thx.49.6.533
Voynow J. A., Zheng S., Kummarapurugu A. B. (2020). Glycosaminoglycans as multifunctional anti-elastase and anti-inflammatory drugs in cystic fibrosis lung disease. Front. Pharmacol. 11, 1011. Epub 2020/08/01. doi:10.3389/fphar.2020.01011
Wang C., Xu J., Yang L., Xu Y., Zhang X., Bai C., et al. (2018b). Prevalence and risk factors of chronic obstructive pulmonary disease in China (the China pulmonary health [cph] study): a national cross-sectional study. Lancet London, Engl. 391 (10131), 1706–1717. Epub 2018/04/14. doi:10.1016/s0140-6736(18)30841-9
Wang Y., Xu J., Meng Y., Adcock I. M., Yao X. (2018a). Role of inflammatory cells in airway remodeling in copd. Int. J. chronic Obstr. Pulm. Dis. 13, 3341–3348. Epub 2018/10/24. doi:10.2147/copd.s176122
World Health Organization (2024). Chronic obstructive pulmonary disease. Copd. Available at: https://www.who.int/news-room/fact-sheets/detail/chronic-obstructive-pulmonary-disease- (Accessed April 22, 2024).
Yang X. C., Liu H., Liu D. C., Tong C., Liang X. W., Chen R. H. (2022). Prognostic value of pan-immune-inflammation value in colorectal cancer patients: a systematic review and meta-analysis. Front. Oncol. 12, 1036890. Epub 2023/01/10. doi:10.3389/fonc.2022.1036890
Zhai W. Y., Duan F. F., Lin Y. B., Lin Y. B., Zhao Z. R., Wang J. Y., et al. (2023). Pan-immune-inflammatory value in patients with non-small-cell lung cancer undergoing neoadjuvant immunochemotherapy. J. Inflamm. Res. 16, 3329–3339. Epub 2023/08/14. doi:10.2147/jir.s418276
Zinellu A., Paliogiannis P., Sotgiu E., Mellino S., Mangoni A. A., Zinellu E., et al. (2020). Blood cell count derived inflammation indexes in patients with idiopathic pulmonary fibrosis. Lung 198 (5), 821–827. Epub 2020/08/28. doi:10.1007/s00408-020-00386-7
Keywords: COPD, pan-immune-inflammation value, NHANES, cross-sectional study, cohort study
Citation: Qiu S, Jiang Q and Li Y (2024) The association between pan-immune-inflammation value and chronic obstructive pulmonary disease: data from NHANES 1999–2018. Front. Physiol. 15:1440264. doi: 10.3389/fphys.2024.1440264
Received: 24 June 2024; Accepted: 24 September 2024;
Published: 07 October 2024.
Edited by:
Pooja Shivshankar, University of Texas Health Science Center at Houston, United StatesReviewed by:
Ran Wang, Anhui Medical University, ChinaMarie-Francoise Joelle Doursout, University of Texas Health Science Center at Houston, United States
Copyright © 2024 Qiu, Jiang and Li. This is an open-access article distributed under the terms of the Creative Commons Attribution License (CC BY). The use, distribution or reproduction in other forums is permitted, provided the original author(s) and the copyright owner(s) are credited and that the original publication in this journal is cited, in accordance with accepted academic practice. No use, distribution or reproduction is permitted which does not comply with these terms.
*Correspondence: Yang Li, bGlfeWFuZzk5QGpsdS5lZHUuY24=