- 1College of Physical Education, Shenzhen University, Shenzhen, China
- 2College of Physical Education, Southwest University, Chongqing, China
Objective: This study investigated the relationship between executive function and prefrontal cortex oxygenation during exercise in young adults with different Cardiorespiratory fitness (CRF) levels.
Methods: A total of 28 amateur runners (n = 14) and sedentary college students (n = 14) were recruited. The maximum oxygen uptake estimated for the sub-maximal intensity run (4.97 miles/h) was used to indicate the different CRF levels. After 1 week, participants must complete the Stroop and 2-Back tasks in silence while performing moderate-intensity exercise. Using 19-channel functional near-infrared spectroscopic (fNIRS) to examine changes in prefrontal cortex oxyhemoglobin.
Results: There was no significant difference in the correctness of the Stroop and 2-Back tasks between the two groups during exercise, but the amateur runner group showed an acceleration in reaction time. fNIRS results showed that during the exercise 2-Back task, the left dorsolateral prefrontal cortex oxyhemoglobin was higher in the amateur runner group than in the sedentary group.
Conclusion: Executive function during exercise was similarly improved in participants with better fitness, suggesting that CRF provides an excellent metabolic reserve and directed allocation for cognitive tasks during exercise.
1 Introduction
Running is the world’s most widely participated sport. In 2021, approximately 49 million people in the United States will have run or jogged at least once (Statista, 2023). Running reduces all-cause mortality risk and improves mental health, such as cognitive function (Hillman et al., 2008; Lee et al., 2014) and even leads to beneficial plastic changes in the brain (Cao et al., 2021). In addition, running is a complex physical activity that involves multitasking in many scenarios. For example, endurance athletes’ coping with fatigue during the competition (Behrens et al., 2023), and implementation of and decision-making about speeding strategies all require significant cognitive function involvement (Hyland-Monks et al., 2018). Simultaneously maintaining or sustaining motor and cognitive tasks is important in human life. It is necessary for daily, physical, vocational, and educational activities (Behrens et al., 2023).
Cognitive functions are the processes associated with specific brain areas (Pessoa, 2008). As a “higher” cognitive function, the executive function (EF) controls and regulates “lower” cognitive processes and goal-directed, future-oriented behavior (Alvarez and Emory, 2006). The brain’s prefrontal cortex (PFC) activity is the main driver of EF (Alvarez and Emory, 2006). Compared with other cognitive domains, EF appears to be more susceptible to the positive effects of various forms of movement (Spirduso, 1975; Schroeter et al., 2002; Tomporowski, 2003; Woo and Sharps, 2003; Hillman et al., 2006). For example, after acute exercise, EF and cortical activity performance in relevant brain areas can be improved (Byun et al., 2014). And during exercise, the response time to perform functions at low and moderate intensities becomes faster compared to the calm state (Davranche et al., 2015; Giles et al., 2018; Cantelon and Giles, 2021).
However, EF is susceptible to cardiorespiratory fitness (CRF). CRF reflects the integrated ability to transport oxygen from the atmosphere to the mitochondria to perform physical work (Ross et al., 2016). Studies have found that exercise-induced improvements in CRF are associated with better EF (Scott et al., 2016; Goenarjo et al., 2021). However, we know little about the performance of EF in dual-task running exercise states in different CRF participants during running exercise. Giles et al. (2018) found that endurance athletes improved response times to Stroop tasks during moderate-intensity exercise and observed a reduction in PFC and task-related oxy-Hb. Most participants currently focused on exercise—EF dual-task studies are physically active young adults, and there is a lack of comparisons between people at different levels of health (Cantelon and Giles, 2021).
Therefore, this study’s main aim was to explore EF’s performance during running exercises in adults with different CRFs. This study also uses functional near-infrared spectroscopic imaging (fNIRS) to observe cortex activity concerning EF tasks. fNIRS is a promising neuroimaging tool relatively insensitive to motion artifacts (Scholkmann et al., 2014; Ludyga et al., 2019; Yan et al., 2020). Portable fNIRS can be used in many complex scenarios and is widely used in sports science (Tempest and Reiss, 2019; Wu et al., 2022). This study hypothesizes that 1) only participants with high CRF will improve EF response time during exercise compared to a single task, and 2) participants with high CRF will have higher PFC activity during the running-EF task.
2 Materials and methods
2.1 Participants
We planned to recruit different groups to represent different CRF levels. The sample size calculation was first performed. The sample size was estimated using G-power 3.1 based on a previous study by Goenarjo et al. (2020b). The results showed that a minimum of twenty participants were needed. We expanded the sample size. Twenty-eight amateur runners and sedentary regular college students were recruited (amateur runners: n = 14, sedentary group: n = 14). The amateur runners were college athletes with an average of over 4 years of training. The International Physical Activity Questionnaire--Short Form (IPAQ-SF) screened the sedentary group. Each sedentary member was sedentary for no less than 8 h per day. Other basic physical indicators are shown in Table 1. In addition, all subjects met the following inclusion criteria: 1) right-handed; 2) no sports injuries within 6 months; 3) normal vision and no color blindness; 4) no neurological disorders such as head trauma or brain injury; 5) not using the antidepressant/anxiety medication; and 6) not required to exercise according to a doctor’s prescription. The Ethics Committee of Shenzhen University reviewed and approved this experiment (PN-202300017), and all the participants signed an informed consent form before participation.
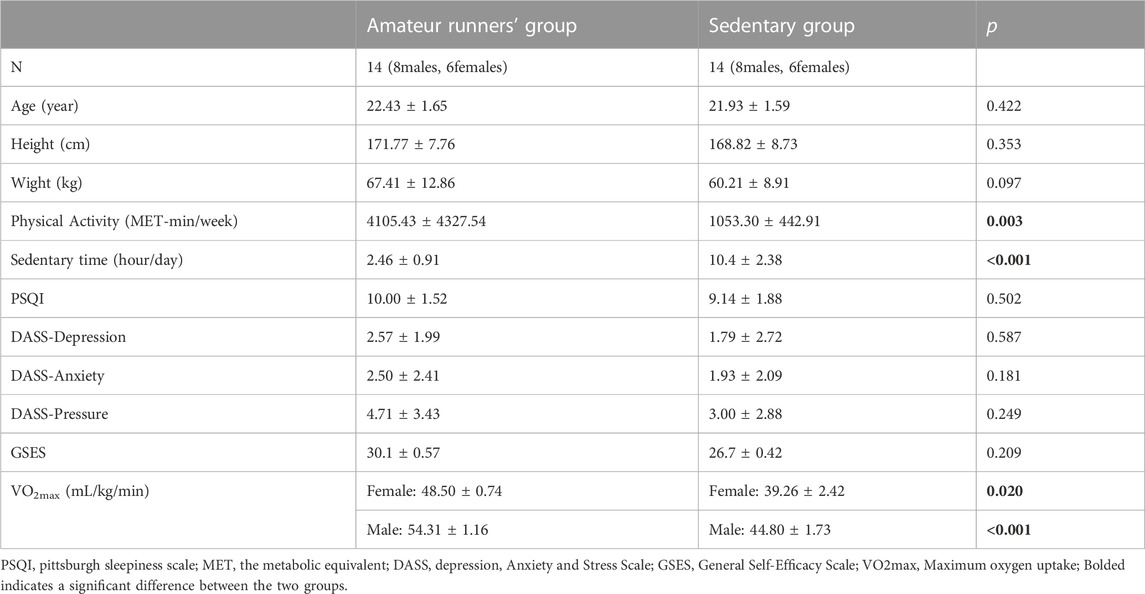
TABLE 1. Basic information for participants at different fitness levels (Mean ± Standard deviation).
2.2 Study design
Participants were tested twice at different time points. They completed an informed consent form and a basic information questionnaire during the first session. The International Physical Activity Questionnaire Short Form was used to screen participants for sedentary time (Craig et al., 2003). The Depression, Anxiety, and Stress Scale (DASS) (Brown et al., 1997), the Pittsburgh Sleepiness Scale (PSQI) (Buysse et al., 1989), and the General Self-Efficacy Scale (GSES) (Cheung and Sun, 1999) were used to evaluate their psychological wellbeing. Participants then completed a cardiorespiratory fitness test by jogging on a treadmill at a given intensity (4.97 miles/h). In the second session, participants wore the fNIRS and performed the Stroop and 2-Back tasks in a quiet and moderate-intensity exercise state for behavioral and fNIRS data collection. The two sessions occurred >7 days apart to avoid the effects of fatigue. During this time, participants were instructed to maintain their daily routine and avoid engaging in extreme physical activity. After 7 days, the experimenter-initiated contact with subjects to participate in the second session. Participants were told to abstain from alcohol or beverages containing caffeine for 24 h before each test.
2.3 Measurement of CRF
As this study may have included an unfit group, we used a more conservative sub-maximal intensity run of a given intensity to estimate maximal oxygen uptake (VO2max). The George prediction method is considered reliable for predicting VO2max (George et al., 1993). The method has many advantages such as low cost, no need to reach exercise limits, and no need for trained personnel. The test is determined by applying a constant load of 8 km/h (4.97 miles/h) on a treadmill. A heart rate belt (Polar Team System, Kempele, Finland) is used to check the heart rate. During the test, the runner starts at a self-selected pace, gradually accelerates to a set speed, and stops the test when a steady-state heart rate (SSHR) has been reached for no more than 3 min. The exercise heart rate is considered a steady state when consecutive heart rates (30-s interval) differ by ≤ 3 beats/min. VO2max is estimated using the following formula:
2.4 Cognitive tasks
The Stroop task and the 2-Back task were used to evaluate the execution function.
2.4.1 Stroop task
For this experiment, we chose a computer-based color-word matching Stroop test with an event-related design implemented using E-Prime 3.0 software (Psychology Software Tools Inc., United States). Many neuroimaging techniques, including fNIRS, have used this classic measure to evaluate cognitive function in the prefrontal cortex (MacLeod, 1991). In the task, subjects observed the names of colors presented in different ink colors. In the inconsistent condition, the color names matched other ink colors (e.g., red words written in green ink). In the neutral condition, the ink color of the color name matched the meaning of the word itself (e.g., a red word written in red ink). A computer screen with a black background randomly shows letters of different colors. The participant must press the keyboard’s corresponding button as fast as possible (F for neutral, J for inconsistent). All words for this task were in Chinese, which consisted of 20 incongruent and 20 congruent experiments. Each word had a presentation time of 0.5 s, and the participant had to choose within 2 s, or it was considered an error. Subjects had a 10-s break between words (Figure 1).
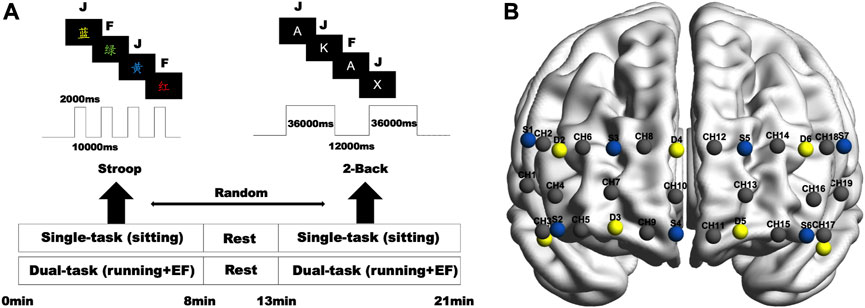
FIGURE 1. (A) Experimental procedure and task protocols. (B) fNIRS probe settings (blue = source; yellow = detector; gray = active channel).
2.4.2 2-Back task
To evaluate working memory, we used a block-based 2-Back task. In each task block, an English capitalized consonant letter appeared in the middle on a black screen for 2 s with an inter-trial interval of 0.5 s. Participants are required to respond when the letter is the same as the previous letters. The participant presses F for unanimous and J for inconsistent. The output records response times and correctness rates. There are five blocks throughout 2-Back, each consisting of 12 trials lasting 36 s, with a 12-s interval between blocks. The percentage of correct responses and the response time are recorded (Figure 1).
2.5 Cognitive tasks during quiet and exercise
Behavioral experiments are conducted in a dark environment without interference from other moving objects. In the second session, participants randomly selected the Stroop task and the 2-Back task in two different states. Each cognitive task lasted approximately 8 min. Participants needed at least 30 s in the exercise state to reach a given heart rate before performing the 1-min cognitive task. There is a 5-min break in between. The dual-task behavior experiment takes place on a treadmill. During exercise, each subject wears a Polar heart rate detector to limit the intensity of the exercise. The experimenter controlled the treadmill’s speed according to the subject’s heart rate. The monitor was set approximately 1.2 m in front of the subject’s body and held in place with a special bracket. Participants held two extended numeric keypads (corresponding to F and J, respectively) that were long enough for the participants to perform cognitive tasks during the exercise. Before the official start, participants were allowed sufficient time to debug the display and keyboard until they reached the most comfortable position. Initially, participants walked at a self-selected pace and gradually transitioned to jogging. Participants began performing the task when the heart rate reached and stabilized at a moderate intensity (Garber et al., 2011). For the definition of exercise intensity, we adopted the recommendations of the American College of Sports Medicine’s Guidance for Prescribing Exercise. We used 64%–76% percent of maximum heart rate (%HRmax) as moderate exercise intensity (Garber et al., 2011). Maximum heart rate was calculated using the Gellish et al. optimization estimation equation: maximum heart rate = 207–0.7 * age (Gellish et al., 2007). The experimenter adjusted the treadmill’s speed according to the participant’s heart rate range to maintain it at a moderate intensity range.
2.6 fNIRS data acquisition and processing
In the experiments, the fNIRS device uses a multi-channel portable Nirsmart (Danyang Huichuang Medical Equipment Co., Ltd., China) to record changes in the signal of oxyhemoglobin (oxy-Hb). The device uses 7 light sources and 7 detectors with a distance of 3 cm between the light sources and the detectors and contains 19 active channels. Effective coverage of the frontopolar, dorsolateral, and ventral lateral prefrontal areas. Each light source emits light at 760 and 850 nm wavelengths, and all channels are set at 11 Hz. The locations of channels were located using a three-dimensional digitizer. The coordinates of the 19 channels on the Montreal Neurological Institute (MNI) standard brain were imported into the NIRS-SPM toolbox to obtain the distribution probability for each channel (Ye et al., 2009). Projection of MNI coordinates of light sources, probes, and channels determined by a 3D localizer onto the cortical surface of the ICBM152 brain template using MATLAB-based BrainNet (Xia et al., 2013) (Figure 1).
fNIRS data were analyzed using the Homer3 NIRS processing package. First, the raw optical data is converted to optical density data (Huppert et al., 2009). Channels with poor signal quality recorded at the beginning of each session during calibration and determined using the enPruneChannels function were excluded (dRange = 1e-03 1e+04, SNRthresh = 2, SDrange = 0–30). Secondly, the hmrR_ MotionartifactByChannel function (tMotion = 0.5, tMask = 1.0, STDEVthresh = 20, AMPthresh = 5.0) helps identify and exclude headache signals on a channel-by-channel basis to lessen the effects of motion artifacts. Third, perform wavelet-based motion correction (Molavi and Dumont, 2012). The data were converted to oxy-Hb concentrations using a modified Beer-Lambert equation after applying bandpass filters (standard cutoff frequencies of 0.001 and 0.2 Hz). The motion artifacts were then corrected using the MotionCorrectCbs function. After pre-processing, the general linear model calculates a beta estimate indicating the hemodynamic response during the task.
2.7 Statistical analysis
The data were statistically analyzed using SPSS 26.0. Using independent samples t-tests to compare physical and psychological variables between the two groups of participants. ANOVA with two-way (state × group) repeated measures was used to test the null hypothesis. Examine whether there are any main or interaction effects of groups (amateur runners or sedentary group) and state (single task or double task) on task performance. Bonferroni was used to correct the results of post hoc multiple comparisons in behavior. After excluding channels for pretreatment in the fNIRS results, a linear mixed-effects model (LMM) was used to compare differences between factors. Using the false discovery rate (FDR) to correct for multiple comparisons. All data are expressed as mean differences (MD), and effect sizes are reported as partial eta squared (η2) in the ANOVA.
3 Result
3.1 Participant characteristics
Table 1 summarizes the characteristics of the participants. t-test results showed no differences in mental health between the two groups (p > 0.05). Clearly, the amateur runners’ group had significantly greater MET than the sedentary group and less sedentary time than the sedentary group (p < 0.01). In addition, VO2max was higher in amateur runners of different genders compared to the sedentary group. These performances can distinguish between two different groups of CRF.
3.2 Behavioral results
3.2.1 Stroop
All behavioral and cognitive outcomes are presented in Figure 2. For correctness, there were neither inter-subject effects nor interactions [F (1, 26) = 1.530, p = 0.227, η2 = 0.056] among the group [F (1, 26) = 0.077, p = 0.784, η2 = 0.003], task condition [F (1, 26) = 0.268, p = 0.609, η2 = 0.010], and state [F (1, 26) = 0.049, p = 0.826, η2 = 0.002]. For reaction time, the main effects of consistency [F= (1, 26) = 31.333, p < 0.001, η2 = 0.547] and state [F (1, 26) = 10.051, p = 0.004, η2 = 0.279] showed significance. No interaction was found [F (1, 26) = 0.337, p = 0.567, η2 = 0.013]. Posthoc analyses showed that the amateur runners’ group improved in both neutral (MD = −75.35 m, p = 0.033, Bonferroni corrected) and inconsistent (MD = −74.06 m, p = 0.036, Bonferroni corrected) responses (Figure 2).
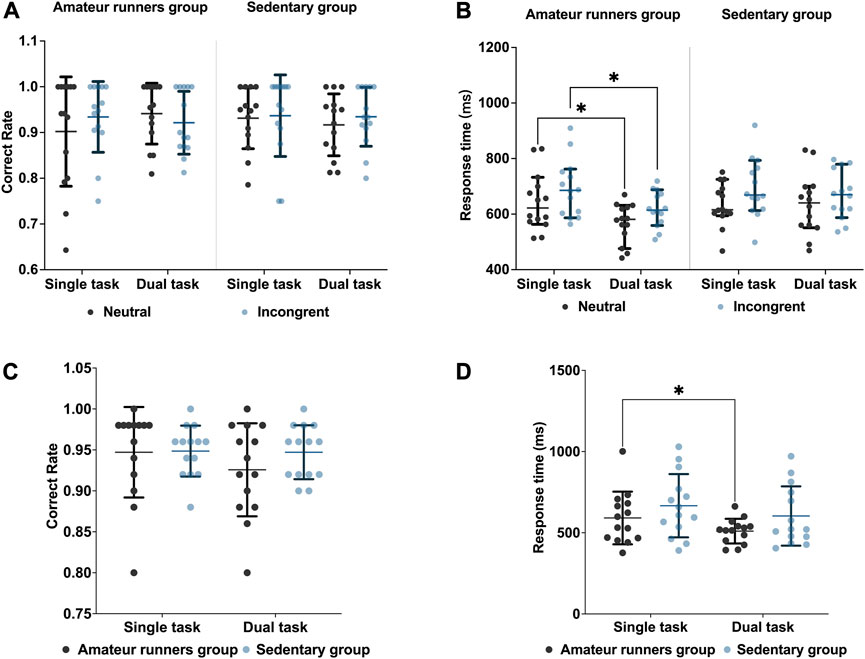
FIGURE 2. Results compared between the two groups: Stroop task: (A) correct rate; (B) response time. 2-Back task: (C) correct rate; (D) response time. Data are expressed as mean ± standard error. * Indicates a significant difference compared to the resting state. p < 0.05. Bonferroni corrected.
3.2.2 2-Back
For correctness in the 2-Back working memory task, the results of the repeated measures ANOVA showed neither a main effect nor an interaction between group [F (1,26) = 0.589, p = 0.450, η2 = 0.022] and state [F (1, 26) = 1.724, p = 0.201, η2 = 0.062]. In comparison, for the response, the state played a significant main effect [F (1, 26) = 6.378, p = 0.018, η2 = 0.197]. Post-hoc analysis showed that only the amateur runners’ group had faster reaction times during dual-task (MD = −93.12 m, p = 0.043, Bonferroni corrected) (Figure 2).
3.3 fNIRS result
3.3.1 Stroop
For the Stroop task, the main effect of state in the LMM results showed higher oxy-Hb in the amateur runner group in the single task state in CH3 (MD = −0.617, p = 0.006, FDR corrected), 17 (MD = −0.723, p = 0.008, FDR corrected). The sedentary group had significantly lower oxy-Hb levels in CH19 (MD = −0.820, p = 0.002, FDR corrected) and CH17(MD = −0.390, p = 0.030, FDR corrected) compared to the single-task state. Among the main effects of the groups, the amateur runners’ group had significantly higher oxy-Hb in CH19 in the single task state compared with the sedentary group (MD = 0.321, p = 0.025, FDR corrected). However, we did not find significant differences between the two groups in the dual task state. (Figure 3).
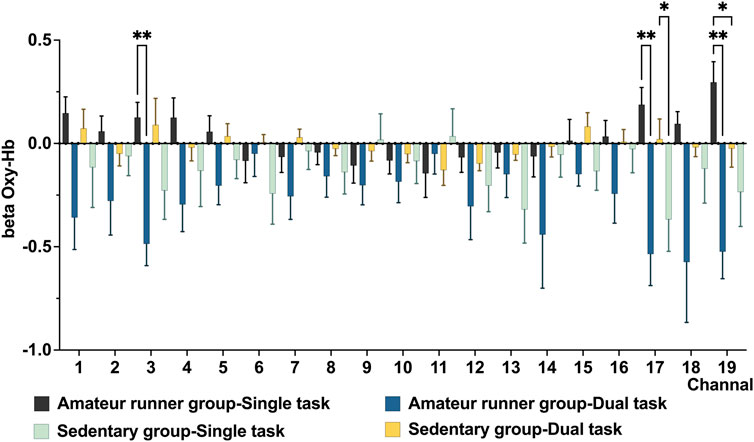
FIGURE 3. Compares prefrontal oxygenation in the Stroop task between the high-fit and low-fit groups in the quiet and exercise states. Data are presented as mean ± standard error. *: p < 0.05, **: p < 0.01, FDR corrected.
3.3.2 2-Back
For the 2-back task, the main effect of state in the LMM showed that the amateur runners’ group had significantly lower oxy-Hb during dual-task in CH11 (MD = −0.08, p = 0.005, FDR corrected), and compared with the single task state, CH15 (MD = −0.05, p = 0.018, FDR corrected) had significantly lower oxygenated hemoglobin levels. In addition, compared with the single task state, the oxy-Hb levels in the sedentary group during exercise were significantly lower in CH15 (MD = −0.06, p = 0.018, FDR corrected) and CH18 (MD = −0.06, p = 0.018, FDR corrected) (Figure 4). In the group main effect, during exercise, the amateur runners’ group had significantly higher oxy-Hb in CH18 than the sedentary group (MD = −0.05, p = 0.018, FDR corrected). The channel was mainly in the left dorsolateral prefrontal lobe (Figure 5).
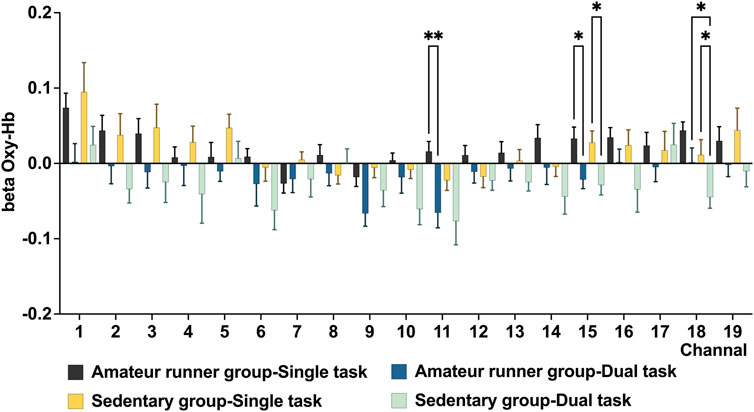
FIGURE 4. In the 2-Back task weight, changes in beta values of prefrontal 0xy-Hb in the amateur runner group and sedentary group in the single task and double task states. Data are presented as mean ± standard error. *: p < 0.05, **: p < 0.01, FDR corrected.
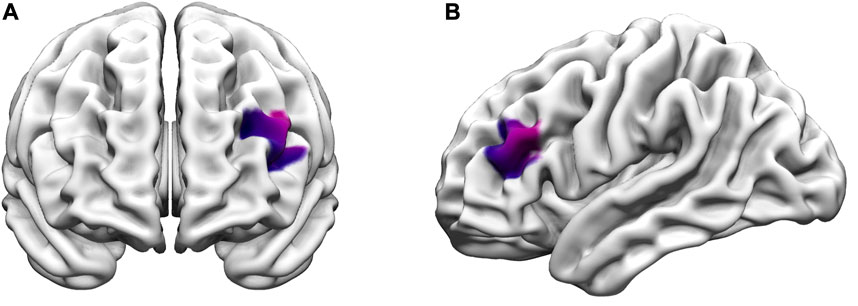
FIGURE 5. Differences in prefrontal oxy-Hb between the amateur runner and sedentary groups during the exercise-2-Back task. The purple color indicates the channels with significant differences (CH18). (A) Anterior view; (B) left view (p < 0.05, FDR corrected).
4 Discussion
This study aimed to explore EF performance and brain oxygenation during exercise in adults with different CRF. The results partially supported our hypotheses. Specifically, this study found amateur runners’ group participants showed improved reaction times in the Stroop and the 2-Back task. Still, there were no changes in accuracy in either group during the exercise. In addition, in the single EF task, participants with the amateur runners’ group had higher oxy-Hb in the left PFC during the single cognitive task. In the dual exercise-EF task, participants in both groups showed lower oxy-Hb in the PFC, but the amateur runners’ group had higher oxy-Hb in the DLPFC during the 2-Back task.
The above findings are consistent with previous meta-analyses, which found a slight improvement in performance on cognitive tasks during acute exercise (Lambourne and Tomporowski, 2010; Chang et al., 2012). Chang et al. concluded that when assessing cognitive performance during exercise, a slight positive effect occurred in physically fit participants but not in participants with a lower health level (Chang et al., 2012). Similarly, we observed an improvement in the high CRF group. Several recent studies found that endurance athletes and physically active participants had improved reaction times (Giles et al., 2018; Zheng et al., 2022). However, our results differed from those of other studies for the low-fitness group. Labelle et al. found that low-fitness individuals showed greater instability in an inhibitory control task (Labelle et al., 2013). Untrained individuals’ simple response patterns during light and vigorous exercise became more sluggish (Brisswalter et al., 1997). And began to lose attention at 60% of maximal heart rate intensity (Hüttermann and Memmert, 2014). In contrast, the attentional performance of exercise experts increases linearly with exercise intensity (Hüttermann and Memmert, 2014). Differently, our findings did not reveal impaired cognitive performance in the sedentary group. The factors responsible for the differences in results may be multifactorial, which may be related to the different CRF measurements, exercise duration, and intensity.
In addition, EF is more sensitive to changes in CRF (Hall et al., 2001). However, Goenarjo et al. (2020b) found no differences in task performance and prefrontal oxygenation during a dual walking-cognitive task. Cognitive performance can improve with exercise, but beyond a certain work intensity, exercise can lead to central nervous system deterioration (Tomporowski, 2003). Specifically, 64%–76% maximum heart rate intensity is more intense for low CRF individuals but favorable for high-fitness individuals because of their higher anaerobic thresholds (Wasserman et al., 1973; Strangman et al., 2002; Şekir et al., 2002). Our results are useful for guiding physical activity. It is thought that increasing Cardiorespiratory levels could improve cognitive performance by increasing prefrontal white and grey matter (Erickson et al., 2014). In conclusion, the relationship between cognitive tasks during exercise and fitness levels is becoming more apparent.
For fNIRS, many studies have observed a decrease in oxy-Hb of the PFC during re-motor cognition (Giles et al., 2018; Huang et al., 2019; Zheng et al., 2022). Zheng et al. (2022) found that during the dual task of motor cognition, there is a redistribution of cerebral blood flow between metabolic and cognitive resources. It is now well established that oxy-Hb content in the prefrontal lobe increases at low to moderate exercise intensities and stabilizes at vigorous exercise intensities (Maidan et al., 2021). We speculate that this may be due to the baseline hemodynamic response in different states. The oxy-Hb response to stimuli under motor and cognitive tasks had higher baseline values than the single task, which may have led to differences in the fitted hemodynamic responses.
The observed between-group differences suggest that more energy-efficient participants during exercise maintained relatively higher oxy-Hb and did not exhibit higher efficiency. This can be attributed to the complex neural regulation required when running on a treadmill, which requires significant metabolic resources and additional cognitive demands of the task (Maidan et al., 2021). Some studies suggest that elite athletes have higher cortical activity during tasks of high complexity (Hung et al., 2004; Endo et al., 2006). Similarly, young people who consume less oxygen under a given load will have a greater oxygen supply to the brain to replenish cognitive resources. Cognitive resource theory suggests that the abilities required for attention and information processing are a limited but renewable set of resources. And the ability to process multiple tasks simultaneously is limited. Exercise can promote angiogenesis and synaptogenesis, which in turn may improve cognitive performance (Swain et al., 2003). In contrast, low CRF participants may mobilize more resources to maintain exercise stability, and they allocate fewer cognitive resources to exercise (Chang et al., 2012). Running at moderate intensities differed more from daily physical activity for participants with low CRF, and this greater than self-determined given intensity was more likely to observe a decrease in oxy-Hb. Results from a study of brain oxygenation in outstanding Kenyan athletes showed a greater decline in PFC oxy-Hb in poorer performers (Santos-Concejero et al., 2017). Whereas a 5% change in intensity is sufficient to alter this stable change in cerebral oxygenation (Santos-Concejero et al., 2017). However, these findings were only observed in the 2-Back task, but not in the Stroop task. This may be because the block-based design of the task can exhibit a more pronounced hemodynamic profile.
This study has many limitations, which may account for the differences between our results and most previous studies. First, previous studies have found that individuals with higher VO2 peak and VO2max and more physically active participants may show better executive function in a single task (Goenarjo et al., 2020a; Goenarjo et al., 2020b; Goenarjo et al., 2021; Salzman et al., 2022). However, no such differences were found when evaluated using the estimated VO2max. Therefore, the reliability of George’s prediction method in evaluating participants’ cognition compared to direct measurement of VO2max still needs further exploration and replication. Second, there may be significant differences by gender and type of exercise in affecting cognition. Based on the different exercise styles, running exercises may require more cognitive resources because they involve postural control and balance (Cantelon and Giles, 2021). A previous meta-analysis showed a worsening of the mean effect of cognitive measures in the running-cognition dual task (Lambourne and Tomporowski, 2010). Third, it is possible that CRF is also associated with better executive functioning in women (Dupuy et al., 2015; Scott et al., 2016). But research on gender differences is still lacking. However, research on gender differences is still lacking. Finally, this study should be as cautious as possible in interpreting the results due to the small sample size. Future research may require larger sample sizes and replicated studies, further exploring the effects of CRF on cognitive performance during exercise in various ways, including gender and intervention.
5 Conclusion
Overall, this study strengthens the evidence for improved EF reaction times in moderate-intensity exercise. Furthermore, participants with better CRF had higher PFC activity during single and dual motor cognitive tasks. Enhancing CRF may reduce the cognitive resources required for the exercise and allocate more cognitive resources to concurrent tasks, such as EF. Our results may provide a theoretical reference for future research and advocacy for fitness activities in non-healthy populations.
Data availability statement
The raw data supporting the conclusion of this article will be made available by the authors, without undue reservation.
Ethics statement
The studies involving humans were approved by The Ethics Committee of Shenzhen University. The studies were conducted in accordance with the local legislation and institutional requirements. The participants provided their written informed consent to participate in this study.
Author contributions
BL and ZR contributed to the conception and design of the study. BL, JY, JW, YQ, and WX obtained, analyzed, or interpreted working data for the study. BL and JY drafted the work or critically revised important intellectual content. ZR provides approval for the publication of the content. All authors contributed to the article and approved the submitted version.
Funding
This work was supported by the National Natural Science Foundation of China (NSFC) (grant number 11002036); Research Foundation for Young Teachers of Shenzhen University (grant number QNJS0274, QNJS-201901294); High-level Scientific Research Foundation for the Introduction of Talent of Shenzhen University (grant number RC00228); Natural Science Featured Innovation Projects in Ordinary Universities in Guangdong Province (grant number 2021KTSCX297); Scientific Research Platform and Project of Colleges and Universities of Education Department of Guangdong Province (2022ZDZX 2087).
Conflict of interest
The authors declare that the research was conducted in the absence of any commercial or financial relationships that could be construed as a potential conflict of interest.
Publisher’s note
All claims expressed in this article are solely those of the authors and do not necessarily represent those of their affiliated organizations, or those of the publisher, the editors and the reviewers. Any product that may be evaluated in this article, or claim that may be made by its manufacturer, is not guaranteed or endorsed by the publisher.
References
Alvarez, J. A., and Emory, E. (2006). Executive function and the frontal lobes: a meta-analytic review. Neuropsychol. Rev. 16, 17–42. doi:10.1007/s11065-006-9002-x
Behrens, M., Gube, M., Chaabene, H., Prieske, O., Zenon, A., Broscheid, K.-C., et al. (2023). Fatigue and human performance: an updated framework. Sports Med. 53, 7–31. doi:10.1007/s40279-022-01748-2
Brisswalter, J., Arcelin, R., Audiffren, M., and Delignières, D. (1997). Influence of physical exercise on simple reaction time: effect of physical fitness. Percept. Mot. Ski. 85, 1019–1027. doi:10.2466/pms.1997.85.3.1019
Brown, T. A., Chorpita, B. F., Korotitsch, W., and Barlow, D. H. (1997). Psychometric properties of the depression anxiety stress scales (DASS) in clinical samples. Behav. Res. Ther. 35, 79–89. doi:10.1016/s0005-7967(96)00068-x
Buysse, D. J., Reynolds, C. F., Monk, T. H., Berman, S. R., and Kupfer, D. J. (1989). The Pittsburgh Sleep quality index: a new instrument for psychiatric practice and research. Psychiatry Res. 28, 193–213. doi:10.1016/0165-1781(89)90047-4
Byun, K., Hyodo, K., Suwabe, K., Ochi, G., Sakairi, Y., Kato, M., et al. (2014). Positive effect of acute mild exercise on executive function via arousal-related prefrontal activations: an fNIRS study. NeuroImage 98, 336–345. doi:10.1016/j.neuroimage.2014.04.067
Cao, L., Zhang, Y., Huang, R., Li, L., Xia, F., Zou, L., et al. (2021). Structural and functional brain signatures of endurance runners. Brain Struct. Funct. 226, 93–103. doi:10.1007/s00429-020-02170-y
Cantelon, J. A., and Giles, G. E. (2021). A review of cognitive changes during acute aerobic exercise. Front. Psychol. 12, 653158. doi:10.3389/fpsyg.2021.653158
Chang, Y. K., Labban, J. D., Gapin, J. I., and Etnier, J. L. (2012). The effects of acute exercise on cognitive performance: a meta-analysis. Brain Res. 1453, 87–101. doi:10.1016/j.brainres.2012.02.068
Cheung, S. K., and Sun, S. Y. (1999). Assessment of optimistic self-beliefs: further validation of the Chinese version of the general self-efficacy Scale. Psychol. Rep. 85, 1221–1224. doi:10.2466/pr0.1999.85.3f.1221
Craig, C. L., Marshall, A. L., Sjöström, M., Bauman, A. E., Booth, M. L., Ainsworth, B. E., et al. (2003). International physical activity questionnaire: 12-country reliability and validity. Med. Sci. Sports Exerc 35, 1381–1395. doi:10.1249/01.MSS.0000078924.61453.FB
Davranche, K., Brisswalter, J., and Radel, R. (2015). Where are the limits of the effects of exercise intensity on cognitive control? J. Sport Health Sci. 4, 56–63. doi:10.1016/j.jshs.2014.08.004
Dupuy, O., Gauthier, C. J., Fraser, S. A., Desjardins-Crèpeau, L., Desjardins, M., Mekary, S., et al. (2015). Higher levels of cardiovascular fitness are associated with better executive function and prefrontal oxygenation in younger and older women. Front. Hum. Neurosci. 9, 66. doi:10.3389/fnhum.2015.00066
Endo, H., Kato, Y., Kizuka, T., and Takeda, T. (2006). A comparison of stimulus synchronous activity in the primary motor cortices of athletes and non-athletes. Exp. Brain Res. 174, 426–434. doi:10.1007/s00221-006-0477-8
Erickson, K. I., Leckie, R. L., and Weinstein, A. M. (2014). Physical activity, fitness, and gray matter volume. Neurobiol. Aging 35, S20–S28. doi:10.1016/j.neurobiolaging.2014.03.034
Garber, C. E., Blissmer, B., Deschenes, M. R., Franklin, B. A., Lamonte, M. J., Lee, I.-M., et al. (2011). American college of sports medicine position stand. Quantity and quality of exercise for developing and maintaining cardiorespiratory, musculoskeletal, and neuromotor fitness in apparently healthy adults: guidance for prescribing exercise. Med. Sci. Sports Exerc 43, 1334–1359. doi:10.1249/MSS.0b013e318213fefb
Gellish, R. L., Goslin, B. R., Olson, R. E., McDONALD, A., Russi, G. D., and Moudgil, V. K. (2007). Longitudinal modeling of the relationship between age and maximal heart rate. Med. Sci. Sports Exerc. 39, 822–829. doi:10.1097/mss.0b013e31803349c6
George, J. D., Vehrs, P. R., Allsen, P. E., Fellingham, G. W., and Fisher, A. G. (1993). Development of a submaximal treadmill jogging test for fit college-aged individuals. Med. Sci. Sports Exerc. 25, 643–646. doi:10.1249/00005768-199305000-00017
Giles, G. E., Eddy, M. D., Brunyé, T. T., Urry, H. L., Graber, H. L., Barbour, R. L., et al. (2018). Endurance exercise enhances emotional valence and emotion regulation. Front. Hum. Neurosci. 12, 398. doi:10.3389/fnhum.2018.00398
Goenarjo, R., Dupuy, O., Fraser, S., Perrochon, A., Berryman, N., and Bosquet, L. (2020b). Cardiorespiratory fitness, blood pressure, and cerebral oxygenation during a dual-task in healthy young males. Behav. Brain Res. 380, 112422. doi:10.1016/j.bbr.2019.112422
Goenarjo, R., Bosquet, L., Berryman, N., Metier, V., Perrochon, A., Fraser, S. A., et al. (2020a). Cerebral oxygenation reserve: the relationship between physical activity level and the cognitive load during a Stroop task in healthy young males. Int. J. Environ. Res. Public Health 17, 1406. doi:10.3390/ijerph17041406
Goenarjo, R., Dupuy, O., Fraser, S., Berryman, N., Perrochon, A., and Bosquet, L. (2021). Cardiorespiratory fitness and prefrontal cortex oxygenation during Stroop task in older males. Physiology Behav. 242, 113621. doi:10.1016/j.physbeh.2021.113621
Hall, C. D., Smith, A. L., and Keele, S. W. (2001). The impact of aerobic activity on cognitive function in older adults: a new synthesis based on the concept of executive control. Eur. J. Cognitive Psychol. 13, 279–300. doi:10.1080/09541440126012
Hillman, C. H., Erickson, K. I., and Kramer, A. F. (2008). Be smart, exercise your heart: exercise effects on brain and cognition. Nat. Rev. Neurosci. 9, 58–65. doi:10.1038/nrn2298
Hillman, C. H., Motl, R. W., Pontifex, M. B., Posthuma, D., Stubbe, J. H., Boomsma, D. I., et al. (2006). Physical activity and cognitive function in a cross-section of younger and older community-dwelling individuals. Health Psychol. 25, 678–687. doi:10.1037/0278-6133.25.6.678
Huang, T., Gu, Q., Deng, Z., Tsai, C., Xue, Y., Zhang, J., et al. (2019). Executive function performance in young adults when cycling at an active workstation: an fNIRS study. Int. J. Environ. Res. Public Health 16, 1119. doi:10.3390/ijerph16071119
Hung, T.-M., Spalding, T. W., Maria, D. L. S., and Hatfield, B. D. (2004). Assessment of reactive motor performance with event-related brain potentials: attention processes in elite table tennis players. J. Sport Exerc. Psychol. 26, 317–337. doi:10.1123/jsep.26.2.317
Huppert, T. J., Diamond, S. G., Franceschini, M. A., and Boas, D. A. (2009). HomER: a review of time-series analysis methods for near-infrared spectroscopy of the brain. Appl. Opt. 48, D280–D298. doi:10.1364/AO.48.00D280
Hüttermann, S., and Memmert, D. (2014). Does the inverted-U function disappear in expert athletes? An analysis of the attentional behavior under physical exercise of athletes and non-athletes. Physiology Behav. 131, 87–92. doi:10.1016/j.physbeh.2014.04.020
Hyland-Monks, R., Cronin, L., McNaughton, L., and Marchant, D. (2018). The role of executive function in the self-regulation of endurance performance: a critical review. Prog. Brain Res. 240, 353–370. doi:10.1016/bs.pbr.2018.09.011
Labelle, V., Bosquet, L., Mekary, S., and Bherer, L. (2013). Decline in executive control during acute bouts of exercise as a function of exercise intensity and fitness level. Brain Cognition 81, 10–17. doi:10.1016/j.bandc.2012.10.001
Lambourne, K., and Tomporowski, P. (2010). The effect of exercise-induced arousal on cognitive task performance: a meta-regression analysis. Brain Res. 1341, 12–24. doi:10.1016/j.brainres.2010.03.091
Lee, D., Pate, R. R., Lavie, C. J., Sui, X., Church, T. S., and Blair, S. N. (2014). Leisure-time running reduces all-cause and cardiovascular mortality risk. J. Am. Coll. Cardiol. 64, 472–481. doi:10.1016/j.jacc.2014.04.058
Ludyga, S., Mücke, M., Colledge, F. M. A., Pühse, U., and Gerber, M. (2019). A combined EEG-fNIRS study investigating mechanisms underlying the association between aerobic fitness and inhibitory control in young adults. Neuroscience 419, 23–33. doi:10.1016/j.neuroscience.2019.08.045
MacLeod, C. M. (1991). Half a century of research on the Stroop effect: an integrative review. Psychol. Bull. 109, 163–203. doi:10.1037/0033-2909.109.2.163
Maidan, I., Mirelman, A., Hausdorff, J. M., Stern, Y., and Habeck, C. G. (2021). Distinct cortical thickness patterns link disparate cerebral cortex regions to select mobility domains. Sci. Rep. 11, 6600. doi:10.1038/s41598-021-85058-z
Molavi, B., and Dumont, G. A. (2012). Wavelet-based motion artifact removal for functional near-infrared spectroscopy. Physiol. Meas. 33, 259–270. doi:10.1088/0967-3334/33/2/259
Pessoa, L. (2008). On the relationship between emotion and cognition. Nat. Rev. Neurosci. 9, 148–158. doi:10.1038/nrn2317
Ross, R., Blair, S. N., Arena, R., Church, T. S., Després, J.-P., Franklin, B. A., et al. (2016). Importance of assessing cardiorespiratory fitness in clinical practice: a case for fitness as a clinical vital sign: A scientific statement from the American heart association. Circulation 134, e653–e699. doi:10.1161/CIR.0000000000000461
Salzman, T., Dupuy, O., and Fraser, S. A. (2022). Effects of cardiorespiratory fitness on cerebral oxygenation in healthy adults: a systematic review. Front. Physiology 13, 838450. doi:10.3389/fphys.2022.838450
Santos-Concejero, J., Billaut, F., Grobler, L., Oliván, J., Noakes, T. D., and Tucker, R. (2017). Brain oxygenation declines in elite Kenyan runners during a maximal interval training session. Eur. J. Appl. Physiol. 117, 1017–1024. doi:10.1007/s00421-017-3590-4
Scholkmann, F., Kleiser, S., Metz, A. J., Zimmermann, R., Mata Pavia, J., Wolf, U., et al. (2014). A review on continuous wave functional near-infrared spectroscopy and imaging instrumentation and methodology. NeuroImage 85, 6–27. doi:10.1016/j.neuroimage.2013.05.004
Schroeter, M. L., Zysset, S., Kupka, T., Kruggel, F., and Yves von Cramon, D. (2002). Near-infrared spectroscopy can detect brain activity during a color-word matching Stroop task in an event-related design. Hum. Brain Mapp. 17, 61–71. doi:10.1002/hbm.10052
Scott, S. P., De Souza, M. J., Koehler, K., Petkus, D. L., and Murray-Kolb, L. E. (2016). Cardiorespiratory fitness is associated with better executive function in young women. Med. Sci. Sports Exerc. 48, 1994–2002. doi:10.1249/MSS.0000000000000974
Şekir, U., Özyener, F., and Gür, H. (2002). Effect of time of day on the relationship between lactate and ventilatory thresholds: a brief report. J. Sports Sci. Med. 1, 136–140.
Spirduso, W. W. (1975). Reaction and movement time as a function of age and physical activity level. J. Gerontology 30, 435–440. doi:10.1093/geronj/30.4.435
Statista (2023). U.S. Americans who went running or jogging 2021. Available at: https://www.statista.com/statistics/190303/running-participants-in-the-us-since-2006/ (Accessed May 7, 2023).
Strangman, G., Culver, J. P., Thompson, J. H., and Boas, D. A. (2002). A quantitative comparison of simultaneous BOLD fMRI and NIRS recordings during functional brain activation. NeuroImage 17, 719–731. doi:10.1006/nimg.2002.1227
Swain, R. A., Harris, A. B., Wiener, E. C., Dutka, M. V., Morris, H. D., Theien, B. E., et al. (2003). Prolonged exercise induces angiogenesis and increases cerebral blood volume in primary motor cortex of the rat. Neuroscience 117, 1037–1046. doi:10.1016/s0306-4522(02)00664-4
Tempest, G. D., and Reiss, A. L. (2019). The utility of functional near-infrared spectroscopy for measuring cortical activity during cycling exercise. Med. Sci. Sports Exerc. 51, 979–987. doi:10.1249/MSS.0000000000001875
Tomporowski, P. D. (2003). Effects of acute bouts of exercise on cognition. Acta Psychol. 112, 297–324. doi:10.1016/S0001-6918(02)00134-8
Wasserman, K., Whipp, B. J., Koyl, S. N., and Beaver, W. L. (1973). Anaerobic threshold and respiratory gas exchange during exercise. J. Appl. Physiology 35, 236–243. doi:10.1152/jappl.1973.35.2.236
Woo, E., and Sharps, M. J. (2003). Cognitive aging and physical exercise. Educ. Gerontol. 29, 327–337. doi:10.1080/713844341
Wu, C.-H., Nien, J.-T., Lin, C.-Y., Li, R.-H., Chu, C.-H., Kao, S.-C., et al. (2022). Cardiorespiratory fitness is associated with sustained neurocognitive function during a prolonged inhibitory control task in young adults: an ERP study. Psychophysiology 59, e14086. doi:10.1111/psyp.14086
Xia, M., Wang, J., and He, Y. (2013). BrainNet viewer: a network visualization tool for human brain connectomics. PLoS One 8, e68910. doi:10.1371/journal.pone.0068910
Yan, W., Zheng, K., Weng, L., Chen, C., Kiartivich, S., Jiang, X., et al. (2020). Bibliometric evaluation of 2000–2019 publications on functional near-infrared spectroscopy. NeuroImage 220, 117121. doi:10.1016/j.neuroimage.2020.117121
Ye, J. C., Tak, S., Jang, K. E., Jung, J., and Jang, J. (2009). NIRS-SPM: statistical parametric mapping for near-infrared spectroscopy. Neuroimage 44, 428–447. doi:10.1016/j.neuroimage.2008.08.036
Keywords: executive function, cognition, exercise, dual task, functional near-infrared spectroscopic
Citation: Liu B, Yu J, Wu J, Qin Y, Xiao W and Ren Z (2023) Runners with better cardiorespiratory fitness had higher prefrontal cortex activity during both single and exercise-executive function dual tasks: an fNIRS study. Front. Physiol. 14:1246741. doi: 10.3389/fphys.2023.1246741
Received: 24 June 2023; Accepted: 07 August 2023;
Published: 14 August 2023.
Edited by:
Eliseo Iglesias-Soler, University of A Coruña, SpainReviewed by:
Salvador Romero-Arenas, Universidad Católica San Antonio de Murcia, SpainMarcos Seijo, University of Greenwich, United Kingdom
Copyright © 2023 Liu, Yu, Wu, Qin, Xiao and Ren. This is an open-access article distributed under the terms of the Creative Commons Attribution License (CC BY). The use, distribution or reproduction in other forums is permitted, provided the original author(s) and the copyright owner(s) are credited and that the original publication in this journal is cited, in accordance with accepted academic practice. No use, distribution or reproduction is permitted which does not comply with these terms.
*Correspondence: Zhanbing Ren, cnpiQHN6dS5lZHUuY24=
†These authors have contributed equally to this work and share first authorship