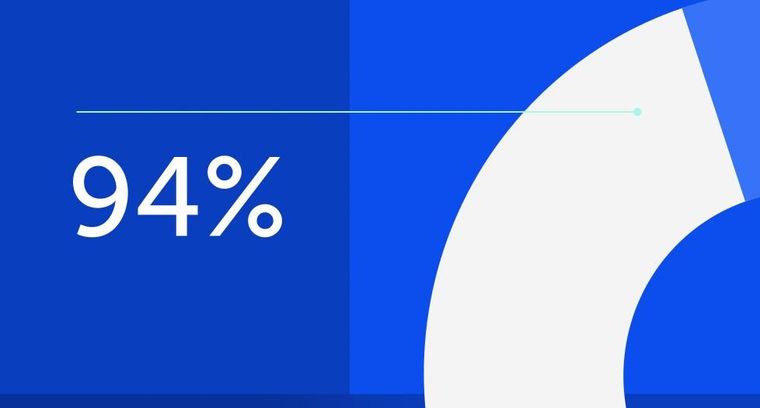
94% of researchers rate our articles as excellent or good
Learn more about the work of our research integrity team to safeguard the quality of each article we publish.
Find out more
ORIGINAL RESEARCH article
Front. Physiol., 17 August 2023
Sec. Invertebrate Physiology
Volume 14 - 2023 | https://doi.org/10.3389/fphys.2023.1244190
Long noncoding RNAs (lncRNAs) play significant roles in the regulation of mRNA expression or in shaping the competing endogenous RNA (ceRNA) network by targeting miRNA. The insect gut is one of the most important tissues due to direct contact with external pathogens and functions in the immune defense against pathogen infection through the innate immune system and symbionts, but there are limited observations on the role of the lncRNA-involved ceRNA network of the Toll/Imd pathway and correlation analysis between this network and bacterial microbiota in the Altica viridicyanea gut. In this research, we constructed and sequenced six RNA sequencing libraries using normal and antibiotic-reared samples, generating a total of 17,193 lncRNAs and 26,361 mRNAs from massive clean data by quality control and bioinformatic analysis. Furthermore, a set of 8,539 differentially expressed lncRNAs (DELs) and 13,263 differentially expressed mRNAs (DEMs), of which related to various immune signaling pathways, such as the Toll/Imd, JAK/STAT, NF-κB, and PI3K-Akt signaling pathways, were obtained between the two experimental groups in A. viridicyanea. In addition, numerous GO and KEGG enrichment analyses were used to annotate the DELs and their target genes. Moreover, six Toll family members and nineteen signal genes from the Toll/Imd signaling pathway were identified and characterized using online tools, and phylogenetic analyses of the above genes proved their classification. Next, a lncRNA-miRNA-mRNA network of the Toll/Imd pathway was built, and it contained different numbers of DEMs in this pathway and related DELs based on prediction and annotation. In addition, qRT-PCR validation and sequencing data were conducted to show the expression patterns of the above DELs and DEMs related to the Toll/Imd signaling pathway. Finally, the correlated investigations between DELs or DEMs of the Toll/Imd signaling pathway and most changes in the gut bacterial microbiota revealed significantly positive or negative relationships between them. The present findings provide essential evidence for innate immune ceRNAs in the beetle gut and uncover new potential relationships between innate immune pathways and the gut bacterial microbiota in insects.
Animals, both vertebrates and invertebrates, have evolved a variety of strategies to recognize and eradicate invading microbes, including innate and adaptive immunity. Due to the lack of an adaptive immune system, insects mainly depend on the innate immune system, a nonspecific immunity that could provide the first line of defense against infections through pattern recognition receptors (PRRs), such as Toll receptors, peptidoglycan-recognition proteins (PGRPs), gram-negative bacteria-binding proteins (GNBPs), and scavenger receptors (SRs) (Myllymaki et al., 2014; Zhang et al., 2022a). Overall, insects can activate the humoral response against pathogens by secreting antimicrobial peptides (AMPs) and initiate the cellular response to fight infections by phagocytosing bacteria and encapsulating parasites (Myllymaki et al., 2014). To date, the production of AMPs through Toll and Imd (immune deficiency) signaling pathways via NF-κB transcription factors has been well investigated in model insects against either gram-positive bacterial/fungal or gram-negative bacterial infections (Silverman et al., 2009; Valanne et al., 2022). In detail, bacterial and fungal structures, with specific pathogen-associated molecular patterns (PAMPs), were recognized and then active ligand Spaetzle to form dimers and bind to Tolls, which is the first step that allows signal transduction into the cell. Intracellular signaling factors, including MyD88, Tube, and Pelle, are combined, and finally, Pelle results in the phosphorylation and degradation of Cactus, which releases two NF-κB transcription factors, Dorsal and dorsal-related immunity factor (Dif), to promote AMP expression (Lemaitre and Hoffmann, 2007; Valanne et al., 2022). Likewise, the transmembrane receptor PGRP-LC leads to recruitment of a signaling complex consisting of Imd, the adaptor protein Fadd, and Dredd, and then the TAB2/TAK1 complex can phosphorylate and activate the IKK complex and finally regulate the activation of Relish, whose N-terminal part (Rel-68) ultimately translocates into the nucleus to promote the transcription of AMP genes in Drosophila, such as Diptericin and Cecropin (Myllymaki et al., 2014).
With the development of high-throughput sequencing technology, RNA sequencing (RNA-seq)-based transcriptome analysis is a useful tool and has been conducted extensively to understand the developmental function or immune responses in insects (Wu et al., 2016a; Chang et al., 2018; Yang et al., 2022). Noncoding RNAs (ncRNAs), categorized as microRNAs (miRNAs), small interfering RNAs (siRNAs), PIWI-interacting RNAs (piRNAs), circular RNAs (circRNAs) and long noncoding RNAs (lncRNAs), are supposed to be “transcriptional noise” owing to their transcription from DNA without, protein-coding functions but are actually functional RNA molecules that play essential roles in regulating mRNA expression (Alexander et al., 2010; Cech and Steitz, 2014). Among these ncRNAs, lncRNAs, longer than 200 nucleotides (nt), are usually divided into exonic, intronic, intergenic, and overlapping lncRNAs based on their location relative to protein-coding transcripts (Quinn and Chang, 2016). Over the past decade, increasing evidence has suggested that they are widely expressed in various tissues and exert diverse biological functions by influencing the stability and translation of mRNAs and then activating or deactivating downstream signaling pathways (Statello et al., 2021). For instance, the lncRNAs CR11538, CR46018 and CR33942 have been shown to prevent or initiate the transcription of immune effectors in the Toll/Imd signaling in Drosophila (Zhou et al., 2021a; Zhou et al., 2021b; Zhou et al., 2022a). Besides, a hot topic is that lncRNAs or circRNAs are regarded as competitive endogenous RNAs (ceRNAs) or miRNA sponges, which could participate in competitively inhibiting miRNAs to bind target genes (Pasquinelli, 2012). To the best of our knowledge, the gut microbiota serves as a complex and dynamic biological system composed of commensal, symbiotic and pathogenic microbial communities, which contribute to host nutrient absorption and energy metabolism and improve host fitness (Evariste et al., 2019). Therefore, it has been demonstrated that gut flora health is largely beneficial to overall host wellbeing. Conversely, an imbalance in gut microbiota composition, termed dysbiosis, may cause host fitness loss and increase susceptibility to pathogens (Zhang et al., 2015). However, there are a limited number of studies on the regulatory roles of ceRNAs under the disturbance of gut microbiome homeostasis using antibiotic treatment in beetles.
Altica viridicyanea, the leaf beetle genus Altica, is regarded as a good model to investigate plant secondary chemistry metabolism by gut microbes based on diversification in its host plant, Geranium nepalense Sweet (Geraniaceae) (Wei et al., 2022). In the present study, we performed RNA library construction and sequencing from normal and antibiotic-reared samples, which produced massive lncRNA and mRNA sequences after quality control and filtration using the MGI2000 platform. Moreover, the characterization, differentially expressed analysis and annotation information of the above data were executed based on bioinformatic analysis between the two experimental groups. Subsequently, we transcriptome-wide identified and characterized the cDNA and protein features of Toll/Imd signaling pathway genes. In addition, the expression models of differentially expressed mRNAs (DEMs) from the above genes and their related differentially expressed lncRNAs (DELs) were selected and calculated according to the transcriptome data and qRT‒PCR validation. Finally, a lncRNA-associated ceRNA regulatory network of the Toll/Imd signaling pathway in the A. viridicyanea gut was successfully established, and we applied correlation analysis between DEMs or DELs from the Toll/Imd signaling pathway and most changes in the gut bacterial microbiota from previous 16S rRNA amplicon sequencing to investigate the disturbance in the gut bacterial microbiota in A. viridicyanea under antibiotic treatment. The combined multiomics data were used to explore the innate immune ceRNA network and interplay of the gut bacterial microbiota and Toll/Imd signaling pathway at the coding and noncoding RNA levels, which will pave new avenues for further observations of host-microbiota interactions and adaptation in insects.
All animal experimental procedures were approved by the Animal Care and Use Committee of Nankai University. In this study, samples of A. viridicyanea individuals were collected from Jizhou district (40.22′N, 117.50′E) in Tianjin, China on 19th May 2022 and were reared in an environmentally controlled growth chamber maintained at 25°C temperature with 60% relative humidity and a 16 h: 8 h (L:D) photoperiod, feeding on Geranium nepalense Sweet (Geraniaceae). Moreover, newly born eggs and hatched larvae of A. viridicyanea were obtained and kept in 90 mm diameter Petri dishes padded with moist filter papers, and then, hatched second larval instars were fed with normal host leaves or with those by antibiotic treatment until sexual maturation (10 days after emerging adults), as described in our previous report (Wei et al., 2020). In brief, 5 mL of antibiotic solution (50 μg/mL tetracycline, 200 μg/mL rifampicin, 100 μg/mL streptomycin = 1:2:4) was used to completely submerged leaves for 5 min. Then, a total of 50 normal (C group) and 50 antibiotic-reared individuals (A group), starved after 2 days, were used to gently dissect the whole gut tissues by clamping the head of living beetles with sterile forceps and removing the head. Finally, ten guts from the C or A group were surface-sterilized in 70% ethanol for 1 min, randomly pooled to build the transcriptome library as one replicate, immediately frozen in liquid nitrogen and stored at −80°C until subsequent total RNA extraction. In addition, the remaining guts after library construction were used for qRT-PCR analysis.
In this study, transcriptome sequencing was performed at Beijing Genomics Institution (BGI; Wuhan, China) Technology Co., Ltd. with three biological replicates of each treatment. Total RNA of all experimental guts was isolated using TRIzol reagent (Invitrogen, United States) according to the manufacturer’s protocols. In addition, RNA purity was assessed using the OD260/OD280 absorbance ratio between 1.8 and 2.0. RNA quality was visualized by 1% agarose gel electrophoresis staining, and RNA quantity was measured with an Agilent 2,100 Bioanalyzer (Agilent Technologies, United States). Finally, 5 μg of total RNA was removed from ribosomal RNAs (rRNAs) and used to prepare the transcriptome library construction.
Briefly, first-strand cDNA was produced with random hexamer primers and M-MuLV Reverse Transcriptase, and then the second strand was synthesized by adding RNaseH, dNTPs, DNA polymerase I, and buffer. Subsequently, the product was purified with AMPure XP beads, the cohesive ends of DNA were repaired using T4 DNA polymerase and Klenow DNA polymerase, and the product was further size-selected and ligated to an adaptor. Furthermore, we purified and amplified each product by AMPure XP beads and PCR (polymerase chain reaction), respectively. At the last step for preparation of the final sequencing library, magnetic beads were used to capture the amplified cDNA fragments, and the quality of all libraries was assessed using a Agilent BioAnalyzer 2,100 system (Agilent Technologies, United States). Later on, the diluted 1 ng/μL cDNA with an average insert size of 250–300 bp (base pair) was loaded for 150 bp paired-end sequencing using the MGI2000 platform.
The raw reads with adaptor contamination, low-quality, and undetermined data were removed using SOAPnuke software (version 1.5.2) to produce clean reads, and the Q20, Q30, and GC contents, representing effective sequencing values, were calculated. Furthermore, the quality of clean reads was estimated by FastQC (version 0.10.1), and then we mapped clean data to the genome of A. viridicyanea (data unpublished) with Hisat 2 (version 2.0.4) (Kim et al., 2019). The mapped reads of each group were assembled by StringTie (version 1.0.4), and finally, the transcriptome results were outputted (Pertea et al., 2015).
First, we applied Cufflinks software (version 2.2.1) to cover the transcripts with a clear chain orientation, and subsequently, the protein coding potentials were found by screening transcripts with no fewer than 2 exons and more than 200 bp in length. Moreover, CNCI software (CNCI_threshold >0 is mRNA, CNCI_threshold < 0 is lncRNA) was used to classify the coding transcripts and noncoding transcripts based on the spectrum of adjacent trinucleotides (Sun et al., 2013). Next, we used CPC (version 0.9-r2) (CPC_threshold >0 is mRNA, CPC_threshold < 0 is lncRNA) and txCdsPredict (txCdsPredict_threshold >500 is mRNA, txCdsPredict_threshold < 500 is lncRNA) software to assess the transcripts with coding potential according to the biological sequence characteristics (Kong et al., 2007). In addition, Pfam_Scan software was used to select the known protein family domains (Finn et al., 2010). In our study, the selected principle is that only transcripts identified by three of the above four methods were considered coding mRNAs or lncRNAs (Zhang et al., 2022b).
The expression levels of total mRNAs and lncRNAs were calculated and normalized to transcripts per million (TPM). In addition, RPKM (reads per kilobase of exon model per million mapped reads) was conducted to estimate their expression abundance in different samples, and the infrequently expressed transcripts with FPKM < 1 in all samples were filtered out. Furthermore, the correlation and principal component analysis (PCA) analyses based on RPKM using StringTie (version 1.0.4) software, and then the significantly differentially expressed mRNA and lncRNA were considered with absolute Log2 | Fold Change | >1 and p-value < 0.001 by edgeR (version 3.14.0) software (Love et al., 2014).
First, to obtain annotation information on the functions of obtained mRNAs, all of them were searched against the Nr (NCBI nonredundant protein sequences), Nt (NCBI nucleotide), SwissProt (a manually annotated and reviewed protein sequence database), KOG (eukaryotic Ortholog Groups), and KEGG (Kyoto Encyclopedia of Genes and Genomes) databases using BLASTX (basic local alignment search tool) and BLASTN with an E-value < 10–5. In addition, Blast2GO was used for GO (Gene Ontology) annotation with an E-value < 10–5 from the Nr annotation results (Fu et al., 2021).
Moreover, the DELs and their target genes were functionally annotated by GO and KEGG databases. The GOseq R package was used to execute the GO enrichment, and KOBAS was used to explore the KEGG enrichment (Yu et al., 2012). The statistical significance between the A and C groups was considered the threshold to select significantly enriched GO terms and KEGG pathways (p-value < 0.01).
To explore the regulatory function of lncRNAs, cis/trans-regulatory algorithms were applied to predict the target genes. First, the regions 0–10 kb upstream or 0–20 kb downstream of cis-acting lncRNAs were screened to identify colocated target genes, and then the lncRNAs were considered trans-acting on the target genes once the binding energy was < −30 and exceeded the above region. Meanwhile, Pearson ≥0.6 and Spearman ≥0.6 correlation coefficients between the lncRNAs and target genes were used to predict the coexpressed target genes. In addition, the overlaps between the lncRNAs and target genes were classified into four categories: overlap, anti-overlap, incomplete, and anti-incomplete (Zhang et al., 2022b).
To identify candidate cDNA sequences of the Toll/Imd signaling pathway from sequencing data in A. viridicyanea, the published above genes from insects were used to search against the available information using TBLASTN and BLASTP databases with E-values < 0.001 as the threshold to find the corresponding gene members (Tong et al., 2015). Furthermore, the ORFs (open reading frames) of the putative cDNA sequences were translated using the ORF finder server, and the conserved domains of all the protein sequences were predicted by the SMART (simple modular architecture research tool) and TMHMM (version 2.0) servers. The signal peptide (SP) was examined using the program SignalP (version 5.0). In addition, for the protein sequence of each gene, basic information, such as molecular weight, length of protein, and isoelectronic point (PI), was identified with the ExPASy ProtParam server (Ren et al., 2021). Later on, the subcellular localization of the six Toll genes was predicted with WoLF PSORT (Zou et al., 2023). Lastly, MAFFT and MEGA (version X; Molecular Evolutionary Genetics Analysis) programs were applied to execute multiple sequence alignment of the amino acid sequences of the Toll/Imd signaling pathway genes from D. melanogaster, Anopheles gambiae, Bombyx mori, Tribolium castaneum, Diabrotica virgifera virgifera and A. viridicyanea, and to construct phylogenetic trees with the neighbor-joining (NJ) method (1,000 replicate bootstraps), respectively (Supplementary Table S2). Meanwhile, the results of phylogenetic analysis were optimized and shown using FigTree software (Tong et al., 2015). In this study, the obtained complete cDNA sequences of Toll/Imd signaling pathway genes were submitted to the GenBank database.
To our knowledge, lncRNAs, known as ceRNAs, can play vital roles in regulating specific target genes by sponging and sequestering miRNAs (Pasquinelli, 2012). Therefore, in order to better construct the ceRNA network based on the lncRNA-miRNA-mRNA axis, all the mRNAs of Toll/Imd signaling pathway were selected from the Section 2.7, and the principle of the selected related lncRNA and miRNA for building the ceRNA network is that the same expression trends of lncRNAs and mRNAs are opposite to the expression of miRNAs, and the p-values of lncRNA-miRNA (Pearson correlation coefficients ≥0.6) and lncRNA-miRNA (Pearson correlation coefficients ≥0.6) pairs were less than 0.05. Lastly, all these prediction was imported into Cytoscape software (version 3.9.1) to export the relevant ceRNA regulatory network (Otasek et al., 2019). Besides, the miRNA sequencing and prediction of the selected miRNA-mRNA pairs of Toll/Imd signaling pathway were in agreement with our previous report (data unpublished).
To integrate the transcriptomic and previous 16 S rRNA amplicon sequencing data (data unpublished), Pearson correlation coefficients were calculated for the integrated analysis of the above data. Concretely, the DEMs and DELs of Toll/Imd signaling pathway and the most abundant changes in gut bacterial microbiota from previous 16S rRNA amplicon sequencing were selected to produce correlation heatmaps using R packages (version 2.15.3). The level of statistical significance in this analysis was set at *p < 0.05, **p < 0.01 and ***p < 0.001.
To compare the expression levels between transcriptome sequencing and experimental analysis, fifteen DEMs and ten DELs of the Toll/Imd signaling pathway were used to perform quantitative real-time reverse transcription PCR (qRT-PCR). EF-1α was chosen as an internal control, and other primers were designed with Primer Premier 5 (Supplementary Table S3). Furthermore, the CFX96 real-time PCR detection system (Bio-Rad Laboratories, Hercules, CA) was used to carry out the 20 μL reaction system with PerfectStart Green qPCR SuperMix (Transgen, China) following the manufacturer’s instructions, including 10 μL of PerfectStart™ Green qRT-PCR SuperMix, 0.4 μL of each primer, 2 μL of cDNA template synthesized from total RNA in Section 2.1, and 7.2 μL of nucleotide-free water. The thermal cycling profile consisted of an initial denaturation at 95°C (30 s), followed by 40 cycles of 95°C for 15 s, 60°C for 30 s, and 72°C for 30 s. Finally, relative expression levels were calculated with the 2−ΔΔCT method (Livak and Schmittgen, 2001), and the graphics of expression patterns were drawn by GraphPad Prism 9.0 (GraphPad Software Inc., United States).
In the present research, a total of six transcriptome libraries from the A. viridicyanea gut, namely, three control (C) and three antibiotic (A) treatment samples, were sequenced using the MGI2000 platform. Sequencing produced a total of 704,740,664 clean reads from 748,521,486 raw reads after removing low-quality, adaptor and N-containing reads. The mean Q30 and Q20 of each sample were 93.47% and 97.33%, respectively, and the mean GC content of the sequencing data was 47.82% (Supplementary Table S1). Furthermore, all these reads were used for transcript assembly and mapping with StringTie and Hisat 2, respectively, suggesting that different numbers of exons, RNA lengths (mRNA and lncRNA) and transcripts were obtained (Supplementary Figure S1). In addition, the clean reads were submitted to the NCBI SRA (sequence read archive) database under accession number PRJNA946198. All of the above sequencing parameters provided accurate evidence for further analyses.
Coding potential methods, including CPC, CNCI, Pfam, and txCdsPredict, were applied to screen the transcripts of mRNAs or lncRNAs. A total of 17,193 lncRNAs and 26,361 mRNAs (17,730 known mRNAs and 8,631 novel mRNAs) were obtained based on the prediction (Figure 1A, B). The lengths of lncRNAs and mRNAs, and the number of exons and transcripts are shown in Supplementary Figure S2.
FIGURE 1. The coding capacity prediction of lncRNAs (A) and mRNAs (B) are shown in a Venn diagram with CNCI, CPC, txCdsPredict, and pfam. (C) The expression profiles of genes from six experimental samples. (D) Expression patterns with hierarchical clustering of genes from six experimental groups. (E) Correlation heatmap of RNA sequencing among the six transcriptome libraries.
At first, the FPKM values were calculated to estimate the expression patterns of all genes. In the C1 to C3 groups, the FPKM values were mainly less than 1, while the FPKM values were mainly between 1 and 10 in the A2 and A3 groups, except for the A1 group (Figure 1C). In our study, a heatmap was used to visualize all differentially expressed RNAs (Figure 1D). In addition, the correlation relationships (R2 values) represented the robustness of biological replicates and the reliability of RNA-seq data among the six groups (Figure 1E).
As shown in Figure 2, a total of 8,539 DELs were identified, including 8,120 upregulated and 419 downregulated DELs between the two experimental groups. In addition, 12,358 and 905 DEMs were upregulated and downregulated, respectively, from a total of 13,263 DEMs between the A and C groups. Of these DEMs, 8,432 and 3,926 were known and novel upregulated DEMs, and 650 and 255 were known and novel downregulated DEMs, respectively (Figure 2).
FIGURE 2. The bar chart shown the numbers of differential expressed lncRNAs, mRNAs, known mRNAs, and novel mRNAs.
For target prediction of lncRNAs, lncRNA-mRNA interactions were screened based on the results of coexpression and colocalization. The results indicated that a total of 6,409 lncRNA-mRNA interaction pairs were cis/trans-acting from 6,035 lncRNAs targeting 6,421 mRNAs. In addition, a total of 4,086 lncRNA-mRNA pairs overlapped from 3,097 lncRNAs targeting 1985 mRNAs (Supplementary Figure S3). In addition, all DELs target a total of 6,425 DEMs in this research.
First, to obtain the basic annotation information of assembled mRNAs, all of them were compared against several databases. In detail, 25,594 mRNAs (97.09%) were annotated in at least one database, and 25,153 (95.42%), 13,072 (49.59%), 18,188 (69%), 21,278 (80.72%), 16,660 (63.20%) and 13,441 (50.99%) mRNAs were annotated against the Nr, Nt, SwissProt, KEGG, KOG, Pfam, and GO databases, respectively. Based on the Nr annotation, this transcriptome was mostly comparable to D. virgifera virgifera (50.65%), followed by Leptinotarsa decemlineata (14.4%), Anoplophora glabripennis (12.99%) and Callosobruchus maculatus (3.63%) (Figure 3A). Next, the GO database was used to comprehensively and widely investigate the basic functions of mRNAs, suggesting that a total of 13,441 mRNAs were classified into three GO categories, including biological process, cellular component, and molecular function (Figure 3B), such as 6,097 mRNAs in cellular process, 8,180 mRNAs in cellular anatomical entity, and 7,110 mRNAs in binding. Furthermore, the KEGG database was also used to systematically analyze gene functions. As shown in Figure 3A, C, total number of 18,188 mRNAs were assigned to six main categories of KEGG metabolic pathways: cellular processes, environmental information processing, genetic information processing, human diseases, metabolism, and organismal systems, especially 1,638 mRNAs in the immune system.
FIGURE 3. (A) Species distribution of A. viridicyanea mRNAs. (B) GO annotation of all mRNAs. X-axis: the number of mRNAs annotated in the GO groups, Y-axis: three major functional categories of GO terms including biological process (Red), cellular component (Blue), and molecular function (Green). (C) KEGG classification of all mRNAs. X-axis: the number of mRNAs annotated in the KEGG database, Y-axis: six major categories of KEGG pathway including cellular processes (Red), environmental information processing (Blue), genetic information processing (Green), human diseases (Purple), metabolism (Orange), and organismal systems (Yellow).
Second, to ascertain the functional details of the DELs and target genes, GO and KEGG enrichment analyses were carried out. Based on the above GO categories, most DELs were distributed in cellular process, cellular anatomical entity, binding, catalytic activity, and so forth (Figure 4A). Likewise, 5,186 target genes were found in cellular anatomical entity, followed by 4,203 target genes in binding, 3,944 target genes in cellular processes, and 3,211 target genes in catalytic activity (Figure 4B). Furthermore, the top 20 significantly enriched pathways of DELs and target genes using KEGG enrichment indicated some immune signaling pathways, including the MAPK signaling pathway, TNF signaling pathway, B-cell receptor signaling pathway, IL-17 signaling pathway, and MAPK signaling pathway-fly (Figure 4C, D). In addition, we also summarized the various immune signaling pathways in the A. viridicyanea gut with different numbers of DELs and DEMs (Table 1). These collected data suggested that lncRNAs may contribute to the regulation of mRNA expression levels related to immune signaling pathways, which could help us to illustrate the immune responses of beetles under pathogen stimulation in the future.
FIGURE 4. GO classification and KEGG annotation of the DELs (A–C) and their target genes (B–D). X-axis of Figure (A, B) the number of mRNAs annotated in the GO database; and Y-axis: three major functional categories of GO terms including biological process (Red), cellular component (Blue), and molecular function (Green). X-axis of Figure (C, D) the percentages of mRNAs belong to the corresponding pathway, and Y-axis: the top 20 pathways. The sizes of bubble represent the number of genes in the corresponding pathway, and the colors of the bubble represent the enrichment q-value of the corresponding pathway.
In order to comprehensively understand one essential immune pathway in insects, we further focused on investigation of Toll/Imd pathway genes and their ceRNA network in A. viridicyanea based on sequencing data. Our observations suggested that six full-length cDNA sequences of Toll family genes were identified based on annotation information and online tools. The cDNA and protein characterization of all Tolls are shown in Table 2, and all sequences have been deposited in the NCBI database under accession numbers from OQ819300 to OQ819306. Concretely, all ORF lengths ranged from 3,972bp to 2,571 bp and encoded polypeptides 1,323 to 856 amino acids (aa) with molecular weights (MWs) of 149.45–98.26 kDa and theoretical isoelectric points (PIs) of 5.66–8.54, respectively. All Tolls contain different numbers of extracellular serial leucine-rich repeat (LRR) motifs, a transmembrane domain, and an intracellular cytoplasmic Toll/IL1 receptor (TIR) domain (Figure 5). Moreover, based on the classification of invertebrate Tolls described by Gerdol et al. (2017), six A. viridicyanea Tolls were divided into three subfamilies: four P-type TLRs (Toll-1 to Toll-4), one sPP-type TLR (Toll-5), and one sP-type TLR (Toll-6), whose subcellular location is the plasma membrane (Table 2).
FIGURE 5. Domain organizations of Toll/Imd signaling pathway proteins in A. viridicyanea using SMART prediction. Complex domains are marked with different shapes and colors.
Furthermore, to explore the signal members of the Toll/Imd pathway in A. viridicyanea, we also selected a total of nineteen extracellular and intracellular genes according to transcriptome sequencing. As shown in Table 3, six Spaetzle, three TAB2, two Relish, and one Myd88, Pelle, Tube, Cactus, Dorsal, Imd, Dredd and TAK1 genes were screened with complete coding DNA sequence (CDS) regions. Furthermore, we also characterized the above protein sequences in A. viridicyanea, including the length of the protein, molecular weight, isoelectronic point and functional domain, indicating that they possess typical domains for signal transduction or binding, such as the DEATH, Spaetzle, ANK, and RHD_DNA_bind domains. All these data showed that information on all Toll/Imd pathway genes with typical functional domains could expand our knowledge for comparative immunology between A. viridicyanea and other insects.
To evaluate the evolutionary relationships among the above family genes, the protein sequences from five insects were aligned to construct neighbor-joining trees (Supplementary Table S2). In line with our classification, the phylogeny result based on a phylogenetic tree with NJ method implied that the A. viridicyanea Tolls were well clustered together with corresponding subfamilies in insects, including P-type Tolls, sPP-type Tolls and sP-type Tolls (Figure 6A). All the pathway genes were also well clustered into each gene family (Figure 6B), which supports their orthologous relationships. Next, the immune recognition and signal transduction of all these genes will be validated in the A. viridicyanea gut.
FIGURE 6. Phylogenetic analysis of Toll/Imd signaling pathway genes in A. viridicyanea and other insects based on the neighbor-joining method using the program MEGAX with 1,000 bootstrap values. (A) Toll family genes, and (B) extracellular and intracellular factors. All accession numbers of amino acid sequences used for phylogenetic tree construction are summarized in Supplementary Table S2, and nodes with posterior probability supports are shown by black or grey colors of circles and pentacles.
Finally, to better understand the expression changes of Toll/Imd signaling pathway genes under antibiotic treatment, we calculated and summarized the profiles of DEMs and DELs of Toll/Imd pathway according to RNA-seq data. The results suggested that we found differential expression patterns of five Tolls, two Spaetzles and some pathway genes between the C and A groups. Most of these genes were significantly upregulated, while TAB2-1 expression significantly decreased during antibiotic rearing. Notably, the expression levels of all NF-κB-related genes were not significantly changed in this study (Figure 7A). In addition, the expression trends by qRT‒PCR were consistent with the differential expression of RNA sequencing, which verified the reliability of our sequencing data.
FIGURE 7. Transcription analysis of DEMs and DELs involved in the Toll/Imd signaling pathway. Heatmap of DEMs (A) and DELs (B) associated with the Toll/Imd signaling pathway. Heatmap colors correspond to the scale values of FPKM. The histograms represent the expression of DEMs (A) and DELs (B) by qRT-PCR analysis.
In this research, to investigate the miRNA sponge capacity of lncRNAs, eight Toll/Imd pathway genes, including Pelle, Dredd, Spaetzle-1, Spaetzle-3, Spaetzle-4, Spaetzle-5, TAK1 and TAB2-1, and their related miRNAs and lncRNAs were predicted and selected to construct a ceRNA network (Figure 8). In total, 76 miRNA‒mRNA pairs and 54 lncRNA‒miRNA pairs were obtained by target prediction. Of these lncRNAs and mRNAs, 10 DELs were involved in the regulation of 6 DEMs in this predicted lncRNA-mediated ceRNA network. In detail, Dredd, TAK1 and TAB2-1 are regulated by 7 miRNAs, and five miRNAs contribute to target Pelle. Meanwhile, 12 miRNAs were found to combine Spaetzle family genes. In addition, the novel miRNA-64-3p targets the majority of lncRNAs in the lncRNA-related ceRNA network of Toll/Imd signaling pathway. As shown in Figure 7B, a heatmap was used to display all above DELs with increasing expression between control and antibiotic groups, which brings together transcriptome sequencing and qRT-PCR validation with similar expression patterns.
FIGURE 8. The predicted lncRNA-miRNA-mRNA network of the Toll/Imd signaling pathway. LncRNA, miRNA and mRNA are represented by red, blue and green ellipses, respectively.
We hypothesized that DELs and DEMs of the Toll/Imd pathway might be involved in response to alterations in the gut bacterial microbiota. Therefore, we detected their association relationships with Pearson correlation coefficients under antibiotic treatment. The results shown in Figure 9 suggest that, after antibiotic treatment, all selected changes in the gut bacterial microbiota were significantly positively or negatively correlated with different numbers of DEMs in the Toll/Imd signaling pathway. In detail, Toll-2 and Toll-3 changes were significantly positively affected by alterations in Bacteroides, Barnesiella, Prevotella, Parasutterella and Ruminococcus, whereas they significantly negatively influenced Toll-5 expression. In contrast, changes in Burkholderia, Eubacterium and Spiroplasma were only significantly correlated with Toll-4 and Toll-6 expression changes. Furthermore, changes in some gut bacterial microbiota, such as Bacteroides, Barnesiella, Prevotella, Parasutterella and Ruminococcus, were mainly significantly negatively involved in influencing some downstream factors, such as Myd88, Tube, Pelle, Imd, Dredd and Cactus, but Akkermansia alteration played the opposite role in affecting the expression of Pelle and Cactus. In addition, alterations in Eubacterium and Spiroplasma significantly positively or negatively affected the majority of the expression of DELs related to the Toll/Imd signaling pathway. However, Bacteroides, Prevotella, Ruminococcus and Akkermansia changes were only significantly positively or negatively associated with one DEL (Figure 9).
FIGURE 9. Integrated analysis between DEMs or DELs and gut bacterial microbiota using Pearson correlation coefficients. Correlation coefficients are shown in different colors, which represent different degrees of positive or negative correlation. Ellipses sizes are proportional to correlation value and correlation significance is performed by *p < 0.05, **p < 0.01 and ***p < 0.001.
A. viridicyanea serves as a good material for investigating the adaptive evolution between insects and host plants. However, there have been few studies on the innate immunity of A. viridicyanea due to the lack of an adaptive immune system, which mainly depends on nonspecific immune responses, such as the activation of immune signaling pathways and production of antimicrobial peptides (Lin et al., 2022; Wei et al., 2022). LncRNAs, incapable of translation into proteins, are significant regulators that regulate gene expression and are involved in various physiological and immunological functions at the transcriptional and posttranscriptional levels, such as the lncRNA-miRNA-mRNA axis based on the competitive endogenous RNA hypothesis (Zhang et al., 2020; Lin et al., 2022). In the last decade, accumulating evidence has confirmed the important roles of lncRNAs in the regulation of innate immunity in insects (Valanne et al., 2019; Zhang et al., 2020; Zhang et al., 2021a; Moure et al., 2022). In this study, we performed transcriptome analysis to detect the characterization and expression profiles of lncRNAs and mRNAs in the A. viridicyanea gut during normal rearing and antibiotic feeding, constructed a lncRNA-mediated ceRNA network of the Toll/Imd signaling pathway, and finally analyzed the correlation between their related DEMs or DELs and changes in the gut bacterial microbiota.
To date, a growing number of lncRNAs have been reported with the development of transcriptome sequencing from various tissues or cells in insects, such as the fat body, silk gland, gonad, and cell lines (Wu et al., 2016b; Lin et al., 2022). We used the gut tissues of A. viridicyanea to carry out transcriptome sequencing in this report, as it is an important digestive and immune tissue in insects. Our study indicated that we obtained a large number of raw data and clean reads, and among these reads, a total of 17,193 lncRNAs and 26,361 mRNAs were identified, which was more than some previous reports (Wu et al., 2016b; Zhang et al., 2020). In addition, the average exon numbers and RNA lengths of all selected lncRNAs were less and shorter than those of all identified mRNAs in the A. viridicyanea gut, suggesting that lncRNAs possess sequence conservation for facilitating their binding function.
Furthermore, differential expression analysis of lncRNAs and mRNAs was conducted to identify DELs and DEMs and obtain their functional annotation using different databases. A total of 8,539 lncRNAs were differentially expressed, which consisted of 8,120 lncRNAs with increasing expression and 419 lncRNAs with decreasing expression after antibiotic feeding, indicating the potential participation of these lncRNAs in various functions in the A. viridicyanea gut. We further detected 12,358 upregulated and 905 downregulated DEMs. Consistent with previous research, this finding also implied that lncRNAs are expressed at relatively lower levels than mRNAs in the A. viridicyanea gut due to their high tissue specificity for exerting specific biological functions (Wu et al., 2016b). Herein, among all these DELs and DEMs, our results illustrated that they were strongly enriched in immune signaling pathways based on GO and KEGG analysis. In detail, the lysosome, MAPK signaling pathway–fly and PI3K-Akt signaling pathway were enriched in the majority of DELs and DEMs. PRR-related pathways, including the NOD-like receptor, Toll-like receptor, C-type lectin receptor, Toll/Imd and RIG-I-like receptor signaling pathways, were also selected, which may play pivotal roles in pathogenic recognition or complementary function during changes in the gut bacterial microbiota of A. viridicyanea (Takeuchi and Akira, 2010). The JAK/STAT signaling pathway in insects is similar to type I interferon signaling in mammals, which induces the transcription of genes to prevent viral replication (Stark and Darnell, 2012). In addition, the interleukin-17 (IL-17) family includes ancient proinflammatory cytokines that combine their specific receptors (IL-17 receptor, IL-17R) to protect the host against pathogens (Cua and Tato, 2010). Collectively, our data show that immune DELs and DEMs might be involved in response to antibiotic treatment in the A. viridicyanea gut.
Toll receptors are important PRRs and type I transmembrane proteins, containing extracellular LRR domain, transmembrane domain, and intracellular TIR domain for recognizing conserved PAMPs and initiating downstream signaling pathways from invertebrates to vertebrates (Medzhitov, 2001; Kawai and Akira, 2010). In invertebrates, multiple cysteine cluster TLRs (mccTLRs) and single cysteine cluster TLRs (sccTLRs) were found and classified based on LRR ectodomain arrangements, but only sccTLRs exist in vertebrate animals (Gerdol et al., 2017). In this study, we selected six Toll family genes from transcriptome sequencing data, which are less than the number of Drosophila and silkworm Tolls (Zhang et al., 2021b). Of these A. viridicyanea Tolls, three subfamilies, including four P-type TLRs (Toll-1 to Toll-4) resembling Drosophila Tolls, one sPP- type TLR (Toll-5) with the same LRR arrangement but shorter than P-type TLRs, and one sP- type TLR (Toll-6) shorter than vertebrate TLRs, were grouped, suggesting that the number and diversity of insect Tolls are less than those in mollusk and echinozoa due to their expansion in most deuterostomes and Lophotrochozoa (Satake and Sekiguchi, 2012; Zhang et al., 2015; Gerdol et al., 2017). Furthermore, Imd is another essential immune defense factor in the Drosophila gut that has been implicated in protection against bacterial pathogens (Lemaitre et al., 1995). Here, we selected an Imd gene, translating a DEATH domain to facilitate signal transduction, in A. viridicyanea.
In Drosophila, the Toll pathway was discovered to be contributed to fungal and bacterial infections and activation by Spaetzles and PGRPs. For regulation of the innate immune system in Drosophila, PGRP-SA cooperates with PGRP-SD in the hemolymph and is required for the activation of Toll pathway by binding to Lys-type PGN (peptidoglycan), and PGRP-LC and PGRP-LE recognize the DAP-type PGN of gram-negative bacteria to activate the Imd pathway (Zhang et al., 2019). Next, the recognition of PAMPs results in the Spaetzle activation, and then causes the dimerization of Tolls, which combine with the intracellular adaptor Myd88 to recruit Tube and Pelle, participate in the phosphorylation and degradation of Cactus, and finally release Dorsal or Dif to induce the expression of AMPs (Valanne et al., 2022). Furthermore, to initiate the Imd signaling pathway, the receptors PGRP-LE and PGRP-LC detect bacterial ligands to promote the interaction between Imd and Fadd, leading to caspase Dredd activation, and then, Relish is released into the nucleus to produce AMPs (Silverman et al., 2003). In our study, we obtained a total of six extracellular Spaetzle genes, whose numbers are similar to those in D. melanogaster (Parker et al., 2001), and different numbers of intracellular signal factors in the Toll/Imd pathway, except for the Fadd gene, suggesting that a classical Toll/Imd pathway may exist in the A. viridicyanea gut. Next, more evidence on molecular and comparative immunology between model insects and A. viridicyanea will be applied to tackle the questions of immune signal transduction and function of the Toll/Imd pathway in A. viridicyanea under bacterial pathogen infection.
LncRNA regulation involves a complex matrix of coding and noncoding RNAs at the transcriptional or posttranscriptional level and has been demonstrated to be related to the modulation of the expression of immune- or metabolism-related genes (Li et al., 2018). For instance, Drosophila lncRNA-CR33942 and lncRNA-CR46018 interact with the transcription factors Dif and Dorsal to promote the Toll pathway (Zhou et al., 2021b; Zhou et al., 2022a; Zhou et al., 2022b). To date, an increasing number of investigations have shown that gene expression can be controlled through lncRNA-mediated ceRNAs with miRNA binding in animals. Specifically, in the ceRNA network, miRNAs interact with lncRNAs, serving as precursors or pre-miRNA enhancers, by miRNA-binding sites, leading to the regulation of gene expression (Pilyugin and Irminger-Finger, 2014; Jiang et al., 2017). In this study, eight mRNAs of the Toll/Imd signaling pathway were targeted by thirty-eight miRNAs interacting with twenty-seven lncRNAs in the A. viridicyanea gut under antibiotic treatment, while Toll family genes were not controlled by miRNAs and lncRNAs in our research. Of these lncRNAs and mRNAs, ten DELs contributed to regulate six DEMs of the Toll/Imd signaling pathway by combining with various miRNAs. For example, two DELs, LTCONS_00044637 and LTCONS_00032806, competitively bind novel-miRNA-893-5p and novel-miRNA-44-5p to regulate the significantly differential expression of Dredd and TAB2-1, respectively. Moreover, the majority of DELs were involved in the expression of essential signal factors, Pelle and Dredd, by competing with the target novel-miRNA-40-5p, novel-miRNA-768-5p, novel-miRNA-893-5p and novel-miRNA-64-3p.
Previous studies on the relationships between the Toll/Imd pathway and commensal bacteria illustrated that the Imd pathway of Drosophila maintains homeostasis of the barrier epithelium with gut bacterial symbionts, which may also be involved in Toll activities during viral infections in the fly gut (Chow and Kagan, 2018). Due to an imbalance in gut microbiota composition influencing host fitness, we determined whether changes in the gut bacterial microbiota affected the lncRNA and mRNA expression of the Toll/Imd pathway in A. viridicyanea during antibiotic treatment. On the basis of the above observations, we found that disturbances in the gut bacterial microbiota can influence the expression of some lncRNAs and mRNAs in the Toll/Imd pathway in the A. viridicyanea gut, demonstrating that host innate immunity through lncRNA-mediated ceRNAs may play essential roles in response to changes in the gut bacterial microbiota of A. viridicyanea. Likewise, there are growing numbers of reports on the gut bacterial microbiota affecting the expression of immune genes in insects. For example, the depression of the thanatin gene, an antimicrobial peptide, in Riptortus pedestris significantly improved the population of Burkholderia gut symbionts during Escherichia coli injection (Lee et al., 2022). Additionally, the gut bacterial microbiota of Apis mellifera and Spodoptera frugiperda can maintain AMP expression in gut tissues in response to future adverse environments (Kwong et al., 2017; Zheng et al., 2023). In red palm weevil (RPW), Rhynchophorus ferrugineus, the effects of the Toll signaling pathway on gut bacterial composition are highly limited and nonspecific under Spaetzle knockdown (Muhammad et al., 2020). Taken together, further studies will be conducted to confirm the lncRNA-related ceRNA regulatory network of the Toll/Imd pathway and elucidate their roles in maintaining gut homeostasis in A. viridicyanea.
In conclusion, we first identified and analyzed lncRNAs and mRNAs in normal and antibiotic-fed A. viridicyanea gut based on transcriptome sequencing, which produced massive raw data and clean reads. In total, 8,539 DELs and 13,263 DEMs were found, some of which were involved in immune responses. Furthermore, different numbers of Toll/Imd signaling pathway genes were identified and characterized using annotation information and online tools. In addition, we predicted and constructed a lncRNA-mediated ceRNA network of the Toll/Imd signaling pathway. Finally, correlation analysis was performed between DELs or DEMs of the Toll/Imd signaling pathway and most changes in the gut bacterial microbiota. Our data provides new insights into innate immune ceRNAs in A. viridicyanea and valuable information for future studies on the interactions between the gut bacterial microbiota and innate immune responses in insects.
The datasets presented in this study can be found in online repositories. The names of the repository/repositories and accession number(s) can be found in the article/Supplementary Material. Further inquiries can be directed to the first or corresponding authors.
The animal study was approved by the Animal Care and Use Committee of Nankai University.
YR: Conceptualization; formal analysis; investigation; methodology; supervision; visualization; writing–original draft; writing–review and editing. JC: Data curation; investigation; methodology; resources; software; writing–review and editing. YW: Investigation; methodology; resources; writing–review and editing. SF: Data curation; formal analysis; investigation; writing–review and editing. WB: Project administration; visualization; writing–review and editing. HX: Funding acquisition; investigation; project administration; supervision; writing–review and editing. All authors contributed to the article and approved the submitted version.
The authors declare financial support was received for the research, authorship, and/or publication of this article. This work was financially supported by the National Natural Science Foundation of China (Grant Nos. 31672334 and 32170456) and the Fundamental Research Funds for the Central Universities (Grant No. 63213120).
The authors would like to thank all laboratory members for technical advices and helpful discussions. BGI (Wuhan, China) constructed the transcriptome libraries, and performed the sequencing.
The authors declare that the research was conducted in the absence of any commercial or financial relationships that could be construed as a potential conflict of interest.
All claims expressed in this article are solely those of the authors and do not necessarily represent those of their affiliated organizations, or those of the publisher, the editors and the reviewers. Any product that may be evaluated in this article, or claim that may be made by its manufacturer, is not guaranteed or endorsed by the publisher.
The Supplementary Material for this article can be found online at: https://www.frontiersin.org/articles/10.3389/fphys.2023.1244190/full#supplementary-material
Alexander, R. P., Fang, G., Rozowsky, J., Snyder, M., and Gerstein, M. B. (2010). Annotating noncoding regions of the genome. Nat. Rev. Genet. 11, 559–571. doi:10.1038/nrg2814
Cech, T., and Steitz, J. (2014). The noncoding RNA revolution-trashing old rules to forge new ones. Cell 157 (1), 77–94. doi:10.1016/j.cell.2014.03.008
Chang, Z. X., Akinyemi, I. A., Guo, D. Y., and Wu, Q. (2018). Characterization and comparative analysis of microRNAs in the rice pest Sogatella furcifera. PLoS ONE 13 (9), e0204517. doi:10.1371/journal.pone.0204517
Chow, J., and Kagan, J. C. (2018). The fly way of antiviral resistance and disease tolerance. Adv. Immunol. 140, 59–93. doi:10.1016/bs.ai.2018.08.002
Cua, D. J., and Tato, C. M. (2010). Innate IL-17-producing cells: the sentinels of the immune system. Nat. Rev. Immunol. 10, 479–489. doi:10.1038/nri2800
Evariste, L., Barret, M., Mottier, A., Mouchet, F., Gauthier, L., and Pinelli, E. (2019). Gut microbiota of aquatic organisms: a key endpoint for ecotoxicological studies. Environ. Pollut. 248, 989–999. doi:10.1016/j.envpol.2019.02.101
Finn, R. D., Mistry, J., Tate, J., Boursnell, C., et al. (2010). The Pfam protein families database. Nucleic Acids Res. 40, D290–D301. doi:10.1093/nar/gkr1065
Fu, S., Duan, Y., Wang, S., Ren, Y., and Bu, W. (2021). Comparative transcriptomic analysis of Riptortus pedestris (Hemiptera: Alydidae) to characterize wing formation across all developmental stages. Insects 12, 226. doi:10.3390/insects12030226
Gerdol, M., Venier, P., Edomi, P., and Pallavicini, A. (2017). Diversity and evolution of TIR domain-containing proteins in bivalves and metazoa: new insights from comparative genomics. Dev. Comp. Immunol. 70, 145–164. doi:10.1016/j.dci.2017.01.014
Jiang, L., Shao, C., Wu, Q. J., Chen, G., Zhou, J., Yang, B., et al. (2017). NEAT1 scaffolds RNA-binding proteins and the Microprocessor to globally enhance pri-miRNA processing. Nat. Struct. Mol. Biol. 24, 816–824. doi:10.1038/nsmb.3455
Kawai, T., and Akira, S. (2010). The role of pattern-recognition receptors in innate immunity: update on toll-like receptors. Nat. Immunol. 11, 373–384. doi:10.1038/ni.1863
Kim, D., Paggi, J. M., Park, C., Bennett, C., and Salzberg, S. L. (2019). Graph-based genome alignment and genotyping with HISAT2 and HISAT-genotype. Nat. Biotechnol. 37, 907–915. doi:10.1038/s41587-019-0201-4
Kong, L., Zhang, Y., Ye, Z. Q., Liu, X. Q., Zhao, S. Q., Wei, L., et al. (2007). CPC: assess the protein-coding potential of transcripts using sequence features and support vector machine. Nucleic Acids Res. 35, W345–W349. doi:10.1093/nar/gkm391
Kwong, W. K., Mancenido, A. L., and Moran, N. A. (2017). Immune system stimulation by the native gut microbiota of honey bees. R. Soc. Open Sci. 4, 170003. doi:10.1098/rsos.170003
Lee, J., Cha, W. H., and Lee, D. W. (2022). Multiple precursor proteins of thanatin isoforms, an antimicrobial peptide associated with the gut symbiont of Riptortus pedestris. Front. Microbiol. 12, 796548. doi:10.3389/fmicb.2021.796548
Lemaitre, B., and Hoffmann, J. (2007). The host defense of Drosophila melanogaster. Annu. Rev. Immunol. 25, 697–743. doi:10.1146/annurev.immunol.25.022106.141615
Lemaitre, B., Kromer-metzger, E., Michaut, L., Nicolas, E., Meister, M., Georgel, P., et al. (1995). A recessive mutation, immune deficiency (imd), defines two distinct control pathways in the Drosophila host defense. Proc. Natl. Acad. Sci. U. S. A. 92, 9465–9469. doi:10.1073/pnas.92.21.9465
Li, B., Jiang, D., Meng, Z., Zhang, Y., Zhu, Z. X., Lin, H. R., et al. (2018). Genome-wide identification and differentially expression analysis of lncRNAs in tilapia. BMC Genom 19 (1), 729. doi:10.1186/s12864-018-5115-x
Lin, S., Yin, H. T., Zhao, Z. M., Chen, Z. K., Zhou, X. M., Zhang, Z. D., et al. (2022). LincRNA_XR209691.3 could promote Bombyx mori nucleopolyhedrovirus replication by interacting with BmHSP70. Insect Mol. Biol. 1–13, 160–172. doi:10.1111/imb.12821
Livak, K. J., and Schmittgen, T. D. (2001). Analysis of relative gene expression data using real-time quantitative PCR and the 2(-Delta Delta C(T)) Method. Methods 25 (4), 402–408. doi:10.1006/meth.2001.1262
Love, M. I., Huber, W., and Anders, S. (2014). Moderated estimation of fold change and dispersion for RNA-seq data with DESeq2. Genome Biol. 15 (12), 550. doi:10.1186/s13059-014-0550-8
Medzhitov, R. (2001). Toll-like receptors and innate immunity. Nat. Rev. Immunol. 1, 1135–1145. doi:10.1038/35100529
Moure, U. A. E., Tan, T., Sha, L., Lu, X., Shao, Z., Yang, G., et al. (2022). Advances in the immune regulatory role of non-coding RNAs (miRNAs and lncRNAs) in insect-pathogen interactions. Front. Immunol. 13, 856457. doi:10.3389/fimmu.2022.856457
Muhammad, A., Habineza, P., Wang, X., Xiao, R., Ji, T., Hou, Y., et al. (2020). Spätzle homolog-Mmediated Toll-like pathway regulates innate immune responses to maintain the homeostasis of gut microbiota in the red palm weevil, Rhynchophorus ferrugineus Olivier (Coleoptera: Dryophthoridae). Front. Microbiol. 11, 846. doi:10.3389/fmicb.2020.00846
Myllymaki, H., Valanne, S., and Ramet, M. (2014). The Drosophila imd signaling pathway. J. Immunol. 192, 3455–3462. doi:10.4049/jimmunol.1303309
Otasek, D., Morris, J. H., Bouças, J., Pico, A. R., and Demchak, B. (2019). Cytoscape automation: empowering workflow-based network analysis. Genome Biol. 20 (1), 185. doi:10.1186/s13059-019-1758-4
Parker, J. S., Mizuguchi, K., and Gay, N. J. (2001). A family of proteins related to Spätzle, the toll receptor ligand, are encoded in the Drosophila genome. Proteins 45, 71–80. doi:10.1002/prot.1125
Pasquinelli, A. (2012). MicroRNAs and their targets: recognition, regulation and an emerging reciprocal relationship. Nat. Rev. Genet. 13, 271–282. doi:10.1038/nrg3162
Pertea, M., Pertea, G. M., Antonescu, C. M., Chang, T. C., Mendell, J. T., and Salzberg, S. L. (2015). StringTie enables improved reconstruction of a transcriptome from RNA-seq reads. Nat. Biotechnol. 33, 290–295. doi:10.1038/nbt.3122
Pilyugin, M., and Irminger-Finger, I. (2014). Long non-coding RNA and microRNAs might act in regulating the expression of BARD1 mRNAs. Int. J. Biochem. Cell Biol. 54, 356–367. doi:10.1016/j.biocel.2014.06.018
Quinn, J. J., and Chang, H. Y. (2016). Unique features of long non-coding RNA biogenesis and function. Nat. Rev. Genet. 17 (1), 47–62. doi:10.1038/nrg.2015.10
Ren, Y. P., Liu, H. X., Fu, S. Y., Dong, W., Pan, B., and Bu, W. (2021). Transcriptome-wide identification and characterization of toll-like receptors response to Vibrio anguillarum infection in Manila clam (Ruditapes philippinarum). Fish. Shellfish Immunol. 111, 49–58. doi:10.1016/j.fsi.2021.01.007
Satake, H., and Sekiguchi, T. (2012). Toll-like receptors of deuterostome invertebrates. Front. Immunol. 3, 34. doi:10.3389/fimmu.2012.00034
Silverman, N., Paquette, N., and Aggarwal, K. (2009). Aggarwal, K. Specificity and signaling in the Drosophila immune response. Invert. Surviv. J. 6, 163–174.
Silverman, N., Zhou, R., Erlich, R. L., Hunter, M., Bernstein, E., Schneider, D., et al. (2003). Immune activation of NF-kappaB and JNK requires Drosophila TAK1. J. Biol. Chem. 278, 48928–48934. doi:10.1074/jbc.M304802200
Stark, G. R., and Darnell, J. E. (2012). The JAK-STAT pathway at twenty. Immunity 36, 503–514. doi:10.1016/j.immuni.2012.03.013
Statello, L., Guo, C. J., Chen, L. L., and Huarte, M. (2021). Gene regulation by long non-coding RNAs and its biological functions. Nat. Rev. Mol. Cell Biol. 22 (2), 96–118. doi:10.1038/s41580-020-00315-9
Sun, L., Luo, H., Bu, D., Zhao, G., Yu, K., Zhang, C., et al. (2013). Utilizing sequence intrinsic composition to classify protein-coding and long non-coding transcripts. Nucleic Acids Res. 41, e166. doi:10.1093/nar/gkt646
Takeuchi, O., and Akira, S. J. C. (2010). Pattern recognition receptors and inflammation. Cell 140 (6), 805–820. doi:10.1016/j.cell.2010.01.022
Tong, C., Liu, Y. Q., Zhang, C. F., Shi, J., Qi, H., and Zhao, K. (2015). Transcriptome-wide identification, molecular evolution and expression analysis of Toll-like receptor family in a Tibet fish, Gymnocypris przewalskii. Fish. Shellfish Immunol. 46, 334–345. doi:10.1016/j.fsi.2015.06.023
Valanne, S., Salminen, T. S., Järvelä-Stölting, M., Vesala, L., and Rämet, M. (2019). Immune-inducible non-coding RNA molecule lincRNA-IBIN connects immunity and metabolism in Drosophila melanogaster. PLoS Pathog. 15, e1007504. doi:10.1371/journal.ppat.1007504
Valanne, S., Vesala, L., Maasdorp, M. K., Salminen, T. S., and Rämet, M. (2022). The Drosophila toll pathway in innate immunity: from the core pathway toward effector functions. J. Immunol. 209, 1817–1825. doi:10.4049/jimmunol.2200476
Wei, J., Segraves, K. A., Li, W. Z., Yang, X. K., and Xue, H. J. (2020). Gut bacterial communities and their contribution to performance of specialist Altica flea beetles. Microb. Ecol. 80, 946–959. doi:10.1007/s00248-020-01590-x
Wei, J., Yang, X. K., Zhang, S. K., Segraves, K. A., and Xue, H. J. (2022). Parallel metatranscriptome analysis reveals degradation of plant secondary metabolites by beetles and their gut symbionts. Mol. Ecol. 31, 3999–4016. doi:10.1111/mec.16557
Wu, P., Cheng, T., Liu, C., Liu, D., Zhang, Q., Long, R., et al. (2016b). Systematic identification and characterization of Long non-coding RNAs in the silkworm, Bombyx mori. PLoS One 11, e0147147. doi:10.1371/journal.pone.0147147
Wu, P., Jiang, X., Guo, X., Li, L., and Chen, T. (2016a). Genome-wide analysis of differentially expressed microRNA in Bombyx mori infected with nucleopolyhedrosis Virus. PLoS ONE 11 (11), e0165865. doi:10.1371/journal.pone.0165865
Yang, X. B., Zhou, C., Yang, J. P., Gong, M. F., Yang, H., Long, G. Y., et al. (2022). Identification and profiling of Sogatella furcifera microRNAs and their potential roles in regulating the developmental transitions of nymph–adult. Insect Mol. Biol. 31 (6), 798–809. doi:10.1111/imb.12805
Yu, G., Wang, L. G., Han, Y., and He, Q. Y. (2012). ClusterProfiler: an R package for comparing biological themes among gene clusters. Omics 16 (5), 284–287. doi:10.1089/omi.2011.0118
Zhang, L., Li, L., Guo, X., Litman, G. W., Dishaw, L. J., and Zhang, G. (2015). Massive expansion and functional divergence of innate immune genes in a protostome. Sci. Rep. 5, 8693. doi:10.1038/srep08693
Zhang, R. N., Li, C. T., Ren, F. F., Ye, M. Q., Deng, X. J., Yi, H. Y., et al. (2019). Functional characterization of short-type peptidoglycan recognition proteins (PGRPs) from silkworm Bombyx mori in innate immunity. Dev. Comp. Immunol. 95, 59–67. doi:10.1016/j.dci.2019.01.015
Zhang, S. L., Yin, H. T., Shen, M. M., Huang, H., Hou, Q., Zhang, Z., et al. (2020). Analysis of lncRNA-mediated gene regulatory network of Bombyx mori in response to BmNPV infection. J. Invertebr. Pathol. 170, 107323. doi:10.1016/j.jip.2020.107323
Zhang, Y. Q., Liang, H. Y., Zou, H. X., Lu, J., Zhang, M., and Liang, B. (2022b). Comprehensive analysis of the lncRNAs, mRNAs, and miRNAs implicated in the immune response of Pinctada fucata martensii to Vibrio parahaemolyticus. Fish. Shellfish Immunol. 130, 132–140. doi:10.1016/j.fsi.2022.09.006
Zhang, Y. Q., Yao, N., Zhang, C. T., Sun, X., Huang, J., Zhao, B., et al. (2022a). LncRNA-mRNA integrated profiling analysis in response to white spot syndrome virus in hepatopancreas in Penaeus japonicus. Fish. Shellfish Immunol. 129, 251–262. doi:10.1016/j.fsi.2022.08.061
Zhang, Z., Li, X., Zhang, J., Li, Y., Wang, Y., Song, Y., et al. (2021a). Toll9 from Bombyx mori functions as a pattern recognition receptor that shares features with toll-like receptor 4 from mammals. Proc. Natl. Acad. Sci. U. S. A. 118, e2103021118. doi:10.1073/pnas.2103021118
Zhang, Z., Zhao, Z., Lin, S., Wu, W., Tang, W., Dong, Y., et al. (2021b). Identification of long noncoding RNAs in silkworm larvae infected with Bombyx mori cypovirus. Arch. Insect Biochem. Physiol. 106, 1–12. doi:10.1002/arch.21777
Zheng, R. W., Cheng, L. L., Peng, J., Li, Q., Yang, F., Yang, D., et al. (2023). Comparative analysis of gut microbiota and immune genes linked with the immune system of wild and captive Spodoptera frugiperda (Lepidoptera: Noctuidae). Dev. Comp. Immunol. 138, 104530. doi:10.1016/j.dci.2022.104530
Zhou, H., Li, S., Pan, W., Wu, S., Ma, F., and Jin, P. (2022a). Interaction of lncRNACR33942 with Dif/Dorsal facilitates antimicrobial peptide transcriptions and enhances Drosophila Toll immune responses. J. Immunol. 208, 1978–1988. doi:10.4049/jimmunol.2100658
Zhou, H., Li, S., Wu, S., Jin, P., and Ma, F. (2021a). lncRNA-CR11538 decoys Dif/Dorsal to reduce antimicrobial peptide products for restoring Drosophila Toll immunity homeostasis. Int. J. Mol. Sci. 22, 10117. doi:10.3390/ijms221810117
Zhou, H., Ni, J., Wu, S., Ma, F., Jin, P., and Li, S. (2021b). lncRNA-CR46018 positively regulates the Drosophila Toll immune response by interacting with Dif/Dorsal. Dev. Comp. Immunol. 124, 104183. doi:10.1016/j.dci.2021.104183
Zhou, H., Wu, S., Liu, L., and Jin, P. (2022b). Drosophila Relish activating lncRNACR33942 transcription facilitates antimicrobial peptide expression in Imd innate immune response. Front. Immunol. 13, 905899. doi:10.3389/fimmu.2022.905899
Keywords: Altica viridicyanea, long noncoding RNAs, Toll/Imd signaling pathway, gut bacterial microbiota, competing endogenous RNA
Citation: Ren Y, Chen J, Wang Y, Fu S, Bu W and Xue H (2023) The lncRNA-mediated ceRNA network of Altica viridicyanea is involved in the regulation of the Toll/Imd signaling pathway under antibiotic treatment. Front. Physiol. 14:1244190. doi: 10.3389/fphys.2023.1244190
Received: 22 June 2023; Accepted: 02 August 2023;
Published: 17 August 2023.
Edited by:
Baojian Zhu, Anhui Agricultural University, ChinaReviewed by:
Qingguo Meng, Nanjing Normal University, ChinaCopyright © 2023 Ren, Chen, Wang, Fu, Bu and Xue. This is an open-access article distributed under the terms of the Creative Commons Attribution License (CC BY). The use, distribution or reproduction in other forums is permitted, provided the original author(s) and the copyright owner(s) are credited and that the original publication in this journal is cited, in accordance with accepted academic practice. No use, distribution or reproduction is permitted which does not comply with these terms.
*Correspondence: Wenjun Bu, d2VuanVuYnVAbmFua2FpLmVkdS5jbg==; Huaijun Xue, eHVlaGpAbmFua2FpLmVkdS5jbg==
Disclaimer: All claims expressed in this article are solely those of the authors and do not necessarily represent those of their affiliated organizations, or those of the publisher, the editors and the reviewers. Any product that may be evaluated in this article or claim that may be made by its manufacturer is not guaranteed or endorsed by the publisher.
Research integrity at Frontiers
Learn more about the work of our research integrity team to safeguard the quality of each article we publish.