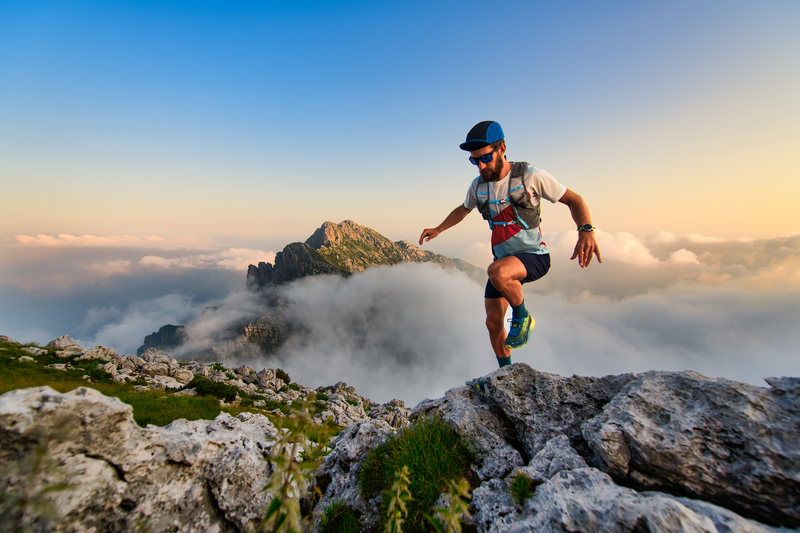
94% of researchers rate our articles as excellent or good
Learn more about the work of our research integrity team to safeguard the quality of each article we publish.
Find out more
ORIGINAL RESEARCH article
Front. Physiol. , 02 May 2023
Sec. Gastrointestinal Sciences
Volume 14 - 2023 | https://doi.org/10.3389/fphys.2023.1175227
Background: Macleaya cordata extract (MCE) is effective in the treatment of enteritis, but its mechanism has not been fully elucidated. Therefore, this study combined network pharmacology and molecular docking technologies to investigate the potential pharmacological mechanism of MCE in the treatment of enteritis.
Methods: The information of active compounds in MCE was accessed through the literature. Furthermore, PubChem, PharmMapper, UniProt, and GeneCards databases were used to analyze the targets of MCE and enteritis. The intersection of drug and disease targets was imported into the STRING database, and the analysis results were imported into Cytoscape 3.7.1 software to construct a protein–protein interaction (PPI) network and to screen core targets. The Metascape database was used for conducting Gene Ontology (GO) enrichment and Kyoto Encyclopedia of Genes and Genomes (KEGG) pathway analyses. AutoDock Tools software was used for the molecular docking of active compounds with the core targets.
Results: MCE has four active compounds, namely, sanguinarine, chelerythrine, protopine, and allocryptopine, and a total of 269 targets after de-duplication. Furthermore, a total of 1,237 targets were associated with enteritis, 70 of which were obtained by aiding the drug–disease intersection with the aforementioned four active compound targets of MCE. Five core targets including mitogen-activated protein kinase 1 (MAPK1) and AKT serine/threonine kinase 1 (AKT1) were obtained using the PPI network, which are considered the potential targets for the four active compounds of MCE in the treatment of enteritis. The GO enrichment analysis involved 749 biological processes, 47 cellular components, and 64 molecular functions. The KEGG pathway enrichment analysis revealed 142 pathways involved in the treatment of enteritis by the four active compounds of MCE, among which PI3K-Akt and MAPK signaling pathways were the most important pathways. The results of molecular docking showed that the four active compounds demonstrated good binding properties at the five core targets.
Conclusion: The pharmacological effects of the four active compounds of MCE in the treatment of enteritis involve acting on signaling pathways such as PI3K-Akt and MAPK through key targets such as AKT1 and MAPK1, thus providing new indications for further research to verify its mechanisms.
Enteritis, a common intestinal disease that can be caused by viruses, bacteria, fungi, or parasites, has the potential to compromise human health (Ryan et al., 2018; Leon-Velarde et al., 2019; Dacal et al., 2020; Lim et al., 2020; Dixon, 2021; Tarris et al., 2021). When the human body is unable to balance the intestinal microflora and pathogenic stimuli, enteritis occurs. In a dysfunctional immune system, the lack of such a balance causes an increase in the inflammatory response (Moreira Lopes et al., 2020). Clinical symptoms include abdominal pain, diarrhea, fever, and even death in severe cases (Cleary, 1998). Chinese herbal medicine is edible and has remarkable medicinal effects, with few residues and fewer side effects. Macleaya cordata is a perennial, erect, and tall herb of the poppy family, with the advantages of clearing away heat, detoxification, subduing swelling, and eliminating parasites. Macleaya cordata extract (MCE) has anti-inflammatory, antibacterial, and anti-tumor effects (Lin et al., 2018). Soler-Vasco et al. (2016) reported that MCE helps reduce the inflammatory responses of intestinal epithelial cells caused by enterotoxigenic Escherichia coli.
Network pharmacology, based on systems biology and network equilibrium theory, combines pharmacology and database application systems, computational chemistry and other tools to directly identify drugs and disease targets from a large amount of data and explain the disease development process and the drug–organism interaction mechanism (Hao and Xiao, 2014; Liu et al., 2015; Zhou et al., 2020). This technique can be further combined with the holistic concept of traditional Chinese medicine (TCM) and evidence-based treatment concept to systematically analyze the mechanism of drug action in the body (Zhang et al., 2019; Wu et al., 2021; Jiashuo et al., 2022). It is well known that the chemical composition of Chinese herbal medicine is complex, whether single or herbal compounds that are, in general, multiple components acting on multiple targets and multiple pathways (Zhang et al., 2017; Yuan et al., 2022). This property leads to difficulties in elucidating the mechanisms of Chinese herbal medicine at the cellular and molecular levels in vivo (Zhang et al., 2017). The interaction between a disease and biomolecule can be determined by combining network pharmacology and TCM. Molecular docking techniques are used to study the binding sites and interactions between small molecules of drugs and target macromolecules, and these can also be used to simulate the affinity process and calculate the binding energy between the two, thus improving the efficiency and relevance of Chinese herbal medicine screening (Pagadala et al., 2017; Han et al., 2018; Salmaso and Moro, 2018; Jiao et al., 2021). So network pharmacology and molecular docking technologies were used in this study to predict and verify the core targets and signaling pathways of the four active compounds of MCE in the treatment of enteritis and build a multi-interaction network of “drug–active compound–target–pathway–disease.” The mechanism of the four active compounds of MCE in the treatment of enteritis was comprehensively and systematically analyzed to provide a theoretical basis for an in-depth study of their roles in the prevention and treatment of enteritis.
Literature search engines such as China National Knowledge Infrastructure (https://www.cnki.net/) and PubMed (https://pubmed.ncbi.nlm.nih.gov/) were used to review the main active substances of MCE and their active compounds. The 2D structures of the active compounds were consulted and downloaded from the PubChem database (https://pubchem.ncbi.nlm.nih.gov/). Then, the 2D structures were imported into the PharmMapper database (http://www.lilab-ecust.cn/pharmmapper/) to obtain UniPlot (Li et al., 2019). Furthermore, protein encodings were converted to gene names using the UniProt database (https://www.uniprot.org/) to obtain the targets for the active compounds.
The GeneCards database (https://www.genecards.org/) was searched using “enteritis” as the keyword, and the results were exported in an Excel format. The data were de-duplicated and filtered based on the relevance score of >1, and the resulting data were considered enteritis-related targets.
The active compound targets of MCE obtained in Section 2.1 and the targets of enteritis obtained in Section 2.2 were imported into List2 and List1 of the Venny 2.1.0 online website (https://bioinfogp.cnb.csic.es/tools/venny/), respectively, to obtain the drug–disease intersection target Venn diagrams. The intersection site in the diagram was clicked to obtain the intersection target in the result table, which gave the potential target of MCE for the treatment of enteritis.
The intersection targets were imported into the STRING database (https://cn.stringdb.org/) and set as “Homo sapiens” with a confidence level of 0.900, hidden unassociated proteins and protein–protein interaction (PPI) data were exported. PPI data were viewed using Cytoscape 3.7.1 software to build the PPI network graphs. The core targets were screened with the topological analysis based on the following criteria: degree ≥2* degree mean, betweenness centrality ≥betweenness centrality mean, and closeness centrality ≥closeness centrality mean. The higher the values of these three criteria, the more important the target was in the network (Xu et al., 2022).
The PPI data were de-duplicated and imported into the Metascape database (https://metascape.org/), and “Homo sapiens” was used as a species for Gene Ontology (GO) enrichment and Kyoto Encyclopedia of Genes and Genomes (KEGG) pathway analyses, which were arranged in the descending order of significance, −LogP. The top 10 enriched biological processes (BP), cellular components (CC), and molecular functions (MF) under the GO classification and the top 20 enriched KEGG pathways were then imported into Bioinformatics (http://www.bioinformatics.com.cn/) for visualization.
The drug, active compounds, intersection targets, pathway, and disease were imported into Cytoscape 3.7.1 software to construct the “drug–active compound–target–pathway–disease” network diagram and analyze the relationship among them. The more the number of connection nodes, the more the number of biological functions the nodes were involved in and the more their importance in the network (Gong et al., 2021).
The 3D structures of the active compounds and core targets were downloaded from the PubChem and PDB databases (https://www.rcsb.org/), respectively. The SDF files of the active compounds were converted to PDB files using Open Babel GUI software. PyMOL software was used to dehydrate and desolvate the core targets. AutoDock Tools software was used to hydrogenate the active compounds and set them as the ligand and to hydrogenate the core targets and set them as the receptor. Molecular docking of the receptor and ligand was performed using AutoDock Tools software, and the docking result with the lowest binding energy was selected and imported into PyMOL software for visual analysis.
The literature review revealed that alkaloids are the main active substances in MCE (Zeng et al., 2013; Ni et al., 2016; Lin et al., 2018). Furthermore, the active compounds are sanguinarine (SAN), chelerythrine (CHE), protopine (PRO), and allocryptopine (ALL) (Table 1), yielding 200, 229, 171, and 156 targets, respectively, and yielding 269 targets after de-weighting (Supplementary Table S1).
From the GeneCards database, 9,027 enteritis-related targets were retrieved, and using de-duplication and screening based on the relevance score of >1, 1,237 enteritis-related targets were obtained, including actin gamma 2 (ACTG2), smooth muscle, RET proto-oncogene (RET), endothelin receptor type B (EDNRB), endothelin 3 (EDN3), SRY-box transcription factor 10 (SOX10), nucleotide-binding oligomerization domain-containing 2 (NOD2), glial cell-derived neurotrophic factor (GDNF), interleukin-6 (IL-6), tetratricopeptide repeat domain 37 (TTC37), and transthyretin (TTR) (Supplementary Table S2).
In total, 269 targets of the four active compounds of MCE and 1,237 targets of enteritis obtained in Section 3.1 and Section 3.2 were imported into Venny 2.1.0 for constructing Venn diagrams. Furthermore, 70 intersection targets were obtained, which were potential targets for the pharmacological effects of the four active compounds of MCE in the treatment of enteritis, including transthyretin (TTR), albumin (ALB), protein kinase C theta type (PRKCQ), interleukin-2 (IL-2), mast/stem cell growth factor receptor kit (KIT), insulin-like growth factor-I (IGF-1), peroxisome proliferator-activated receptor gamma (PPARG), tyrosine-protein kinase (BTK), vitamin D3 receptor (VDR), and retinol-binding protein 4 (RBP4) (Supplementary Table S3), which accounts for 4.9% of all targets (Figure 1).
FIGURE 1. Venn diagram for the intersection of targets of enteritis and the four active compounds of MCE. The purple color on the left is the enteritis target, and the yellow color on the right is the target of the four active compounds of MCE; their intersection represents potential targets for the pharmacological effects of the four active compounds of MCE in the treatment of enteritis.
Using the STRING database, the highest confidence level (0.900) was selected in the minimum required interaction score column, while other conditions remained unchanged; we exported the Excel table and obtained 49 targets after de-weighting. After importing the 49 targets into Cytoscape 3.7.1 software, a PPI network diagram of enteritis and the four active compounds of targets of MCE (Figure 2) was obtained, with 49 nodes and 120 connections. In addition, topological analysis of these 49 nodes was performed using the plugin CytoNCA. According to the requirements, degree ≥ 9.795918367, betweenness centrality ≥0.044380518, and closeness centrality ≥0.343112989, the core targets were screened, including heat-shock protein 90 alpha family class A member 1 (HSP90AA1), mitogen-activated protein kinase 1 (MAPK1), HRas proto-oncogene, GTPase (HRAS), Janus kinase 2 (JAK2), and AKT serine/threonine kinase 1 (AKT1) (Table 2).
FIGURE 2. Protein–protein interaction (PPI) network diagram of the intersection target of enteritis and the four active compounds of MCE. Nodes represent targets, and the size and color are proportional to the degree value; the higher the degree value, the larger the node, which is indicated with colors from blue to red. Edges represent the association between targets, and thickness is related to the combination score. The thicker the line, the higher the binding between targets.
GO enrichment analysis provided a systematic reflection of the mechanism of the four active compounds of MCE in the treatment of enteritis. A total of 749 BP, 47 CC, and 64 MF were obtained (Supplementary Table S4) via Metascape, and the top 10 enriched BP, CC, and MF are visualized and detailed in Figure 3. BP was primarily involved in the positive regulation of transferase and kinase activity, protein phosphorylation, positive regulation of cell motility, and response to hormone and reproductive system development. CC primarily included vesicle lumen, secretory granule lumen, and cytoplasmic vesicle lumen, whereas MF included protein serine/threonine/tyrosine kinase activity, protein kinase activity, phosphotransferase activity, nitric oxide synthase regulatory activity, and protein serine/threonine kinase activity.
FIGURE 3. Visualization of Gene Ontology (GO) enrichment analysis. Biological process (BP), cellular component (CC), and molecular function (MF) of GO enrichment analysis are shown as green, orange, and purple bars, respectively.
The KEGG pathway analysis allows the prediction of the role of protein interaction networks in different cell activities. In this study, Metascape was used for KEGG pathway analysis, and 142 pathways were obtained (Supplementary Table S5), among which the top 20 enriched pathways are shown in Figure 4. The analysis showed that cancer-related pathways, PI3K-Akt signaling pathway, MAPK signaling pathway, FoxO signaling pathway, lipid and atherosclerosis, and Rap1 signaling pathway were mainly involved.
FIGURE 4. Kyoto Encyclopedia of Genes and Genomes (KEGG) signaling pathway enrichment analysis. Bubble color and size distribution represent −logP values and the number of genes involved in this signaling pathway, respectively.
To visualize the potential molecular mechanism of the four active compounds of MCE in the treatment of enteritis, a “drug–active compound–target–pathway–disease” network diagram was built using Cytoscape 3.7.1 software. In the diagram, there are 96 nodes and 410 connections (Figure 5). Furthermore, 58 targets are connected to CHE, 55 to SAN, 44 each to ALL and PRO, 17 to the PI3K-Akt signaling pathway, 14 each to the cancer-related and MAPK signaling pathways, and 13 to the FoxO signaling pathway.
FIGURE 5. Drug–active compound–target–pathway–disease network diagram. The yellow hexagon represents MCE, the red hexagon represents enteritis, four blue squares represent MCE active compounds, 20 green squares represent pathways, and 70 purple diamonds represent intersection targets.
To determine the binding between small molecules of the drug and target macromolecules, binding energy was used: less than −5 kcal/mol indicates good affinity; the lower the binding energy, the stronger the affinity (Li et al., 2022). Molecular docking between the four active compounds of MCE and the five core targets was performed based on the results of the aforementioned analysis: the ligands were SAN, CHE, PRO, and ALL, and the receptors were HSP90AA1 (PDB ID: 7S9H), MAPK1 (PDB ID: 6G54), HRAS (PDB ID: 7JII), JAK2 (PDB ID: 7F7W), and AKT1 (PDB ID: 7NH5). The molecular docking binding energies were all less than −5 kcal/mol, indicating that the active compounds and the target had high affinity and could stably bind (Figure 6).
FIGURE 6. Heatmap of the molecular docking results between active compounds and core targets. The vertical coordinates represent the four active compounds of MCE, the horizontal coordinates represent the core targets, and the colors from red to white indicate the strongest to weakest binding abilities.
The best binding results were obtained for SAN and PRO with AKT1 and for CHE and ALL with HSP90AA1 (Figures 7A–D). SAN formed one hydrogen bond with Ser 205 in AKT1, PRO formed one hydrogen bond with Arg 273 and one hydrogen bond with Thr 87 in AKT1, CHE formed four hydrogen bonds with two Lys 74 residues in HSP90AA1, and ALL formed one hydrogen bond with Leu 76 in HSP90AA1 and three hydrogen bonds with two Lys 74 residues.
FIGURE 7. Best docking combinations for the four active compounds of MCE and core targets. The green model represents the MCE active compounds; the purple model represents the core targets, and the binding sites and binding distances of active compounds and target proteins are shown in the figure: (A) SAN–AKT1; (B) PRO–AKT1; (C) CHE–HSP90AA1; and (D) ALL–HSP90AA1.
According to the literature, the primary constituents of MCE are isoquinoline alkaloids, which contain active compounds such as SAN, CHE, PRO, and ALL (Zeng et al., 2013; Ni et al., 2016; Lin et al., 2018). These compounds possess anti-inflammatory, antibacterial, insecticidal, anti-tumor, and intestinal health-improving functions. Wang et al. (2021) showed that MCE aids the increase in the height of intestinal villi and the decrease in crypt depth, thus improving nutrient utilization. It also increases the numbers of Lactobacillus and decreases those of Escherichia–Shigella, Streptococcus, Proteobacteria, and Actinobacteriota, thus ensuring optimal intestinal health. The four active compounds of MCE primarily act on AKT1 and MAPK1 to activate PI3K-Akt and MAPK signaling pathways for treating enteritis. MCE provides inflammation regulation through PI3K-Akt and MAPK signaling pathways and shows anti-inflammatory effects by inhibiting MAPK activation to alter the synthesis of inflammatory mediators (Dong et al., 2021; Zeng et al., 2022). These results are consistent with our network pharmacology prediction.
According to the network pharmacology analysis, there are 269 targets for the four active compounds of MCE, 1,237 targets for enteritis, and 70 targets for the intersection of drug and disease targets. The construction of the PPI network helped explore the core targets, and HSP90AA1, MAPK1, HRAS, JAK2, and AKT1 were obtained according to condition screening. HSPs are primarily induced by stressors, and the percentage of cellular proteins will elevate when the organism is subjected to microbial stimuli, such as parasites, fungi, viruses, or heat shock proteins (Zuehlke et al., 2015; Bolhassani and Agi, 2019; Shan et al., 2020). Most HSPs act as molecular chaperones for a variety of cellular processes in the organism (proper folding, activation, and assembly of proteins) (Li et al., 2019). The HSP90AA1 gene encodes the heat shock protein 90α, a stress-inducible heterodimer of the molecular chaperone HSP90 (Zuehlke et al., 2015). The regulation of inflammatory cytokines expressed by HSP90, which is elevated when stimulated by inflammation and hypoxia, is primarily achieved through the activation of the NLRP3 inflammasome and cellular transcription factor NF-κB. This activity induces the activation of caspase-1 and the maturation and secretion of the downstream pro-inflammatory factors IL-1β and IL-18 (Sarvestani and McAuley, 2017; Li et al., 2019). MAPK1 is a significant MAPK signaling pathway component of the MAPK signaling pathway (Luo et al., 2020). This target component can regulate a variety of cellular physiological and pathological functions, such as cell growth, adhesion, and differentiation through transcription, translation, and cytoskeleton rearrangement, and it is a key signaling molecule in the treatment of inflammation-related diseases (Dong et al., 2021). HRAS activates the RAS/MAPK signaling pathway, which in turn regulates gene transcription and cell cycle (cell growth, division, maturation, and differentiation) (Kodaz et al., 2017). JAK2, a member of the intracellular tyrosine kinase family, is a potential target in the treatment of ulcerative colitis (Danese et al., 2019), as it regulates pathophysiological processes, such as inflammatory response, apoptosis, and cell cycle, primarily through its involvement in the JAK-STAT signaling pathway. JAK2 activates not only STAT3 but also ERK and p38 MAPK pathways (Truong et al., 2017), regulating innate and acquired immunity and reducing chronic inflammation in the intestine (Zhao et al., 2021). AKT1, a serine/threonine protein kinase and one of the key downstream regulatory proteins of PI3K, is involved in a variety of cellular processes in vivo, such as cell proliferation, metabolism, apoptosis, and angiogenesis, using serine and/or threonine phosphorylation of downstream substrates (Hers et al., 2011). AKT1, the major isoform of Akt in endothelial cells, plays a key role in causing cardiovascular diseases, where the pathogenesis of atherosclerosis is closely linked to the inflammatory response (Chen et al., 2005; Fougerat et al., 2009; Hers et al., 2011). Thus, the four active compounds of MCE can achieve a multi-component and multi-targeted treatment for enteritis by acting on the core targets.
GO and KEGG enrichment analyses are helpful in exploring the signaling pathway of the four active compounds of MCE in the treatment of enteritis. According to GO enrichment analysis, the four active compounds of MCE helped in the treatment of enteritis primarily through the involvement of transferase and kinase activity, protein phosphorylation, and cell motility. In addition, cancer-related pathways, PI3K–Akt signaling pathway, MAPK signaling pathway, FoxO signaling pathway, and Rap1 signaling pathway play a vital role in the treatment of enteritis using the four active compounds of MCE. Because the PI3K-Akt signaling pathway enriched five core targets, it could be the main signaling pathway for processing enteritis. The PI3K-Akt signaling pathway is the main pathway in the regulation of inflammation, primarily in the regulation of inflammatory cell recruitment, expression, and activation of inflammatory mediators (Fougerat et al., 2009; Huang et al., 2011). This pathway can also promote inflammation by activating NF-κB to cause the release of inflammatory factors (IL-6 and IL-8), thereby sustaining and amplifying the inflammatory response (Huang et al., 2011). Fang et al. (2018) showed that berberine ameliorates necrotizing enterocolitis through the PI3K-Akt signaling pathway. Yan et al. (2022) showed that Huang Bai Jian Pi decoction relieves diarrhea and regulates the expression of inflammatory factors through the PI3K-Akt signaling pathway. These results suggest that the PI3K-Akt signaling pathway plays a regulatory role in enteritis, which is consistent with our network pharmacological prediction. The MAPK signaling pathway is involved in various biological processes such as immunity, stress, cell growth, apoptosis, and differentiation, and it plays a key role in the release of pro-inflammatory cytokines (Luo et al., 2020; Afroz et al., 2021). The MAPK family includes three subfamilies, namely, ERK, JNK, and p38 MAPK, which are associated with intestinal mucosal injury and are activated in inflammatory bowel disease to induce the release of cytokines such as IL-8 (Luo et al., 2020). Dong et al. (2020a) reported that Astragalus polysaccharide could alleviate lipopolysaccharide (LPS)-induced intestinal inflammation via the MAPK signaling pathway. Dong et al. (2020b) showed that oleanolic acid maintained the intestinal barrier, improved Salmonella typhimurium-induced diarrhea in mice, and reduced intestinal inflammation through the MAPK signaling pathway. Therefore, the MAPK signaling pathway plays a regulatory role in enteritis, a result consistent with our network pharmacological prediction. Thus, it is evident that the four active compounds of MCE possess pharmacological effects in the treatment of enteritis through multiple pathways.
Based on the aforementioned network pharmacological analysis, four active compounds, SAN, CHE, PRO, and ALL, are stably bound to five core targets, HSP90AA1, MAPK1, HRAS, JAK2, and AKT1, which was validated using molecular docking. Thus, the interaction between the active compounds and the target may be one of the most important pharmacological mechanisms of the four active compounds of MCE in the treatment of enteritis, an outcome that needs to be verified by further experiments.
However, the potential pharmacological mechanism of the four active compounds of MCE in the treatment of enteritis was explored using only network pharmacology and molecular docking techniques. In addition, the current network information is not comprehensive, and the real-time data are not available in the databases. Therefore, the findings of this study need to be further validated in animals for better clinical applications.
The four main active compounds of MCE involved in the treatment of enteritis are SAN, CHE, PRO, and ALL, and the five core targets are HSP90AA1, MAPK1, HRAS, JAK2, and AKT1. PI3K-Akt and MAPK, obtained through network pharmacology and molecular docking technologies, are the main signaling pathways. These results are of great significance for further exploration of the potential pharmacological mechanism of MCE in the treatment of enteritis.
Publicly available datasets were analyzed in this study. The names of the repository/repositories and accession number(s) can be found in the article/Supplementary Material, further inquiries can be directed to the corresponding author.
Conceptualization, PY, CZ, and LC; methodology, PY and HH; software, PY and QM; validation, XL, LD, and LZ; formal analysis, YP, ZC, BS, and DH; investigation, HH; resources, LX; data curation, LD and LZ; writing—original draft preparation, YP, ZC, HH, LX, DL, and ZL; writing—review and editing, SB, HD, QM, and LC; visualization, YP; supervision, LC; project administration, LC, SB, and HD; funding acquisition, LC, SB, and HD. All authors have read and agreed to the published version of the manuscript.
This work was carried out with support from the National Natural Science Foundation of China (Grant No. 32002325), the Chongqing Technical Innovation and Application Development Special Project (Grant No. cstc2019jsccx-lyjsBX0003 and cstc2021jscx-lyjsAX0008), the Natural Science Foundation of Chongqing, China (Grant No. 2022NSCQ-MSX5267), and the Fundamental Research Funds for the Central Universities (Grant No. SWU-KT22011).
The authors declare that the research was conducted in the absence of any commercial or financial relationships that could be construed as a potential conflict of interest.
All claims expressed in this article are solely those of the authors and do not necessarily represent those of their affiliated organizations, or those of the publisher, the editors, and the reviewers. Any product that may be evaluated in this article, or claim that may be made by its manufacturer, is not guaranteed or endorsed by the publisher.
The Supplementary Material for this article can be found online at: https://www.frontiersin.org/articles/10.3389/fphys.2023.1175227/full#supplementary-material
Afroz, S., Fairuz, S., Joty, J. A., Uddin, M. N., and Rahman, M. A. (2021). Virtual screening of functional foods and dissecting their roles in modulating gene functions to support post COVID-19 complications. J. Food Biochem. 45, e13961. doi:10.1111/jfbc.13961
Bolhassani, A., and Agi, E. (2019). Heat shock proteins in infection. Clin. Chim. Acta 498, 90–100. doi:10.1016/j.cca.2019.08.015
Chen, J., Somanath, P. R., Razorenova, O., Chen, W. S., Hay, N., Bornstein, P., et al. (2005). Akt1 regulates pathological angiogenesis, vascular maturation and permeability in vivo. Nat. Med. 11, 1188–1196. doi:10.1038/nm1307
Cleary, R. K. (1998). Clostridium difficile-Associated diarrhea and colitis: Clinical manifestations, diagnosis, and treatment. Dis. Colon Rectum 41, 1435–1449. doi:10.1007/BF02237064
Dacal, E., Köster, P. C., and Carmena, D. (2020). Diagnóstico molecular de parasitosis intestinales. Enferm Infecc Microbiol Clin (Engl38 (1), 24–31. doi:10.1016/j.eimc.2020.02.005
Danese, S., Argollo, M., Le Berre, C., and Peyrin-Biroulet, L. (2019). JAK selectivity for inflammatory bowel disease treatment: Does it clinically matter? Gut 68, 1893–1899. doi:10.1136/gutjnl-2019-318448
Dixon, B. R. (2021). Giardia duodenalis in humans and animals - transmission and disease. Res. Vet. Sci. 135, 283–289. doi:10.1016/j.rvsc.2020.09.034
Dong, N., Li, X., Xue, C., Zhang, L., Wang, C., Xu, X., et al. (2020a). Astragalus polysaccharides alleviates LPS-induced inflammation via the NF-κB/MAPK signaling pathway. J. Cell Physiol. 235, 5525–5540. doi:10.1002/jcp.29452
Dong, N., Xue, C., Zhang, L., Zhang, T., Wang, C., Bi, C., et al. (2020b). Oleanolic acid enhances tight junctions and ameliorates inflammation in Salmonella typhimurium-induced diarrhea in mice via the TLR4/NF-κB and MAPK pathway. Food Funct. 11, 1122–1132. doi:10.1039/c9fo01718f
Dong, Z., Liu, M., Zou, X., Sun, W., Liu, X., Zeng, J., et al. (2021). Integrating network pharmacology and molecular docking to analyse the potential mechanism of action of Macleaya cordata (willd.) R. Br. In the treatment of bovine hoof disease. Vet. Sci. 9, 11. doi:10.3390/vetsci9010011
Fang, C., Xie, L., Liu, C., Fu, C., Ye, W., Liu, H., et al. (2018). Berberine ameliorates neonatal necrotizing enterocolitis by activating the phosphoinositide 3-kinase/protein kinase B signaling pathway. Exp. Ther. Med. 15, 3530–3536. doi:10.3892/etm.2018.5858
Fougerat, A., Gayral, S., Malet, N., Briand-Mesange, F., Breton-Douillon, M., and Laffargue, M. (2009). Phosphoinositide 3-kinases and their role in inflammation: Potential clinical targets in atherosclerosis? Clin. Sci. (Lond). 116, 791–804. doi:10.1042/CS20080549
Gong, P., Wang, D., Cui, D., Yang, Q., Wang, P., Yang, W., et al. (2021). Anti-aging function and molecular mechanism of Radix Astragali and Radix Astragali preparata via network pharmacology and PI3K/Akt signaling pathway. Phytomedicine 84, 153509. doi:10.1016/j.phymed.2021.153509
Han, K., Zhang, L., Wang, M., Zhang, R., Wang, C., and Zhang, C. (2018). Prediction methods of herbal compounds in Chinese medicinal herbs. Molecules 23, 2303. doi:10.3390/molecules23092303
Hao, D. C., and Xiao, P. G. (2014). Network pharmacology: A rosetta stone for traditional Chinese medicine. Drug Dev. Res. 75, 299–312. doi:10.1002/ddr.21214
Hers, I., Vincent, E. E., and Tavaré, J. M. (2011). Akt signalling in health and disease. Cell Signal 23, 1515–1527. doi:10.1016/j.cellsig.2011.05.004
Huang, X. L., Xu, J., Zhang, X. H., Qiu, B. Y., Peng, L., Zhang, M., et al. (2011). PI3K/Akt signaling pathway is involved in the pathogenesis of ulcerative colitis. Inflamm. Res. 60, 727–734. doi:10.1007/s00011-011-0325-6
Jiao, X., Jin, X., Ma, Y., Yang, Y., Li, J., Liang, L., et al. (2021). A comprehensive application: Molecular docking and network pharmacology for the prediction of bioactive constituents and elucidation of mechanisms of action in component-based Chinese medicine. Comput. Biol. Chem. 90, 107402. doi:10.1016/j.compbiolchem.2020.107402
Jiashuo, W. U., Fangqing, Z., Zhuangzhuang, L. I., Weiyi, J., and Yue, S. (2022). Integration strategy of network pharmacology in traditional Chinese medicine: A narrative review. J. Tradit. Chin. Med. 42, 479–486. doi:10.19852/j.cnki.jtcm.20220408.003
Kodaz, H., Kostek, O., Hacioglu, M. B., Erdogan, B., Kodaz, C. E., Hacibekiroglu, I., et al. (2017). Frequency of RAS mutations (KRAS, NRAS, HRAS) in human solid cancer. Eurasian J. Med. Oncol. 7, 1–7. doi:10.14744/ejmo.2017.22931
Leon-Velarde, C. G., Jun, J. W., and Skurnik, M. (2019). Yersinia phages and food safety. Viruses 11, 1105. doi:10.3390/v11121105
Li, C., Zhang, W., Yu, Y., Cheng, C., Han, J., Yao, X., et al. (2019). Discovery of the mechanisms and major bioactive compounds responsible for the protective effects of Gualou Xiebai Decoction on coronary heart disease by network pharmacology analysis. Phytomedicine 56, 261–268. doi:10.1016/j.phymed.2018.11.010
Li, F., Song, X., Su, G., Wang, Y., Wang, Z., Qing, S., et al. (2019). AT-533, a Hsp90 inhibitor, attenuates HSV-1-induced inflammation. Biochem. Pharmacol. 166, 82–92. doi:10.1016/j.bcp.2019.05.003
Li, X., Wei, S., Niu, S., Ma, X., Li, H., Jing, M., et al. (2022). Network pharmacology prediction and molecular docking-based strategy to explore the potential mechanism of Huanglian Jiedu Decoction against sepsis. Comput. Biol. Med. 144, 105389. doi:10.1016/j.compbiomed.2022.105389
Lim, S. C., Knight, D. R., and Riley, T. V. (2020). Clostridium difficile and one health. Clin. Microbiol. Infect. 26, 857–863. doi:10.1016/j.cmi.2019.10.023
Lin, L., Liu, Y., Huang, J., Liu, X., Qing, Z., Zeng, J., et al. (2018). Medicinal plants of the genus Macleaya (Macleaya cordata, Macleaya microcarpa): A review of their phytochemistry, pharmacology, and toxicology. Phytother. Res. 32, 19–48. doi:10.1002/ptr.5952
Liu, C., Liu, R., Fan, H., Xiao, X., Chen, X., Xu, H., et al. (2015). Quantitative susceptibility mapping: Contrast mechanisms and clinical applications. Chin. Herb. Med. 7, 3–17. doi:10.18383/j.tom.2015.00136
Luo, R., Huang, X., Yan, Z., Gao, X., Wang, P., Yang, Q., et al. (2020). Identification and characterization of MAPK signaling pathway genes and associated lncRNAs in the ileum of piglets infected by Clostridium perfringens type C. Biomed. Res. Int. 2020, 8496872. doi:10.1155/2020/8496872
Moreira Lopes, T. C., Mosser, D. M., and Gonçalves, R. (2020). Macrophage polarization in intestinal inflammation and gut homeostasis. Inflamm. Res. 69, 1163–1172. doi:10.1007/s00011-020-01398-y
Ni, H., Martínez, Y., Guan, G., Rodríguez, R., Más, D., Peng, H., et al. (2016). Analysis of the impact of isoquinoline alkaloids, derived from Macleaya cordata extract, on the development and innate immune response in swine and poultry. Biomed. Res. Int. 2016, 1352146. doi:10.1155/2016/1352146
Pagadala, N. S., Syed, K., and Tuszynski, J. (2017). Software for molecular docking: A review. Biophys. Rev. 9, 91–102. doi:10.1007/s12551-016-0247-1
Ryan, U., Hijjawi, N., and Xiao, L. (2018). Foodborne cryptosporidiosis. Int. J. Parasitol. 48, 1–12. doi:10.1016/j.ijpara.2017.09.004
Salmaso, V., and Moro, S. (2018). Bridging molecular docking to molecular dynamics in exploring ligand-protein recognition process: An overview. Front. Pharmacol. 9, 923. doi:10.3389/fphar.2018.00923
Sarvestani, S. T., and McAuley, J. L. (2017). The role of the NLRP3 inflammasome in regulation of antiviral responses to influenza A virus infection. Antivir. Res. 148, 32–42. doi:10.1016/j.antiviral.2017.10.020
Shan, Q., Ma, F., Wei, J., Li, H., Ma, H., and Sun, P. (2020). Physiological functions of heat shock proteins. Curr. Protein Pept. Sci. 21, 751–760. doi:10.2174/1389203720666191111113726
Soler-Vasco, L., Hermes, R., and Niewold, T. A. (2016). <i&gt;Macleaya cordata&lt;/i&gt; Extract Reduces Inflammatory Responses of Intestinal Epithelial Cells <i&gt;in vitro&lt;/i&gt;. Am. J. Plant Sci. 7, 1531–1537. doi:10.4236/ajps.2016.711144
Tarris, G., de Rougemont, A., Charkaoui, M., Michiels, C., Martin, L., and Belliot, G. (2021). Enteric viruses and inflammatory bowel disease. Viruses 13, 104. doi:10.3390/v13010104
Truong, A. D., Rengaraj, D., Hong, Y., Hoang, C. T., Hong, Y. H., and Lillehoj, H. S. (2017). Differentially expressed JAK-STAT signaling pathway genes and target microRNAs in the spleen of necrotic enteritis-afflicted chicken lines. Res. Vet. Sci. 115, 235–243. doi:10.1016/j.rvsc.2017.05.018
Wang, F., Yin, Y., Yang, M., Chen, J., Fu, C., and Huang, K. (2021). Effects of combined supplementation of Macleaya cordata extract and benzoic acid on the growth performance, immune responses, antioxidant capacity, intestinal morphology, and microbial composition in weaned piglets. Front. Vet. Sci. 8, 708597. doi:10.3389/fvets.2021.708597
Wu, Q., Yang, F., and Tang, H. (2021). Based on network pharmacology method to discovered the targets and therapeutic mechanism of Paederia scandens against nonalcoholic fatty liver disease in chicken. Poult. Sci. 100, 55–63. doi:10.1016/j.psj.2020.09.087
Xu, L., Song, X., Zhang, Y., Lin, N., Wang, J., and Dai, Q. (2022). Investigation of the mechanism of action of Shengxuexiaoban Capsules against primary immune thrombocytopenia using network pharmacology and experimental validation. Phytomedicine 106, 154413. doi:10.1016/j.phymed.2022.154413
Yan, Z., Zhang, K., Zhang, K., Wang, G., Wang, L., Zhang, J., et al. (2022). Huang Bai jian Pi decoction alleviates diarrhea and represses inflammatory injury via PI3K/Akt/NF-κB pathway: In vivo and in vitro studies. J. Ethnopharmacol. 292, 115212. doi:10.1016/j.jep.2022.115212
Yuan, Z., Pan, Y., Leng, T., Chu, Y., Zhang, H., Ma, J., et al. (2022). Progress and prospects of research ideas and methods in the network pharmacology of traditional Chinese medicine. J. Pharm. Pharm. Sci. 25, 218–226. doi:10.18433/jpps32911
Zeng, J., Liu, Y., Liu, W., Liu, X., Liu, F., Huang, P., et al. (2013). Integration of transcriptome, proteome and metabolism data reveals the alkaloids biosynthesis in Macleaya cordata and Macleaya microcarpa. Plos One 8, e53409. doi:10.1371/journal.pone.0053409
Zeng, Z., Zhang, H., Gui, G., Luo, J., and Liu, S. (2022). Macleaya cordata extract modulates inflammation via inhibition of the NF-κB and MAPK signaling pathways in porcine alveolar macrophages induced by Glaesserella parasuis. Can. J. Vet. Res. 86, 254–260. in press.
Zhang, R., Yu, S., Bai, H., and Ning, K. (2017). TCM-Mesh: The database and analytical system for network pharmacology analysis for TCM preparations. Sci. Rep. 7, 2821. doi:10.1038/s41598-017-03039-7
Zhang, R., Zhu, X., Bai, H., and Ning, K. (2019). Network pharmacology databases for traditional Chinese medicine: Review and assessment. Front. Pharmacol. 10, 123. doi:10.3389/fphar.2019.00123
Zhao, Y., Luan, H., Jiang, H., Xu, Y., Wu, X., Zhang, Y., et al. (2021). Gegen Qinlian decoction relieved DSS-induced ulcerative colitis in mice by modulating Th17/Treg cell homeostasis via suppressing IL-6/JAK2/STAT3 signaling. Phytomedicine 84, 153519. doi:10.1016/j.phymed.2021.153519
Zhou, Z., Chen, B., Chen, S., Lin, M., Chen, Y., Jin, S., et al. (2020). Applications of network pharmacology in traditional Chinese medicine research. Evid. Based Complement. Altern. Med. 2020, 1646905. doi:10.1155/2020/1646905
Keywords: Macleaya cordata extract, enteritis, network pharmacology, molecular docking, mechanism
Citation: Yang P, Zhong C, Huang H, Li X, Du L, Zhang L, Bi S, Du H, Ma Q and Cao L (2023) Potential pharmacological mechanisms of four active compounds of Macleaya cordata extract against enteritis based on network pharmacology and molecular docking technology. Front. Physiol. 14:1175227. doi: 10.3389/fphys.2023.1175227
Received: 27 February 2023; Accepted: 17 April 2023;
Published: 02 May 2023.
Edited by:
Priyankar Dey, Thapar Institute of Engineering & Technology, IndiaReviewed by:
Jianxi Li, Chinese Academy of Agricultural Sciences, ChinaCopyright © 2023 Yang, Zhong, Huang, Li, Du, Zhang, Bi, Du, Ma and Cao. This is an open-access article distributed under the terms of the Creative Commons Attribution License (CC BY). The use, distribution or reproduction in other forums is permitted, provided the original author(s) and the copyright owner(s) are credited and that the original publication in this journal is cited, in accordance with accepted academic practice. No use, distribution or reproduction is permitted which does not comply with these terms.
*Correspondence: Liting Cao, Y2FvbGl0aW5nQHN3dS5lZHUuY24=
†These authors have contributed equally to this work and share first authorship
Disclaimer: All claims expressed in this article are solely those of the authors and do not necessarily represent those of their affiliated organizations, or those of the publisher, the editors and the reviewers. Any product that may be evaluated in this article or claim that may be made by its manufacturer is not guaranteed or endorsed by the publisher.
Research integrity at Frontiers
Learn more about the work of our research integrity team to safeguard the quality of each article we publish.