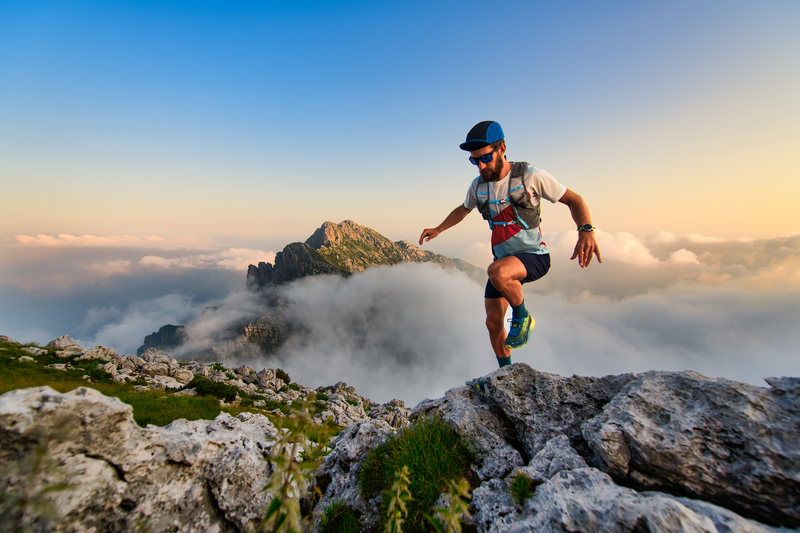
94% of researchers rate our articles as excellent or good
Learn more about the work of our research integrity team to safeguard the quality of each article we publish.
Find out more
ORIGINAL RESEARCH article
Front. Physiol. , 08 March 2023
Sec. Exercise Physiology
Volume 14 - 2023 | https://doi.org/10.3389/fphys.2023.1066325
This article is part of the Research Topic Chronic adaptations and acute adjustments in the behavior of the neuromusculoskeletal system in health and pathology View all 9 articles
Visual feedback that reinforces accurate movements may motivate skill acquisition by promoting self-confidence. This study investigated neuromuscular adaptations to visuomotor training with visual feedback with virtual error reduction. Twenty-eight young adults (24.6 ± 1.6 years) were assigned to error reduction (ER) (n = 14) and control (n = 14) groups to train on a bi-rhythmic force task. The ER group received visual feedback and the displayed errors were 50% of the real errors in size. The control group was trained with visual feedback with no reduction in errors. Training-related differences in task accuracy, force behaviors, and motor unit discharge were contrasted between the two groups. The tracking error of the control group progressively declined, whereas the tracking error of the ER group was not evidently reduced in the practice sessions. In the post-test, only the control group exhibited significant task improvements with smaller error size (p = .015) and force enhancement at the target frequencies (p = .001). The motor unit discharge of the control group was training-modulated, as indicated by a reduction of the mean inter-spike interval (p = .018) and smaller low-frequency discharge fluctuations (p = .017) with enhanced firing at the target frequencies of the force task (p = .002). In contrast, the ER group showed no training-related modulation of motor unit behaviors. In conclusion, for young adults, ER feedback does not induce neuromuscular adaptations to the trained visuomotor task, which is conceptually attributable to intrinsic error dead-zones.
A visuomotor task requires the coupling of visual targets to effector motor behaviors with error-based negative feedback (Taylor et al., 2014). Visual feedback sensitivity could affect effector motor behaviors, including changes in common synaptic inputs and discharge patterns of spinal motor neurons (Laine et al., 2014; Farina et al., 2017). In addition to the error-based negative feedback, a reward-based action-selection process can reinforce positive aspects of movement execution to advance motor learning. The basal ganglia (Kim et al., 2017) and parietal cortex (Mutha et al., 2011) are primarily involved in reward processing. Researchers have shown that errorless practice in the early stage of skill acquisition fosters implicit learning (White et al., 2014; Kal et al., 2018), and motor skills are more resistant to degradation in a pressure situation (Lee et al., 2016). There is a critical balance of error making and error avoidance to optimize motor learning (Kelley, 1969; Krakauer and Mazzoni, 2011; Lee et al., 2016).
Variations in the error size of feedback can develop a new calibration process for performance adjustments. Regardless of the feedback form, virtually signaling a worse outcome (or a greater error size) than the real performance is known as error amplification (EA) feedback (Kao et al., 2013; Abdollahi et al., 2014; Chen et al., 2017a). EA could tax the limited attention resources for processing exaggerated error information (Milanese et al., 2016; Marchal-Crespo et al., 2017; Milanese et al., 2017). In contrast, error reduction (ER) feedback displays smaller execution errors than actual performance feedback does (Hwang et al., 2017; Hwang et al., 2020). ER is associated with some behavioral advantages: It positively reinforces task success and reduces stress from committing errors, such as by enhancing motivation and self-efficacy during task completion (Hwang et al., 2020). Conceptually, ER is a trade-off between the error-based adaptation and reward-based action-selection processes. Central control of motor units in a static force task could vary with the use of ER; such variation would manifest as the less coherent discharge of motor units with higher recruitment thresholds (Hwang et al., 2017). Although ER seems to temporarily undermine task performance in skilled subjects (Hwang et al., 2017), it might improve task performance by reducing performance stress during the early stages of skill acquisition (Ong et al., 2010).
To our knowledge, no previous studies have investigated the effect of visuomotor training with ER for young adults from the aspects of force dynamics and motor unit behaviors. For virtual minimization of execution errors, ER could reinforce correct schemas for less performance stress in the initial skill acquisition (Ong et al., 2010; Hwang et al., 2020). Hence, the subjects could be better motivated and thus achieve a superior learning effect after training (Chiviacowsky and Wulf, 2007; Saemi et al., 2012). For potential perception-induced motor facilitation, the purpose of this study was to contrast behavioral and neurophysiological mechanisms underlying short-term training with and without ER for a bi-rhythmic force task. Namely, the participants learned to couple a force trajectory to a target signal of combined sinusoidal waves of two different frequencies, with half of participants receiving ER feedback. We hypothesized that ER training would result in a better performance than would training with feedback with no reduction in errors, based on our previous results that showed potential immediate effect of visual ER for older adults (Hwang et al., 2020). Regarding the potential benefit of visual ER, ER training was hypothesized to increase coherent discharge at the target frequencies and reduce the discharge variability of motor units for young adults during a bi-rhythmic force task.
Twenty-eight healthy adults were randomly assigned to one of two groups: an error reduction (ER) group (n = 14; 7 males, 9 females, mean age ±SD = 24.6 ± 1.6 years) and a control group (n = 14; 8 males, 8 females, mean age ±SD = 25.2 ± 1.8 years). They were self-reported to be right-hand dominant without known history of neuromuscular disease. The subjects visited the laboratory on 3 occasions (pre-test, training visits, and post-test) scheduled on three successive days. The study was approved by the Chung-Shan Medical University Hospital Institutional Review Board (No.CS2-16072), and all subjects provided written informed consent for the experiment.
This study used a randomized, repeated measures, between-groups, parallel design. The participants visited the laboratory 3 times at 1-day intervals (Figure 1). During the first visit for the pre-test (Day 1), maximal voluntary contraction (MVC) of isometric index abduction was defined as the highest value of three successive MVC tests of 3 s, which were separated by 3-min rest periods. Three trials of a bi-rhythmic force task under visual feedback were assessed during the pre-test visit. The participants were seated 60 cm in front of a computer monitor, with the forearm of the dominant limb resting and restrained on a thermoplastic splint. They exerted the isometric force by index abduction of the dominant hand to couple a target signal on a computer screen. The target signal comprised a 3-s latent period, a 3-s ramp-up/ramp-down phase to 30% MVC, and 3 s of the static level of 30% MVC at the beginning and end of the contraction. In the window of interest (9th–35th seconds), the bi-rhythmic force tasks required the participants to exert combined sinusoidal forces of 0.2 Hz and 0.5 Hz that fluctuated around 30% ± 2% MVC (Figure 1, lower left). Each contraction trial was 44 s. Rest periods of at least 2 min were allowed between the experimental trials in the pre-test. The particular forms of contraction were designed for EMG decomposition using a previous proof-of-algorithm. In addition, the bi-rhythmic force task was expected to add task difficulty and performance stress, unlike the static and mono-rhythmic force tasks used in the previous studies, which manipulated the error size in the visual feedback (Chen et al., 2017a; Chen et al., 2017b; Chen et al., 2017c; Hwang et al., 2020). On Day 2, the practice session contained 15 contraction trials of the same task scheme used on Day 1. Rest periods of 1 min were allowed between training trials. During the final visit for the post-test (Day 3), the participants completed three trials of the bi-rhythmic force task identical to the pre-test.
FIGURE 1. Experimental training protocol. The pre-test (Day1) and post-test (Day 3) consisted of a bi-rhythmic force task to contrast the short-term training effect using feedback with no reduction in errors (control) and error reduction (ER) feedback. The bi-rhythmic task required the subjects to track a visual target that combined sinusoidal waves of 0.2 Hz and 0.5 Hz fluctuating around 30% ± 2% MVC (subplot in the lower left). With simple mathematical transformation (subplot in the lower right), the subject perceived errors reduced by 50% with error reduction feedback during training. The force output displayed on the monitor (visualized force, VF) was equal to the sum of half of the real force (RF) plus half of the target signal (T) (VF = 0.5*(RF + T)). Hence, the size of the visualized tracking error was half of the real error (RE) of the force-tracking task (VE = T-VF = T-0.5*(RF + T) = 0.5*(T-RF) = 0.5*RE). The training task in Day 2 was the same bi-rhythmic force task.
The subjects in the ER and control groups received different forms of visual feedback when they practiced the bi-rhythmic force tasks. Subjects in the ER group received feedback that was reduced to half the size of the no reduction in errors during training. The force output displayed on the monitor (visualized force, VF) was equal to the sum of half of the real force (RF) plus half of the target signal (T) (VF = 0.5*RF + 0.5*T) (Chen et al., 2017b; Hwang et al., 2017). The size of the visualized tracking error (VE) was reduced so that the participant saw only half of the real error (RE) of the force-tracking task (VE = 0.5*RE) (Figure 1, lower right). The control group was trained with visual feedback that guided force-tracking with feedback with no reduction in errors. On Day 1 and Day 3, the ER and control groups performed bi-rhythmic force-tracking visually guided with feedback with no reduction in errors in the pre-test and post-test.
A force transducer (Model: MB-100, Interface Inc., United States of America) was used to measure the force at the dominant index finger. The force signal was sampled at 1 kHz with an analog-to-digital converter (model USB6251; National Instruments, Austin, United States of America) controlled by a custom program on a LabVIEW platform (LabVIEW v.8.5, National Instruments Inc., Austin, TX, United States of America). A multi-electrode surface EMG with 5 surface pin sensors (0.5 mm diameter) at the corners of a 5 × 5 mm square (Bagnoli sEMG system, Delsys Inc., United States of America) was synchronized to detect the muscle activity of the first dorsal interosseous (FDI) muscle. The electrode position in the pre-test was replicated at every visit to confirm consistent electrode placement in the pre-test and post-test. The EMG signals from each pin sensor were amplified (gain = 1,000) and band-pass filtered (cut-off frequencies of 20 Hz and 450 Hz), followed by a digitization process at a rate of 20 kHz (De Luca et al., 2015a). EMG data were recorded with EMG works v.4.1 (Delsys Inc., United States of America).
The force signal was first low-pass filtered (cut-off frequency: 6 Hz) to preclude high-frequency noises and center on the effects of visuo-motor processes on force outputs in the 0–4 Hz band. Only the force output in the window of interest (9th–35th seconds) was analyzed. For the pre-test, training session, and post-test, task error in the time domain was denoted as the root mean square (RMS) of the mismatch between the target signal and filtered force output (ErrRMS). During the practice session, the task errors of the 1st–15th trials were standardized with task errors of the first trial (or normalized task error). Estimated with the FFT-based Welch method (segment length: 3.25 s, overlapping time segment: 25% window length), task accuracy in the spectral domain was represented with a summated peak amplitude of the target frequencies (0.2 Hz and 0.5 Hz) (P0.2+0.5Hz) in the amplitude-normalized power spectra of the tracking force. The spectral resolution was 0.002 Hz.
With the Precision Decomposition III algorithm (version 1.1, Delsys, Inc., Natick, MA, United States of America) (De Luca et al., 2006; Nawab et al., 2010), multi-channel surface EMG signals were decomposed to the action potential “templates” of motor unit action potential trains (MUAPTs). The decomposition algorithm is reported to reliably detect motor unit activities during rhythmic contractions, rendering motor unit (MU) spike trains having values of 0 or 1 (De Luca et al., 2015a). Only MUs with decomposition accuracy rates higher than 90% were further analyzed (De Luca et al., 2006; Nawab et al., 2010), according to the results of the Decomposition–Synthesis–Decomposition–Compare (DSDC) test. The discharge variables of the motor units in the time window of interest were determined from the whole decomposed EMG data. ISImean was the mean value of all inter-spike intervals for an individual MUAPT, and M-ISIGAV was the averaged value of the ISImean for all motor units. The temporal variability of a single MU was determined using the coefficient of variation of ISI (CV-ISI) in one MUAPT, and CV-ISIGAV was the mean value of CV-ISI for all MUs in an experimental trial. The cumulative discharge rate was characterized by the convolution of the cumulative spike trains of properly-identified motor units with a Hanning window of 400 ms (Figure 2). The cumulative discharge rate was divided by the number of properly-identified MUs in an experimental trial to obtain the mean discharge trace (MDT), which highlights the low-frequency neural drive to a muscle by suppressing independent synaptic noises (Farina and Negro, 2015; Farina et al., 2016; Hwang et al., 2019). The power spectrum of the MDT was estimated with a fast Fourier transform and the same Welch method with the same parametric settings was used for the force data. The summated peak amplitudes of target frequencies at 0.2 Hz and 0.5 Hz (P0.2+0.5 Hz) in the MDT were also determined.
FIGURE 2. Decomposition results of surface electromyography (EMG) and acquisition of mean discharge trace (MDT) from the cumulative spike train. The smoothing process was performed by convolution of the cumulative spike trains of all identifiable motor units with a Hanning window of 400 ms in duration. Root mean square and spectrum analysis were applied to the MDT in the pre-test and post-test.
Hotelling’s T-squared statistics were used to examine the group differences (ER vs control) in behavioral performances, including the task accuracy (ErrRMS and P0.2+0.5 Hz of force output). Hotelling’s T-squared statistics were also used to examine the group differences (ER vs control) in the discharge variables of individual motor units (M-ISIGAV and CV-ISIGAV) and the characteristics of the mean discharge trace (RMS and P0.2+0.5 Hz). The post-hoc test was the Simes test, which would not produce over-correction with the Bonferroni test. For all post-hoc hypotheses (
The left plot of Figure 3 contrasts evolutional changes in normalized task errors (task errors of the 2nd–15th trials relative to the task error in the first trial) between the ER and control groups during the training sessions. For the control group, normalized task errors showed a decreasing trend with training sessions. The normalized task errors of the control group after the fourth practice trial were visibly smaller than the 95% confidence interval (CI), estimated from the task errors of the pre-test of the control group. In contrast, normalized task errors of the ER group were less susceptible to visuomotor practice. The normalized task errors of all practice sessions of the ER group were not evidently smaller than the 95% CI of pre-test errors. For the control group, the results of paired t-test revealed that absolute task error of the 15th trial (.694% ± .211% MVC) was significantly smaller than that of the first trial (.972% ± .359% MVC) (t13 = 5.108; p < .001). For the ER group, absolute task error of the 15th trial (.984% ± .346% MVC) did not significantly differ from that of the first trial (1.001% ± .316% MVC) (t13 = .243; p = .811). All fourteen participants (14/14) in the control group consistently exhibited reductions in absolute task errors of the last practice trial (Figure 3, the right lower plot), as compared with that in the first practice trial. In contrast, the task errors of eight of the fourteen participants (8/14) in the ER group increased in the last practice trial (Figure 3, the right upper plot).
FIGURE 3. Evolutional changes in normalized task errors during the training session for the ER and control groups. The control group showed a decreasing trend of normalized errors across training sessions. Absolute task error was denoted as the RMS value of the mismatches between the target and tracking force (% MVC). (95% CI: 95% confidence interval of the pre-test task error normalized with task error of the first trial).
With respect to the training benefits, task accuracies in the time and frequency domains of the pre-test and post-test were indexed with the RMS values of the mismatches between the target and force signal (ErrRMS) and the combined peak amplitudes of the target frequencies (P0.2+0.5 Hz) in the force output, respectively. Figure 4 shows a typical example of pooled power spectra of tracking force in the pre-test and post-test for the ER and control groups; Table 1 shows the results of Hotelling’s T-squared statistics, which contrast the task accuracies in the time and frequency domains between the pre-test and post-test for the ER and control groups. Task accuracies between the pre-test and post-test were significantly different in the control group (p = .015, ηp2 = .505) but not in the ER group (p = .150). In the control group, ErrRMS was smaller (p = .002, ηp2 = .539) and P0.2+0.5 Hz (p = .001, ηp2 = .555) was greater in the post-test than in the pre-test.
FIGURE 4. The contrast of pooled normalized power spectra of force tracking between the pre-test and post-test from representative subjects in the ER and control groups.
TABLE 1. Means and standard errors of task accuracies in the time/spectral domains in the pre-test and post-test for the ER and control groups. (ErrRMS: root mean square of the task error; P0.2+0.5Hz: combined amplitudes of 0.2 Hz and 0.5 Hz spectral peaks in the force output).
Across individuals, the average numbers of MUs accurately decomposed in each experimental trial in the pre-test for the ER and control groups were 23.6 ± 3.3 (range: 17.3–27.7) and 24.3 ± 4.8 (range: 18.0–29.3), respectively. In the post-test, those numbers were 26.3 ± 3.6 (range: 17.7–32.3) and 26.0 ± 3.9 (range: 17.3–30.0), respectively. Modulation in motor unit behaviors was assessed with changes in discharge patterns (M-ISIGAV and CV-ISIGAV) of motor units and the characteristics of the mean discharge trace (RMS, P0.2+0.5 Hz) after training. Hotelling’s T-squared statistics were used to examine the training-related change in MU discharge pattern, and the results are summarized in Table 2(A). Only the control group showed a significant modulation of discharge variables of MUs (p = .003, ηp2 = .630); the ER group did not (p = .294). For the control group, M-ISIGAV in the post-test was greater than M-ISIGAV in the pre-test (p = .018, ηp2 = .360). Figure 5 shows the pooled power spectra of the pooled discharge trace in the pre-test and post-test from representative subjects in the ER and control groups, respectively. It was evident that the target frequencies (0.2 Hz and 0.5 Hz) in the pooled discharge trace of the representative subject in the control group were enhanced after training. Unlike training in the control group (p = .001, ηp2 = .667), training in the ER group did not modulate the mean discharge trace (p = .397, ηp2 = .143) (Table 2 (B)). Post-hoc analysis revealed that the RMS and P0.2+0.5 Hz of the mean discharge trace of the control group were tuned to the training effect. Compared with those in the pre-test, RMS was smaller (p = .017, ηp2 = .367) but P0.2+0.5 Hz was greater (p = .002, ηp2 = .550) in the post-test, respectively.
TABLE 2. Means and standard errors of variables of inter-spike interval (ISI) (A) and mean discharge trace (MDT) (B) in the pre-test and post-test for the ER and control groups. (M-ISIGAV = the averaged value of the mean inter-spike interval for all motor units; CV-ISI: the coefficient of variation of ISI; CV-ISIGAV: the mean value of the coefficient of variation of inter-spike intervals for all MUs; RMS: root mean square; P0.2+0.5Hz: the combined amplitudes of the 0.2 Hz and 0.5 Hz spectral peaks). (A).
FIGURE 5. The contrast of pooled normalized power spectra of mean discharge trace (MDT) between the pre-test and post-test from representative subjects in the ER and control groups.
Contrary to expectations, ER training on a bi-rhythmic force task did not result in significant skill improvements in the post-test. The reduction in task errors with ER was not evident in the training session. There was no evident training-related modulation of motor unit behaviors with ER. In contrast, training with feedback with no reduction in errors brought about significant task improvement, together with a greater inter-spike interval, smaller fluctuations of the pooled discharge rate, and enhanced MU discharges at the target frequencies.
It was surprising to find lack of task improvement after practice with ER for the young adults. The practice curve clearly showed a tendency of performance decline across all practice trials for the ER group, as compared with the control counterpart (Figure 3). Temporary ER-induced decline in performance was previously reported in static (Hwang et al., 2017) and rhythmic (Chen et al., 2017b) force tracking. In healthy young adults, ER feedback tended to oversimplify visuomotor control with a premature feedforward process for a false impression of task success (Chen et al., 2017b). In the initial stage of motor learning, visual error feedback can guide the subjects the way to effectively minimize committed errors by trying various movement solutions. The control group with full-size of error feedback improved their tracking performance after the third practice trial (Figure 3). However, the ER group did not reduce their task errors, except in the eighth and 10th practice trials, and their task performance had not improved at the end of the training. For the first few practice trials of the ER group, the expected beneficial effect of perceived improvement in performance might have been undermined by the destructive oversimplification strategy. This observation speaks for the existence of an intrinsic error dead-zone in the visual or sensorimotor system, as proposed by Wolpert et al. (1992). An error dead-zone indicates that error detection is a magnitude-dependent process. Only an error of at least one magnitude above the detection threshold is meaningful to a control system incorporating a feedback loop. No new corrective movements can be launched, as the control system does not respond to the range of error inputs smaller than the error dead-zone (Miall et al., 1993). However, to date, the actual size of the error dead-zone remains unclear. On average, it was at least 0.45 times the size of the execution error in the first trial. The subjects who received ER were incapable of correcting errors smaller than 0.9 times the normalized error; meanwhile, they visually perceived the execution errors at only 0.5 times their size. That is, the subjects could hardly correct the visual errors that fell into the error dead-zone (0.9*0.5 of the error size of the first trial). For the young adults, virtual perception of small errors below the dead-zone led to a false impression of task success, which led to reductions in the awareness and cognitive involvement necessary for context updating and refinement of the bi-rhythmic force tracking (Seidler et al., 2012). They prematurely finalize movement strategy without adequate corrective attempts for stratifying with the “right” performance in the latter practice trials (Chen et al., 2017b).
In line with the lack of significant practice-related changes in performance gain and force structures, ER training did not mediate MU discharge to improve their performance in the motor task in the post-test. Unlike those of the control group, motor unit behaviors did not significantly adapt to short-term ER training for mastery of the designated bi-rhythmic force task (Figures 4, 5). The skill improvement in the participants trained with the full error size was associated with the potentiated P0.2 + 0.5 Hz of the pooled discharge rate in the post-test (Table 2(B)). The scenario suggested the enhanced rate coding of motor units at the target frequency during the bi-rhythmic isometric force task (Knight and Kamen, 2007). The rhythmic discharges of motor units in unison are believed to be regulated by a neural drive of central origin (Iyer et al., 1994; De Luca et al., 2015a). Another issue pertinent to task performance is steady force control around the baseline force at 30% MVC. As force outputs are averaged twitch force of the active motor units, force variability (or unfused twitch forces) can be estimated experimentally from the size of fluctuations for summated motor unit discharge trains (Farina and Negro, 2015). Previous researches show a high resemblance between force fluctuations and cumulative series of discharge race (or MDT) (Farina and Negro, 2015; Farina et al., 2016). Based on this perspective, force variability of the baseline force can be characterized by the size of low frequency oscillations in motor unit discharge patterns under 4 Hz, or RMS value of MDT (Table 2(B)) (Vaillancourt et al., 2003; Negro et al., 2009; Castronovo et al., 2018). Unlike the control group, it is worth noting that the ER group did not demonstrate a training-related reduction in MDT size. This fact probably reflects the trained group could not add to force accuracy due to fluctuations of the baseline force level. Finally, the trained control group demonstrated a greater inter-spike interval (M_ISIGAV) in the post-test, rather than the ER group (Table 2(A)). This result suggested that the visuomotor training improved the efficiency of muscle activation in the control group, replicating the finding of a previous work on training-induced decline in EMG activity (Moore and Marteniuk, 1986; Lay et al., 2002; Brueckner et al., 2019). The improvement of the muscle activation efficiency can likely be attributed to a reduction in muscle coactivation for enhanced short-latency reciprocal inhibition during skill acquisition (Suzuki et al., 2012; Floeter et al., 2013). Unfortunately, ER training failed to develop effective neural strategies for a trained bi-rhythmic force task. After ER training, the young adults did not present either enhancement of coherent discharge at the target frequency or reduction in variability of motor unit discharge.
The primary concerns of this study were the inter-day reliability and variations in the motor units at different experimental visits, despite the electrode placement being carefully tracked across days. Several previous studies have consistently provided evidence of high intra- and inter-day absolute and relative reliability for motor unit characteristics (ICCs >0.77–0.93) with decomposition-based surface EMG across a range of submaximal contraction intensities (De Luca et al., 2015a; Martinez-Valdes et al., 2016; Colquhoun et al., 2018). Hence, motor unit variables were recommended for monitoring adaptations in a longer-term intervention study (Hoshizaki et al., 2020). In addition, the validity of EMG decomposition is another issue of debate (Farina and Enoka, 2011; De Luca et al., 2015b). We cannot completely deny the likelihood of small decomposition errors (Piotrkiewicz and Türker, 2017). To be rigorous, we applied the decompose-synthesize-decompose-compare (DSDC) test (Nawab et al., 2010; De Luca et al., 2015b) to ensure the accuracy of the decomposition results. Although the accuracy of the EMG decomposition results in this study was not independently validated, the use of the DSDC test for accuracy assessment of surface EMG decomposition was highly compatible with the results of intramuscular EMG decomposed in EMGlab software (McGill et al., 2005; Hu et al., 2014). Finally, this work did not analyze motor unit recruitment strategies between groups, acknowledging that the firing rate of a motor unit is a function of its recruitment threshold. However, this limitation is unlikely to affect the conclusions of the present work, as the force levels in both experiment groups were identical. Presumably, the recruitment thresholds of decomposed motor units were not group dependent.
The healthy young adults who receive error reduction feedback did not improve their performance in a rhythmic motor task after training and did not increase the modulation of their motor unit discharges at the target frequencies. In addition, visuomotor training with error reduction does not improve the efficiency of motor production for force generation, in light of the lack of adaptive reduction in the inter-spike intervals of motor units. Failure to improve visuomotor performance with error reduction associates with insignificant modulation of low-frequency neural drives to motor units. The present findings emphasize the role of sufficient visual error perception in learning to master human–machine interfaces.
The original contributions presented in the study are included in the article/supplementary material, further inquiries can be directed to the corresponding author.
The studies involving human participants were reviewed and approved by the Chung-Shan Medical University Hospital Institutional Review Board (No.CS2-16072). The patients/participants provided their written informed consent to participate in this study.
Y-TL: Writing-original draft; Investigation; Methodology; Resources; Visualization; Y-CC: Conceptualization; Formal analysis; Supervision; Validation; G-CC: Methodology; Software. I-SH: Conceptualization; Data curation; Project administration; Writing-review and editing; Funding acquisition.
This research was jointly supported by grants from the Ministry of Science and Technology, Taiwan, R.O.C., under Grant Nos. MOST 108-2410-H-468-021 and MOST 107-2314-B-006-017-MY3.
The authors declare that the research was conducted in the absence of any commercial or financial relationships that could be construed as a potential conflict of interest.
All claims expressed in this article are solely those of the authors and do not necessarily represent those of their affiliated organizations, or those of the publisher, the editors and the reviewers. Any product that may be evaluated in this article, or claim that may be made by its manufacturer, is not guaranteed or endorsed by the publisher.
Abdollahi, F., Case Lazarro, E. D., Listenberger, M., Kenyon, R. V., Kovic, M., Bogey, R. A., et al. (2014). Error augmentation enhancing arm recovery in individuals with chronic stroke: A randomized crossover design. Neurorehabil Neural Repair 28 (2), 120–128. doi:10.1177/1545968313498649
Brueckner, D., Göpfert, B., Kiss, R., and Muehlbauer, T. (2019). Effects of motor practice on learning a dynamic balance task in healthy young adults: A wavelet-based time-frequency analysis. Gait Posture 70, 264–269. doi:10.1016/j.gaitpost.2019.03.019
Castronovo, A. M., Mrachacz-Kersting, N., Stevenson, A., Holobar, A., Enoka, R. M., and Farina, D. (2018). Decrease in force steadiness with aging is associated with increased power of the common but not independent input to motor neurons. J. Neurophysiol. 120 (4), 1616–1624. doi:10.1152/jn.00093.2018
Chen, Y. C., Lin, L. L., Lin, Y. T., Hu, C. L., and Hwang, I. S. (2017c). Variations in static force control and motor unit behavior with error amplification feedback in the elderly. Front. Hum. Neurosci. 11, 538. doi:10.3389/fnhum.2017.00538
Chen, Y. C., Lin, Y. T., Chang, G. C., and Hwang, I. S. (2017a). Paradigm shifts in voluntary force control and motor unit behaviors with the manipulated size of visual error perception. Front. Physiol. 8, 140. doi:10.3389/fphys.2017.00140
Chen, Y. C., Lin, Y. T., Chang, G. C., and Hwang, I. S. (2017b). Perceptual influences of error size on voluntary force control during a compound sinusoidal force task. Hum. Mov. Sci. 56, 46–53. doi:10.1016/j.humov.2017.10.011
Chiviacowsky, S., and Wulf, G. (2007). Feedback after good trials enhances learning. Res. Q. Exerc Sport 78 (2), 40–47. doi:10.1080/02701367.2007.10599402
Colquhoun, R. J., Tomko, P. M., Magrini, M. A., Muddle, T., and Jenkins, N. (2018). The influence of input excitation on the inter- and intra-day reliability of the motor unit firing rate versus recruitment threshold relationship. J. Neurophysiol. 120 (6), 3131–3139. doi:10.1152/jn.00490.2018
De Luca, C. J., Adam, A., Wotiz, R., Gilmore, L. D., and Nawab, S. H. (2006). Decomposition of surface EMG signals. J. Neurophysiol. 96 (3), 1646–1657. doi:10.1152/jn.00009.2006
De Luca, C. J., Chang, S. S., Roy, S. H., Kline, J. C., and Nawab, S. H. (2015a). Decomposition of surface EMG signals from cyclic dynamic contractions. J. Neurophysiol. 113 (6), 1941–1951. doi:10.1152/jn.00555.2014
De Luca, C. J., Nawab, S. H., and Kline, J. C. (2015b). Clarification of methods used to validate surface EMG decomposition algorithms as described by Farina et al. J. Appl. Physiol. 118(8), 1084. doi:10.1152/japplphysiol.00061.2015
Farina, D., Castronovo, A. M., Vujaklija, I., Sturma, A., Salminger, S., Hofer, C., et al. (2017). Common synaptic input to motor neurons and neural drive to targeted reinnervated muscles. J. Neurosci. 37 (46), 11285–11292. doi:10.1523/JNEUROSCI.1179-17.2017
Farina, D., and Enoka, R. M. (2011). Surface EMG decomposition requires an appropriate validation. J. Neurophysiol. 105 (2), 981–982. doi:10.1152/jn.00855.2010
Farina, D., and Negro, F. (2015). Common synaptic input to motor neurons, motor unit synchronization, and force control. Exerc Sport Sci. Rev. 43 (1), 23–33. doi:10.1249/JES.0000000000000032
Farina, D., Negro, F., Muceli, S., and Enoka, R. M. (2016). Principles of motor unit physiology evolve with advances in technology. Physiology 31 (2), 83–94. doi:10.1152/physiol.00040.2015
Floeter, M. K., Danielian, L. E., and Kim, Y. K. (2013). Effects of motor skill learning on reciprocal inhibition. Restor. Neurol. Neurosci. 31 (1), 53–62. doi:10.3233/RNN-120247
Hoshizaki, T., Clancy, E. A., Gabriel, D. A., and Green, L. A. (2020). The reliability of surface EMG derived motor unit variables. J. Electromyogr. Kinesiol. 52, 102419. doi:10.1016/j.jelekin.2020.102419
Hu, X., Rymer, W. Z., and Suresh, N. L. (2014). Accuracy assessment of a surface electromyogram decomposition system in human first dorsal interosseus muscle. J. Neural Eng. 11 (2), 026007. doi:10.1088/1741-2560/11/2/026007
Hwang, I. S., Hu, C. L., Huang, W. M., Tsai, Y. Y., and Chen, Y. C. (2020). Potential motor benefits of visual feedback of error reduction for older adults. J. Aging. Phys. Act., 1–9. doi:10.1123/japa.2019-0405
Hwang, I. S., Hu, C. L., Yang, Z. R., Lin, Y. T., and Chen, Y. C. (2019). Improving Precision force control with low-frequency error amplification feedback: Behavioral and neurophysiological mechanisms. Front. Physiol. 10, 131. doi:10.3389/fphys.2019.00131
Hwang, I. S., Lin, Y. T., Huang, W. M., Yang, Z. R., Hu, C. L., and Chen, Y. C. (2017). Alterations in neural control of constant isometric contraction with the size of error feedback. PloS One 12 (1), e0170824. doi:10.1371/journal.pone.0170824
Iyer, M. B., Christakos, C. N., and Ghez, C. (1994). Coherent modulations of human motor unit discharges during quasi-sinusoidal isometric muscle contractions. Neurosci. Lett. 170 (1), 94–98. doi:10.1016/0304-3940(94)90247-x
Kal, E., Prosée, R., Winters, M., and van der Kamp, J. (2018). Does implicit motor learning lead to greater automatization of motor skills compared to explicit motor learning? A systematic review. PloS One 13 (9), e0203591. doi:10.1371/journal.pone.0203591
Kao, P. C., Srivastava, S., Agrawal, S. K., and Scholz, J. P. (2013). Effect of robotic performance-based error-augmentation versus error-reduction training on the gait of healthy individuals. Gait Posture 37 (1), 113–120. doi:10.1016/j.gaitpost.2012.06.025
Kelley, C. R. (1969). What is adaptive training? Hum. Factors 11 (6), 547–556. doi:10.1177/001872086901100602
Kim, T., Hamade, K. C., Todorov, D., Barnett, W. H., Capps, R. A., Latash, E. M., et al. (2017). Reward based motor adaptation mediated by basal ganglia. Front. Comput. Neurosci. 11, 19. doi:10.3389/fncom.2017.00019
Knight, C. A., and Kamen, G. (2007). Modulation of motor unit firing rates during a complex sinusoidal force task in young and older adults. J. Appl. Physiol. 102 (1), 122–129. doi:10.1152/japplphysiol.00455.2006
Krakauer, J. W., and Mazzoni, P. (2011). Human sensorimotor learning: Adaptation, skill, and beyond. Curr. Opin. Neurobiol. 21 (4), 636–644. doi:10.1016/j.conb.2011.06.012
Laine, C. M., Yavuz, S. U., and Farina, D. (2014). Task-related changes in sensorimotor integration influence the common synaptic input to motor neurones. Acta Physiol. (Oxf). 211 (1), 229–239. doi:10.1111/apha.12255
Lay, B. S., Sparrow, W. A., Hughes, K. M., and O'Dwyer, N. J. (2002). Practice effects on coordination and control, metabolic energy expenditure, and muscle activation. Hum. Mov. Sci. 21 (5-6), 807–830. doi:10.1016/s0167-9457(02)00166-5
Lee, T. D., Eliasz, K. L., Gonzalez, D., Alguire, K., Ding, K., and Dhaliwal, C. (2016). On the role of error in motor learning. J. Mot. Behav. 48 (2), 99–115. doi:10.1080/00222895.2015.1046545
Marchal-Crespo, L., Rappo, N., and Riener, R. (2017). The effectiveness of robotic training depends on motor task characteristics. Exp. Brain Res. 235 (12), 3799–3816. doi:10.1007/s00221-017-5099-9
Martinez-Valdes, E., Laine, C. M., Falla, D., Mayer, F., and Farina, D. (2016). High-density surface electromyography provides reliable estimates of motor unit behavior. Clin. Neurophysiol. 127 (6), 2534–2541. doi:10.1016/j.clinph.2015.10.065
McGill, K. C., Lateva, Z. C., and Marateb, H. R. (2005). Emglab: An interactive EMG decomposition program. J. Neurosci. Methods 149 (2), 121–133. doi:10.1016/j.jneumeth.2005.05.015
Miall, R. C., Weir, D. J., and Stein, J. F. (1993). Intermittency in human manual tracking tasks. J. Mot. Behav. 25 (1), 53–63. doi:10.1080/00222895.1993.9941639
Milanese, C., Cavedon, V., Corte, S., and Agostini, T. (2017). The effects of two different correction strategies on the snatch technique in weightlifting. J. Sports Sci. 35 (5), 476–483. doi:10.1080/02640414.2016.1172727
Milanese, C., Corte, S., Salvetti, L., Cavedon, V., and Agostini, T. (2016). Correction of a technical error in the golf swing: Error amplification versus direct instruction. J. Mot. Behav. 48 (4), 365–376. doi:10.1080/00222895.2015.1102699
Moore, S. P., and Marteniuk, R. G. (1986). Kinematic and electromyographic changes that occur as a function of learning a time-constrained aiming task. J. Mot. Behav. 18 (4), 397–426. doi:10.1080/00222895.1986.10735388
Mutha, P. K., Sainburg, R. L., and Haaland, K. Y. (2011). Critical neural substrates for correcting unexpected trajectory errors and learning from them. Brain 134 (12), 3647–3661. doi:10.1093/brain/awr275
Nawab, S. H., Chang, S. S., and De Luca, C. J. (2010). High-yield decomposition of surface EMG signals. Clin. Neurophysiol. 121 (10), 1602–1615. doi:10.1016/j.clinph.2009.11.092
Negro, F., Holobar, A., and Farina, D. (2009). Fluctuations in isometric muscle force can be described by one linear projection of low-frequency components of motor unit discharge rates. J. Physiol. 587 (24), 5925–5938. doi:10.1113/jphysiol.2009.178509
Ong, N. T., Bowcock, A., and Hodges, N. J. (2010). Manipulations to the timing and type of instructions to examine motor skill performance under pressure. Front. Psychol. 1, 196. doi:10.3389/fpsyg.2010.00196
Piotrkiewicz, M., and Türker, K. S. (2017). Onion skin or common drive? Front. Cell. Neurosci. 11, 2. doi:10.3389/fncel.2017.00002
Saemi, E., Porter, J. M., Ghotbi-Varzaneh, A., Zarghami, M., and Maleki, F. (2012). Knowledge of results after relatively good trials enhances self-efficacy and motor learning. Psychol. Sport Exerc 13 (4), 378–382. doi:10.1016/j.psychsport.2011.12.008
Seidler, R. D., Bo, J., and Anguera, J. A. (2012). Neurocognitive contributions to motor skill learning: The role of working memory. J. Mot. Behav. 44 (6), 445–453. doi:10.1080/00222895.2012.672348
Suzuki, Y., Nomura, T., Casadio, M., and Morasso, P. (2012). Intermittent control with ankle, hip, and mixed strategies during quiet standing: A theoretical proposal based on a double inverted pendulum model. J. Theor. Biol. 310, 55–79. doi:10.1016/j.jtbi.2012.06.019
Taylor, J. A., Krakauer, J. W., and Ivry, R. B. (2014). Explicit and implicit contributions to learning in a sensorimotor adaptation task. J. Neurosci. 34 (8), 3023–3032. doi:10.1523/JNEUROSCI.3619-13.2014
Vaillancourt, D. E., Larsson, L., and Newell, K. M. (2003). Effects of aging on force variability, single motor unit discharge patterns, and the structure of 10, 20, and 40 Hz EMG activity. Neurobiol. Aging 24 (1), 25–35. doi:10.1016/s0197-4580(02)00014-3
White, L., Ford, M. P., Brown, C. J., Peel, C., and Triebel, K. L. (2014). Facilitating the use of implicit memory and learning in the physical therapy management of individuals with alzheimer disease: A case series. J. Geriatr. Phys. Ther. 37 (1), 35–44. doi:10.1519/JPT.0b013e3182862d2c
Wolpert, D. M., Miall, R. C., Winter, J. L., and Stein, J. F. (1992). Evidence for an error deadzone in compensatory tracking. J. Mot. Behav. 24 (4), 299–308. doi:10.1080/00222895.1992.9941626
Keywords: EMG, motor control, motor units, visuomotor integration, error
Citation: Lin Y-T, Chen Y-C, Chang G-C and Hwang I-S (2023) Failure to improve task performance after visuomotor training with error reduction feedback for young adults. Front. Physiol. 14:1066325. doi: 10.3389/fphys.2023.1066325
Received: 14 October 2022; Accepted: 22 February 2023;
Published: 08 March 2023.
Edited by:
Alessandro Del Vecchio, University of Erlangen Nuremberg, GermanyReviewed by:
Christopher Latella, Edith Cowan University, AustraliaCopyright © 2023 Lin, Chen, Chang and Hwang. This is an open-access article distributed under the terms of the Creative Commons Attribution License (CC BY). The use, distribution or reproduction in other forums is permitted, provided the original author(s) and the copyright owner(s) are credited and that the original publication in this journal is cited, in accordance with accepted academic practice. No use, distribution or reproduction is permitted which does not comply with these terms.
*Correspondence: Ing-Shiou Hwang, aXNod2FuZ0BtYWlsLm5ja3UuZWR1LnR3
Disclaimer: All claims expressed in this article are solely those of the authors and do not necessarily represent those of their affiliated organizations, or those of the publisher, the editors and the reviewers. Any product that may be evaluated in this article or claim that may be made by its manufacturer is not guaranteed or endorsed by the publisher.
Research integrity at Frontiers
Learn more about the work of our research integrity team to safeguard the quality of each article we publish.