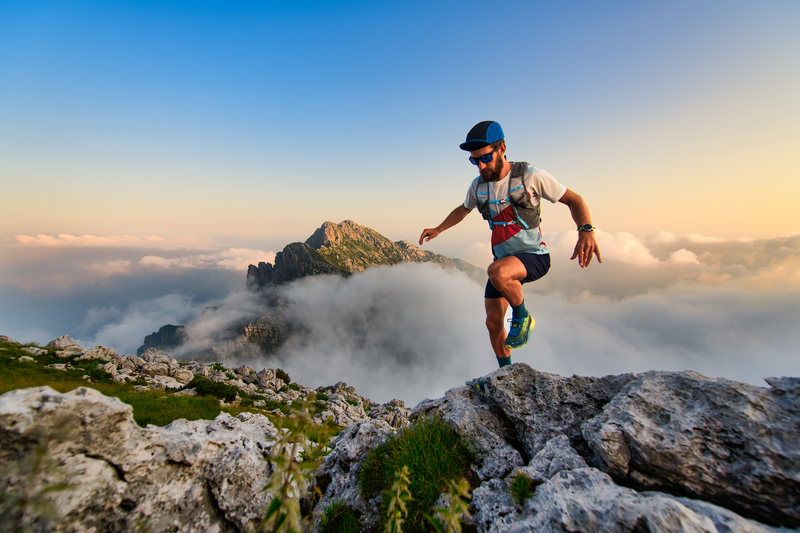
95% of researchers rate our articles as excellent or good
Learn more about the work of our research integrity team to safeguard the quality of each article we publish.
Find out more
ORIGINAL RESEARCH article
Front. Physiol. , 29 September 2022
Sec. Fractal Physiology
Volume 13 - 2022 | https://doi.org/10.3389/fphys.2022.965768
This article is part of the Research Topic Complexity Analysis and Complexity Loss in Physiological Systems: Advances and Applications View all 4 articles
Background: Appropriate monitoring and early recognition of postoperative cognitive improvement (POCI) are essential. Near-infrared spectroscopy (NIRS) showed the predictive potential of POCI. Non-linear dynamical analysis is a powerful approach for understanding intraoperative regional cerebral oxygen saturation (rSO2).
Objective: We hypothesized that the sample entropy (SampEn) value of intraoperative rSO2 has the potential to predict POCI.
Methods: This retrospective cohort study was conducted from June 2019 and December 2020 in a tertiary hospital in Beijing, China. A total of 126 consecutive patients who underwent carotid endarterectomy (CEA) were screened. 57 patients were included in this analysis. The primary outcome was the diagnostic accuracy of rSO2 for the prediction of POCI.
Results: 33 patients (57.9%) developed POCI on postoperative day. The SampEn values of rSO2 were significantly higher in the POCI group (p < 0.05). SampEn remained an independent predictor of POCI in multivariate analysis. The area under the ROC curve (AUC) value of SampEn of rSO2 for POCI were 0.706 (95% CI, 0.569–0.843; p = 0.008). Addition of preoperative MoCA assessment and blood pressure-lowering treatment increased the AUC to 0.808 (95% CI, 0.697–0.919; p < 0.001).
Conclusions: The SampEn value of rSO2 showed promise as a predictor of POCI. Non-linear analysis could be used as a supplementary method for intraoperative physiological signals.
Carotid endarterectomy (CEA) has proven effective for the treatment of high-grade cervical carotid stenosis (Writers, 1998; Halliday et al., 2010). CEA has been associated with cognitive improvement after surgery, termed postoperative cognitive improvement (POCI) (Shi et al., 2016; Robison et al., 2019; Relander et al., 2021; Piegza et al., 2021). It has been established that POCI after CEA is related to improvement in executive functions, which may benefit from improvement in cerebral hemodynamics and associated neuronal metabolism (Relander et al., 2021). Improvements in these functions facilitate daily functioning and working abilities. Appropriate monitoring and early recognition of POCI are essential, which would prompt the scrutiny of underlying factors and promote early intervention in achieving POCI. However, validated tools for predicting POCI remain elusive.
Near-infrared spectroscopy (NIRS) showed the predictive potential of POCI. It allows for continuous and noninvasive monitoring of regional cerebral oxygen saturation (rSO2) (Murkin and Arango, 2009), reflecting the balance between cerebral oxygen consumption and demand. However, NIRS monitoring has not been widely used in clinical practice because of concerns regarding the diagnostic accuracy of intraoperative rSO2 in identifying postoperative cognitive changes (Semrau et al., 2021). The available literature (Kim et al., 2016; Holmgaard et al., 2019) has used measures to define intraoperative hypoxemia, such as a binary event based on a single rSO2 value or by cumulative or consecutive time with rSO2 under a certain threshold. More sophisticated definitions consider hypoxemia time and severity, such as the cumulative time of rSO2 under a threshold, area under a threshold, or time-weighted average under a threshold.
However, these definitions discard much information and therefore poorly characterize NIRS-based rSO2. Non-linear dynamical analysis is a powerful approach for understanding physiological signals (Henriques et al., 2020). Sample entropy (SampEn) (Richman and Moorman, 2000; Yentes et al., 2013; Delgado-Bonal and Marshak, 2019; Porta et al., 2019), a non-linear measure of regularity, has been widely applied in clinical cardiovascular studies. Greater entropy is often associated with more randomness and a lower risk of disease. We hypothesized that SampEn could be used to characterize and quantify the regularity of intraoperative rSO2. The major objective of this study was to estimate the entropy value of rSO2 for predicting the development of POCI in patients undergoing CEA.
We reviewed our database of patients with carotid stenosis who underwent CEA between June 2019 and December 2020. Patients eligible for inclusion were adults with symptomatic or asymptomatic unilateral moderate-to-severe carotid stenosis who were referred for CEA. The exclusion criteria were as follows: 1) cardiogenic stroke; 2) hemorrhagic stroke; 3) previous vascular intervention treatment, such as CEA, stenting, clips, or coils of aneurysms; 4) significant stenoses in the intracranial vasculature on computed tomography angiography (stenosis ≥50%); 5) heart failure; and 6) cerebral neoplasms. 7) Intraoperative rSO2 and cognitive assessment data were missing. A total of 57 patients were identified according to the above criteria and were included in the analysis.
Baseline characteristics were collected by searching a patient database, including age, sex, height, weight, educational level, affected side, clinical symptoms, history of hypertension, hyperlipidemia, diabetes, coronary heart disease, stroke, transient ischemic attack (TIA), smoking, and drinking. Data on preoperative medication use, including antiplatelet, anticoagulant, lipid-altering, glucose-lowering, and blood pressure-lowering agent use, were also collected.
Ethical approval for this study (No 2019-413-02) was provided by the Medical Science Research Ethics Committee of Peking University Third Hospital, Beijing, China (Chairperson Prof Chunli Song) on 12 May 2020. The requirement for written informed consent was waived by the Ethics Committee.
CEA was performed by the same neurosurgeon under general anesthesia using a standard approach according to hospital practice. All patients received the same anesthesia protocol. The surgeon performed deep neck dissection and vessel manipulation under a microscope. Mean arterial pressure increased by 10% before clamping. Shunting was performed if necessary. The patient was administered intravenous heparin postoperatively.
On the day of the surgery, rSO2 (FORE-SIGHT ELITE™; CAS Medical Systems, Branford, CT, United States) was placed on the patient’s forehead and remained in place throughout the surgery. Surgeons and nursing staff were blinded to the rSO2 values. All the rSO2 values were measured every 2 s. The rSO2 values collected from the affected side were used for the data analysis. Mean rSO2 was calculated for the intraoperative period. Minimum and maximum rSO2 values were defined as the lowest and highest recorded value during surgery. The rSO2 baseline was defined as the mean value during a 2 min period before the induction of anesthesia, while the patients were breathing room air. In addition, the relative decreases in rSO2 were analyzed at multiple thresholds (<baseline, <90% of baseline, and <80% of baseline). The duration below the threshold value of rSO2 and the area under the threshold value of rSO2 were calculated. The duration of all CEA surgeries differed between 61 and 157 min, with a mean surgical time of 98 min. All the rSO2 values were measured every 2 s. And thus, the size of the rSO2 time series used was between 1830 and 4710, with a mean size of 2940.
Sample entropy (SampEn) is a technique used to measure the amount of regularity and unpredictability of fluctuations over time-series data (Richman and Moorman, 2000; Yentes et al., 2013; Delgado-Bonal and Marshak, 2019; Porta et al., 2019), and to discriminate series for which clear feature recognition is difficult. It is applicable to relatively short and noisy datasets, with at least 100 data points (Richman and Moorman, 2000; Delgado-Bonal and Marshak 2019). SampEn assigns a non-negative number to a time series with larger values corresponding to greater randomness or less predictability in the data.
The SampEn algorithm is as follows (Richman and Moorman, 2000; Yentes et al., 2013). Given a sequence of numbers u = {u(1),u(2),…,u(N)} of length N, a non-negative integer m, with m ≤ N and a positive real number r, we define the blocks x(i) = {u(i),u(i+1),…,u(i+m−1)} and x(j) = {u(j),u(j+1),…,u(j+m−1)}, and calculate the distance between them as d[×(i),×(j)] = maxk = 1,2,. . .,m(|u(i+k−1)−u(j+k−1)|). We define the total number of possible vectors by calculating for each template vector:
and adding all the template vectors:
In the same way, we define the total number of matches by calculating for each model vector:
and adding them as:
Therefore, Bm(r) is the probability that two sequences are similar for m points, while Am(r) is the probability that two sequences are similar for m+1 points. Since the number of matches is always less than or equal to the number of possible vectors, the ratio Am(r)/Bm(r) is a conditional probability less than unity. The parameter Sample Entropy is defined as SampEn(m,r) = limN→∞{−log[Am(r)/Bm(r)]}. Given N data points, we estimate this parameter by defining the statistic SampEn(m,r,N) = −log[Am(r)/Bm(r))].
The parameters, m and r were used for calculating SampEn: m is the length of compared runs, which is suggested to have the value of m = 2, and r is effectively a filter to define the similar pattern, which equals to 0.1 and 0.25 times the SD of the original time series (Richman and Moorman, 2000). In the presence of nonlinear dynamics (Porta et al., 2019), sample entropy was calculated to characterize intraoperative rSO2. The surrogateTest function in R package nonlinearTseries (version 0.2.12) (Constantino A. Garcia, 2022) was used to test the presence of nonlinear dynamics of each individual rSO2 time-series data.
Sample entropy was calculated using EntropyHub (www.EntropyHub.xyz), an open-source toolkit for entropic time series analysis (Flood and Grimm, 2021). In the present study, we computed SampEn with m = 2 and r equal to 0.1, 0.15, 0.2 and 0.25 times the SD of the original time series, obtaining SampEn (2, 0.1, N), SampEn (2, 0.15, N), SampEn (2, 0.2, N) and SampEn (2, 0.25, N), corresponding to SampEn 01, SampEn 015, SampEn 02 and SampEn 025, respectively.
Cognitive performance was tested pre- and postoperatively using the Peking Union Medical College Hospital version of the Montreal Cognitive Assessment (MoCA) (Tan et al., 2021). A physician obtained the MoCA scores of the patients in a 48-h period before surgery. In addition, cognitive performance was assessed with the MoCA on three or four immediate postoperative days by the same physician. The physician was blinded to the patients’ clinical information and intraoperative rSO2 values. The total MoCA score was adjusted based on years of education. Add one point for an individual who has 12 years or less of formal education. Cognitive improvement was defined as at least a three-point increase in the MoCA score on the third or fourth day after surgery compared with preoperative data.
A sample of 33 from the positive group and 24 from the negative group achieved 83% power to detect a difference of 0.2150 between the area under the receiver operating characteristic curve (AUC) under the null hypothesis of 0.5000 and an AUC under the alternative hypothesis of 0.7150 using a two-sided z-test at a significance level of 0.050.
Statistical analyses were performed using SPSS version 24 (IBM Corp., Armonk, NY, United States). Categorical variables are presented as n (%). Continuous data are expressed as mean (SD) when normally distributed or as median with first and third quartiles when not normally distributed. Categorical data were compared between patients with and without POCI using a χ2 test or Fisher’s exact test, and continuous data were compared using an unpaired Student’s t-test or Mann–Whitney U-test. Baseline characteristics and intraoperative rSO2 parameters that were significant in the univariate analysis at a threshold of p < 0.1 were entered into a multivariable logistic regression model (variable selection method: Backward Likelihood Ratio). The predictive ability of SampEn for rSO2 was assessed using a receiver operating characteristic curve (ROC), and the AUC was calculated to assess the performance in predicting POCI. The predictive ability of SampEn, in combination with potentially influencing factors, was assessed using receiver operating characteristic (ROC) analysis and AUC values. Statistical significance was set at p < 0.05.
A total of 126 patients who underwent CEA at our hospital between June 2019 and December 2020 were screened. Ten patients were excluded for the following reasons: a previous history of radiotherapy to the neck area (n = 2), previous treatment for carotid stenosis (n = 3), and severe stenosis or occlusion of the ipsilateral intracranial artery (n = 5). Among the 116 eligible patients, 59 were excluded owing to missing data (missing clinical data, n = 5; missing postoperative cognitive assessment data, n = 11; missing NIRS monitoring data, n = 43). The remaining 57 patients were included in this analysis. Thirty-three (57.9%) patients developed POCI.
Table 1 shows the baseline characteristics of patients with and without POCI. The patients’ demographic characteristics were similar between the two groups. Clinical data were also similar between the groups, including the affected side, symptoms, history of hyperlipidemia, diabetes, stroke, and TIA. The proportions of self-reported smokers and drinkers did not differ between groups. The use of antiplatelet, anticoagulant, lipid-altering, and glucose-lowering drugs did not differ between groups. Patients with POCI showed worse preoperative cognitive performance on the MoCA. Moreover, the proportion of patients with hypertension, coronary heart disease, and recent use of blood pressure-lowering therapy was significantly lower in the POCI group.
A comparison of intraoperative rSO2 between patients with and without POCI is shown in Table 2. The baseline rSO2 values were similar in both groups (73 vs 74%, p = 0.630). The mean rSO2 values, the lowest and highest rSO2 values throughout surgery, were also not significantly different between the groups. Furthermore, the duration and the area under the curve below 100, 90%, and 80% of baseline did not differ between patients with and without POCI.
Figures 1A,B show typical tracings of rSO2 measured by NIRS in the POCI and non-POCI group. Among the 57 individuals, the rSO2 signal of 53 subjects, including 23 (95.8%) from the non-POCI group, and 30 (90.9%) from the POCI group, showed nonlinear dynamics. The surrogate data testing rejected null hypothesis that rSO2 signal data of 53 subjects comes from a linear stochastic process (supplementary material). Patients with POCI had higher complexity in their rSO2 time series than the non-POCI group. The complexity of intraoperative rSO2 time series is increased, prior to the onset of POCI. As shown in Table 2, the SampEn 01, SampEn 015, and SampEn 02 values were significantly higher in the POCI group (p = 0.008, 0.014 and 0.024, respectively). The SampEn 025 values were higher in the POCI group, although the difference was not statistically significant. SampEn 01, SampEn 015, and SampEn 02 showed greater promise than SampEn 025. SampEn 01 was further analyzed, due to the greater difference between groups.
FIGURE 1. Typical tracings of rSO2 measured by NIRS in the POCI group. Sample entropy was 0.800. rSO2, regional cerebral oxygen saturation; NIRS, Near-infrared spectroscopy; POCI, postoperative cognitive improvement. Typical tracings of rSO2 measured by NIRS in the non-POCI group. Sample entropy was 0.123, respectively. rSO2, regional cerebral oxygen saturation; NIRS, Near-infrared spectroscopy; POCI, postoperative cognitive improvement.
As shown in Table 3, SampEn 01 remained an independent predictor of POCI in multivariate analysis. Figure 2 graphs the ROC curves for the SampEn 01value of rSO2 signal. The AUC value of SampEn 01 for POCI was 0.706 (95% CI, 0.569–0.843; p = 0.008), respectively. Addition of preoperative MoCA assessment and blood pressure-lowering treatment increased the AUC to 0.808 (95% CI, 0.697–0.919; p < 0.001).
FIGURE 2. Receiver operating characteristic curves of the SampEn value of rSO2 signal to predict POCI. SampEn, sample entropy; rSO2, regional cerebral oxygen saturation; POCI, postoperative cognitive improvement.
In this retrospective observational study on the diagnostic accuracy of NIRS-based rSO2 in predicting POCI in patients undergoing CEA, the SampEn and SampEn values of rSO2 showed the predictive potential of POCI. The addition of preoperative MoCA assessment and blood pressure-lowering treatment could improve the diagnostic performance.
Cognitive improvement after surgery is an important issue in perioperative medicine. Previous studies have reported cognitive benefits after CEA (Shi et al., 2016; Robison et al., 2019; Relander et al., 2021; Piegza et al., 2021). In the present study, we found that patients with preoperative better cognitive function derived greater benefit from CEA than those who had lower preoperative MoCA scores. Further study is needed to examine the mechanisms. POCI is associated with better hemodynamic parameters (Robison et al., 2019; Relander et al., 2021), suggesting that intraoperative cerebral perfusion monitoring could be a valuable predictive indicator. Several studies have reported that rSO2 can be used to assess cerebral perfusion and the resulting oxygenation (Kim et al., 2016; Holmgaard et al., 2019; Murkin and Arango, 2019). The available literature used baseline rSO2 and absolute and relative declines in rSO2 to establish the association between rSO2 and postoperative cognitive changes. However, these measures discarded much information and, therefore, poorly characterized the NIRS-based rSO2. Current evidence indicates that if there is a link between intraoperative rSO2 values and cognitive changes, better measures are needed to characterize rSO2 (Ortega-Loubon et al., 2019).
To the best of our knowledge, this is the first study to apply non-linear analysis to intraoperative rSO2. The results of the present study showed that the development of POCI was associated with a significant increase in the SampEn value of rSO2 signals. SampEn remained an independent predictor of POCI in multivariate analysis adjusting for preoperative cognitive function. The findings indicated that SampEn may be used to characterize intraoperative rSO2 and predict POCI in patients undergoing CEA. Researchers have discovered a mechanism to explain the association between the sample entropy of physiological signals and clinical outcomes. Existing studies (Pincus, 1994; Costello et al., 2020) indicate that in healthier individuals, more information processing (i.e., engagement of the regulatory components) in response to environmental challenges, such as hypoxia, is expected. As entropy is a measure of information content in complex physiological time series, evidence indicates that the entropy of physiological signals is higher in healthier individuals. Thus, patients with significantly higher SampEn values of the rSO2 signal, suggesting better cerebral perfusion and oxygenation, were more likely to be associated with better postoperative neurological outcomes.
This study has the following limitations. First, this study had a small sample size and was conducted in patients who underwent CEA at a single center in Beijing, China. Directions for future research include an association between the entropy value of rSO2 and postoperative cognitive changes in various clinical settings and populations. Intraoperative information was not available, including blood pressure monitoring, anesthesia, and operative procedures, which limited the exploration of the influencing factors associated with the entropy value of rSO2. Therefore, we did not provide potential intervention measures to achieve cognitive benefits after surgery. In addition, this study suggested that the entropy value of rSO2 was associated with POCI in the acute stage 4 days post-surgery. However, POCI in the stable phase, 3 months after CEA, was not assessed.
In summary, we found that the SampEn value of the rSO2 signal showed predictive potential for POCI. This is the first study to use SampEn to verify the diagnostic value of rSO2 for postoperative cognitive changes. Non-linear analysis of physical signals could be a supplementary method to perioperative indices, including NIRS-based rSO2. However, further work is required to test the potential value of our methodology by using a larger dataset.
The raw data supporting the conclusions of this article will be made available by the authors, without undue reservation.
The studies involving human participants were reviewed and approved by Peking University Third Hospital Medical Science Research Ethics Committee (No 2019-413-02, Date 2020-05-12). Written informed consent for participation was not required for this study in accordance with the national legislation and the institutional requirements.
TW and NL conceived and designed the study. XW analyzed the data and was responsible for drafting and editing the paper and its final contents. RH, WY, and HY helped with the implementation. All the authors contributed to the refinement of the manuscript and approved the final manuscript.
This work was supported by National Natural Science Foundation of China (82071308), Beijing Natural Science Foundation (7192219), and National Natural Science Foundation of China (81701067, and 82101264).
We are grateful for the support of the staff of the Department of Neurosurgery at Peking University Third Hospital.
The authors declare that the research was conducted in the absence of any commercial or financial relationships that could be construed as a potential conflict of interest.
All claims expressed in this article are solely those of the authors and do not necessarily represent those of their affiliated organizations, or those of the publisher, the editors and the reviewers. Any product that may be evaluated in this article, or claim that may be made by its manufacturer, is not guaranteed or endorsed by the publisher.
The Supplementary Material for this article can be found online at: https://www.frontiersin.org/articles/10.3389/fphys.2022.965768/full#supplementary-material
Costello J. T., Bhogal A. S., Williams T. B., Bekoe R., Sabir A., Tipton M. J., et al. (2020). Effects of normobaric hypoxia on oxygen saturation variability. High. Alt. Med. Biol. 21 (1), 76–83. doi:10.1089/ham.2019.0092
Delgado-Bonal A., Marshak A. (2019). Approximate entropy and sample entropy: A comprehensive tutorial. Entropy (Basel) 21 (6), 541. doi:10.3390/e21060541
ESCT Writers (1998). Randomised trial of endarterectomy for recently symptomatic carotid stenosis: Final results of the MRC European carotid surgery trial (ECST). Lancet 351 (9113), 1379–1387. doi:10.1016/S0140-6736(97)09292-1
Flood M. W., Grimm B. (2021). EntropyHub: An open-source toolkit for entropic time series analysis. PLoS One 16 (11), e0259448. doi:10.1371/journal.pone.0259448
Garcia Constantino A. (2022). nonlinearTseries: Nonlinear time series analysis. R package version 0.2.12. Available at: https://CRAN.R-project.org/package=nonlinearTseries.
Halliday A., Harrison M., Hayter E., Kong X., Mansfield A., Marro J., et al. (2010). 10-year stroke prevention after successful carotid endarterectomy for asymptomatic stenosis (ACST-1), a multicentre randomised trial. Lancet 376 (9746), 1074–1084. doi:10.1016/S0140-6736(10)61197-X
Henriques T., Ribeiro M., Teixeira A., Castro L., Antunes L., Costa-Santos C. (2020). Nonlinear methods most applied to heart-rate time series: A review. Entropy (Basel) 22 (3), 309. doi:10.3390/e22030309
Holmgaard F., Vedel A. G., Rasmussen L. S., Paulson O. B., Nilsson J. C., Ravn H. B. (2019). The association between postoperative cognitive dysfunction and cerebral oximetry during cardiac surgery: A secondary analysis of a randomised trial. Br. J. Anaesth. 123 (2), 196–205. doi:10.1016/j.bja.2019.03.045
Kim J., Shim J. K., Song J. W., Kim E. K., Kwak Y. L. (2016). Postoperative cognitive dysfunction and the change of regional cerebral oxygen saturation in elderly patients undergoing spinal surgery. Anesth. Analg. 123 (2), 436–444. doi:10.1213/ANE.0000000000001352
Murkin J. M., Arango M. (2009). Near-infrared spectroscopy as an index of brain and tissue oxygenation. Br. J. Anaesth. 103 (1), i3–i13. doi:10.1093/bja/aep299
Ortega-Loubon C., Herrera-Gómez F., Bernuy-Guevara C., Jorge-Monjas P., Ochoa-Sangrador C., Bustamante-Munguira J., et al. (2019). Near-infrared spectroscopy monitoring in cardiac and noncardiac surgery: Pairwise and network meta-analyses. J. Clin. Med. 8 (12), 2208. doi:10.3390/jcm8122208
Piegza M., Wieckiewicz G., Wierzba D., Piegza J. (2021). Cognitive functions in patients after carotid artery revascularization-A narrative review. Brain Sci. 11 (10), 1307. doi:10.3390/brainsci11101307
Pincus S. M. (1994). Greater signal regularity may indicate increased system isolation. Math. Biosci. 122 (2), 161–181. doi:10.1016/0025-5564(94)90056-6
Porta A., Bari V., De Maria B., Cairo B., Vaini E., Malacarne M., et al. (2019). On the relevance of computing a local version of sample entropy in cardiovascular control analysis. IEEE Trans. Biomed. Eng. 66 (3), 623–631. doi:10.1109/TBME.2018.2852713
Relander K., Hietanen M., Nuotio K., Ijäs P., Tikkala I., Saimanen E., et al. (2021). Cognitive dysfunction and mortality after carotid endarterectomy. Front. Neurol. 11, 593719. doi:10.3389/fneur.2020.593719
Richman J. S., Moorman J. R. (2000). Physiological time-series analysis using approximate entropy and sample entropy. Am. J. Physiol. Heart Circ. Physiol. 278 (6), H2039–H2049. doi:10.1152/ajpheart.2000.278.6.H2039
Robison T. R., Heyer E. J., Wang S., Caccappolo E., Mergeche J. L., Shah S. S., et al. (2019). Easily screenable characteristics associated with cognitive improvement and dysfunction after carotid endarterectomy. World Neurosurg. 121, e200–e206. doi:10.1016/j.wneu.2018.09.076
Semrau J. S., Motamed M., Ross-White A., Boyd J. G. (2021). Cerebral oximetry and preventing neurological complication post-cardiac surgery: A systematic review. Eur. J. Cardiothorac. Surg. 59 (6), 1144–1154. doi:10.1093/ejcts/ezaa485
Shi G. M., Jiang T., Zhang H., Li M. H., Wang M., Liu Y. K., et al. (2016). Carotid endarterectomy and carotid artery stenting lead to improved cognitive performance in patients with severe carotid artery stenosis. Curr. Neurovasc. Res. 13 (1), 45–49. doi:10.2174/1567202613666151116143757
Tan J. P., Wang X., Zhang S., Zhao Y., Lan X., Li N., et al. (2021). Accuracy of the short-form montreal cognitive assessment Chinese versions. Front. Aging Neurosci. 13, 687824. doi:10.3389/fnagi.2021.687824
Keywords: cerebral oxygen saturation, postoperative cognitive changes, carotid endarterectomy (CEA), non-linear analyses, sample entropy (SampEn)
Citation: Wang X, Huo R, Yuan W, Yuan H, Wang T and Li N (2022) Utility of sample entropy from intraoperative cerebral NIRS oximetry data in the diagnosis of postoperative cognitive improvement. Front. Physiol. 13:965768. doi: 10.3389/fphys.2022.965768
Received: 10 June 2022; Accepted: 05 September 2022;
Published: 29 September 2022.
Edited by:
Alberto Porta, University of Milan, ItalyReviewed by:
Luiz Eduardo Virgilio Silva, Children’s Hospital of Philadelphia, United StatesCopyright © 2022 Wang, Huo, Yuan, Yuan, Wang and Li. This is an open-access article distributed under the terms of the Creative Commons Attribution License (CC BY). The use, distribution or reproduction in other forums is permitted, provided the original author(s) and the copyright owner(s) are credited and that the original publication in this journal is cited, in accordance with accepted academic practice. No use, distribution or reproduction is permitted which does not comply with these terms.
*Correspondence: Tao Wang, d2FuZ3Rhb0Biam11LmVkdS5jbg==; Nan Li, bGluYW45MTdAMTYzLmNvbQ==
†These authors have contributed equally to this work and are co-corresponding authors
Disclaimer: All claims expressed in this article are solely those of the authors and do not necessarily represent those of their affiliated organizations, or those of the publisher, the editors and the reviewers. Any product that may be evaluated in this article or claim that may be made by its manufacturer is not guaranteed or endorsed by the publisher.
Research integrity at Frontiers
Learn more about the work of our research integrity team to safeguard the quality of each article we publish.