- 1State Key Laboratory for Biology of Plant Diseases and Insect Pests, Institute of Plant Protection, Chinese Academy of Agricultural Sciences, Beijing, China
- 2Department of Entomology, College of Plant Protection, Henan Agricultural University, Zhengzhou, China
- 3Key Laboratory of Biohazard Monitoring, Green Prevention and Control for Artificial Grassland, Ministry of Agriculture and Rural Affairs, Institute of Grassland Research of Chinese Academy of Agricultural Sciences, Hohhot, China
Insect brains play important roles in the regulation of sex-biased behaviors such as mating and oviposition. The neural structure and function of brain differences between males and females have been identified, in which the antenna lobes (AL) showed the most discrepancy, however, the whole repertoire of the genes expressed in the brains and the molecular mechanism of neural signaling and structural development are still unclear. In this study, high-throughput transcriptome analysis of male and female brains was carried on in the Asia corn borer, Ostrinia furnacalis, and a total of 39.23 Gb data and 34,092 unigenes were obtained. Among them, 276 genes displayed sex-biased expression by DEG analysis, of which 125 genes were highly expressed in the males and 151 genes were highly expressed in the females. Besides, by homology analysis against genes that have been confirmed to be related to brain neurodevelopment, a total of 24 candidate genes were identified in O. furnacalis. In addition, to further screen the core genes that may be important for sex-biased nerve signaling and neurodevelopment, protein-protein interaction networks were constructed for the sex-biased genes and neurodevelopment genes. We identified 10 (Mhc, Mlc1, Mlc2, Prm, Mf, wupA, TpnC25D, fln, l(2)efl, and Act5C), 11 (PPO2, GNBP3, Spn77Ba, Ppn, yellow-d2, PGRP-LB, PGRP-SD, PGRP-SC2, Hml, Cg25C, and vkg) and 8 (dac, wg, hh, ci, run, Lim1, Rbp9, and Bx) core hub genes that may be related to brain neural development from male-biased, female-biased, and neurodevelopment gene groups. Our results provide a reference for further analysis of the dimorphism of male and female brain structures in agricultural pests.
Introduction
Insects display different behaviors between males and females, and these differences are probably caused by the dimorphism of brain structure and function (Cachero et al., 2010). For example, in Lepidopteran insects, females release sex pheromones and males could detect the pheromones through a sensitive olfactory system (Berg et al., 1995; Hansson et al., 1995; Wang et al., 2018). In the olfactory central nervous system of the brains, the antennal lobe (AL) of males contains a macroglomerular complex (MGC) structure with the main function of sex pheromone recognition (Anton and Homberg, 1999; Zhao and Berg, 2010; Dong et al., 2020). However, the brain structure is quite different between males and females. The female brains have a larger female glomerulus (LFG) instead of MGC, which may function to perceive information related to female-specific behaviors (Rospars and Hildebrand, 2000; Berg et al., 2002; Skiri et al., 2005). The dimorphism of brain structures and functions may be specifically regulated by some genes expressed in the brains, but only a few of them were identified and functionally characterized in insects, especially in agricultural pests.
Sex-biased genes in the brains might be involved in the different neural signaling and structural development. The sex-determining genes doublesex (dsx) and fruitless (fru) regulate the Drosophila takeout gene and affect male courtship behavior (Dauwalder et al., 2002). In addition, some genes displayed similar expression patterns between males and females and they exhibited complex functions in neural development. For example, dachshund (dac) encoded a new nuclear protein that was necessary for the development of normal eye and mushroom bodies (Mardon et al., 1994; Kurusu et al., 2000). The scratch (scrt) was expressed in neuronal precursor cells and encoded a predicted zinc finger transcription factor and it was confirmed to be involved in neuronal development (Roark et al., 1995). However, most of these studies were sporadic and focused on one or two specific genes, while a whole organism view of genes expressed in the brains is still needed (Kasai et al., 1998; Janssens et al., 2010).
The development of sequencing technology has allowed for the collection of whole repertoires of genes expressed in the brains and is beneficial to our understanding of the exhaustive molecular mechanism of neural signaling and structural development of the brains. Transcriptome analyses have been reported in many insects including fruit flies (Zhan et al., 2007; Hughes et al., 2012), bees (Vleurinck et al., 2016; Li et al., 2019; Steffen and Rehan, 2020), ants (Calkins et al., 2018; Romain et al., 2018; Wang et al., 2020), wasps (Berens et al., 2017), butterflies (Zhu et al., 2008; Lugena et al., 2019), silkworm (Wang et al., 2015), and two species of Noctuidae moths (Walker Ⅲ et al., 2019; Cinel and Taylor, 2019). Candidate genes that regulate job differentiation in social insects such as bees and ants and genes related to age and clock in fruit flies have been identified. In the case of Drosophila melanogaster, circadian transcriptome analysis of the brain demonstrates that extensive circadian rhythm control of noncoding RNAs (ncRNAs) was involved in circadian rhythm control (Hughes et al., 2012).
Transcriptome data generally contains thousands of genes, and these data can be narrowed down by a determination of the protein-protein interaction (PPI) network which is used to screen and pick up core genes. The PPI network intuitively displays the main characteristics of the interaction and functional characteristics of proteins in birth objects and reflects the unique and essential proteins, which have been widely used in gene function annotation and prediction (Li et al., 2013; Zhang and Zhang, 2020; Xin and Zhang, 2021). STRING (https://STRING-db.org/) is a database that searches for known and predicted interactions between proteins, built through PPI networks to better understand complex regulatory networks in organisms (von Mering et al., 2005; Szklarczyk et al., 2019). In insects, PPI networks are used only in D. melanogaster or Bombyx mori for screening key proteins related to insecticide resistance, olfactory systems, and detoxification enzymes (Zhang and Zhang, 2019 and 2020; Xin and Zhang, 2020 and 2021).
In the Ostrinia genus (Lepidoptera: Crambidae), 21 species have been characterized including serious agricultural pests of maize, the Asian corn borer Ostrinia furnacalis, and the European corn borer Ostrinia nubilalis (Mutuura and Munroe, 1970; Huang et al., 1998). Olfactory system genes involved in pheromone perception have been identified and functionally analyzed in this genus, indicating that the peripheral nervous system was different between males and females (Ishikawa et al., 1999; Miura et al., 2010; Yang et al., 2015; Liu et al., 2018). As the main olfactory center of the insect brain, AL contains a large number of glomeruli, similar to the olfactory bulb in the brain of vertebrates (Zhao and Berg, 2010). In addition, studies on the brain structure showed that males of O. nubilalis have similar large glomerular complexes in the AL, but these large complexes were not found in the females (Karpati et al., 2008). The regulatory gene differences may be related to sex-biased genes or neurodevelopmental genes, but the related candidate genes are completely unknown in this genus.
In this study, high-throughput transcriptome analysis was used to identify the whole repertoire of the genes expressed in the brains of males and females in O. furnacalis. Among them, genes with sex-biased expressions were identified by DEG analysis. Neural development genes that were equally expressed between males and females were identified using homology analysis. In addition, PPI network analysis was carried on to further obtain the core hub genes for neural signaling and structural development in the brains. Our results provide a basis for functional studies of the central nervous system of agricultural pests and will help to develop new target genes for pest control in agriculture.
Materials and methods
Insect rearing and tissues collection
O. furnacalis were maintained in the Chinese Academy of Agricultural Sciences, Beijing, China, under laboratory conditions with an artificial diet at 27 ± 1°C, 16:8 (L:D), and 70% relative humidity. Adults were fed with a 10% sugar solution. Brains from 3-day-old males and females were dissected out in fresh Ringer’s solution (in mM; 150 NaCl, 3 CaCl2, 3 KCl, 25 sucrose, and 10 N-Tris [hydroxy-methyl]-methyl-2-amino-ethanesulfonic acid, pH 6.9) on ice, frozen in liquid nitrogen, and stored at −80°C for the following experiments.
RNA extraction and transcriptome sequencing
Total RNA was isolated from 30 brains of males (or females) using TRIzol reagent (Invitrogen, Carlsbad, CA, United States) according to the manufacturer’s instructions. The RNA was dissolved in RNase-free water, and the quality was assessed by gel electrophoresis. The concentration and purity of RNA were determined on a NanoDrop ND-2000 spectrophotometer (NanoDrop products, Wilmington, DE, United States). A total amount of 1 μg RNA per sample was used as input material for RNA sample preparations. Sequencing libraries were generated using NEBNext®Ultra™ RNA Library Prep Kit for Illumina® (NEB, United States) following the manufacturer’s recommendations and sequenced on an Illumina Hiseq 2000 platform. Three repeats of each sample were used for the sequencing.
Transcriptome assembly and gene functional annotation
Raw reads were filtered and their qualities were calculated by Q30. Read number, base number, and GC-content were calculated from the filtered clean reads. Trinity was used for the de novo assembly (Grabherr et al., 2011). The obtained unigenes were annotated using different databases including NR (Deng et al., 2006), Swiss-Prot (Apweiler et al., 2004), GO (Ashburner et al., 2000), COG (Tatusov et al., 2000), KOG (Koonin et al., 2004), eggNOG (Huerta-Cepas et al., 2016), and KEGG (Kanehisa et al., 2004). The HMMER (Eddy, 1998) software was used to compare with the Pfam (Finn et al., 2014) database to obtain the annotation information of unigenes. BLAST parameter e-values were not greater than 1−5, and the HMMER parameter e-value was not greater than 1−10.
Differentially expressed gene analysis
Reads were mapped to the unigenes using Bowtie (Langmead et al., 2009), and the expression level of each unigene was estimated with RSEM (Li and Dewey, 2011). The different expression patterns of unigenes between males and females were calculated with FPKM values by DESeq2 (Anders and Huber, 2010; Trapnell et al., 2010). The generally accepted and effective Benjamini–Hochberg method was used to correct the significant p-values obtained by the original hypothesis test. Then, the differentially expressed genes among the sample groups annotated to the GO database were enriched and analyzed by topGO (Alexa and Rahnenfuhrer, 2010) software. In addition, the differentially expressed genes were classified by COG and eggNOG, as well as KEGG annotation and pathway enrichment analysis.
Identification of developmental genes
Through a literature review, we downloaded the protein sequences of developmental genes from previous studies from NCBI and used them as a query to screen the candidate neural development genes against the unigenes expressed in male and female brains in O. furnacalis. By best hit, only the best and longest sequence results were used. The obtained protein sequences were subsequently verified by BLAST orientation in the NCBI database to remove low confidence genes in order to finally obtained the candidate neural development genes in O. furnacalis.
Construction and analysis of PPI network
PPI networks were constructed using STRING 11.5 (https://cn.STRING-db.org) (von Mering et al., 2005). We chose D. melanogaster as the organism. The PPI network was optimized and analyzed by Cytoscape 3.7.1 (Shannon et al., 2003). The core modules in the PPI network (Node Score cutoff: 0.2, K-score: 2) were screened by the Molecular Complex Detection (MCODE) plug-in (Bader and Hogue, 2003). The CytoHubba algorithm plug-in was used to find the key targets and subnetworks of PPI networks and determine the hub genes. Finally, the degree and intermediary centrality of each node was calculated by the Network Analyzer plug-in (Brandes, 2008).
Results
Sequencing and annotation
By sequencing three cDNA libraries of each sex, more than 131 million reads were obtained, of which 67,102,198 reads and 64,226,235 reads were obtained from female and male brains, respectively (Table 1). The sequencing raw data was uploaded to the NCBI Sequence Read Archive database (Accession number: PRJNA818099). The Q30 values were 94.51%–94.93%, and the overall GC percentages and mapping ratio were 45.01%–46.72% and 82.88%–85.15%, respectively. The assembly results obtained by Trinity are summarized in Table 2. A total of 34,092 unigenes were obtained, with a total length of 42,252,036 bp. The average length and the N50 length of unigenes were 1239.35 bp and 2,352 bp, respectively. According to different annotation methods, 16,174 unigenes were annotated, in which 7,085 unigenes ranged from 300 to 1,000 bp in length, and 9,089 unigenes were over 1,000 bp in length (Table 3).
Analysis of differentially expressed gene
By DEG analysis, 276 genes displayed sex-biased expression, with 125 and 151 highly expressed genes found in the male and female brains, respectively (Figure 1A, Supplementary Table S1). Among them, 6 genes were specifically expressed in females and 15 genes were specifically expressed in males (Table 4), while 18 genes were 50 times different between males and females (Table 5). Through hierarchical clustering analysis, the clustering results of the DEGs among sample groups were obtained (Figure 1B), which again demonstrated the obvious difference between females and males. In addition, 220 of the 276 sex-biased genes were annotated and their functions were analyzed against different databases (Table 3). By GO annotation, 100 genes were clustered into different functional groups including cellular components, molecular function, and biological process, in which cellular components contained more male-biased genes and biological processes contained more female-biased genes (Figure 2A). By COG annotation, 79 genes were clustered into 19 functional groups. Female and male DEGs corresponded to 17 and 16 functional groups, which accounted for the largest proportion in carbohydrate transport and metabolism categories, respectively. Meanwhile, the second-largest category of female DEGs included 6 genes, which specifically corresponded to the cell wall, membrane, or envelope biogenesis (Figure 2B). According to KEGG enrichment analysis, the longevity regulating pathway in males and the AGE-RAGE signaling pathway in diabetic complications in females corresponded to the largest number of genes, and the enrichment results were the most reliable. (Figures 2C,D).
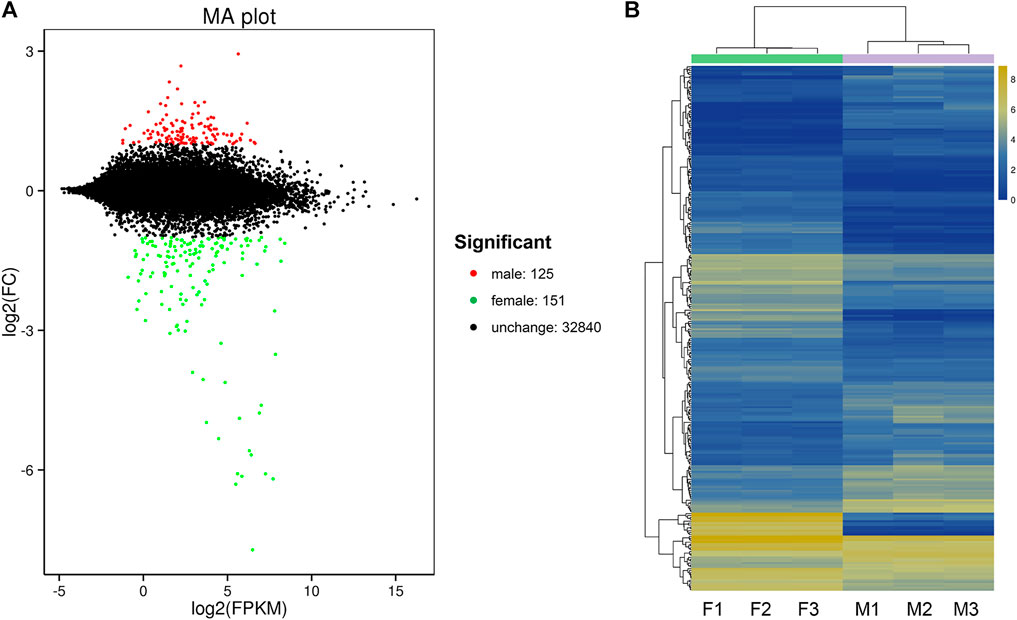
FIGURE 1. Differentially expressed gene (DEG) analysis for the brain transcriptomes in Ostrinia furnacalis. (A) MA plot for differentially expressed genes. Each dot in the MA map represents one gene. The abscissa is A value: log2 (FPKM) is the logarithm value of the mean expression quantity of the two samples; ordinate is M value: log2 (FC), is the logarithm value of the multiple of gene expression difference between the two samples, which was used to measure the difference of gene expression. In the picture, green and red dots represent genes with significant differences in expression, green represents downregulation of gene expression, red represents upregulation of gene expression, and black dots represent genes with no significant difference in expression. (B) Cluster diagram of expression patterns of DEGs. Different columns represent different samples, and different rows represent different genes. The color represents the logarithm of the FPKM base 2 of the gene expression in the sample. F: female; M: male.
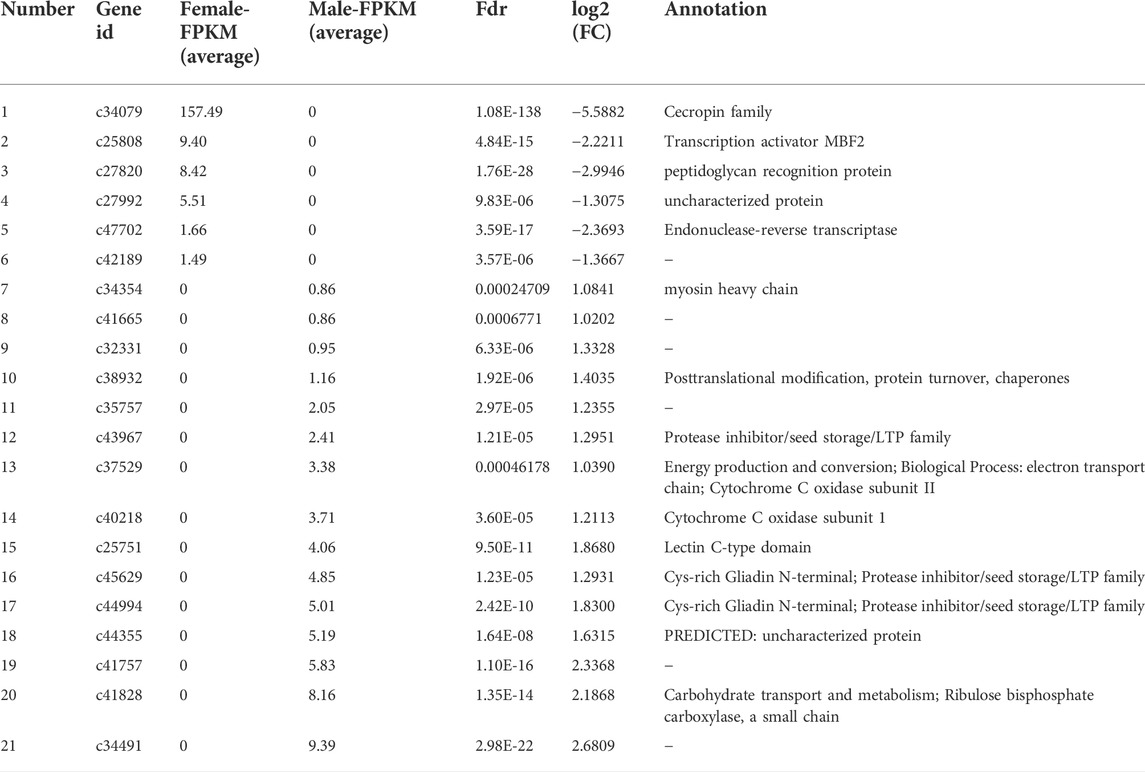
TABLE 4. Summary of genes that displayed sex-specific expressions in the brains of Ostrinia furnacalis.
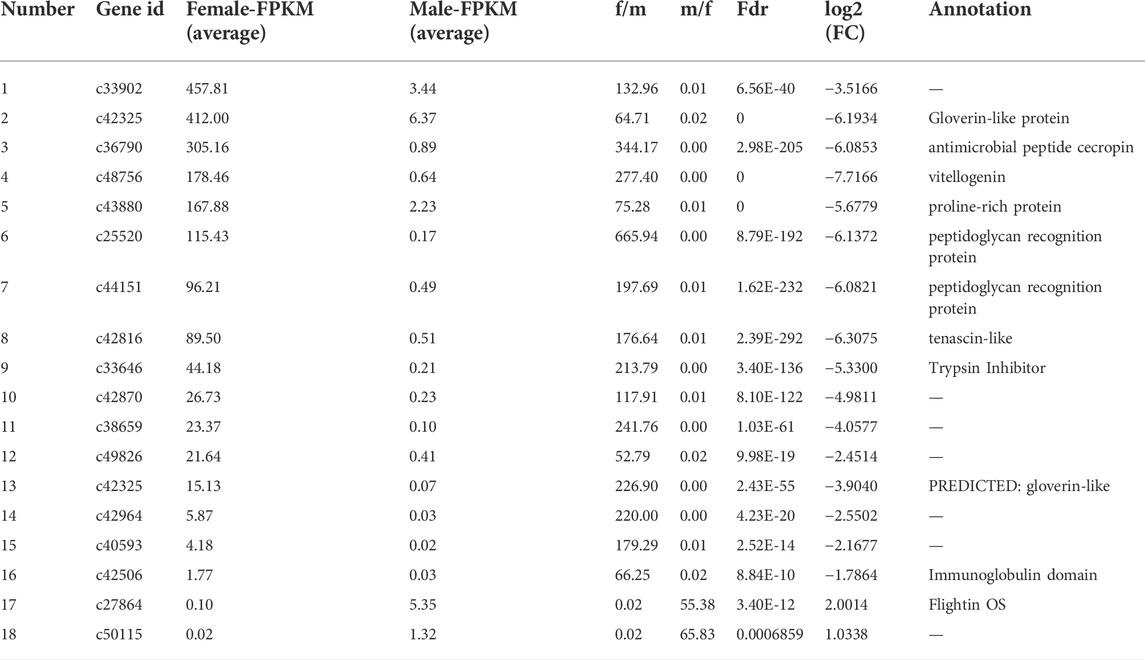
TABLE 5. Summary of genes that displayed sex-biased expressions (more than 50 times) in the brains of Ostrinia furnacalis.
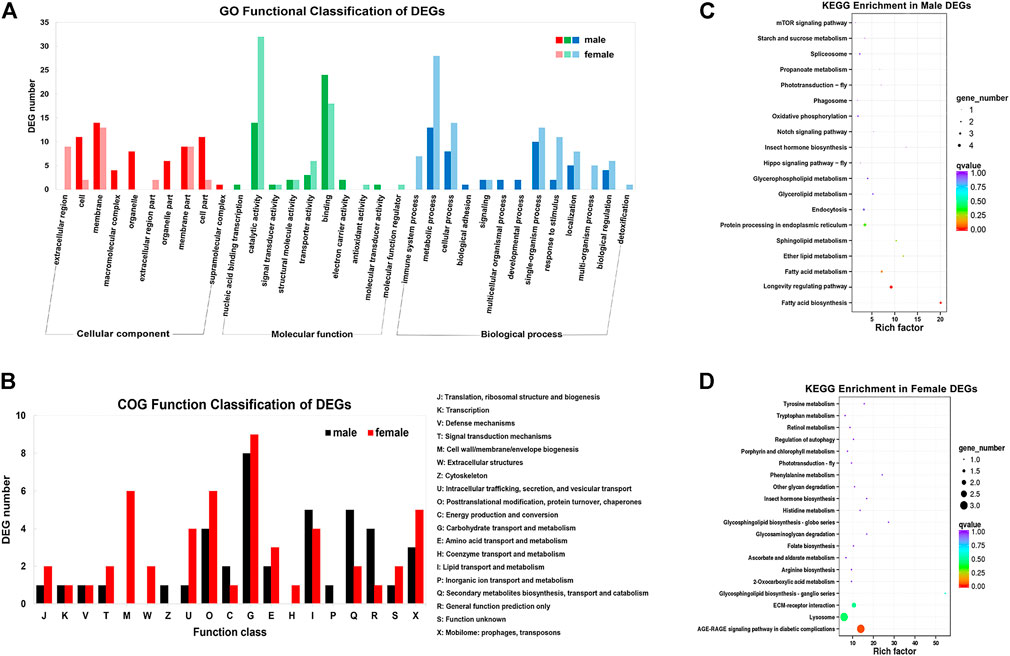
FIGURE 2. Gene annotation for the unigenes from brain transcriptomes in Ostrinia furnacalis. (A) GO function classification of DEGs. The abscissa is GO classification and the ordinate is DEG number. This figure shows the gene enrichment of each secondary function of GO under the background of DEG in male and female brains. Dark colors represent DEG in the female brain and light colors represent DEG in the male brain. (B) COG function classification of DEGs. The horizontal coordinate is the DEG function class, and the vertical coordinate is the DEG Frequency. Detailed COG notes corresponding to the abscissa are on the right. (C–D) KEGG enrichment in male and female DEGs. Each circle represents a KEGG pathway, the ordinate coordinate represents the pathway name, and the abscissa is the enrichment factor, which represents the ratio of the proportion of genes in the differential gene that are annotated to a pathway based on the proportion of genes in all genes that are annotated to that pathway. The larger the enrichment factor, the more significant the level of enrichment of the gene indicating differential expression in that pathway. The color of the circle represents the q value, and the smaller the q value, the more reliable the enrichment significance of the differentially expressed gene in that pathway. The size of the circle indicates the number of genes enriched in the pathway, and the larger the circle, the more genes.
Identification of candidate neurodevelopment genes in O. furnacalis
We obtained the protein sequences of 343 developmental genes expressed in the brains of 163 different species such as Apis mellifera (Li et al., 2019), D. melanogaster (Dahl et al., 1997; Posnien et al., 2011; Sinigaglia et al., 2013), Tribolium castaneum (Choe and Brown, 2007), Bombyx mori (Ferguson et al., 2014), and Bemisia tabaci (He et al., 2020) from NCBI (Supplementary Table S2). By local BLAST analysis of the sequencing data against the query, 40,285 candidates were obtained from the homology analysis of developmental genes. Subsequently, 2,365 candidates were obtained through the best hit method. In addition, candidates that only had a few matches compared to the query genes were deleted, and the remaining 108 candidates were re-verified by BLAST in the NCBI database. Finally, 24 candidate genes were obtained that could be the neural development genes in O. furnacalis (Table 6, Supplementary Table S3).
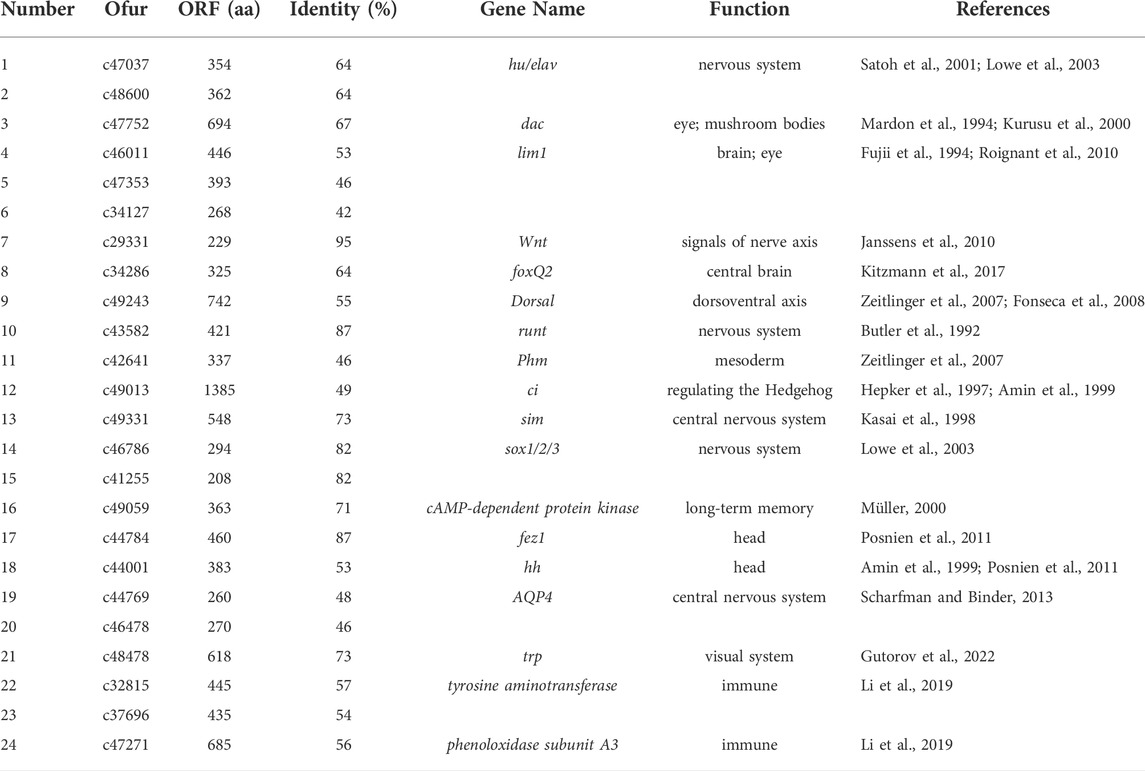
TABLE 6. List of 24 brain neurodevelopment candidate genes identified in Ostrinia furnacalis. The citation of Table 6 is missing and must be cited. Please note that Figures and Tables must be cited sequentially.
PPI network analysis for sex-biased genes
Two PPI networks were constructed from 125 male DEGs and 151 female DEGs using the STRING database. Among them, the male network matched 56 nodes and 52 edges (enrichment p-value: <1.0−16, average node degree: 1.86) (Figure 3A). The female network matched 50 nodes and 25 edges (enrichment p-value: <2.15−10, average node degree: 1.00) (Figure 4A). Through the MCODE algorithm of Cytoscape, the core interaction network modules that played an important role in the stability of the entire protein interaction network were selected. The core network of males contained 9 hub genes (Mhc, Mlc1, Mlc2, Prm, Mf, wupA, TpnC25D, fln, and Act5C) and 64 interactions (score = 8.000) (Figure 3B). The core network of females also contained 9 hub genes (PPO2, GNBP3, Spn77Ba, Ppn, yellow-d2, PGRP-LB, PGRP-SD, Cg25C, and vkg) and 22 interaction relationships (score = 2.750) (Figure 4B). The CytoHubba plug-in was used again to extract the hub genes (male: Mhc, Mlc1, Mlc2, Prm, Mf, wupA, TpnC25D, fln, l(2)efl, and Act5C) (Figure 3C) and (female: PPO2, GNBP3, Spn77Ba, Ppn, yellow-d2, PGRP-LB, Cg25C, PGRP-SC2, and Hml) (Figure 4C). Combining the two algorithms, 125 male DEGs obtained a total of 10 core hub genes, which were mostly described in STRING as related to development and reproduction. 151 female DEGs obtained a total of 11 core hub genes, which were mostly related to immune function (Table 7). When using the NetworkAnalyzer plug-in to calculate the degree and betweenness centrality of each node, the genes with higher degree and betweenness centrality values were basically consistent with the core hub genes we found. In the male network, the degree of Mlc1 was the highest, and the betweenness centrality of Act5C was the highest (Figure 3D, Supplementary Table S4). The degree and the betweenness centrality of PPO2 were the highest in the female network (Figure 4D, Supplementary Table S5).
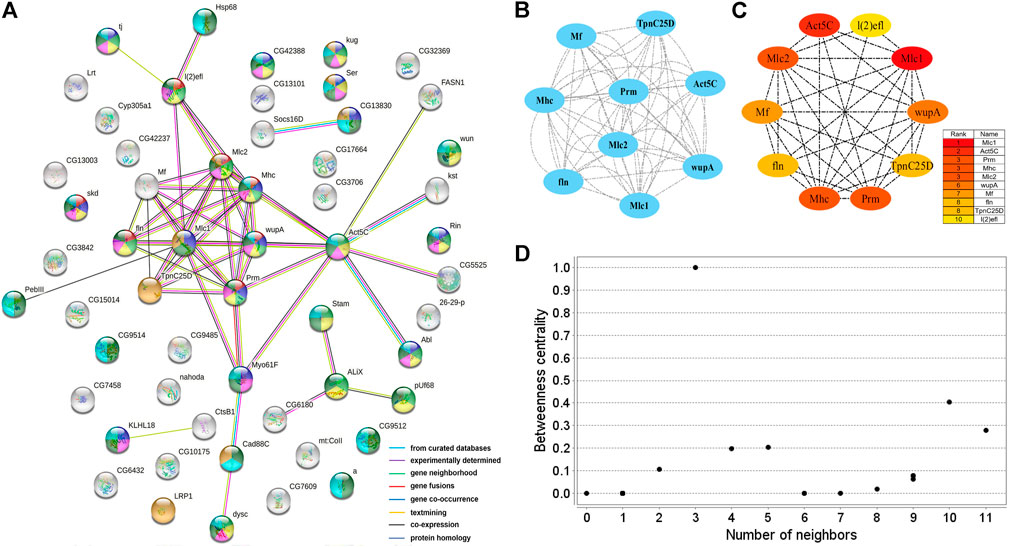
FIGURE 3. PPI network analysis of 125 male-biased genes in the brains of Ostrinia furnacalis. (A) PPI network constructed from 125 DEGs in the male brain. Network nodes represent interacting proteins and contain the three-dimensional protein structures that are known or predicted. Red: muscle structure development. Light green: cell development. Dark blue: tissue development. Yellow: cell differentiation. Pink: anatomical structure morphogenesis. Baby blue: multicellular organism development. Dark green: anatomical structure development. Orange: calcium ion binding. The colored border lines indicate the type of evidence that supports these associations. (B) PPI core network module obtained by the MCODE algorithm. Nodes represent interacting proteins. Edges represent interactions. (C) PPI core proteins were detected by the CytoHubba algorithm. The color is related to the degree of the node, decreasing from red to yellow. The redder the color, the more critical the protein. (D) Betweenness centrality analysis of protein nodes. Black nodes represent highly intermediate proteins. The ordinate represents the value of the betweenness centrality. The horizontal axis represents the number of adjacent proteins.
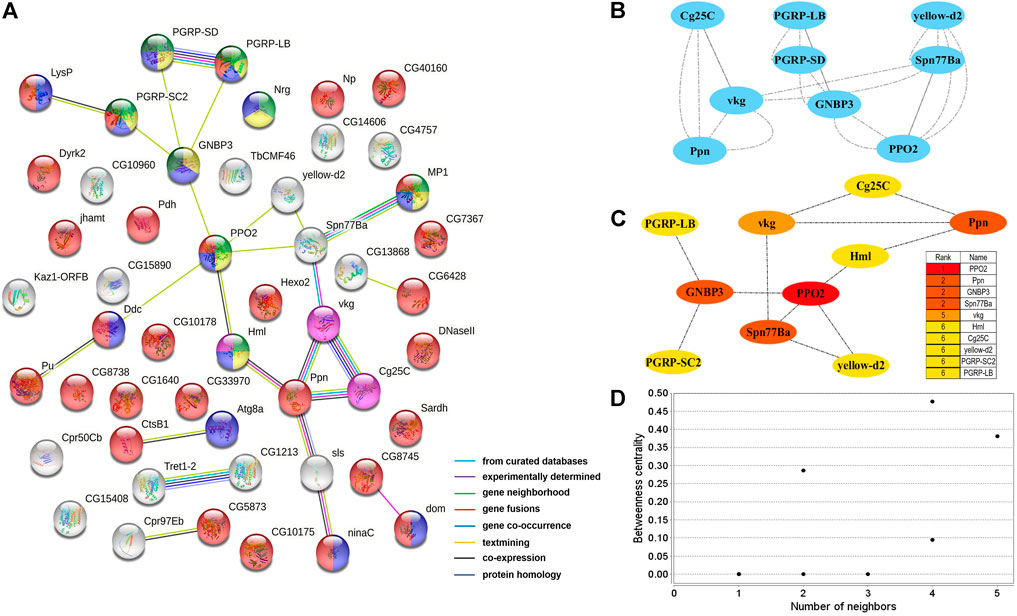
FIGURE 4. PPI network analysis of 151 female-biased genes in the brains of Ostrinia furnacalis. (A) PPI network constructed from 151 DEGs in the female brain. Network nodes represent interacting proteins and contain the three-dimensional protein structures that are known or predicted. Light green: innate immune response. Yellow: immune response. Blue: response to external stimulus. Red: catalytic activity. Pink: ECM-receptor interaction. Dark green: Toll and Imd signaling pathway. The colored border lines indicate the type of evidence that supports these associations. (B) PPI core network module obtained by the MCODE algorithm. Nodes represent interacting proteins. Edges represent interactions. (C) PPI core proteins were detected by the CytoHubba algorithm. The color is related to the degree of the node, decreasing from red to yellow. The redder the color, the more critical the protein. (D) Betweenness centrality analysis of protein nodes. Black nodes represent highly intermediate protiens. The ordinate represents the value of the betweenness centrality. The horizontal axis represents the number of adjacent proteins.
PPI network analysis for neurodevelopment candidate genes
A protein interaction network with 24 nodes and 15 edges (enrichment p-value: <1.27−07, average node degree: 1.36) was obtained by matching 24 candidate genes for brain neural development with the STRING database (Figure 5A). Cytoscape’s MCODE algorithm was used to select one core network module with close relationships and important roles in the stability of the whole protein interaction network, involving 4 hub genes (ci, wg, hh, and dac) and 6 interaction relationships (score = 4.000) (Figure 5B). Furthermore, the CytoHubba plug-in was used to extract 8 hub genes (dac, wg, hh, ci, run, Lim1, Rbp9, and Bx) from the PPI network by degree (Figure 5C), and compared with the results of MCODE, the 4 highest hub genes were consistent. The two algorithms obtained a total of 8 core hub genes, which were mostly related to neurodevelopmental functions in STRING (Table 7). Using the NetworkAnalyzer plug-in, the highest degree and betweenness centrality was obtained for wg, dac, Lim1, Rbp9, and hh, which were all included in the 8 hub genes identified in this work (Figure 5D, Supplementary Table S5).
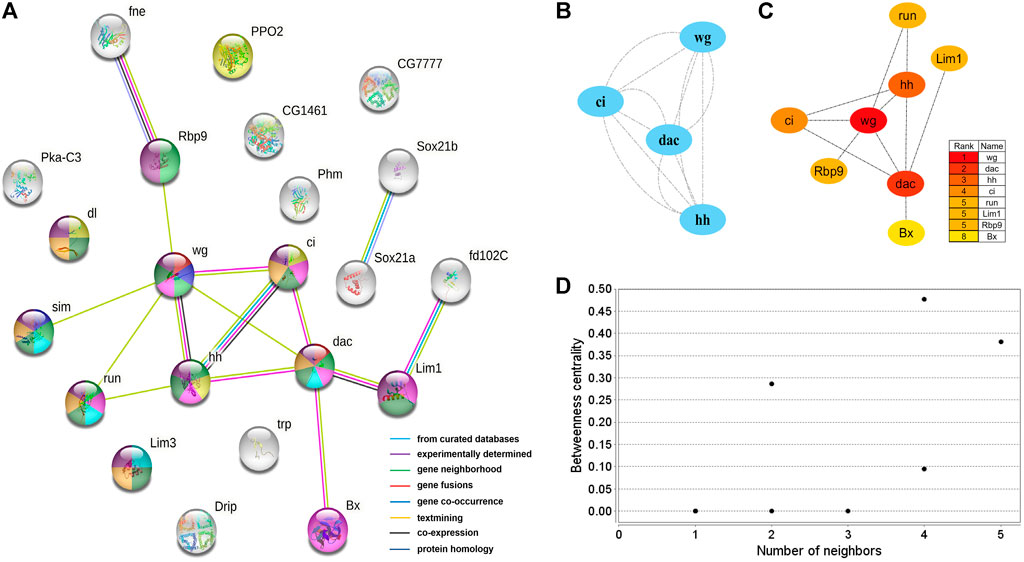
FIGURE 5. PPI network of 24 brain neurodevelopment genes. (A) PPI analysis of 24 candidate genes for brain neurodevelopment. Network nodes represent interacting proteins and contain the three-dimensional protein structures that are known or predicted. Red: genital disc sexually dimorphic development. Dark blue: ectoderm development. Light green: central nervous system development. Yellow: immune response. Pink: sensory organ development. Light blue: axonogenesis. Dark green: neuron differentiation. Orange: neuron development. Prune: nervous system development. The colored border lines indicate the type of evidence that supports these associations. (B) PPI core network module obtained by the MCODE algorithm. Nodes represent interacting proteins. Edges represent interactions. (C) PPI core proteins were detected by the CytoHubba algorithm. The color is related to the degree of the node, decreasing from red to yellow. The redder the color, the more critical the protein. (D) Betweeness centrality analysis of protein nodes. Black nodes represent highly intermediate proteins. The ordinate represents the value of the betweeness centrality. The horizontal axis represents the number of adjacent proteins.
Discussion
In this study, we identified 276 sex-biased genes and 24 neurodevelopmental candidate genes, of which 29 core genes were screened by PPI network analysis. Most of the 24 neurodevelopmental candidate genes homologous to the functionally known genes were equally expressed between males and females. Interestingly, sex-biased genes displayed significant differences in gene functions between sexes. In the males, sex-biased genes were identified to be associated with development, but female-biased genes were identified to be associated with immunity. One hypothesis to explain this phenomenon is that the normal neurodevelopmental candidate genes might be involved in the basic progress of neurodevelopment so that they were equally expressed in the male and female brains, while the newly identified male-biased genes might be specifically involved in the regulation of development of male-specific MGC structure; this needs to be further confirmed by gene knock-out or knock-down studies.
In the male brains, 125 genes displayed male-specific or biased expressions, of which 11 were identified to be the core genes in the PPI network analysis, and most were functionally associated with development and reproduction (Table 7). The c26838 gene corresponded to myosin alkali light chain 1 (Mlc1), which had the highest degree in the PPI network analysis, and was shown to be involved in mesodermal development (Picchio et al., 2018). The c38747 gene corresponds to Actin-5C (Act5C), which was one of the core hub genes highly expressed in the developing cells, with various functions related to development and reproduction, and it plays important roles in cytokinesis and spermatogenesis (Wagner et al., 2002; Noguchi and Miller, 2003; Wilson et al., 2008; Edwards et al., 2009; Guan et al., 2019). The c40474 gene corresponds to wings up A (wupA) and, encodes Troponin I, with involvement in muscle and nervous system development and maintenance (Fishilevich et al., 2019). The c45921 gene corresponds to myosin heavy chain (Mhc) and is known to be involved in muscle cell differentiation, cell component movement, and flight (Picchio et al., 2018). These results indicated that the core genes with male-specific or biased expressions might play important roles in the brain neural development and might be related to the development of MGC structure in the male brains in O. furnacalis.
In the female brains, 151 genes displayed female-specific or biased expressions, of which 10 were identified to be the core genes in the PPI network analysis, and most were functionally associated with immunity (Table 7). Among them, the c47271 gene corresponds to Prophenoloxidase 2 (PPO2), which plays an important role in both melanin formation and immunity (Banerjee et al., 2019; Schmid et al., 2019). The c46696 gene corresponds to serine protease inhibitor 77Ba (Spn77Ba), which was identified to be involved in the regulation of immune responses by inducing systemic expression of the antifungal peptide drosomycin through the Toll pathway and disruption of tracheal melanosis (Tang et al., 2008). GNBP3 encodes a hemolymphatic protein, while PGRP-SD, PGRP-SC2, and PGRP-LB encode peptidoglycan-recognition proteins, which are a family of pattern recognition molecules identified to be involved in the regulation of the Toll and Imd signaling pathway. These pathways are related to immune response and development, and correspond corresponding to the c25523, c25520, c42129, and c45285 genes (Tanji et al., 2007; Royet et al., 2011; Iatsenko et al., 2016; Zhu et al., 2017; Yang et al., 2018; Orlans et al., 2021). Immune genes may be partly related to microglia, which are the resident immune cells in the brain (Hammond et al., 2019). Interestingly, the female-biased genes were mostly related to immunity, but the exact reason for this accumulation of immunity-related genes remains unknown and requires further study. In addition, several peptides (c25808, c34079, c36790, c42325) and peptidase (c33646) were identified as highly expressed in the female brains, which might be involved in female-specific signaling or the development of the LFG structure in O. furnacalis.
Additionally, 24 candidate genes for brain neurodevelopment were identified by homology analysis, and eight of them were identified to be core genes by PPI network analysis. These genes might be associated with neuronal differentiation, nervous system development, cell differentiation, and anatomical morphogenesis in the brain. Among them, only one gene (Phenoloxidase subunit A3) displayed female-biased expression and the others were equally expressed between male and female brains. Phenoloxidase is a polycopper oxidase that plays an important role in melanin synthesis, cuticle pigmentation, wound healing, and defense against microbial and parasitic invasion (Elsik et al., 2014). The key core gene dachshund (dac, c47752) was identified being be involved in the development of eyes and mushroom bodies (Mardon et al., 1994; Kurusu et al., 2000). Hedgehogs (hh, c44001) play an important role in the development of head segment polarity (Amin et al., 1999; Ntini and Wimmer, 2011; Posnien et al., 2011), as well as regulation of eye size and pattern (Míguez et al., 2020). In addition, hh and cubitus interruptus (ci, c49013) were identified that being involved in regulating the Hedgehog signaling pathway, which is critical in embryonic development and adult tissue homeostasis (Joulia et al., 2005).
Data availability statement
The datasets presented in this study can be found in online repositories. The names of the repository/repositories and accession number(s) can be found below: https://www.ncbi.nlm.nih.gov/, PRJNA818099.
Author contributions
GW, XZ, and BY designed the experiment. YC, YZ, CD, and LL carried out the experiments. YC and BY wrote the manuscript. YC and BY analyzed the experimental results.
Funding
Projects are subsidized by the National Natural Science Foundation of China (31861133019 and 32172501).
Acknowledgments
We thank our colleague Q-YW for the help with insect rearing.
Conflict of interest
The authors declare that the research was conducted in the absence of any commercial or financial relationships that could be construed as a potential conflict of interest.
Publisher’s note
All claims expressed in this article are solely those of the authors and do not necessarily represent those of their affiliated organizations, or those of the publisher, the editors, and the reviewers. Any product that may be evaluated in this article, or claim that may be made by its manufacturer, is not guaranteed or endorsed by the publisher.
Supplementary material
The Supplementary Material for this article can be found online at: https://www.frontiersin.org/articles/10.3389/fphys.2022.953538/full#supplementary-material
References
Alexa A., Rahnenfuhrer J. (2010). topGO: Enrichment analysis for gene ontology. R package version 2.0. doi:10.18129/B9.bioc.topGO
Amin A., Li Y. B., Finkelstein R. (1999). Hedgehog activates the EGF receptor pathway during Drosophila head development. Development 126 (12), 2623–2630. doi:10.1242/dev.126.12.2623
Anders S., Huber W. (2010). Differential expression analysis for sequence count data. Genome Biol. 11 (10), R106. doi:10.1186/gb-2010-11-10-r106
Anton S., Homberg U. (1999). “Antennal lobe structure,” in Insect olfaction (Berlin, Heidelberg: Springer), 97–124. doi:10.1007/978-3-662-07911-9_5
Apweiler R., Bairoch A., Wu C. H., Barker W. C., Boeckmann B., Ferro S., et al. (2004). UniProt: The universal protein knowledgebase. Nucleic Acids Res. 32 (Suppl. l_1), D115–D119. doi:10.1093/nar/gkh131
Ashburner M., Ball C. A., Blake J. A., Botstein D., Butler H., Cherry J. M., et al. (2000). Gene ontology: Tool for the unification of biology. The gene ontology consortium. Nat. Genet. 25 (1), 25–29. doi:10.1038/75556
Bader G. D., Hogue C. W. V. (2003). An automated method for finding molecular complexes in large protein interaction networks. BMC Bioinforma. 4, 2. doi:10.1186/1471-2105-4-2
Banerjee U., Girard J. R., Goins L. M., Spratford C. M. (2019). Drosophila as a genetic model for hematopoiesis. Genetics 211 (2), 367–417. doi:10.1534/genetics.118.300223
Berens A. J., Tibbetts E. A., Toth A. L. (2017). Cognitive specialization for learning faces is associated with shifts in the brain transcriptome of a social wasp. J. Exp. Biol. 220 (12), 2149–2153. doi:10.1242/jeb.155200
Berg B. G., Galizia C. G., Brandt R., Mustaparta H. (2002). Digital atlases of the antennal lobe in two species of tobacco budworm moths, the oriental Helicoverpa assulta (male) and the American Heliothis virescens (male and female). J. Comp. Neurol. 446 (2), 123–134. doi:10.1002/cne.10180
Berg B. G., Tumlinson J. H., Mustaparta H. (1995). Chemical communication in Heliothine moths. IV. Receptor neuron responses to pheromone compounds and formate analogs in the male tobacco budworm moth Heliothis virescens. J. Comp. Physiol. A 177, 527–534. doi:10.1007/BF00207182
Brandes U. (2008). On variants of shortest-path betweenness centrality and their generic computation. Soc. Netw. 30 (2), 136–145. doi:10.1016/j.socnet.2007.11.001
Butler B. A., Soong J., Gergen J. P. (1992). The Drosophila segmentation gene runt has an extended cis-regulatory region that is required for vital expression at other stages of development. Mech. Dev. 39 (1), 17–28. doi:10.1016/0925-4773(92)90022-C
Cachero S., Ostrovsky A. D., Yu J. Y., Dickson B. J., Jefferis G. S. (2010). Sexual dimorphism in the fly brain. Curr. Biol. 20, 1589–1601. doi:10.1016/j.cub.2010.07.045
Calkins T. L., Tamborindeguy C., Pietrantonio P. V. (2018). GPCR annotation, G proteins, and transcriptomics of fire ant (Solenopsis invicta) queen and worker brain: An improved view of signaling in an invasive superorganism. Gen. Comp. Endocrinol. 264, 89–103. doi:10.1016/j.ygcen.2018.12.008
Choe C. P., Brown S. J. (2007). Evolutionary flexibility of pair-rule patterning revealed by functional analysis of secondary pair-rule genes, paired and sloppy-paired in the short-germ insect, Tribolium castaneum. Dev. Biol. 302 (1), 281–294. doi:10.1016/j.ydbio.2006.09.037
Cinel S. D., Taylor S. J. (2019). Prolonged bat call exposure induces a broad transcriptional response in the male fall armyworm (Spodoptera frugiperda; Lepidoptera: Noctuidae) brain. Front. Behav. Neurosci. 13, 36. doi:10.3389/fnbeh.2019.00036
Dahl E., Balling R., Koseki H. (1997). Pax genes and organogenesis. Bioessays 19 (9), 755–765. doi:10.1002/bies.950190905
Dauwalder B., Tsujimoto S., Moss J., Mattox W. (2002). The Drosophila takeout gene is regulated by the somatic sex-determination pathway and affects male courtship behavior. Genes Dev. 16 (22), 2879–2892. doi:10.1101/gad.1010302
Deng Y. Y., Li J. Q., Wu S. F., Zhu Y. P., Chen Y. W., He F. C. (2006). Integrated nr database in protein annotation system and its localization. Comput. Eng. 32 (5), 71–72. doi:10.1109/INFOCOM.2006.241
Dong J. F., Jiang N. J., Zhao X. C., Tang R. (2020). Antennal lobe atlas of an emerging corn pest, Athetis dissimilis. Front. Neuroanat. 14, 23. doi:10.3389/fnana.2020.00023
Eddy S. R. (1998). Profile hidden Markov models. Bioinformatics 14 (9), 755–763. doi:10.1093/bioinformatics/14.9.755
Edwards A. C., Zwarts L., Yamamoto A., Callaerts P., Mackay T. F. (2009). Mutations in many genes affect aggressive behavior in Drosophila melanogaster. BMC Biol. 7 (1), 29. doi:10.1186/1741-7007-7-29
Elsik C. G., Worley K. C., Bennett A. K., Beye M., Camara F., Childers C. P., et al. (2014). Finding the missing honey bee genes: Lessons learned from a genome upgrade. BMC Genomics 15 (1), 86. doi:10.1186/1471-2164-15-86
Ferguson L., Marletaz F., Carter J-M., Taylor W. R., Gibbs M., Breuker C. J., et al. (2014). Ancient expansion of the Hox cluster in Lepidoptera generated four Homeobox genes implicated in extra-embryonic tissue formation. PLoS Genet. 10 (10), e1004698. doi:10.1371/journal.pgen.1004698
Finn R. D., Bateman A., Clements J., Coggill P., Eberhardt R. Y., Eddy S. R., et al. (2014). Pfam: The protein families database. Nucleic Acids Res. 42 (D1), D222–D230. doi:10.1093/nar/gkt1223
Fishilevich E., Bowling A. J., Frey M. L. F., Wang P-H., Lo W., Rangasamy M., et al. (2019). RNAi targeting of rootworm Troponin I transcripts confers root protection in maize. Insect biochem. Mol. Biol. 104, 20–29. doi:10.1016/j.ibmb.2018.09.006
Fonseca R. N. D., Levetzow C. V., Kalscheuer P., Basal A., Zee M. V. D., Roth S., et al. (2008). Self-regulatory circuits in dorsoventral axis formation of the short-germ beetle Tribolium Castaneum. Dev. Cell 14 (4), 605–615. doi:10.1016/j.devcel.2008.02.011
Fujii T., Pichel J. G., Taira M., Toyama R., Dawid I. B., Westphal H., et al. (1994). Expression patterns of the murine LIM class homeobox gene lim1 in the developing brain and excretory system. Dev. Dyn. 199 (1), 73–83. doi:10.1002/aja.1001990108
Grabherr M. G., Haas B. J., Yassour M., Levin J. Z., Thompson D. A., Amit I., et al. (2011). Full-length transcriptome assembly from RNA-Seq data without a reference genome. Nat. Biotechnol. 29 (7), 644–652. doi:10.1038/nbt.1883
Guan D. L., Ding R. R., Hu X. Y., Yang X. R., Xu S. Q., Gu W., et al. (2019). Cadmium-induced genome-wide DNA methylation changes in growth and oxidative metabolism in Drosophila melanogaster. BMC Genomics 20 (1), 356. doi:10.1186/s12864-019-5688-z
Gutorov R., Katz B., Rhodes-Mordov E., Zaguri R., Brandwine-Shemmer T., Minke B., et al. (2022). The role of membrane lipids in light-activation of Drosophila TRP channels. Biomolecules 12 (3), 382. doi:10.3390/biom12030382
Hammond T. R., Dufort C., Dissing-Olesen L., Giera S., Young A., Wysoker A., et al. (2019). Single-cell RNA sequencing of microglia throughout the mouse lifespan and in the injured brain reveals complex cell-state changes. Immunity 50 (1), 253–271. doi:10.1016/j.immuni.2018.11.004
Hansson B. S., Almaas T. J., Anton S. (1995). Chemical communication in Heliothine moths. V. antennal lobe projection patterns of pheromone-detecting olfactory receptor neurons in the male Heliothis virescens (Lepidoptera, Noctuidae). J. Comp. Physiol. A 177, 535–543. doi:10.1007/BF00207183
He C., Liu S., Liang J., Zeng Y., Wang S., Wu Q., et al. (2020). Genome‐wide identification and analysis of nuclear receptors genes for lethal screening against Bemisia tabaci Q. Pest Manag. Sci. 76, 2040–2048. doi:10.1002/ps.5738
Hepker J., Wang Q. T., Motzny C. K., Holmgren R., Orenic T. V. (1997). Drosophila cubitus interruptus forms a negative feedback loop with patched and regulates expression of Hedgehog target genes. Development 124 (2), 549–558. doi:10.1242/dev.124.2.549
Huang Y. P., Takanashi T., Hoshizaki S., Tatsuki S., Honda H., Yoshiyasu Y., et al. (1998). Geographic variation in sex pheromone of Asian corn borer, Ostrinia furnacalis, in Japan. J. Chem. Ecol. 24 (12), 2079–2088. doi:10.1023/a:1020737726636
Huerta-Cepas J., Szklarczyk D., Forslund K., Cook H., Heller D., Walter M. C., et al. (2016). eggNOG 4.5: a hierarchical orthology framework with improved functional annotations for eukaryotic, prokaryotic and viral sequences. Nucleic Acids Res. 44, D286–D293. doi:10.1093/nar/gkv1248
Hughes M. E., Grant G. R., Paquin C., Qian J., Nitabach M. N. (2012). Deep sequencing the circadian and diurnal transcriptome of Drosophila brain. Genome Res. 22 (7), 1266–1281. doi:10.1101/gr.128876.111
Iatsenko I., Kondo S., Mengin-Lecreulx D., Lemaitre B. (2016). PGRP-SD, an extracellular pattern-recognition receptor, enhances peptidoglycan-mediated activation of the Drosophila Imd pathway. Immunity 45 (5), 1013–1023. doi:10.1016/j.immuni.2016.10.029
Ishikawa Y., Takanashi T., Kim C-g., Hoshizaki S., Tatsuki S., Huang Y. P. (1999). Ostrinia spp. in Japan: Their host plants and sex pheromones. Entomologia Exp. Appl. 91, 237–244. doi:10.1007/978-94-017-1890-5_30
Janssens S., Denayer T., Deroo T., Roy F. V., Vleminckx K. (2010). Direct control of Hoxd1 and Irx3 expression by Wnt/β-catenin signaling during anteroposterior patterning of the neural axis in Xenopus. Int. J. Dev. Biol. 54 (10), 1435–1442. doi:10.1387/ijdb.092985sj
Joulia L., Bourbon H. M., Cribbs D. L. (2005). Homeotic proboscipedia function modulates hedgehog-mediated organizer activity to pattern adult Drosophila mouthparts. Dev. Biol. 278 (2), 496–510. doi:10.1016/j.ydbio.2004.11.003
Kanehisa M., Goto S., Kawashima S., Okuno Y., Hattori M. (2004). The KEGG resource for deciphering the genome. Nucleic Acids Res. 32 (Database issue), D277–D280. doi:10.1093/nar/gkh063
Karpati Z., Dekker T., Hansson B. S. (2008). Reversed functional topology in the antennal lobe of the male European corn borer. J. Exp. Biol. 211 (20), 2841–2848. doi:10.1242/jeb.017319
Kasai Y., Stahl S., Crews S. (1998). Specification of the Drosophila CNS midline cell lineage: Direct control of single-minded transcription by dorsal/ventral patterning genes. Gene Expr. 7 (3), 171–189.
Kitzmann P., Weißkopf M., Schacht M. I., Bucher G. (2017). A key role for foxQ2 in anterior head and central brain patterning in insects. Development 144 (16), 2969–2981. doi:10.1242/dev.147637
Koonin E. V., Fedorova N. D., Jackson J. D., Jacobs A. R., Krylov D. M., Makarova K. S., et al. (2004). A comprehensive evolutionary classification of proteins encoded in complete eukaryotic genomes. Genome Biol. 5 (2), R7. doi:10.1186/gb-2004-5-2-r7
Kurusu M., Nagao T., Walldorf U., Flister S., Gehring W. J., Furukubo-Tokunaga K. (2000). Genetic control of development of the mushroom bodies, the associative learning centers in the Drosophila brain, by the eyeless, twin of eyeless, and dachshund genes. Proc. Natl. Acad. Sci. U. S. A. 97 (5), 2140–2144. doi:10.1073/pnas.040564497
Langmead B., Trapnell C., Pop M., Salzberg S. L. (2009). Ultrafast and memory-efficient alignment of short DNA sequences to the human genome. Genome Biol. 10 (3), R25. doi:10.1186/gb-2009-10-3-r25
Li B., Dewey C. N. (2011). Rsem: Accurate transcript quantification from RNA-seq data with or without a reference genome. BMC Bioinforma. 12 (1), 323. doi:10.1186/1471-2105-12-323
Li B. Q., You J., Chen L., Zhang J., Zhang N., Li H. P., et al. (2013). Identification of lung-cancer-related genes with the shortest path approach in a protein-protein interaction network. Biomed. Res. Int. 2013, 267375. doi:10.1155/2013/267375
Li Z. Z., Yu T. T., Chen Y. P., Heerman M., He J. F., Huang J. N., et al. (2019). Brain transcriptome of honey bees (Apis mellifera) exhibiting impaired olfactory learning induced by a sublethal dose of imidacloprid. Pestic. Biochem. Physiol. 156, 36–43. doi:10.1016/j.pestbp.2019.02.001
Liu W., Jiang X. C., Cao S., Yang B., Wang G. R. (2018). Functional studies of sex pheromone receptors in Asian corn borer Ostrinia furnacalis. Front. Physiol. 9, 591. doi:10.3389/fphys.2018.00591
Lowe C. J., Wu M., Salic A., Evans L., Lander E., Stange-Thomann N., et al. (2003). Anteroposterior patterning in hemichordates and the origins of the chordate nervous system. Cell 113 (7), 853–865. doi:10.1016/s0092-8674(03)00469-0
Lugena A. B., Zhang Y., Menet J. S., Merlin C. (2019). Genome-wide discovery of the daily transcriptome, DNA regulatory elements and transcription factor occupancy in the monarch butterfly brain. PLoS Genet. 15 (7), e1008265. doi:10.1371/journal.pgen.1008265
Mardon G., Solomon M. N., Rubin G. M. (1994). Dachshund encodes a nuclear protein required for normal eye and leg development in Drosophila. Development 120 (12), 3473–3486. doi:10.1242/dev.120.12.3473
Míguez D. G., García-Morales D., Casares F. (2020). Control of size, fate and time by the Hh morphogen in the eyes of flies. Curr. Top. Dev. Biol. 137, 307–332. doi:10.1016/bs.ctdb.2019.10.011
Miura N., Nakagawa T., Touhara K., Ishikawa Y. (2010). Broadly and narrowly tuned odorant receptors are involved in female sex pheromone reception in Ostrinia moths. Insect biochem. Mol. Biol. 40 (1), 64–73. doi:10.1016/j.ibmb.2009.12.011
Müller U., Muller U. (2000). Prolonged activation of cAMP-dependent protein kinase during conditioning induces long-term memory in honeybees. Neuron 27 (1), 159–168. doi:10.1016/s0896-6273(00)00017-9
Mutuura A., Munroe E. (1970). Taxonomy and distribution of the European corn borer and allied species: Genus Ostrinia (Lepidoptera: Pyralidae). Mem. Entomol. Soc. Can. 71 (S71), 1–112. doi:10.4039/entm10271fv
Noguchi T., Miller K. G. (2003). A role for actin dynamics in individualization during spermatogenesis in Drosophila melanogaster. Development 130, 1805–1816. doi:10.1242/dev.00406
Ntini E., Wimmer E. A. (2011). Unique establishment of procephalic head segments is supported by the identification of cis-regulatory elements driving segment-specific segment polarity gene expression in Drosophila. Dev. Genes Evol. 221 (1), 1–16. doi:10.1007/s00427-011-0354-8
Orlans J., Vincent-Monegat C., Rahioui I., Sivignon C., Butryn A., Soulère L., et al. (2021). PGRP-LB: An inside view into the mechanism of the amidase reaction. Int. J. Mol. Sci. 22, 4957. doi:10.3390/ijms22094957
Picchio L., Legagneux V., Deschamps S., Renaud Y., Chauveau S., Paillard L., et al. (2018). Bruno-3 regulates sarcomere component expression and contributes to muscle phenotypes of myotonic dystrophy type 1. Dis. Model. Mech. 11 (5), dmm031849. doi:10.1242/dmm.031849
Posnien N., Koniszewski N. D. B., Hein H. J., Bucher G. (2011). Candidate gene screen in the red flour beetle Tribolium reveals six3 as ancient regulator of anterior median head and central complex development. PLoS Genet. 7 (12), e1002416. doi:10.1371/journal.pgen.1002416
Roark M., Sturtevant M. A., Emery J., Vaessin H., Grell E., Bier E., et al. (1995). scratch, a pan-neural gene encoding a zinc finger protein related to snail, promotes neuronal development. Genes Dev. 9 (19), 2384–2398. doi:10.1101/gad.9.19.2384
Roignant J-Y., Legent K., Janody F., Treisman J. E. (2010). The transcriptional co-factor Chip acts with LIM-homeodomain proteins to set the boundary of the eye field in Drosophila. Development 137 (2), 273–281. doi:10.1242/dev.041244
Romain L., Oxley P. R., Kronauer D. J. C. (2018). Clonal raider ant brain transcriptomics identifies candidate molecular mechanisms for reproductive division of labor. BMC Biol. 16 (1), 89. doi:10.1186/s12915-018-0558-8
Rospars J. P., Hildebrand J. G. (2000). Sexually dimorphic and isomorphic glomeruli in the antennal lobes of the sphinx moth Manduca sexta. Chem. Senses 25 (2), 119–129. doi:10.1093/chemse/25.2.119
Royet J., Gupta D., Dziarski R. (2011). Peptidoglycan recognition proteins: Modulators of the microbiome and inflammation. Nat. Rev. Immunol. 11 (12), 837–851. doi:10.1038/nri3089
Satoh G., Wang Y., Zhang P., Satoh N. (2001). Early development of amphioxus nervous system with special reference to segmental cell organization and putative sensory cell precursors: A study based on the expression of pan-neuronal marker gene hu/elav. J. Exp. Zool. 291 (4), 354–364. doi:10.1002/jez.1134
Scharfman H. E., Binder D. K. (2013). Aquaporin-4 water channels and synaptic plasticity in the hippocampus. Neurochem. Int. 63 (7), 702–711. doi:10.1016/j.neuint.2013.05.003
Schmid M. R., Dziedziech A., Arefin B., Kienzle T., Wang Z., Akhter M., et al. (2019). Insect hemolymph coagulation: Kinetics of classically and non-classically secreted clotting factors. Insect biochem. Mol. Biol. 109, 63–71. doi:10.1016/j.ibmb.2019.04.007
Shannon P., Markiel A., Ozier O., Baliga N. S., Wang J. T., Ramage D., et al. (2003). Cytoscape: A software environment for integrated models of biomolecular interaction networks. Genome Res. 13 (11), 2498–2504. doi:10.1101/gr.1239303
Sinigaglia C., Busengdal H., Leclère L., Technau U., Mail F. R. (2013). The bilaterian head patterning gene six3/6 controls aboral domain development in a cnidarian. PLoS Biol. 11 (2), e1001488. doi:10.1371/journal.pbio.1001488
Skiri H. T., Helge R., Berg B. G., Mustaparta H. (2005). Consistent organization of glomeruli in the antennal lobes of related species of Heliothine moths. J. Comp. Neurol. 491 (4), 367–380. doi:10.1002/cne.20692
Steffen M. A., Rehan S. M. (2020). Genetic signatures of dominance hierarchies reveal conserved cis‐regulatory and brain gene expression underlying aggression in a facultatively social bee. Genes Brain Behav. 19 (1), e12597. doi:10.1111/gbb.12597
Szklarczyk D., Gable A. L., Lyon D., Junge A., Wyder S., Huerta-Cepas J., et al. (2019). STRING v11: Protein-protein association networks with increased coverage, supporting functional discovery in genome-wide experimental datasets. Nucleic Acids Res. 47 (D1), D607–D613. doi:10.1093/nar/gky1131
Tang H., Kambris Z., Lemaitre B., Hashimoto C. (2008). A serpin that regulates immune melanization in the respiratory system of Drosophila. Dev. Cell 15 (4), 617–626. doi:10.1016/j.devcel.2008.08.017
Tanji T., Hu X. D., Weber A. N. R., Ip Y. T. (2007). Toll and IMD pathways synergistically activate an innate immune response in Drosophila melanogaster. Mol. Cell. Biol. 27 (12), 4578–4588. doi:10.1128/MCB.01814-06
Tatusov R. L., Galperin M. Y., Natale D. A., Koonin E. V. (2000). The COG database: A tool for genome-scale analysis of protein functions and evolution. Nucleic Acids Res. 28 (1), 33–36. doi:10.1093/nar/28.1.33
Trapnell C., Williams B. A., Pertea G., Mortazavi A., Kwan G., Baren M., et al. (2010). Transcript assembly and quantification by RNA-Seq reveals unannotated transcripts and isoform switching during cell differentiation. Nat. Biotechnol. 28 (5), 511–515. doi:10.1038/nbt.1621
Vleurinck C., Raub S., Sturgill D., Oliver B., Beye M. (2016). Linking genes and brain development of honeybee workers: A whole-transcriptome approach. PLoS One 11 (8), e0157980. doi:10.1371/journal.pone.0157980
von Mering C., Jensen L. J., Snel B., Hooper S. D., Krupp M., Foglierini M., et al. (2005). String: Known and predicted protein-protein associations, integrated and transferred across organisms. Nucleic Acids Res. 33, D433–D437. doi:10.1093/nar/gki005
Wagner C. R., Mahowald A. P., Miller K. G. (2002). One of the two cytoplasmic actin isoforms in Drosophila is essential. Proc. Natl. Acad. Sci. U. S. A. 99 (12), 8037–8042. doi:10.1073/pnas.082235499
Walker Ⅲ W. B., Roy A., Anderson P., Schlyter F., Hansson B. S., Larsson M. C., et al. (2019). Transcriptome analysis of gene families involved in chemosensory function in Spodoptera littoralis (Lepidoptera: Noctuidae). BMC Genomics 20 (1), 428. doi:10.1186/s12864-019-5815-x
Wang B., Liu Y., Wang G. R. (2018). Proceeding from in vivo functions of pheromone receptors: Peripheral-coding perception of pheromones from three closely related species, Helicoverpa armigera, H. assulta, and Heliothis virescens. Front. Physiol. 9, 1188. doi:10.3389/fphys.2018.01188
Wang G. B., Zhang J. J., Shen Y. W., Zheng Q., Feng M., Xiang X. W., et al. (2015). Transcriptome analysis of the brain of the silkworm Bombyx mori infected with Bombyx mori nucleopolyhedrovirus: A new insight into the molecular mechanism of enhanced locomotor activity induced by viral infection. J. Invertebr. Pathol. 128, 37–43. doi:10.1016/j.jip.2015.04.001
Wang M. Y., Liu Y., Wen T. G., Liu W. W., Gao Q. H., Zhao J., et al. (2020). Chromatin accessibility and transcriptome landscapes of Monomorium pharaonis brain. Sci. Data 7 (1), 217. doi:10.1038/s41597-020-0556-x
Wilson R. J., Goodman J. L., Strelets V. B., Consortium F. (2008). FlyBase: Integration and improvements to query tools. Nucleic Acids Res. 36, D588–D593. doi:10.1093/nar/gkm930
Xin S. H., Zhang W. J. (2021). Construction and analysis of the protein-protein interaction network for the detoxification enzymes of the silkworm, Bombyx mori. Arch. Insect Biochem. Physiol. 108 (4), e21850. doi:10.1002/arch.21850
Xin S. H., Zhang W. J. (2020). Construction and analysis of the protein-protein interaction network for the olfactory system of the silkworm Bombyx mori. Arch. Insect Biochem. Physiol. 105, e21737. doi:10.1002/arch.21737
Yang B., Ozaki K., Ishikawa Y., Matsuo T. (2015). Identification of candidate odorant receptors in Asian corn borer Ostrinia furnacalis. PLoS One 10 (3), e0121261. doi:10.1371/journal.pone.0121261
Yang Y. T., Lee M. R., Lee S. J., Kim S., Nai Y. S., Kim J. S., et al. (2018). Tenebrio molitor Gram-negative-binding protein 3 (TmGNBP3) is essential for inducing downstream antifungal Tenecin 1 gene expression against infection with Beauveria bassiana JEF-007. Insect Sci. 25 (6), 969–977. doi:10.1111/1744-7917.12482
Zeitlinger J., Zinzen R. P., Stark A., Kellis M., Zhang H., Young R. A., et al. (2007). Whole-genome ChIP-chip analysis of Dorsal, Twist, and Snail suggests integration of diverse patterning processes in the Drosophila embryo. Genes Dev. 21 (4), 385–390. doi:10.1101/gad.1509607
Zhan M., Yamaza H., Sun Y., Sinclair J., Li H., Zou S., et al. (2007). Temporal and spatial transcriptional profiles of aging in Drosophila melanogaster. Genome Res. 17 (8), 1236–1243. doi:10.1101/gr.6216607
Zhang G. L., Zhang W. J. (2020). Direct protein-protein interaction network for insecticide resistance based on subcellular localization analysis in Drosophila melanogaster. J. Environ. Sci. Health. B 55 (8), 732–748. doi:10.1080/03601234.2020.1782114
Zhang G. L., Zhang W. J. (2019). Protein-protein interaction network analysis of insecticide resistance molecular mechanism in Drosophila melanogaster. Arch. Insect Biochem. Physiol. 100 (1), e21523. doi:10.1002/arch.21523
Zhao X. C., Berg B. G. (2010). Arrangement of output information from the 3 macroglomerular units in the heliothine moth Helicoverpa assulta: Morphological and physiological features of male-specific projection neurons. Chem. Senses 35 (6), 511–521. doi:10.1093/chemse/bjq043
Zhu H. S., Casselman A., Reppert S. M. (2008). Chasing migration genes: A brain expressed sequence tag resource for summer and migratory monarch butterflies (Danaus plexippus). PLoS One 3 (1), e1345. doi:10.1371/journal.pone.0001345
Keywords: PPI network, sex-biased genes, transcriptome analysis, Ostrinia furnacalis, brain neurodevelopmental genes
Citation: Chang Y, Yang B, Zhang Y, Dong C, Liu L, Zhao X and Wang G (2022) Identification of sex-biased and neurodevelopment genes via brain transcriptome in Ostrinia furnacalis. Front. Physiol. 13:953538. doi: 10.3389/fphys.2022.953538
Received: 26 May 2022; Accepted: 01 July 2022;
Published: 08 August 2022.
Edited by:
Ya-Nan Zhang, Huaibei Normal University, ChinaReviewed by:
Dingze Mang, Tokyo University of Agriculture and Technology, JapanKang He, Zhejiang University, China
Sheng Sheng, Jiangsu University of Science and Technology, China
Copyright © 2022 Chang, Yang, Zhang, Dong, Liu, Zhao and Wang. This is an open-access article distributed under the terms of the Creative Commons Attribution License (CC BY). The use, distribution or reproduction in other forums is permitted, provided the original author(s) and the copyright owner(s) are credited and that the original publication in this journal is cited, in accordance with accepted academic practice. No use, distribution or reproduction is permitted which does not comply with these terms.
*Correspondence: Guirong Wang, wangguirong@caas.cn; Bin Yang, byang@ippcaas.cn