- 1Duke Molecular Physiology Institute, Duke University School of Medicine, Duke University, Durham, NC, United States
- 2Department of Orthopaedic Surgery, Duke University School of Medicine, Duke University, Durham, NC, United States
- 3Department of Biomedical Engineering, Pratt School of Engineering, Duke University, Durham, NC, United States
- 4Department of Genetics, University of North Carolina School of Medicine, University of North Carolina, Chapel Hill, NC, United States
- 5Department of Medicine, Duke University School of Medicine, Duke University, Durham, NC, United States
Exercise affects the expression of microRNAs (miR/s) and muscle-derived extracellular vesicles (EVs). To evaluate sarcoplasmic and secreted miR expression in human skeletal muscle in response to exercise-mimetic contractile activity, we utilized a three-dimensional tissue-engineered model of human skeletal muscle (“myobundles”). Myobundles were subjected to three culture conditions: no electrical stimulation (CTL), chronic low frequency stimulation (CLFS), or intermittent high frequency stimulation (IHFS) for 7 days. RNA was isolated from myobundles and from extracellular vesicles (EVs) secreted by myobundles into culture media; miR abundance was analyzed by miRNA-sequencing. We used edgeR and a within-sample design to evaluate differential miR expression and Pearson correlation to evaluate correlations between myobundle and EV populations within treatments with statistical significance set at p < 0.05. Numerous miRs were differentially expressed between myobundles and EVs; 116 miRs were differentially expressed within CTL, 3 within CLFS, and 2 within IHFS. Additionally, 25 miRs were significantly correlated (18 in CTL, 5 in CLFS, 2 in IHFS) between myobundles and EVs. Electrical stimulation resulted in differential expression of 8 miRs in myobundles and only 1 miR in EVs. Several KEGG pathways, known to play a role in regulation of skeletal muscle, were enriched, with differentially overrepresented miRs between myobundle and EV populations identified using miEAA. Together, these results demonstrate that in vitro exercise-mimetic contractile activity of human engineered muscle affects both their expression of miRs and number of secreted EVs. These results also identify novel miRs of interest for future studies of the role of exercise in organ-organ interactions in vivo.
Introduction
Skeletal muscle is the largest organ system in the human body. It is responsible for locomotion and known to play critical roles in whole-body glucose metabolism and energy homeostasis. Skeletal muscle also contributes to homeostatic adaptation in peripheral organs (Plomgaard et al., 2012; Guo et al., 2017). Importantly, emerging research in muscle-derived extracellular vesicles (EVs), which carry proteins, lipids, mRNAs and microRNAs (miRs), has provided further insight into mechanisms of crosstalk between muscle and other tissues (Guescini et al., 2010; Forterre et al., 2014; Yoon et al., 2014; Rome et al., 2019).
Epigenetic changes can generally be described as the alterations in gene expression profiles not attributed to changes in DNA sequence (Peschansky and Wahlestedt, 2014). There are three main types of epigenetic mechanisms: i) DNA methylation; ii) histone modification; and iii) non-coding RNA (ncRNA)-associated gene silencing (Lacal and Ventura, 2018; Jacques et al., 2019). Small regulatory RNAs (sRNA) are a class of ncRNA consisting of short interfering RNA (siRNA) and, of importance to this study, miRs. miRs are ∼15–25 nucleotides in length and are generally associated with gene silencing (O'Brien et al., 2018) via post-translational modification and RNA degradation. Canonically, miRs are synthesized through the microprocessor complex consisting of DGCR8, Drosha, and the enzyme ribonuclease III (Denli et al., 2004; O'Brien et al., 2018). All cells export various cellular cargo, including miRs, through EVs–which are synthesized via budding of the plasma membrane or through maturation of endosomes by which intraluminal vesicles accumulate, forming multivesicular bodies, which are then released through exocytosis (De Jong et al., 2014; Rome et al., 2019; Valentino et al., 2021).
The release of EVs by exercising muscle is one means by which exercise affects other organs and tissues (Nederveen et al., 2020; Zhang et al., 2021). Importantly, the number of muscle-derived EVs (specifically small EVs 100–130 nm in size, frequently referred as exosomes in early studies) increases following various modes of exercise (Frühbeis et al., 2015; Whitham et al., 2018; Denham and Spencer, 2020; Vechetti et al., 2021b). Additionally, expression of muscle-derived and circulating miRs are altered following acute and chronic exercise (Backes et al., 2014; Xu et al., 2015; Masi et al., 2016; Ogasawara et al., 2016). To date, studies that have sought to quantify miRs per EV have demonstrated very low molecule counts at ∼1-2 miRs per EV (Chevillet et al., 2014; Helwa et al., 2017), however the concentration of EVs in the circulation is very high, estimated at 1010 per mL (Johnsen et al., 2019), suggesting that EVs could be a possible means of gene silencing, which requires a concentration of ∼1,000 copies of a miR per cell (Mukherji et al., 2011). Further, recent work has estimated ∼5% of circulating tetraspanin-positive EVs are muscle-derived in vivo (Estrada et al., 2022).
The purpose of the current study was to evaluate the differential expression of miRs, identified and quantified using microRNA sequencing (miRNAseq), in both three-dimensional tissue-engineered models of human skeletal muscle (“myobundles”) (Madden et al., 2015) and their secreted EVs following chronic low frequency and intermittent high frequency electrical-stimulation (e-stim) treatment as a method of simulating exercise. E-stim is commonly used as an exercise mimetic for in vitro studies evaluating the effects of exercise on cellular physiology (Nikolić et al., 2012; Tarum et al., 2017). These in vitro skeletal muscle culture models (monolayer and engineered 3D tissues) provide a means of studying skeletal muscle development, function, and plasticity (Madden et al., 2015; Rao et al., 2018; Khodabukus, 2021), whilst also affording the capability to investigate the biological processes regulated by miRs in skeletal muscle (Cheng et al., 2016; Rhim et al., 2020). Furthermore, these systems can also be used to evaluate crosstalk between muscle and other cell types via co-culture and/or treating other cell types in isolation with EVs collected from muscle culture systems. To date, numerous studies have demonstrated effects of e-stim on metabolism, force production, and fiber phenotype (Nikolić et al., 2012; Khodabukus et al., 2015; Khodabukus et al., 2019; Chen et al., 2021) in 2D and 3D muscle culture models. However, the effects of e-stim on the expression of miRs within 3D-engineered skeletal muscle and muscle-derived EVs remain understudied. Furthermore, we sought to elucidate the correlation between intracellular and EV miRs to identify miRs that may be preferentially enriched in either muscle and EVs.
Methods
Human skeletal muscle samples were obtained from three donors (12 years old female, 18 years old female, and 16 years old male) with informed consent under Duke University Institutional Review Board (IRB) approved protocols (Pro00048509 and Pro00012628). Tissue samples were derived from paraspinal muscle and collected via surgical waste. An overview of the study design can be found in Figure 1 (generated using BioRender.com). Briefly, paraspinal muscle tissue was collected and subsequently minced and expanded prior to 3D muscle myobundle formation. Following myobundle formation, engineered tissues were subjected to one of three conditions; i) Control (CTL); ii) chronic low frequency stimulation (CLFS); or iii) intermittent high frequency stimulation (IHFS). Following treatment, culture media were collected and underwent EV isolation. Total RNA was then isolated from myobundles and EVs prior to submitting for miRNAseq. The methodologies used herein are described in further detail below.
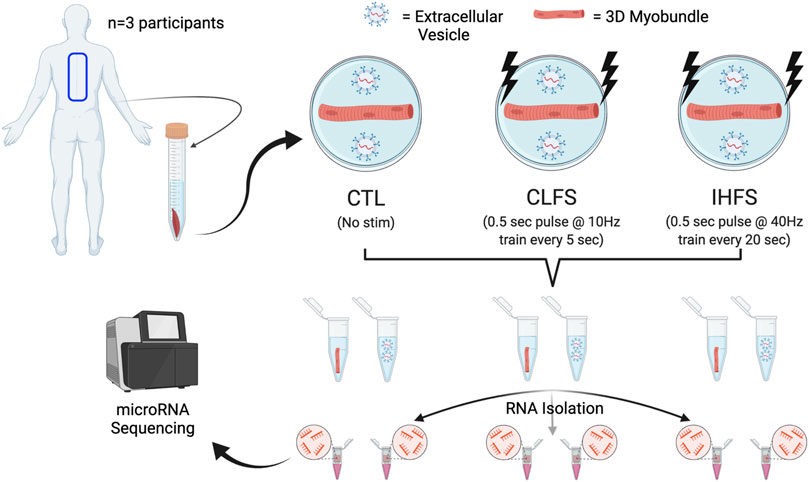
FIGURE 1. Study design. Abbreviations: CTL, control; CLFS, chronic low frequency stimulation; IHFS, intermittent high frequency stimulation.
Myoblast isolation and formation of engineered myobundle
Following tissue procurement), myoblasts were isolated, grown, and subsequently used to engineer three-dimensional engineered muscle tissues (myobundles) as previously described (Madden et al., 2015; Khodabukus et al., 2019; Khodabukus et al., 2020). Briefly, muscle samples were minced and digested with 0.05% trypsin at 37
Three-dimensional engineered muscle myobundles were generated within polydimethylsiloxane (PDMS) molds containing two semi-cylindrical wells (7 mm long
Electrical stimulation protocol
Three myobundles were generated from each participant tissue sample; myobundles were subjected to either a CTL condition, which received no electrical stimulation, or one of two electrical stimulation protocols (CLFS and IHFS), which began after 7 days of differentiation. Thus, each treatment condition had n = 3 myobundles. Myobundles subjected to CLFS received continuous electrical stimulation consisting of a 0.5s 10Hz train followed by a 4.5s rest. The IHFS protocol consisted of a 0.5s 40Hz train delivered every 20 s for 1h, followed by a 7 h rest period. E-stim protocols were conducted for a period of 7-days. Electrical impulses delivered to the myobundles were bipolar at an amplitude of 70 mA with a duration of 2 ms and were delivered using a D330 Multistim System (Digimeter Ltd.; Hertfordshire, United Kingdom) and programmed using a custom-made pulse generating Labview program (NI; Austin, TX, United States).
EV isolation
The serum-free media in the engineered muscle myobundle culture were collected and replaced with fresh media daily. EVs were isolated from the serum-free media supernatants collected on day 6 following cultures in the absence or presence of electrical stimulation. Briefly, a standard volume of 1 ml of supernatant was used for each corresponding myobundle. Supernatants were completely thawed on ice and subsequently centrifuged at 2,000 g for 10 min at 4
RNA extraction and sequencing
RNA isolation was performed on the muscle myobundles and EVs prior to submitting samples for miRNAseq at the Duke University Center for Genomic and Computational Biology. RNA was extracted from myobundles using the Aurum™ Total RNA Mini Kit (Bio-Rad; Hercules, CA, United States) in accordance with manufacturers specification. Extraction of RNA from EVs was performed using ExoQuick® Exosome RNA Column Purification Kit (System Bioscience; Palo Alto, CA, United States) in accordance with the manufacturer’s specifications. RNA was subsequently dissolved in 20 μL RNAse-free water and underwent quantification via the Qubit™ RNA HS Assay Kit (Thermo Fisher Scientific; Waltham, MA, United States) according to the manufacturer’s specifications.
Library preparation of myobundle and EV RNA samples was completed using the NextSeq 500 Mid-Output Library Kit (Illumina Inc.; San Diego, CA, United States). Following library preparation, sequencing was completed on the Illumina NextSeq500 (Illumina; San Diego, CA, United States) yielding 75bp paired-end reads at a depth of 16 M.
Data processing, normalization, and statistical analysis
The UMI-tools (Smith et al., 2017) algorithm was used to process miRNAseq data, parsing the Illumina adapters and extracting the unique molecular identifier (UMI) sequence for each read. Reads were then mapped to GRCh38/hg38 using the Bowtie alignment tool (Langmead et al., 2009). Reads were kept for use in subsequent analyses if they mapped to no more than 13 genomic locations. miR counts were compiled using custom scripts that compared the mapped read coordinates to the miRbase miR database (Kozomara and Griffiths-Jones, 2014). Deduplication of reads was then completed using UMI-tools, based on the mapping coordinate and the UMI identified from the read. Reads that matched the coordinates of the known mature miR were kept if they perfectly matched the coordinates of the miR seed whilst not varying more than 2 nt on the 3’ end of the mature miR.
Following the processing of data, a total of 991 miRs were identified in the raw, non-normalized data set. These raw data were then filtered and normalized using the BioConductor package edgeR (Robinson et al., 2010; McCarthy et al., 2012; Chen et al., 2016). Briefly, raw data (991 miRs) were read into R (R Core team, 2020) and subsequently filtered to remove low expressors using a counts per million (CPM) threshold of 0.5 (corresponding to ∼10 counts) whilst additionally requiring more than one sample to meet this threshold to be included in the filtered data set analyses. Following filtering, normalization factors were calculated using the trimmed mean of M-values method, and dispersion was estimated. We used a generalized linear model (GLM) quasi-negative binomial regression (QLF-test) to elucidate differentially expressed miRs while accounting for within sample comparisons with an a-priori significance threshold of p < 0.05. Importantly, QLF-tests were performed between populations (EV vs. MB) within treatment conditions (CTL, CLFS, IHFS), and within each population between each treatment condition (i.e., EV CLFS vs. CTL; MB CLFS vs. CTL). Critically, we chose to normalize the myobundle and EV data separately for the between treatment within population (myobundle or EV) analyses and perform normalization again on the total data set for the within treatment, between population analyses. Because of the small sample size and the discovery nature of this investigation, we opted to forego use of adjusted p-values to identify significant miRs. Following differential expression analysis, miRs meeting the significance threshold were evaluated for correlation with treatment using JMP Pro (JMP, Version 16, SAS Institute Inc., Cary, NC, 1989–2022) using TMM normalized counts. Additionally, we evaluated the overlap of differentially expressed miRs and normalization outputs using Venn diagram analysis via Venny (Oliveros, 2015). A repeated measures one-way ANOVA was used to evaluate differences between populations for miRs identified as being enriched in either the myobundle or EV populations. In the case of significance, we performed paired samples t-tests between populations, within each treatment, to identify differences in raw counts between populations.
Results
Differential miR expression within treatment
Given that exercise could change miRNA expression and secretion, we evaluated the differential expression pattern of miRs within each treatment condition in both the myobundle and EVs. These analyses yielded 152 differentially expressed miRs overall (p < 0.05, Supplementary Table S1). Seven of the top ten miRs (miR-27a-5p, -133a-5p, -107, -181a-3p, -590-3p, -37a-5p, and -1185-1-3p) were enriched in the myobundle (p < 0.001; Figure 2A), whereas three (miR-483-5p, -342-3p, and let-7d-3p) were enriched in EVs (p < 0.001; Figure 2A).
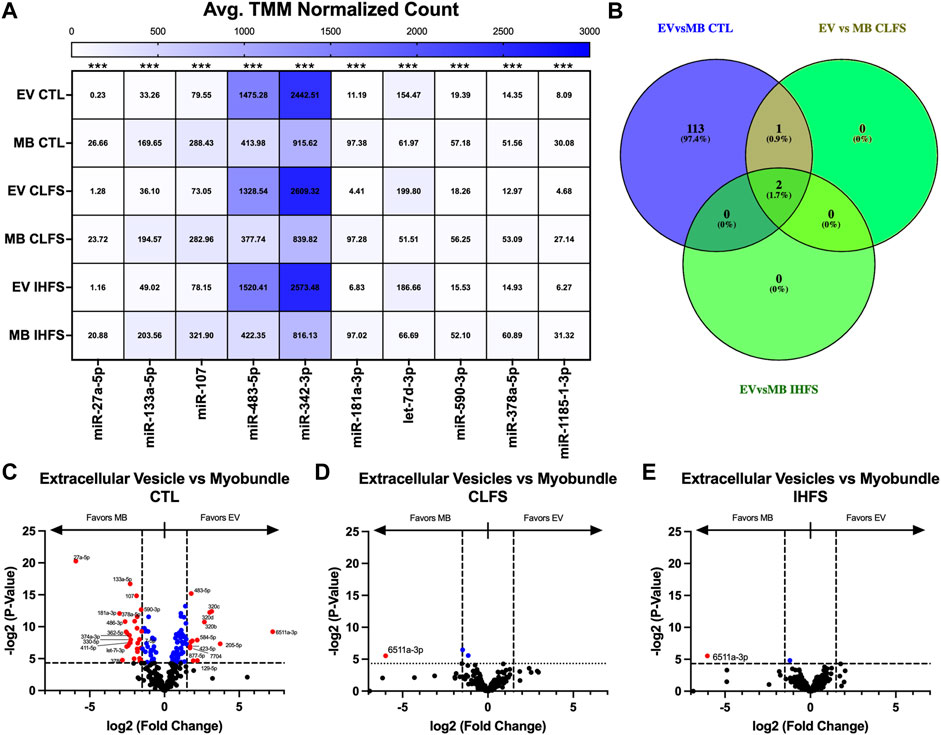
FIGURE 2. Differential miR Expression Between Population and Within Treatment Condition. Abbreviations: EV, extracellular vesicles; MB, myobundle; CTL, control; CLFS, chronic low frequency stimulation; IHFS, intermittent high frequency stimulation. Legend: data for Panel (A) is presented as mean normalized count. Panel (B) represents overlap of miRs for analyses completed. Panel (C–E) are volcano plots with blue data points depicting p < 0.05 and red data points depicting p < 0.05 and logFC of >1.5 or < -1.5.
Exercise has the potential to affect muscle and EV miRs differently. We therefore, evaluated for differences in miR expression within the myobundles compared with EVs to identify miRs that could be differentially expressed in tissue vs. secreted vesicles in response to the exercise-mimetic treatments. Comparing myobundle vs. EV populations, we identified 116 miRs differentially expressed in the CTL treatment group (p < 0.05, Figure 2C), 3 differentially expressed miRs (miR-543, -487b-3p, and -6511a-3p) in the CLFS treatment group (p < 0.05; Figure 2D), and 2 differentially expressed miRs (miR-6511a-3p and -543) in the IHFS treatment group (p < 0.05; Figure 2E). Notably, in our analysis of overlap between treatments groups, only miR-6511a-3p and -543 were significantly differentially expressed (different in myobundle vs. EV) in all 3 treatment conditions and only miR-487b-3p (enriched in myobundle relative to EVs) was differentially expressed in both the CTL and CLFS groups (Figure 2B). Overall, these data demonstrate that e-stim upregulates select miRs that largely favor the myobundle and thus may play a role in tissue regulatory processes.
Given the possibility that EVs transduce signals directly from muscle, we also identified miRs whose expression in EVs was significantly correlated with expression in myobundles (Table 1; Supplementary Figure S2). In the CTL treatment condition, we identified 18 miRs that were significantly correlated between myobundle and EV populations (p < 0.05); 15 of these miRs were strongly positively correlated (Pearson R > 0.9, R2 > 0.99) whereas 3 miRs were strongly negatively correlated (Pearson R < -0.9, R2 > 0.99). In the CLFS treatment condition, we identified 5 miRs as significantly correlated (p < 0.05), 3 miRs having strongly positively correlated (R > 0.9) and 2 miRs strongly negatively correlated (R < -0.9, R2 > 0.99). Following IHFS stimulation, we identified 2 miRs, both were strongly negatively correlated between the myobundle and EVs (R < -0.9, R2 > 0.99, p < 0.05).
Hierarchical clustering and overrepresentation analysis
Additionally, hierarchical clustering and over representation analyses were performed on the 152 miRs that met the QLF-test significance threshold. These 152 miR clustered by stimulation condition (CLFS and IHFS) and source (EV and myobundle). To further examine these relationships, we performed clustering of the 15 most highly expressed miRs in myobundle compared with EVs and the 15 most highly expressed in EVs compared with myobundles. Notably, these subgroup analyses also clustered by stimulation condition (CLFS and IHFS) and source (EV and myobundle) (Figures 3B,C). These results show that miR responding to e-stim, clustered together consistent with a similarity of these treatment conditions.
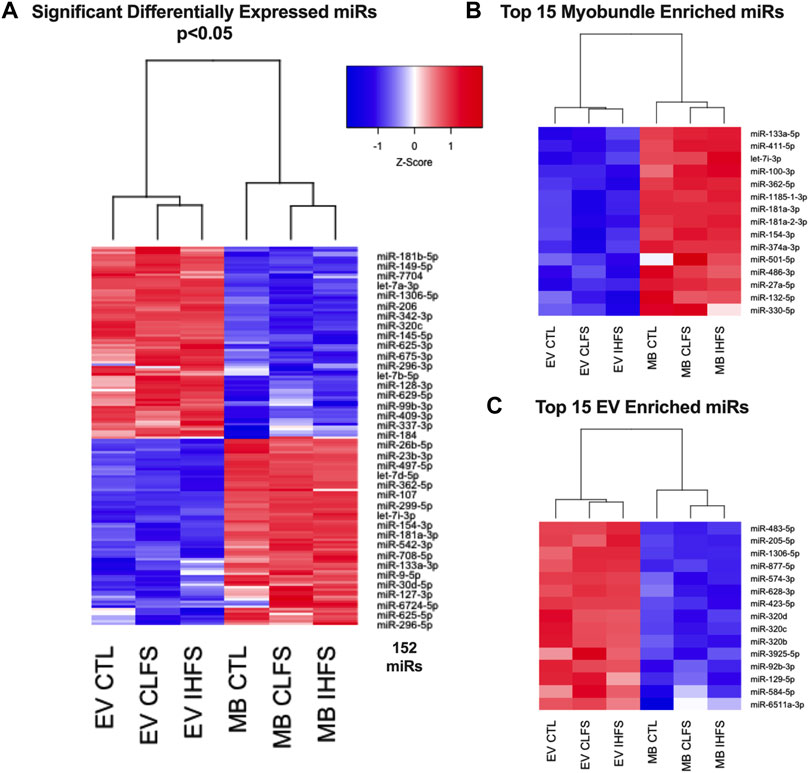
FIGURE 3. Relationships of Differentially Expressed miRs. Abbreviations: EV, extracellular vesicles; MB, myobundle; CTL, control; CLFS, chronic low frequency stimulation; IHFS, intermittent high frequency stimulation. Legend: data for panels (A–C) are presented as mean Z-Score with nodes depicting relationships between population and treatment.
Over representation analysis was performed using the experimentally validated miRNA Enrichment Analysis and Annotation Tool (miEAA) (Kern et al., 2020); top pathways were identified by the number of observed miRs within the pathway. Using the aforementioned 152 miRs and the raw data set prior to filtering (n = 991) as the reference data set, we identified 286 KEGG pathways that were overrepresented in our significant miR set (p < 0.05, Supplementary Table S2). The top 10 KEGG pathways can be found in Figure 4; notably, the well documented muscle pathways included in the top 10 were: PI3K/AKT signaling, FoxO signaling, MAPK signaling, and Focal Adhesion pathways (p < 0.05). Notably, these miRs were expressed in both the myobundle and EVs, with ∼50% of identified miRs being more highly expressed in the myobundle in all treatment conditions (Supplementary Table S1). Because these miRs were expressed in both populations, it is plausible that pathways identified herein can be regulated locally through muscle derived miRs and systemically via miRs carried by EVs.
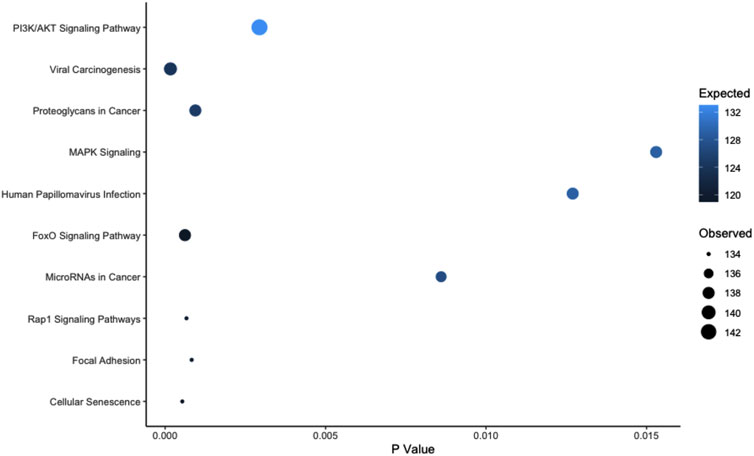
FIGURE 4. Top 10 Overrepresented KEGG Pathways Based on Observed miRs. Abbreviations: PI3K, phosphoinositide 3-kinase; AKT, protein kinase B; MAPK, mitogen activated protein kinase; FoxO, forkhead box, Rap-1, ras-related protein 1. Legend: pathways are presented by dot size (observed miR count) and color (expected miR count) and plotted in order based on the observed count.
Myobundle differential miR expression between treatments
To evaluate the myobundle miR response to exercise-mimetic conditions, we compared CLFS to CTL, IHFS to CTL, and IHFS to CLFS. Overall, we identified 8 miRs (miR-6724-5p, -499a-5p, -126-5p, -126-3p, -487b-3p, -543, -330-5p, and -542-3p) that met the significance threshold (p < 0.05; Figure 5A). CLFS compared to CTL yielded 18 differentially expressed miRs (p < 0.05; Figure 5C) with 15 miRs enriched in the CLFS treatment compared to 3 enriched in CTL. IHFS compared to CTL yielded 9 differentially expressed miRs (p < 0.05; Figure 5D) with 5 miRs enriched in the IHFS treatment compared to 4 miRs enriched in the CTL treatment. IHFS compared to CLFS, yielded 7 differentially expressed miRs (p < 0.05; Figure 5E) with 6 miRs enriched in the CLFS treatment.
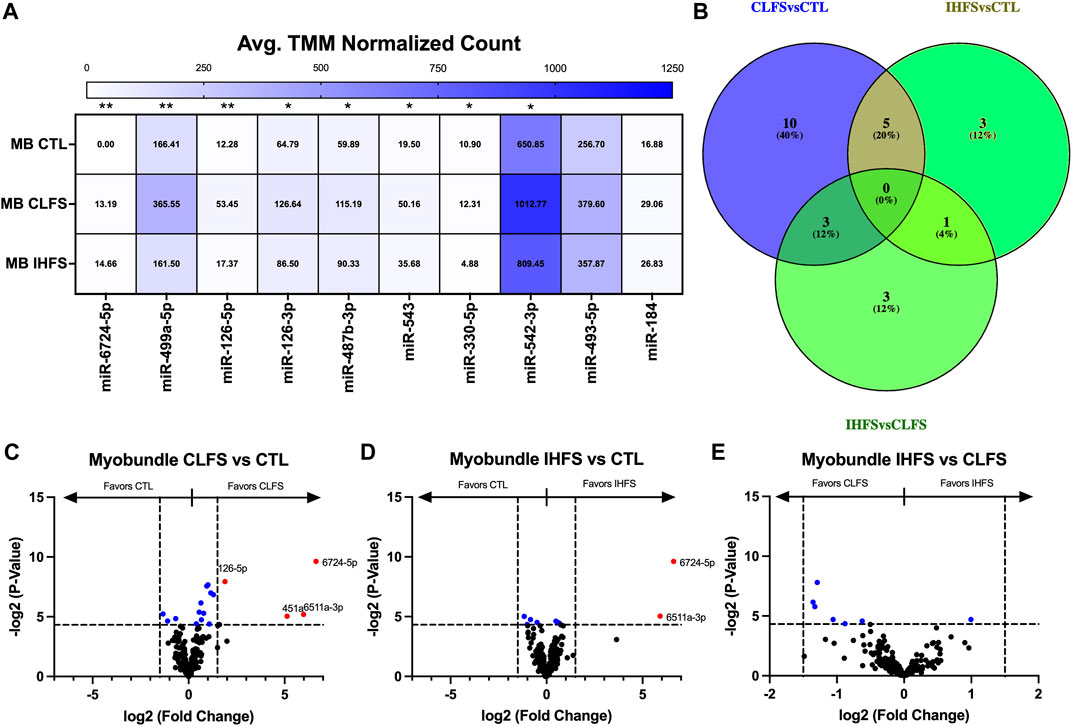
FIGURE 5. Differential Myobundle miR Expression Between Treatments. Abbreviations: EV, extracellular vesicles; MB, myobundle; CTL, control; CLFS, chronic low frequency stimulation; IHFS, intermittent high frequency stimulation. Legend: data for Panel (A) is presented as mean normalized count. Panel (B) represents overlap of miRs for analyses completed. Panels (C–E) are volcano plots with blue data points depicting p < 0.05 and red data points depicting p < 0.05 and logFC of >1.5 or < -1.5.
Although none of these differentially expressed miRs were shared across all treatment conditions (Figure 5B), 5 miRs were differentially expressed in common in the CLFS vs. CTL and IHFS vs. CTL treatments suggesting that these may be exercise responsive miRs.
EV differential miR expression between treatments
To evaluate the EV miR response to exercise-mimetic conditions, we compared all three treatment groups in addition to each pair of conditions (CLFS to CTL, IHFS to CTL, and IHFS to CLFS). Across all treatment groups, we identified miR-345-5p as differentially expressed (p = 0.011, Figure 6A). CLFS compared to CTL yielded one differentially expressed miR, miR-483-3p, that was higher in CLFS (p = 0.032, Figure 6C). We identified two differentially expressed miRs for IHFS vs. CTL, miR-345-5p (higher in IHFS, p = 0.004; Figure 6D) and miR-195-5p (higher in CTL, p = 0.030; Figure 6D). There were no differentially expressed miRs identified for IHFS compared to CLFS (p > 0.05, Figure 6E). Of interest, there was no overlap identified for these comparisons (Figure 6B). We take this to mean that specific stimulation conditions (simulating exercise in vivo) may modulate specific miRs.
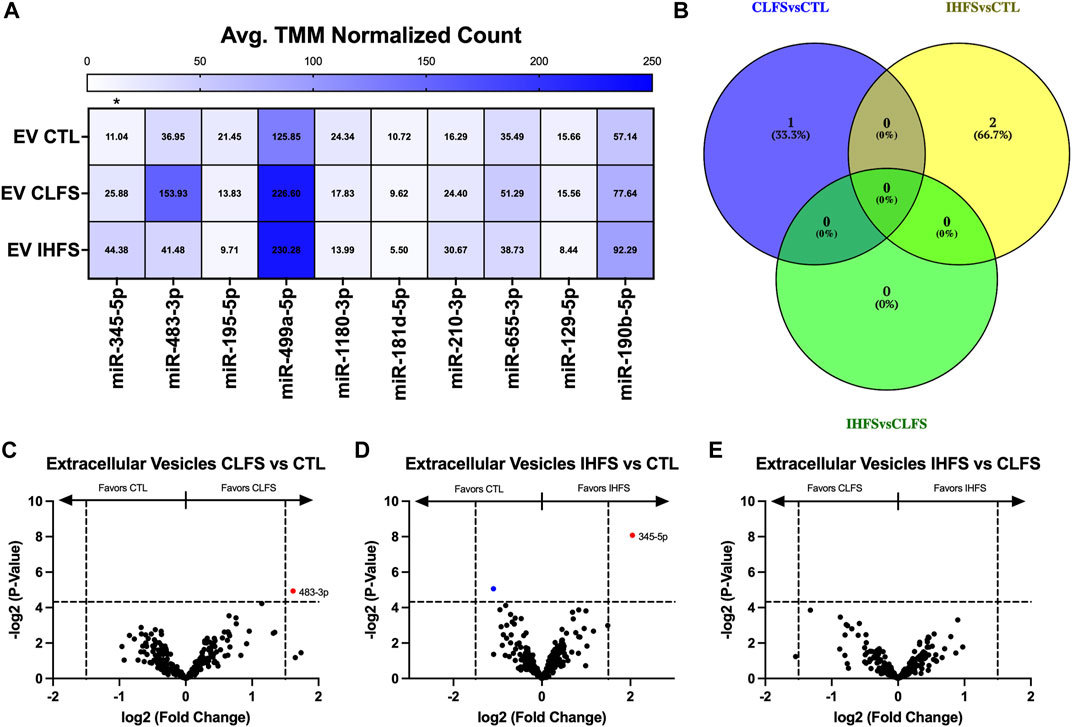
FIGURE 6. Differential Extracellular Vesicle miR Expression Between Treatments. Abbreviations: EV, extracellular vesicles; MB, myobundle; CTL, control; CLFS, chronic low frequency stimulation; IHFS, intermittent high frequency stimulation. Legend: data for Panel (A) is presented as mean normalized count. Panel (B) represents overlap of miRs for analyses completed. Panels (C–E) are volcano plots with blue data points depicting p < 0.05 and red data points depicting p < 0.05 and logFC of >1.5 or < -1.5.
Identification of miRs preferentially expressed in myobundles or EVs
It should be noted that the default filtering algorithm in the edgeR package seeks to remove low expressors (generally 0.5 counts when expressed as counts per million) if the gene cannot be expressed in all samples for any condition (Chen et al., 2016). This may have unintended consequences of eliminating extreme samples of preferentially expressed miRs (i.e., miRs exclusively expressed in EVs or myobundles). For this reason, we evaluated the previously filtered data sets (filtered to remove low expressors) for overlap to identify miRs whose expression was preferentially enriched in myobundles or EVs (Figure 7A). Overall, 27 miRs were preferentially expressed in myobundles (Figure 7B) while 21 miRs were preferentially expressed in EVs (Figure 7C). Excitingly, this analysis revealed 5 miRs with extreme expression preference (p < 0.05) for the myobundle (miRs-27a-5p, -486-3p, -100-3p, -330-5p, and -181-3p; Supplementary Figures S3A–E). Additionally, we identified miR-454-5p as having extreme expression preference for EVs (p = 0.039; Supplementary Figure S4A) and 3 additional miRs as trending toward extreme expression preference (p < 0.1) for EVs (miRs-887-5p, -7706, and 1226-3p; Supplementary Figures S4B–D).
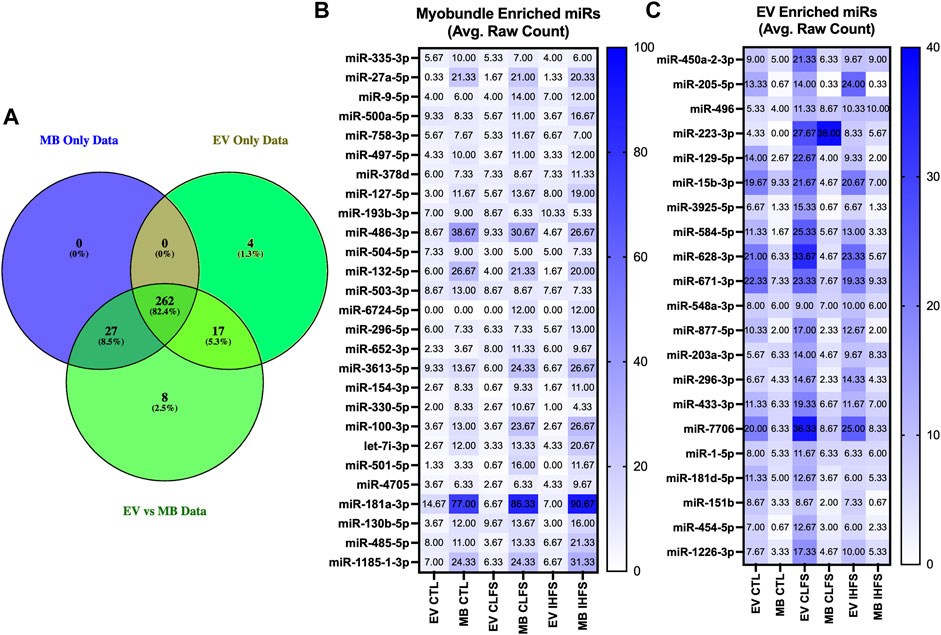
FIGURE 7. Myobundle and Extracellular Vesicle Enriched miRs. Abbreviations: EV, extracellular vesicles; MB, myobundle; CTL, control; CLFS, chronic low frequency stimulation; IHFS, intermittent high frequency stimulation. Legend: Panel (A) represents the overlap of the overall data set used for between population within treatment analyses and the data sets used for the individual myobundle and EV analyses between treatments. Panel (B) represents n = 27 miRs enriched within the myobundle. Panel c represents n = 21 miRs enriched in EVs. Data for panels (B,C) are presented as mean raw count.
Comparison of normalization methods
The processing and normalization of data can impact differential expression results. Following data processing using BOWTIE, we elected to normalize our data using the trimmed mean of M-values (TMM) within the edgeR package. Importantly, we compared this methodology to two other commonly used methods: transcripts per million (TPM) and DESEQ2. While we did not directly compare these outputs to evaluate biological and technical variability, we did perform overlap analyses on miRs identified as differentially expressed from each package using the myobundle data sets comparing CLFS to CTL. Using a p-value threshold of p < 0.05 for all normalized data sets, there was a notable difference in the quantity of differentially expressed miRs identified by each method: there were 18, 20 and 54 miRs identified as differentially expressed by TMM-edgeR, VST-DESEQ2, and TPM, respectively; 11 miR overlapped among all these data sets, with an additional 6 miR overlap between the TMM-edgeR and TPM data sets (Supplementary Figure S5A). Increasing the threshold stringency for the TPM data set to p < 0.01 resulted in a reduction in the number of differentially expressed miRs from 54 to 16; compared to the other two data sets, this yielded an overlap of 7 differentially expressed miRs between all data sets, 10 miRs with TMM-edgeR and 8 miRs with VST-DESEQ2 (Supplementary Figure S5B). These results demonstrate that overall, TPM identifies a larger number of differentially expressed miRs; when constrained to a more stringent p < 0.01 threshold, TPM yields a similar number of differentially expressed miRs compared with DESEQ2 and edgeR set to a p < 0.05 threshold. This suggests study design, analysis models, and the strengths and shortfalls of different bioinformatic approaches should be considered prior to selection of a bioinformatic approach.
Discussion
While previous studies have assessed the effects and expression of miRs in tissue-engineered muscle (Rhim et al., 2010; Koning et al., 2012; Cheng et al., 2016), to our knowledge, this study is the first to apply miRNAseq to examine differential miR expression in myobundles subjected to two types of e-stim protocols, and the EVs that these tissues generate. Chief findings include identifying 152 miRs that are differentially expressed between myobundles and EVs with 3 and 2 miRs identified as differentially expressed between myobundles and EVs within the CLFS and IHFS treatment conditions, respectively. Furthermore, within the myobundles, CLFS and IHFS treatment resulted in 18 and 9 differentially expressed miRs compared to CTL, respectively, while within EVs, we only found 1 differentially expressed miR for CLFS and 2 for IHFS compared to CTL. A summary of findings for contrast analyses can be found in Table 2.
Skeletal muscle exports various myokines and EVs that exert effects on other target tissues (Pedersen and Febbraio, 2008; Pratesi et al., 2013; Rome et al., 2019; Darkwah et al., 2021). In our study, differentially expressed miRs in myobundles vs EVs were miR-543, -487b-3p, and -6511a-3p for CLFS and miR-543 and -6511a-3p for IHFS treatment. Notably, all these miRs were enriched in the myobundles compared with EVs. Previous work has shown that miR-543 plays a role in proliferation of C2C12 cells via targeting Krüppel-like factor 6 (KLF-6), which is a suppressor of multiple tumor cells (Lang et al., 2013; Kang et al., 2020). Interestingly, although miR-487b-3p was not related to any of the pathways identified in this study, miR-6511a-3p was identified in the viral carcinogenesis, proteoglycans in cancer, MAPK signaling, microRNAs in cancer, Rap1 signaling, and focal adhesion pathways, and miR-543 was found in all the top 10 overrepresented pathways. Notably, miRs-6511a-3p and -487b-3p have yet to be investigated in the context of human skeletal muscle.
Using the 152 miRs identified through differential expression analysis of myobundles vs EVs, we identified 286 enriched KEGG pathways in this study. Several of these pathways are known to be important in the regulation of skeletal muscle, including PI3K/AKT signaling, MAPK signaling, and focal adhesion pathways. In addition to being important for regulation of skeletal muscle, the previously mentioned pathways as well as others identified in our analysis have also been identified as critical components in myogenic differentiation of pluripotent stem cells (Fei et al., 2021). Focal adhesion kinase (FAK), which localizes around focal adhesion sites, can be activated through mechanotransduction and through growth factors (Quach et al., 2009; Graham et al., 2015) and has effects that regulate cell growth, differentiation, migration, and survival (Schaller, 2001). Mitogen activated protein kinase (MAPK) pathway is well characterized and known to play a vital role in mechanotransduction. As reviewed by Zhang and Liu, MAPK can be activated through multiple stimuli and plays roles in proliferation, differentiation, development, inflammation, apoptosis, and more (Zhang and Liu, 2002). The PI3K/AKT axis is a well characterized pathway in skeletal muscle. Interestingly, Briata and colleagues found that during myogenesis, this pathway is responsible for myomiR maturation in the presence of KH-type splicing regulatory protein (KSRP) through a switch between two distinct KSRP functions leading to activation of miR maturation through interaction at the terminal loop of select miRs or promoting the decay of myogenin (Briata et al., 2011; Briata et al., 2012). This suggests that select understudied miRs, identified in our study (e.g., miRs-6511a-3p and -487b-3p), potentially regulate pathways that are important throughout the muscle cell cycle.
Within EVs, we identified miR-345-5p as differentially expressed comparing IHFS to CTL. Previously, miR-345-5p was shown to be upregulated in rectus abdominis muscle biopsies of cachectic individuals, which was linked to regulation of the ubiquitin-proteasome pathway (Narasimhan et al., 2017; Yedigaryan and Sampaolesi, 2021). Additionally, comparing CLFS to CTL within EVs, we identified miR-483-3p as differentially expressed, which has previously been linked to inhibition of muscle cell proliferation and differentiation through negative regulation of the PI3K/AKT pathway (Song et al., 2019). In a recent review from Rome and colleagues, miRs-451, -6239, -6240, -6236, -144, -223, -5112, 3062, -142a, -2137, and -720 were noted as having higher expression in muscle-derived EVs than in muscle cells (Rome et al., 2019); among these, only miRs-451 and -223 were included in our analyses. In our data, miRs-451 and -223 were not identified as differentially expressed between myobundles and EVs, however, normalized counts for both miRs were higher in EVs compared to myobundles for CTL and in myobundles compared to EVs for CLFS and IHFS treatments.
In general, known muscle specific miRs (termed myomiRs) changed minimally in our study. For instance, miR-133a-5p (a myomiR related to regulation and function of skeletal muscle) increased minimally (log2FC = 0.228; p = 0.314) in myobundles following CLFS, with a similar trend following IHFS (log2FC = 0.389; p = 0.180). Additionally, other notable muscle-specific miRs, such as miRs-1, -181a, -23a, -27a, and -206, changed minimally in expression. It is worth noting that many of the prior studies investigating changes in miR expression used RT-qPCR or, in some cases, custom microRNA arrays. Notably, these methods–while very accurate and useful–limit interpretation due to the small quantity of genes that are generally interrogated. Previously, in vivo models using mouse muscle (van Rooij et al., 2009; Liu et al., 2011; Chemello et al., 2019; Bjorkman et al., 2020; Vechetti et al., 2021a) and in vitro models using transfection in C2C12s and human primary cells (Cheng et al., 2016; Chemello et al., 2019), have reported differential regulation of myomiRs-1, -133a, -206, -208b, and -27a. In some human studies evaluating various forms of training stimuli, miRs-1, -133a and b, -181a, -29 b were upregulated (Russell et al., 2013) while other stimuli resulted in downregulation of these miRs and others such as miRs-378 and -486 (Drummond et al., 2008; Nielsen et al., 2010; Mueller et al., 2011; Ringholm et al., 2011; Fyfe et al., 2016). While minimal changes in these commonly interrogated myomiRs in our study are hard to reconcile with the existing literature, it is possible that the myobundle, made using cells from paraspinal muscles (predominantly type I), respond differently to stimulation compared to the in vivo studies generally sampling from the vastus lateralis (∼50% type I and 50% type II fibers). Notably, previous work using three-dimensional culture models derived from the tibialis anterior and soleus muscles in rodents has shown different physiological responses to electrical stimulation (Huang et al., 2006). Additionally, although CTL myobundles did not receive electrical stimulation, these tissues do contract spontaneously at <15% of peak force generation, which may contribute to EV secretion and attenuate differences in miR expression with e-stim.
The use of TMM for analysis of miRNAseq data, as used here, has been shown to be effective at reducing sample variance and generating more accurate downstream analytical results (Tam et al., 2015). Prior to normalization and differential expression analysis, it is generally recommended to remove low expressors through filtering because in general, removal of low expressors increases sensitivity in downstream analyses (i.e., differential expression) and does not meaningfully affect significance of other genes within the data set (Bourgon et al., 2010; Sha et al., 2015). However, this may have the unintended consequence of eliminating informative missingness (highly skewed expression in one group and relatively low or undetectable expression another group). For this reason, we recommend a post-hoc evaluation of raw counts of miR excluded through filtering to evaluate for the occurrence of this circumstance.
We also sought to compare TMM outputs with two other commonly used normalization strategies, VST-DESEQ2 and TPM. We conclude that VST-DESEQ2 and TMM-edgeR yield similar outputs, whereas TPM normalization appears to identify a larger number of differentially expressed targets from the same dataset. However, when the TPM threshold is set at p < 0.01, the output results are similar to TMM-edgeR and VST-DESEQ2. It is worthwhile to note that TPM accounts for sequencing depth and gene length but does not account for differences in library composition (Corchete et al., 2020; Zhao et al., 2021). It has been posited that normalizing to sequencing depth alone is insufficient for miRNAseq analysis (Garmire and Subramaniam, 2012; Dillies et al., 2013). Conversely, normalization using TMM-edgeR and VST-DESEQ2 overcomes differences in library size and quantifications through use of scaling factors (Dillies et al., 2013). Taken together, the current information suggests that depending on study design, TMM-edgeR and VST-DESEQ2 are more suited to miRNAseq data because they account for variables such as library size and library composition whereas TPM may not be suitable for miRNAseq data because library composition is not accounted for.
As with many studies utilizing human samples, we were limited by sample size. Contamination of the isolated EV pool by other extracellular particles such as protein and lipids is a known limitation of polymer based precipitation (PBP) isolation methods (Zarovni et al., 2015; Li et al., 2017; Brennan et al., 2020). In the context of miR, PBP has been shown to yield higher recovery of EV derived miRs (Chung et al., 2020). As discussed by Zhang and colleagues (Zhang et al., 2022) there is no established “gold standard” for EV isolation applicable to all subsequent analyses, therefore we chose PBP because of higher EV yields given our specific interest of profiling miRs from the myobundle and myobundle derived EVs. Additionally, miRNAseq is still a relatively new technique with numerous methods available for data processing. Following data processing but prior to filtering out low expressors, 991 miRs met the processing criterion, a low value compared to other work evaluating small RNA sequencing expression in native skeletal muscle. While we used an approach that has been shown to minimize variation and generate accurate differential expression data (Tam et al., 2015), many other approaches and available analysis packages could have been used herein; however, there is no current standard practice for analysis of miRNAseq data.
Conclusion
While miR expression and secreted EVs have been previously analyzed in skeletal muscle in vivo, this is the first study to do this analysis for engineered human skeletal muscle tissues devoid of confounding factors from the systemic environment or the multicellular nature of native muscle tissue. The miRs identified herein have been implicated in numerous pathways related to health, disease, metabolism, and regulation and development of skeletal muscle. For instance, we identified miR-6511a-3p as responsive to both CLFS and IHFS stimulation while also noting its presence in several pathways related to regulation of muscle (i.e., MAPK, Rap1, and focal adhesion signaling) however, miR-6511a-3p has yet to be investigated in human muscle tissue. These data provide novel miR targets for future research to elucidate the effects of exercise on muscle and muscle signals transduced by their EVs and are relevant to identifying mechanisms by which muscle regulates endogenous gene expression and how muscle communicates with other tissue.
Data availability statement
Raw data files were deposited to the National Institutes of Health (NIH) Gene Expression Omnibus (GEO) repository and can be found online (https://www.ncbi.nlm.nih.gov/geo/) Accession ID: GSE203157. All relevant data pertaining to EV isolation methods has been uploaded to the EV-Track knowledge base (EV-Track ID: EV220309) (Van Deun et al., 2017).
Ethics statement
The studies involving human participants were reviewed and approved by Duke University Institutional Review Board. Written informed consent to participate in this study was provided by the participants’ legal guardian/next of kin.
Author contributions
CV, NB, GT, AK, and VK devised project aims and outcomes, and all authors provided critical insight. AK performed tissue culture, XZ performed EV isolation, AK and XZ performed RNA isolation, DC aligned data, CV performed bioinformatic analyses. GT, NB, XZ, and VK provided resources for project outcomes. CV and VK primarily drafted the manuscript and all authors provided critical edits and approved the manuscript for submission.
Funding
Funding For this project was provided by the National Institutes of Health (grants R01AG054840, R01AG070146, R01AR070543, UH3TR002142, and P30AG028716).
Acknowledgments
Figure 1 was generated using BioRender.com. The authors would like to thank Dr. Nicolas Devos, and the Duke Center for Genomics and Computational Biology for performing the miRNAseq.
Conflict of interest
The authors declare that the research was conducted in the absence of any commercial or financial relationships that could be construed as a potential conflict of interest.
Publisher’s note
All claims expressed in this article are solely those of the authors and do not necessarily represent those of their affiliated organizations, or those of the publisher, the editors and the reviewers. Any product that may be evaluated in this article, or claim that may be made by its manufacturer, is not guaranteed or endorsed by the publisher.
Supplementary material
The Supplementary Material for this article can be found online at: https://www.frontiersin.org/articles/10.3389/fphys.2022.937899/full#supplementary-material
References
Backes C., Leidinger P., Keller A., Hart M., Meyer T., Meese E., et al. (2014). Blood born miRNAs signatures that can serve as disease specific biomarkers are not significantly affected by overall fitness and exercise. PLoS One 9, e102183. doi:10.1371/journal.pone.0102183
Bjorkman K. K., Guess M. G., Harrison B. C., Polmear M. M., Peter A. K., Leinwand L. A. (2020). miR-206 enforces a slow muscle phenotype. J. Cell Sci. 133, jcs243162. doi:10.1242/jcs.243162
Bourgon R., Gentleman R., Huber W. (2010). Independent filtering increases detection power for high-throughput experiments. Proc. Natl. Acad. Sci. U. S. A. 107, 9546–9551. doi:10.1073/pnas.0914005107
Brennan K., Martin K., Fitzgerald S. P., O’Sullivan J., Wu Y., Blanco A., et al. (2020). A comparison of methods for the isolation and separation of extracellular vesicles from protein and lipid particles in human serum. Sci. Rep. 10, 1039. doi:10.1038/s41598-020-57497-7
Briata P., Chen C. Y., Giovarelli M., Pasero M., Trabucchi M., Ramos A., et al. (2011). KSRP, many functions for a single protein. Front. Biosci. 16, 1787–1796. doi:10.2741/3821
Briata P., Lin W. J., Giovarelli M., Pasero M., Chou C. F., Trabucchi M., et al. (2012). PI3K/AKT signaling determines a dynamic switch between distinct KSRP functions favoring skeletal myogenesis. Cell Death Differ. 19, 478–487. doi:10.1038/cdd.2011.117
Chemello F., Grespi F., Zulian A., Cancellara P., Hebert-Chatelain E., Martini P., et al. (2019). Transcriptomic analysis of single isolated myofibers identifies miR-27a-3p and miR-142-3p as regulators of metabolism in skeletal muscle. Cell Rep. 26, 3784–3797. doi:10.1016/j.celrep.2019.02.105
Chen X., Wang K., Chen J., Guo J., Yin Y., Cai X., et al. (2009). In vitro evidence suggests that miR-133a-mediated regulation of uncoupling protein 2 (UCP2) is an indispensable step in myogenic differentiation. J. Biol. Chem. 284, 5362–5369. doi:10.1074/jbc.M807523200
Chen Y., Lun A. T., Smyth G. K. (2016). From reads to genes to pathways: Differential expression analysis of RNA-seq experiments using rsubread and the edgeR quasi-likelihood pipeline. F1000Res. 5, 1438. doi:10.12688/f1000research.8987.1
Chen Z., Li B., Zhan R. Z., Rao L., Bursac N. (2021). Exercise mimetics and JAK inhibition attenuate IFN-γ-induced wasting in engineered human skeletal muscle. Sci. Adv. 7, eabd9502. doi:10.1126/sciadv.abd9502
Cheng C. S., Ran L., Bursac N., Kraus W. E., Truskey G. A. (2016). Cell density and joint microRNA-133a and microRNA-696 inhibition enhance differentiation and contractile function of engineered human skeletal muscle tissues. Tissue Eng. Part A 22, 573–583. doi:10.1089/ten.TEA.2015.0359
Chevillet J. R., Kang Q., Ruf I. K., Briggs H. A., Vojtech L. N., Hughes S. M., et al. (2014). Quantitative and stoichiometric analysis of the microRNA content of exosomes. Proc. Natl. Acad. Sci. U. S. A. 111, 14888–14893. doi:10.1073/pnas.1408301111
Chilton W. L., Marques F. Z., West J., Kannourakis G., Berzins S. P., O'Brien B. J., et al. (2014). Acute exercise leads to regulation of telomere-associated genes and microRNA expression in immune cells. PLoS One 9, e92088. doi:10.1371/journal.pone.0092088
Chung K. Y., Quek J. M., Neo S. H., Too H. P. (2020). Polymer-based precipitation of extracellular vesicular miRNAs from serum improve gastric cancer miRNA biomarker performance. J. Mol. Diagn. 22, 610–618. doi:10.1016/j.jmoldx.2020.01.016
Corchete L. A., Rojas E. A., Alonso-López D., DE Las Rivas J., Gutiérrez N. C., Burguillo F. J. (2020). Systematic comparison and assessment of RNA-seq procedures for gene expression quantitative analysis. Sci. Rep. 10, 19737. doi:10.1038/s41598-020-76881-x
Cui S. F., Li W., Niu J., Zhang C. Y., Chen X., Ma J. Z. (2015). Acute responses of circulating microRNAs to low-volume sprint interval cycling. Front. Physiol. 6, 311. doi:10.3389/fphys.2015.00311
Cui S. F., Wang C., Yin X., Tian D., Lu Q. J., Zhang C. Y., et al. (2016). Similar responses of circulating MicroRNAs to acute high-intensity interval exercise and vigorous-intensity continuous exercise. Front. Physiol. 7, 102. doi:10.3389/fphys.2016.00102
Dillies M. A., Rau A., Aubert J., Hennequet-Antier C., Jeanmougin M., Servant N., et al. (2013). A comprehensive evaluation of normalization methods for Illumina high-throughput RNA sequencing data analysis. Brief. Bioinform. 14, 671–683. doi:10.1093/bib/bbs046
Darkwah S., Park E. J., Myint P. K., Ito A., Appiah M. G., Obeng G., et al. (2021). Potential roles of muscle-derived extracellular vesicles in remodeling cellular microenvironment: Proposed implications of the exercise-induced myokine, irisin. Front. Cell Dev. Biol. 9, 634853. doi:10.3389/fcell.2021.634853
De Jong O. G., Van Balkom B. W., Schiffelers R. M., Bouten C. V., Verhaar M. C. (2014). Extracellular vesicles: Potential roles in regenerative medicine. Front. Immunol. 5, 608. doi:10.3389/fimmu.2014.00608
Denham J., Spencer S. J. (2020). Emerging roles of extracellular vesicles in the intercellular communication for exercise-induced adaptations. Am. J. Physiol. Endocrinol. Metab. 319, E320-e329. doi:10.1152/ajpendo.00215.2020
Denli A. M., Tops B. B., Plasterk R. H., Ketting R. F., Hannon G. J. (2004). Processing of primary microRNAs by the Microprocessor complex. Nature 432, 231–235. doi:10.1038/nature03049
Dey B. K., Gagan J., Dutta A. (2011). miR-206 and -486 induce myoblast differentiation by downregulating Pax7. Mol. Cell. Biol. 31, 203–214. doi:10.1128/MCB.01009-10
Drummond M. J., Mccarthy J. J., Fry C. S., Esser K. A., Rasmussen B. B. (2008). Aging differentially affects human skeletal muscle microRNA expression at rest and after an anabolic stimulus of resistance exercise and essential amino acids. Am. J. Physiol. Endocrinol. Metab. 295, E1333–E1340. doi:10.1152/ajpendo.90562.2008
Estrada A. L., Valenti Z. J., Hehn G., Amorese A. J., Williams N. S., Balestrieri N. P., et al. (2022). Extracellular vesicle secretion is tissue-dependent ex vivo and skeletal muscle myofiber extracellular vesicles reach the circulation in vivo. Am. J. Physiol. Cell Physiol. 322, C246–c259. doi:10.1152/ajpcell.00580.2020
Fei W., Liu M., Zhang Y., Cao S., Wang X., Xie B., et al. (2021). Identification of key pathways and hub genes in the myogenic differentiation of pluripotent stem cell: A bioinformatics and experimental study. J. Orthop. Surg. Res. 16, 4. doi:10.1186/s13018-020-01979-x
Forterre A., Jalabert A., Chikh K., Pesenti S., Euthine V., Granjon A., et al. (2014). Myotube-derived exosomal miRNAs downregulate Sirtuin1 in myoblasts during muscle cell differentiation. Cell Cycle 13, 78–89. doi:10.4161/cc.26808
Frühbeis C., Helmig S., Tug S., Simon P., Krämer-Albers E. M. (2015). Physical exercise induces rapid release of small extracellular vesicles into the circulation. J. Extracell. Vesicles 4, 28239. doi:10.3402/jev.v4.28239
Fyfe J. J., Bishop D. J., Zacharewicz E., Russell A. P., Stepto N. K. (2016). Concurrent exercise incorporating high-intensity interval or continuous training modulates mTORC1 signaling and microRNA expression in human skeletal muscle. Am. J. Physiol. Regul. Integr. Comp. Physiol. 310, R1297–R1311. doi:10.1152/ajpregu.00479.2015
Garmire L. X., Subramaniam S. (2012). Evaluation of normalization methods in mammalian microRNA-Seq data. Rna 18, 1279–1288. doi:10.1261/rna.030916.111
Graham Z. A., Gallagher P. M., Cardozo C. P. (2015). Focal adhesion kinase and its role in skeletal muscle. J. Muscle Res. Cell Motil. 36, 305–315. doi:10.1007/s10974-015-9415-3
Guescini M., Canonico B., Lucertini F., Maggio S., Annibalini G., Barbieri E., et al. (2015). Muscle releases alpha-sarcoglycan positive extracellular vesicles carrying miRNAs in the bloodstream. PLoS One 10, e0125094. doi:10.1371/journal.pone.0125094
Guescini M., Guidolin D., Vallorani L., Casadei L., Gioacchini A. M., Tibollo P., et al. (2010). C2C12 myoblasts release micro-vesicles containing mtDNA and proteins involved in signal transduction. Exp. Cell Res. 316, 1977–1984. doi:10.1016/j.yexcr.2010.04.006
Guo B., Zhang Z. K., Liang C., Li J., Liu J., Lu A., et al. (2017). Molecular communication from skeletal muscle to bone: A review for muscle-derived myokines regulating bone metabolism. Calcif. Tissue Int. 100, 184–192. doi:10.1007/s00223-016-0209-4
Helwa I., Cai J., Drewry M. D., Zimmerman A., Dinkins M. B., Khaled M. L., et al. (2017). A comparative study of serum exosome isolation using differential ultracentrifugation and three commercial reagents. PLoS One 12, e0170628. doi:10.1371/journal.pone.0170628
Huang W., Guo L., Zhao M., Zhang D., Xu H., Nie Q. (2019). The inhibition on MDFIC and PI3K/AKT pathway caused by miR-146b-3p triggers suppression of myoblast proliferation and differentiation and promotion of apoptosis. Cells 8, 656. doi:10.3390/cells8070656
Huang Y. C., Dennis R. G., Baar K. (2006). Cultured slow vs. fast skeletal muscle cells differ in physiology and responsiveness to stimulation. Am. J. Physiol. Cell Physiol. 291, C11–C17. doi:10.1152/ajpcell.00366.2005
Jacques M., Hiam D., Craig J., Barrès R., Eynon N., Voisin S. (2019). Epigenetic changes in healthy human skeletal muscle following exercise- a systematic review. Epigenetics 14, 633–648. doi:10.1080/15592294.2019.1614416
Johnsen K. B., Gudbergsson J. M., Andresen T. L., Simonsen J. B. (2019). What is the blood concentration of extracellular vesicles? Implications for the use of extracellular vesicles as blood-borne biomarkers of cancer. Biochim. Biophys. Acta. Rev. Cancer 1871, 109–116. doi:10.1016/j.bbcan.2018.11.006
Kang T., Xing W., XI Y., Chen K., Zhan M., Tang X., et al. (2020). MiR-543 regulates myoblast proliferation and differentiation of C2C12 cells by targeting KLF6. J. Cell. Biochem. 2020, 4827–4837. doi:10.1002/jcb.29710
Kern F., Fehlmann T., Solomon J., Schwed L., Grammes N., Backes C., et al. (2020). miEAA 2.0: integrating multi-species microRNA enrichment analysis and workflow management systems. Nucleic Acids Res. 48, W521-w528. doi:10.1093/nar/gkaa309
Khodabukus A., Baar K. (2009). Regulating fibrinolysis to engineer skeletal muscle from the C2C12 cell line. Tissue Eng. Part C Methods 15, 501–511. doi:10.1089/ten.TEC.2008.0286
Khodabukus A., Baehr L. M., Bodine S. C., Baar K. (2015). Role of contraction duration in inducing fast-to-slow contractile and metabolic protein and functional changes in engineered muscle. J. Cell. Physiol. 230, 2489–2497. doi:10.1002/jcp.24985
Khodabukus A., Kaza A., Wang J., Prabhu N., Goldstein R., Vaidya V. S., et al. (2020). Tissue-engineered human myobundle system as a platform for evaluation of skeletal muscle injury biomarkers. Toxicol. Sci. 176, 124–136. doi:10.1093/toxsci/kfaa049
Khodabukus A., Madden L., Prabhu N. K., Koves T. R., Jackman C. P., Muoio D. M., et al. (2019). Electrical stimulation increases hypertrophy and metabolic flux in tissue-engineered human skeletal muscle. Biomaterials 198, 259–269. doi:10.1016/j.biomaterials.2018.08.058
Khodabukus A. (2021). Tissue-engineered skeletal muscle models to study muscle function, plasticity, and disease. Front. Physiol. 12, 619710. doi:10.3389/fphys.2021.619710
Koning M., Werker P. M., Van Der Schaft D. W., Bank R. A., Harmsen M. C. (2012). MicroRNA-1 and microRNA-206 improve differentiation potential of human satellite cells: A novel approach for tissue engineering of skeletal muscle. Tissue Eng. Part A 18, 889–898. doi:10.1089/ten.TEA.2011.0191
Kozomara A., Griffiths-Jones S. (2014). miRBase: annotating high confidence microRNAs using deep sequencing data. Nucleic Acids Res. 42, D68–D73. doi:10.1093/nar/gkt1181
Kropp J., Degerny C., Morozova N., Pontis J., Harel-Bellan A., Polesskaya A. (2015). miR-98 delays skeletal muscle differentiation by down-regulating E2F5. Biochem. J. 466, 85–93. doi:10.1042/BJ20141175
Lacal I., Ventura R. (2018). Epigenetic inheritance: Concepts, mechanisms and perspectives. Front. Mol. Neurosci. 11, 292. doi:10.3389/fnmol.2018.00292
Lang U. E., Kocabayoglu P., Cheng G. Z., Ghiassi-Nejad Z., Muñoz U., Vetter D., et al. (2013). GSK3β phosphorylation of the KLF6 tumor suppressor promotes its transactivation of p21. Oncogene 32, 4557–4564. doi:10.1038/onc.2012.457
Langmead B., Trapnell C., Pop M., Salzberg S. L. (2009). Ultrafast and memory-efficient alignment of short DNA sequences to the human genome. Genome Biol. 10, R25. doi:10.1186/gb-2009-10-3-r25
Li P., Kaslan M., Lee S. H., Yao J., Gao Z. (2017). Progress in exosome isolation techniques. Theranostics 7, 789–804. doi:10.7150/thno.18133
Liu N., Bezprozvannaya S., Shelton J. M., Frisard M. I., Hulver M. W., Mcmillan R. P., et al. (2011). Mice lacking microRNA 133a develop dynamin 2–dependent centronuclear myopathy. J. Clin. Invest. 121, 3258–3268. doi:10.1172/JCI46267
Lozano-Velasco E., Vallejo D., Esteban F. J., Doherty C., Hernández-Torres F., Franco D., et al. (2015). A pitx2-MicroRNA pathway modulates cell proliferation in myoblasts and skeletal-muscle satellite cells and promotes their commitment to a myogenic cell fate. Mol. Cell. Biol. 35, 2892–2909. doi:10.1128/MCB.00536-15
Madden L., Juhas M., Kraus W. E., Truskey G. A., Bursac N. (2015). Bioengineered human myobundles mimic clinical responses of skeletal muscle to drugs. Elife 4, e04885. doi:10.7554/eLife.04885
Masi L. N., Serdan T. D., Levada-Pires A. C., Hatanaka E., Silveira L. D., Cury-Boaventura M. F., et al. (2016). Regulation of gene expression by exercise-related micrornas. Cell. Physiol. biochem. 39, 2381–2397. doi:10.1159/000452507
Mccarthy D. J., Chen Y., Smyth G. K. (2012). Differential expression analysis of multifactor RNA-Seq experiments with respect to biological variation. Nucleic Acids Res. 40, 4288–4297. doi:10.1093/nar/gks042
Motohashi N., Alexander M. S., Shimizu-Motohashi Y., Myers J. A., Kawahara G., Kunkel L. M. (2013). Regulation of IRS1/Akt insulin signaling by microRNA-128a during myogenesis. J. Cell Sci. 126, 2678–2691. doi:10.1242/jcs.119966
Mueller M., Breil F. A., Lurman G., Klossner S., Flück M., Billeter R., et al. (2011). Different molecular and structural adaptations with eccentric and conventional strength training in elderly men and women. Gerontology 57, 528–538. doi:10.1159/000323267
Mukherji S., Ebert M. S., Zheng G. X., Tsang J. S., Sharp P. A., Van Oudenaarden A. (2011). MicroRNAs can generate thresholds in target gene expression. Nat. Genet. 43, 854–859. doi:10.1038/ng.905
Narasimhan A., Ghosh S., Stretch C., Greiner R., Bathe O. F., Baracos V., et al. (2017). Small RNAome profiling from human skeletal muscle: Novel miRNAs and their targets associated with cancer cachexia. J. Cachexia Sarcopenia Muscle 8, 405–416. doi:10.1002/jcsm.12168
Nederveen J. P., Warnier G., Di Carlo A., Nilsson M. I., Tarnopolsky M. A. (2020). Extracellular vesicles and exosomes: Insights from exercise science. Front. Physiol. 11, 604274. doi:10.3389/fphys.2020.604274
Nielsen S., Scheele C., Yfanti C., Akerström T., Nielsen A. R., Pedersen B. K., et al. (2010). Muscle specific microRNAs are regulated by endurance exercise in human skeletal muscle. J. Physiol. 588, 4029–4037. doi:10.1113/jphysiol.2010.189860
Nikolić N., Bakke S. S., Kase E. T., Rudberg I., Flo Halle I., Rustan A. C., et al. (2012). Electrical pulse stimulation of cultured human skeletal muscle cells as an in vitro model of exercise. PLoS One 7, e33203. doi:10.1371/journal.pone.0033203
O'Brien J., Hayder H., Zayed Y., Peng C. (2018). Overview of MicroRNA biogenesis, mechanisms of actions, and circulation. Front. Endocrinol. 9, 402. doi:10.3389/fendo.2018.00402
Ogasawara R., Akimoto T., Umeno T., Sawada S., Hamaoka T., Fujita S. (2016). MicroRNA expression profiling in skeletal muscle reveals different regulatory patterns in high and low responders to resistance training. Physiol. Genomics 48, 320–324. doi:10.1152/physiolgenomics.00124.2015
Oliveros J. C. (2015). Venny. An interactive tool for comparing lists with Venn’s diagrams. Available: https://bioinfogp.cnb.csic.es/tools/venny/index.html.
Pedersen B. K., Febbraio M. A. (2008). Muscle as an endocrine organ: Focus on muscle-derived interleukin-6. Physiol. Rev. 88, 1379–1406. doi:10.1152/physrev.90100.2007
Peschansky V. J., Wahlestedt C. (2014). Non-coding RNAs as direct and indirect modulators of epigenetic regulation. Epigenetics 9, 3–12. doi:10.4161/epi.27473
Plomgaard P., Halban P. A., Bouzakri K. (2012). Bimodal impact of skeletal muscle on pancreatic β-cell function in health and disease. Diabetes Obes. Metab. 14 (3), 78–84. doi:10.1111/j.1463-1326.2012.01641.x
Pratesi A., Tarantini F., Di Bari M. (2013). Skeletal muscle: An endocrine organ. Clin. Cases Min. Bone Metab. 10, 11–14. doi:10.11138/ccmbm/2013.10.1.011
Quach N. L., Biressi S., Reichardt L. F., Keller C., Rando T. A. (2009). Focal adhesion kinase signaling regulates the expression of caveolin 3 and beta1 integrin, genes essential for normal myoblast fusion. Mol. Biol. Cell 20, 3422–3435. doi:10.1091/mbc.e09-02-0175
Rao L., Qian Y., Khodabukus A., Ribar T., Bursac N. (2018). Engineering human pluripotent stem cells into a functional skeletal muscle tissue. Nat. Commun. 9, 126. doi:10.1038/s41467-017-02636-4
Rhim C., Cheng C. S., Kraus W. E., Truskey G. A. (2010). Effect of microRNA modulation on bioartificial muscle function. Tissue Eng. Part A 16, 3589–3597. doi:10.1089/ten.TEA.2009.0601
Rhim C., Kraus W. E., Truskey G. A. (2020). Biomechanical effects on microRNA expression in skeletal muscle differentiation. AIMS Bioeng. 7, 147–164. doi:10.3934/bioeng.2020014
Ringholm S., Biensø R. S., Kiilerich K., Guadalupe-Grau A., Aachmann-Andersen N. J., Saltin B., et al. (2011). Bed rest reduces metabolic protein content and abolishes exercise-induced mRNA responses in human skeletal muscle. Am. J. Physiol. Endocrinol. Metab. 301, E649–E658. doi:10.1152/ajpendo.00230.2011
Robinson M. D., Mccarthy D. J., Smyth G. K. (2010). edgeR: a Bioconductor package for differential expression analysis of digital gene expression data. Bioinformatics 26, 139–140. doi:10.1093/bioinformatics/btp616
Rome S., Forterre A., Mizgier M. L., Bouzakri K. (2019). Skeletal muscle-released extracellular vesicles: State of the art. Front. Physiol. 10, 929. doi:10.3389/fphys.2019.00929
Russell A. P., Lamon S., Boon H., Wada S., Güller I., Brown E. L., et al. (2013). Regulation of miRNAs in human skeletal muscle following acute endurance exercise and short-term endurance training. J. Physiol. 591, 4637–4653. doi:10.1113/jphysiol.2013.255695
Sarkar S., Dey B. K., Dutta A. (2010). MiR-322/424 and -503 are induced during muscle differentiation and promote cell cycle quiescence and differentiation by down-regulation of Cdc25A. Mol. Biol. Cell 21, 2138–2149. doi:10.1091/mbc.e10-01-0062
Sato T., Yamamoto T., Sehara-Fujisawa A. (2014). miR-195/497 induce postnatal quiescence of skeletal muscle stem cells. Nat. Commun. 5, 4597. doi:10.1038/ncomms5597
Schaller M. D. (2001). Biochemical signals and biological responses elicited by the focal adhesion kinase. Biochim. Biophys. Acta 1540, 1–21. doi:10.1016/s0167-4889(01)00123-9
Sha Y., Phan J. H., Wang M. D. (2015). Effect of low-expression gene filtering on detection of differentially expressed genes in RNA-seq data. Annu. Int. Conf. IEEE Eng. Med. Biol. Soc. 2015, 6461–6464. doi:10.1109/EMBC.2015.7319872
Singh G. B., Cowan D. B., Wang D.-Z. (2020). Tiny regulators of massive tissue: MicroRNAs in skeletal muscle development, myopathies, and cancer cachexia. Front. Oncol. 10, 598964. doi:10.3389/fonc.2020.598964
Smith T., Heger A., Sudbery I. (2017). UMI-Tools: Modeling sequencing errors in unique molecular identifiers to improve quantification accuracy. Genome Res. 27, 491–499. doi:10.1101/gr.209601.116
Song C., Yang Z., Dong D., Xu J., Wang J., Li H., et al. (2019). miR-483 inhibits bovine myoblast cell proliferation and differentiation via IGF1/PI3K/AKT signal pathway. J. Cell. Physiol. 234, 9839–9848. doi:10.1002/jcp.27672
Tam S., Tsao M. S., Mcpherson J. D. (2015). Optimization of miRNA-seq data preprocessing. Brief. Bioinform. 16, 950–963. doi:10.1093/bib/bbv019
Tarum J., Folkesson M., Atherton P. J., Kadi F. (2017). Electrical pulse stimulation: An in vitro exercise model for the induction of human skeletal muscle cell hypertrophy. A proof-of-concept study. Exp. Physiol. 102, 1405–1413. doi:10.1113/EP086581
Valentino T. R., Rule B. D., Mobley C. B., Nikolova-Karakashian M., Vechetti I. J. (2021). Skeletal muscle cell growth alters the lipid composition of extracellular vesicles. Membr. (Basel) 11, 619. doi:10.3390/membranes11080619
Van Deun J., Mestdagh P., Agostinis P., Akay Ö., Anand S., Anckaert J., et al. (2017). EV-TRACK: Transparent reporting and centralizing knowledge in extracellular vesicle research. Nat. Methods 14, 228–232. doi:10.1038/nmeth.4185
Van Rooij E., Quiat D., Johnson B. A., Sutherland L. B., Qi X., Richardson J. A., et al. (2009). A family of microRNAs encoded by myosin genes governs myosin expression and muscle performance. Dev. Cell 17, 662–673. doi:10.1016/j.devcel.2009.10.013
Vechetti I. J., Peck B. D., Wen Y., Walton R. G., Valentino T. R., Alimov A. P., et al. (2021a). Mechanical overload-induced muscle-derived extracellular vesicles promote adipose tissue lipolysis. Faseb J. 35, e21644. doi:10.1096/fj.202100242R
Vechetti I. J., Valentino T., Mobley C. B., Mccarthy J. J. (2021b). The role of extracellular vesicles in skeletal muscle and systematic adaptation to exercise. J. Physiol. 599, 845–861. doi:10.1113/JP278929
Wei W., Zhang W. Y., Bai J. B., Zhang H. X., Zhao Y. Y., Li X. Y., et al. (2016a). The NF-κB-modulated microRNAs miR-195 and miR-497 inhibit myoblast proliferation by targeting Igf1r, Insr and cyclin genes. J. Cell Sci. 129, 39–50. doi:10.1242/jcs.174235
Wang H., Zhang Q., Wang B., Wu W., Wei J., Li P., et al. (2018). miR-22 regulates C2C12 myoblast proliferation and differentiation by targeting TGFBR1. Eur. J. Cell Biol. 97, 257–268. doi:10.1016/j.ejcb.2018.03.006
Wei X., Li H., Zhang B., Li C., Dong D., Lan X., et al. (2016b). miR-378a-3p promotes differentiation and inhibits proliferation of myoblasts by targeting HDAC4 in skeletal muscle development. RNA Biol. 13, 1300–1309. doi:10.1080/15476286.2016.1239008
Whitham M., Parker B. L., Friedrichsen M., Hingst J. R., Hjorth M., Hughes W. E., et al. (2018). Extracellular vesicles provide a means for tissue crosstalk during exercise. Cell Metab. 27, 237–251. e4. doi:10.1016/j.cmet.2017.12.001
Wong C. F., Tellam R. L. (2008). MicroRNA-26a targets the histone methyltransferase Enhancer of Zeste homolog 2 during myogenesis. J. Biol. Chem. 283, 9836–9843. doi:10.1074/jbc.M709614200
Xu M., Chen X., Huang Z., Chen D., Yu B., Chen H., et al. (2018). MicroRNA-139-5p suppresses myosin heavy chain I and IIa expression via inhibition of the calcineurin/NFAT signaling pathway. Biochem. Biophys. Res. Commun. 500, 930–936. doi:10.1016/j.bbrc.2018.04.202
Xu T., Liu Q., Yao J., Dai Y., Wang H., Xiao J. (2015). Circulating microRNAs in response to exercise. Scand. J. Med. Sci. Sports 25, e149–54. doi:10.1111/sms.12421
Yedigaryan L., Sampaolesi M. (2021)., 10. Cells, 3035. doi:10.3390/cells10113035Therapeutic implications of miRNAs for muscle-wasting conditionsCells
Yoon Y. J., Kim O. Y., Gho Y. S. (2014). Extracellular vesicles as emerging intercellular communicasomes. BMB Rep. 47, 531–539. doi:10.5483/bmbrep.2014.47.10.164
Zarovni N., Corrado A., Guazzi P., Zocco D., Lari E., Radano G., et al. (2015). Integrated isolation and quantitative analysis of exosome shuttled proteins and nucleic acids using immunocapture approaches. Methods 87, 46–58. doi:10.1016/j.ymeth.2015.05.028
Zhang W., Liu H. T. (2002). MAPK signal pathways in the regulation of cell proliferation in mammalian cells. Cell Res. 12, 9–18. doi:10.1038/sj.cr.7290105
Zhang X., Baht G. S., Huang R., Chen Y. H., Molitoris K. H., Miller S. E., et al. (2022). Rejuvenation of neutrophils and their extracellular vesicles is associated with enhanced aged fracture healing. Aging Cell 2022, e13651. doi:10.1111/acel.13651
Zhang X., Hubal M. J., Kraus V. B. (2020). Immune cell extracellular vesicles and their mitochondrial content decline with ageing. Immun. Ageing 17, 1. doi:10.1186/s12979-019-0172-9
Zhang Y., Kim J. S., Wang T. Z., Newton R. U., Galvão D. A., Gardiner R. A., et al. (2021). Potential role of exercise induced extracellular vesicles in prostate cancer suppression. Front. Oncol. 11, 746040. doi:10.3389/fonc.2021.746040
Keywords: extracellular vescicles, microRNA, skeletal muscle, engineered tissue, miRNA sequencing
Citation: Vann CG, Zhang X, Khodabukus A, Orenduff MC, Chen Y-H, Corcoran DL, Truskey GA, Bursac N and Kraus VB (2022) Differential microRNA profiles of intramuscular and secreted extracellular vesicles in human tissue-engineered muscle. Front. Physiol. 13:937899. doi: 10.3389/fphys.2022.937899
Received: 06 May 2022; Accepted: 11 July 2022;
Published: 25 August 2022.
Edited by:
Kenneth Verboven, Universiteit Hasselt, BelgiumReviewed by:
Wanling Xuan, University of South Florida, United StatesMichela Pozzobon, University of Padua, Italy
Copyright © 2022 Vann, Zhang, Khodabukus, Orenduff, Chen, Corcoran, Truskey, Bursac and Kraus. This is an open-access article distributed under the terms of the Creative Commons Attribution License (CC BY). The use, distribution or reproduction in other forums is permitted, provided the original author(s) and the copyright owner(s) are credited and that the original publication in this journal is cited, in accordance with accepted academic practice. No use, distribution or reproduction is permitted which does not comply with these terms.
*Correspondence: Virginia B. Kraus, vbk@duke.edu