- National Technical University of Athens, School of Rural, Surveying and Geoinformatics Engineering, Athens, Greece
Diabetic foot complications have multiple adverse effects in a person’s quality of life. Yet, efficient monitoring schemes can mitigate or postpone any disorders, mainly by early detecting regions of interest. Nowadays, optical sensors and artificial intelligence (AI) tools can contribute efficiently to such monitoring processes. In this work, we provide information on the adopted imaging schemes and related optical sensors on this topic. The analysis considers both the physiology of the patients and the characteristics of the sensors. Currently, there are multiple approaches considering both visible and infrared bands (multiple ranges), most of them coupled with various AI tools. The source of the data (sensor type) can support different monitoring strategies and imposes restrictions on the AI tools that should be used with. This review provides a comprehensive literature review of AI-assisted DFU monitoring methods. The paper presents the outcomes of a large number of recently published scholarly articles. Furthermore, the paper discusses the highlights of these methods and the challenges for transferring these methods into a practical and trustworthy framework for sufficient remote management of the patients.
1 Introduction
Nowadays, millions of people worldwide are living with diabetes. Mistreated diabetes may lead to adverse situations, including the development of diabetic foot ulcers (DFU) or appearance of regions susceptible to infection (Jaly et al., 2020). Infections can range from mild (e.g., cellulitis) to severe ones, such as those affecting the bones (e.g., osteomyelitis). A severe infection can lead to a life-threatening emergency situation (e.g., sepsis), that requires treatment with antibiotics, administered intravenously, and surgical intervention for drainage, debridement, or amputation (Lim et al., 2017). Nevertheless, these cases could be prevented through regular assessment (Cousart and Handley, 2017).
On the one hand, DFU is treated by specialized medical experts. On the other hand, patient’s self-care, outside the clinic, is of utmost significance (Bus and van Netten, 2016). In that context, patient empowerment towards self-monitoring is considered of high-importance. Self-management and monitoring can contribute in preventing an initial ulcer appearance, detecting susceptible signs in a foot region, monitoring existing ulcers to prevent further complications and possible recurrent ulcerations (Armstrong et al., 2017).
There are multiple signs/symptoms related to diabetic foot ulcer. These signs involve skin color change (redness), temperature change, damage to the skin due to abnormal foot plantar pressure, change in pain level or appearance of a new pain, swelling, or odor (Schaper et al., 2016). Most of these indication signs can be captured and, thus, monitored, using various optical and/or laser sensors. Nowadays, red-green-blue (RGB) and thermal sensors have relatively low acquisition costs, are not bulky, and can be integrated to portable devices. Sensory inputs, coupled with deep learning (DL) models, can provide robust mechanisms for preventing undesirable or emergency situations (Tulloch et al. (2020)).
DFU monitoring and prevention is an active research field and there are many important publications in the area of study. Machine learning models including both, shallow learning, and DL approaches have been widely used to support DFU monitoring techniques. (Goyal et al., 2020). Yet, to the best of our knowledge, a sensor-driven survey, spanning multiple monitoring capable ranges, is missing. In this paper, we investigate how smart, low-cost devices (Najafi et al., 2020) embedded with AI tools, could contribute to self-prevention and monitoring of the DFU. This survey focuses on two research topics: a) DFU pathology monitoring, through optical sensors and b) analysis/interpretation of the sensor-oriented data, using machine learning/AI tools.
The remaining of the paper is organized as follows: Section 2 describes the basic monitoring areas, regarding the DFU pathology and clinical features related to DFU detection and monitoring. Sections 3 summarizes the noninvasive sensors that are utilized in DFU monitoring, whereas Section 4 is a brief overview of the machine learning techniques that are applied in DFU monitoring. Section 5 summarizes and analyzes the sensors along with the machine learning techniques that are utilized focusing on Near-Infrared (NIR), Mid-Infrared (MIR) and Long-Infrared (LIR) clinical studies. Section 6 concludes this work and highlights the challenges towards a practical and trustworthy framework for self-monitoring, AI-assisted DFU monitoring approaches.
2 DFU monitoring fundamentals
2.1 DFU development risk factors
DFU is characterized by a complex multifactorial pathogenesis. In the following paragraphs, the most prevalent risk factors and parameters for foot ulcer recurrence (Armstrong et al., 2017) are briefly described, since the medical history of the patient is related to DFU development and progression. The risk factors include: a) the duration of diabetes, b) the history of vascular intervention, c) the amputation, and d) the existence of callus. In the same study, factors such as age, gender, body mass index, smoking, nephropathy, tinea pedis, and hyperkeratosis were not related to DFU.
At first, the duration of diabetes and the blood glucose levels are crucial factors and, thus, consist a key indicator for diabetic foot monitoring (Cheng et al., 2021). Secondly, loss of sense in the foot, due to diabetic neuropathy, in combination with foot deformities, poorly fitting footwear, and excessive pressure, can result in callosities formation that may lead to ulcers development. Thirdly, the peripheral arterial disease (PAD) can cause a decrease in blood circulation, which may cause ulceration, or delay foot healing by reducing the oxygen delivery to peripheral tissues. PAD maym, also, cause a reduce in temperature on plantar foot. Lastly, oxygen concentration can be indirectly calculated; given that hemoglobin-associated oxygen accounts for roughly 97% of total oxygen being transported, the dynamic relationship between oxygen and hemoglobin levels can be assessed for primary determination of oxygen transport.
As such, clinical studies suggest that patients, who have developed or are at risk of developing a diabetic foot ulcer, should periodically monitor and assess the following four factors: a) blood glucose levels (Kateel et al., 2018), b) foot deformity/foot pressure (Yazdanpanah et al., 2018), c) foot temperature (Mejaiti et al., 2018) and d) hemoglobin concentration (Salman et al., 2017). Moreover, the duration of diabetes, the medication history of the patient, and the history of vascular interventions (if any) are also important factors for foot ulcer recurrence.
2.2 DFU clinical features
A diabetic foot ulcer is an open wound with a circular appearance, located on the bottom of the foot and often preceded by a haemorrhagic subepidermal blister. Tissue around the ulcer may become black, and in some cases may gangrene will developed. Foot ulcers are usually painless, leading to delay visits to health professionals. Pedal pulses may be absent and reduced sensation can be demonstrated.
3 Non-invasive sensor technologies for DFU monitoring
The widespread acceptance of non-invasive sensor technologies is essential for the following reasons: a) to monitor major risk factors associated with diabetic foot ulcer, b) to empower patients in self-care, and c) to effectively deliver the remote monitoring and multi-disciplinary prevention needed for those at-risk people and d) to address the health care access disadvantage that people living in remote areas (Najafi and Mishra (2021)). Harnessing sensor technologies to remotely manage diabetic foot is of major importance. In this section we summarize both the sensor technologies and spectrum’s rage that had been used to this day, for monitoring purposes.
3.1 Techniques in the visible spectrum
Visible spectroscopy (VIS) is defined by the luminous efficiency functions ranging between wavelengths of λ = 380 nm and λ = 780 nm (Salman et al., 2017). These sensors measure the surface wound size, or identify the wound boundary/area, since they are visible effects under the light. Moreover, RGB sensors distinguish multiple types of skin deformities (van Netten et al., 2017). Therefore, digital images are utilized for monitoring the wounds’ characteristics.
RGB images are usually identified in computer applications, hand-held devices and mobile applications for wound assessment and monitoring. Monitoring devices equipped with RGB (red-green-blue) sensors is a low cost solution, which can be applied easily for self-monitoring or in primary prevention. However, they do not yield oxygenation or birefringence-related characteristics compared to other approaches. VIS cannot support temperature, glucose, or hemoglobin concentration monitoring. Nevertheless, if we move beyond the visible spectrum, near-/mid-/far-infrared wavelengths can support clinical diagnostics. Figure 1 illustrates the ranges of sensors under consideration in this study. Spectral information other than optical can also be referred to as hyperspectral.
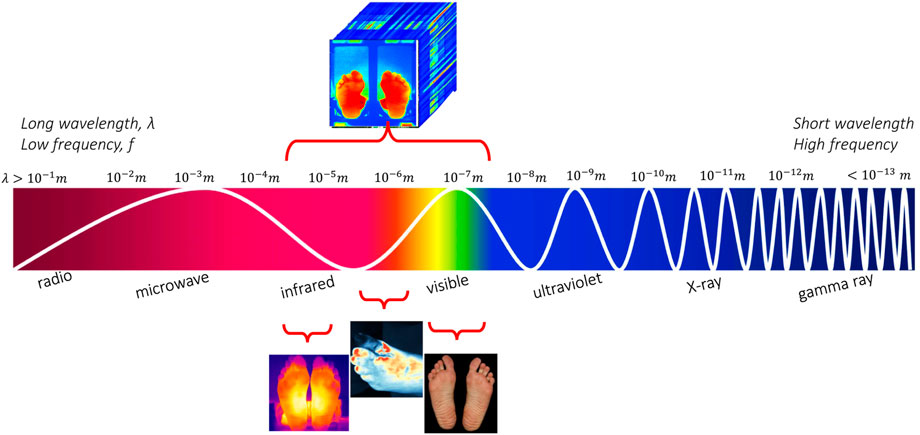
FIGURE 1. The electromagnetic spectrum along with the imagery techniques for the diabetic foot monitoring.
3.2 Techniques in the near-infrared spectrum
NIR spectroscopy provides a viable approach for monitoring saturation levels under the skin. Tissues are relatively transparent to near-infrared light and the absorption of light dependents on the oxygenation status of the tissue (Nouvong et al., 2009). Furthermore, the absorption, scattering, and reflection properties of the propagation of light in a tissue are affected by the wavelength. Yet, the difference between oxygenated and deoxygenated blood can be easily identified in the NIR spectrum. In particular, NIR spectroscopy (700nm − 1300 nm) captures hemoglobin saturation (HbO2, Hb) and peripheral/tissue oxy saturation (StO2, SpO2), or deoxy levels, which are important indicators for DFU early detection (Xie et al., 2021).
3.3 Thermal infrared imaging technologies
Infrared (IR) imaging techniques are utilized for tissue assessment. IR detectors produce a heat map of the foot surface and are utilized for the assessment of the temperature variations in the plantar region. Thermal imaging has been previously used for the diagnosis of many medical conditions, including skin/breast cancer, arthritis, allergy, burns, among others (Gurjarpadhye et al., 2015).
3.4 Hyperspectral imaging technologies
Hyperspectral imaging (HSI) provides information about the chemical properties of materials and their spatial distribution (Khan et al., 2018). HSI covers a continuous portion of the light spectrum, including infrared, near-, mid- and far-infrared (FIR) spectral ranges. Thus, HSI captures the subtle spectral differences under different pathological conditions (Fei, 2020), contrary to multispectral imaging, which supports a limited number of discrete spectral bands. HSI data structures involve multiple 2D images (spatial information) at discrete wavelengths (spectral information) to produce a hypercube (x, y, λ); each pixel in the image is described by a diffuse reflectance spectrum. However, low-cost smartphone-based hyperspectral imaging systems are not mature yet, even though there is a rapid progress in this field Stuart et al. (2021). Thus, hyperspectral imagery data sources provide rich information for different ulcer characteristics, however the equipment that is needed is expensive.
3.5 Comparison of the different optical imaging techniques
Considering the major factors to monitor and the capabilities of the previously described sensors, we could advocate favorably that diabetic feet monitoring using non-invasive sensors is a feasible solution. Yet, successful monitoring requires the combination of different types of sensors, covering multiple ranges, including VIS, near-, mid-, and far-infrared. Table 1 summarizes the range of wavelengths for the investigated sensors.
VIS (400–700 nm) captures different skin color and texture properties. NIR spectroscopy (700nm − 1300 nm) can capture hemoglobin saturation (HbO2, Hb) and peripheral/tissue oxy saturation (StO2, SpO2), or deoxy levels, which are important indicators for DFU early detection (Xie et al., 2021). Thermal Infrared spectroscopy can detect hyperthermia (or hypothermia), among ROIs of a foot (or comparatively between right/left foot), that consist major factors for microcirculation and edema assessment in DFU (Rubins et al., 2019). Finally, the Mid-IR absorbance (5.7 μm − 9.3 μm) spectrum contains rich information about the proteomics, lipidomics and metabolomics (e.g., glucose) (Kottmann et al., 2012).
4 Machine learning techniques for DFU monitoring
4.1 Overview of the machine learning techniques for DFU
4.1.1 Supervised learning techniques
Machine learning and signal processing methods can contribute significantly in early diagnosis, predictive modelling, analytics, and characterization of the diabetic foot (Tulloch et al., 2020; Yap et al., 2021). ML approaches focusing on DFU detection have received renewed attention, mostly thanks to the increased number of datasets with RGB images (Yap et al., 2022). To this day, various models have been developed using supervised learning methods to detect/localize abnormalities on medical images related to diabetic foot (Zhang et al., 2022). These tasks may involve: (i) classification (Goyal et al., 2020; Xu et al., 2021), (ii) object detection (Cassidy et al., 2021), and (iii) semantic segmentation (Rania et al., 2020; Kendrick et al., 2022). Depending on the scenario, different types of training sets are used, and a minimum but sufficient amount of data is required.
Nowadays, research depends, mainly, on deep learning approaches for solving complex problems. The main advantage lies in the capability to discover hidden patterns in the data or establish a better understanding of intricate relationships among many interdependent variables. Given the sensors availability, and the spectral bands range per sensor, we have large amounts of data appropriate for training purposes. Consequently, deep learning approaches occurred naturally as an application scenario in DFU monitoring, based on optical information (Voulodimos et al., 2018).
In medical applications, the availability of labeled training data is extremely limited owing to the nature of the data containing protected health information. However, supervised machine learning requires a great amount of labeled data to train a model, which is at the origin of the main bottleneck in model development. In literature, the studies address the lack of labeled data by proposing alternative methods to overcome this problem (Alzubaidi et al., 2021). As shown in sub-sections that follow, unsupervised or semi-supervised schemes are efficient for these scenarios.
4.1.2 Unsupervised learning techniques
Unsupervised learning based models have been used to surpass the problem of labelled data, since these algorithms can infer adequate feature representations of input values, without using labelled data. Typically, in healthcare related studies unsupervised learning manifests as clustering techniques, which detect hidden patterns or groupings in data Tulloch et al. (2020). Extracting meaningful features from the original raw values is useful for reducing the dimension of feature space and achieving better clustering performance Ji et al. (2020).
4.1.3 Semi-supervised learning techniques
Other approaches may involve tensor-based Makantasis et al. (2021) and semi-supervised learning Protopapadakis et al. (2021). Both techniques exploit feature space projections, which allow for better handling of sets of high-order data and/or limited training sets. The former case, i.e., tensor-based learning, imposes canonical/Polyadic (CP) decomposition of rank-R on its weights, leading to a significant reduction of the number of hyperparameters during training. The later case, couples labeled and unlabeled data to form additional regularization terms, resulting in higher performance models.
4.2 Identified sensor/AI tools synergies
Diabetic foot can cause severe morbidity in diabetic patients with a subsequent increased cost for the health care system. Prevention and early diagnosis are the keys to decrease the prevalence of diabetic foot. Due to Covid-19 pandemic evolution, public health guidelines should be reconfigured to support and manage diabetic foot patients including remote consultations. Self-foot assessment is a critical tool for the highly beneficial early detection of ulcers. Development and proper usage of an objective tool that can assess the foot of a diabetic patient are key interventions towards prediction and early diagnosis of diabetic foot with significant benefits for the patient and the health care system. Various sensory systems can be used towards this direction. Table 2 highlights the most important conclude remarks per sensory system.
5 Grouping of the machine learning approaches in DFU monitoring depending on the range of the electromagnetic spectrum
Non-invasive sensors have contributed in a great extend for diabetic foot monitoring. In addition, the recently released datasets captured from these sensors provide a breeding ground for the application of machine learning algorithms. In this section, we group the literature works and the proposed approaches, based on the type of sensor that is utilised during capturing and the electromagnetic spectrum that is covered. In particular, we divide the literature work in four main categories that is works dealing with: i) data captured from the visible spectrum, ii) hyper-spectral imaging data, iii) thermal imaging data, and iv) data from the middle-IR spectrum.
In every single subsection, the respective research works are structured in a table format. The columns of each table are: i) an identifier of the presented work, ii) information about the equipment that is utilized from data capturing as well as information about the environmental conditions during the data capturing process, iii) the number of the participants in the clinical study, their inclusion/exclusion criteria if available and the participants number in total, in the forth column we have iv) the adopted modelling approach/pipeline and finally, we present v) the experimental results, the utilized metrics and the final findings of the study.
5.1 AI techniques and imagery data of the visible spectrum
In this subsection, we summarize the literature works that process imagery data of the visible spectrum (RGB). The research interest in DFU is growing, as the number of reported cases of diabetes is also grown at a worldwide level. Early attempts in training machine learning models in this domain have shown promising results. Wang et al. (2019) use four different support vector machines (SVMs) to determine wound area in images. Yap et al. assessed the reliability of an application to standardize the image capture of DFUs Yap et al. (2018). Nanda et al. (2022) evaluate the performance of different shallow learning techniques, including Random Forests (RF), SVM, Naive Bayes (NB), K-nearest neighbour (KNN) methods, for the identification of the risk factors associated with development of diabetic foot ulcer. Recently, the release of the Diabetic Foot Ulcers Grand Challenge (DFUC 2020) dataset consisting of labelled images has attracted the interest for applying machine learning algorithms in diabetic foot monitoring applications. The dataset consists of 2,496 ulcers in the training set and 2,097 ulcers in the testing set.
With the entrance of new labelled datasets, the implementation of deep learning approaches is feasible. As regards the classification, in the work of Alatrany et al. (2022), they used a deep learning autoencoder as a feature extractor and subsequently trained multiple ML models to accurately classify healthy and DFU skin regions. Feature fusion techniques that combine low- and high-level information could be combined as proposed in Das et al. (2022), to improve the capabilities in identification of DFU normal and abnormal classes. As regards semantic segmentation tasks, Cui et al. compare SVM algorithms among with two convolutional neural networks (CNN)-based network architectures (U-Net and a patch-based CNN), showing that the U-Net approach yields the best performance compared to others Cui et al. (2019). Another work that supports these findings is the work of Ohura et al. (2019), who compared four different CNN architectures and identified U-Net as the best performing method, with sensitivity of 85.8% and specificity of 98.8% on the DFU dataset. Recently, the modern implementations in DFU detection methods include the popular deep learning models for object detection, such as Faster R–CNN, YOLOv3, YOLOv5, and EfficientDet (Yap et al., 2021). Also, ensemble method are proposed in the literature as well as networks consisting of cascade attention networks ((Cai and Vasconcelos, 2019)).
Table 3 summaries the literature works where RGB imaging data used for diabetic foot applications, along with the analysis procedure followed and the findings of each study. Both shallow and deep learning methods are considered.
5.2 AI techniques and hyperspectral imagery data
Near-infrared radiation can be transmitted through the body, since it is not absorbed by water or hemoglobin Pasquini (2003). The near infrared spectra consist of vibrational overtones and combination absorption features with spectral signatures that allow identification and mapping of different materials. Oxygenation/deoxygenation of hemoglobin, oxidized cytochrome c oxidase, and oxygenated/deoxygenated myoglobin have a unique absorption spectrum in the NIR region Ciurczak and Igne (2014). The diagnostic potential of NIR spectroscopy has been previously used to study a range of diverse conditions, including diabetes mellitus chronic complications Aitchison et al. (2018), atherosclerotic occlusive disease Saito et al. (2018), Alzheimer’s disease Khagi et al. (2018), etc.
Triggered by the different absorption spectra of oxygen and deoxyhemoglobin, in biomedical HSI, most of the researchers have utilized reflectance spectra to estimate oxygen saturation (SpO2) values from peripheral tissue (Yang et al., 2018). Hyperspectral tissue oxygenation measurements can readily indicate changes in tissue surrounding the ulcer, when comparing ulcers that heal and ulcers that do not heal.
Hyperspectral data have various spectral bands. However, due to the curse of dimensionality, the existence of multiple spectral bands may decrease the performance of the classification algorithms (Zhang et al., 2020). Principal component analysis (PCA) is a common technique for dimensionality reduction in medical hyperspectral datasets.
Then, ML algorithms automatically classify ulcers as healing or non-healing. Techniques, such as SVMs and neural networks (NN) or even CNNs, are widely used for medical hyperspectral image classification. HSI technique was used for healing prediction in routine practice. The data were analyzed to detect differences between patients with DFUs that healed and those with DFUs that did not heal. Table 4 summarizes the research on diabetic foot monitoring in the near infrared spectrum.
5.3 Mid-infrared sensing for DFU
Mid-IR photoacoustic (PA) spectroscopy techniques utilize wavelengths of light in a range that allows monitoring glucose concentration levels in epidermal skin. However, MIR spectroscopy does not penetrate in deep skin layers and it is usually observed signal deterioration due to the strong absorption of water, which is in abundance in human body. To overcome this limitation, Kottman et al. Kottmann et al. (2012) proposed a hybrid setup that consists of a photoacoustic device and a tunable quantum cascade laser (QCL) to track glucose in deep epidermal layers. This dual-wavelength approach yields a considerably improved stability and lower uncertainty compared to the traditional MIR spectroscopic techniques (Table 5).
5.4 Infrared thermal sensing for DFU
Long Wavelength Infrared cameras can be utilized for temperature inspection applications, due to their capability in detecting distinct temperature differences. Infrared thermography (IRT) is a fast, passive, contactless and non-invasive technique for temperature monitoring, for various parts of the human body (Vardasca et al., 2019a). Body’s temperature distribution is an important indicator for various disease patterns, and thermography stands as an adequate and flexible procedure, which is easy to use and has a low-cost (Sarawade and Charniya, 2018).
A thermal camera consists of five components: the optic system, the detector, the amplifier, the signal processing component, and the display (Sarawade and Charniya, 2018). Medical Infrared Thermography output is a two-dimensional digital image that provides data about the physiology of tissues (Hillen et al., 2020). Currently, multiple studies focus on the diagnosis of diabetic foot diseases using skin temperature variation (van Netten et al., 2013). A commonly adopted approach involves the division of the images into Regions of Interest (ROIs) and, then, the comparison of the relative difference in temperature, between the right and the left foot respectively.
Given the thermal imaging of the two feet, the mean temperature difference (ΔT) between each ROI of the right foot and the respective ROI of the left foot is calculated. Having computed the mean absolute temperature difference between corresponding points in both feet, various machine learning techniques are proposed for automatic diagnosis of diabetic foot. The detection is based on various criteria. Some of them compare the temperature between the studied feet and the healthy participant’s feet, while others compare the relative difference of the temperature in the homologous ROI points of the two feet. Also, there are a few studies that use unsupervised techniques to cluster the severity risk of diabetic foot ulcers for a single foot (Khandakar et al. (2022)). Table 6 provides a summary on the thermal/infrared imaging systems used for diabetic foot applications, along with the analysis procedure followed and the findings of each study.
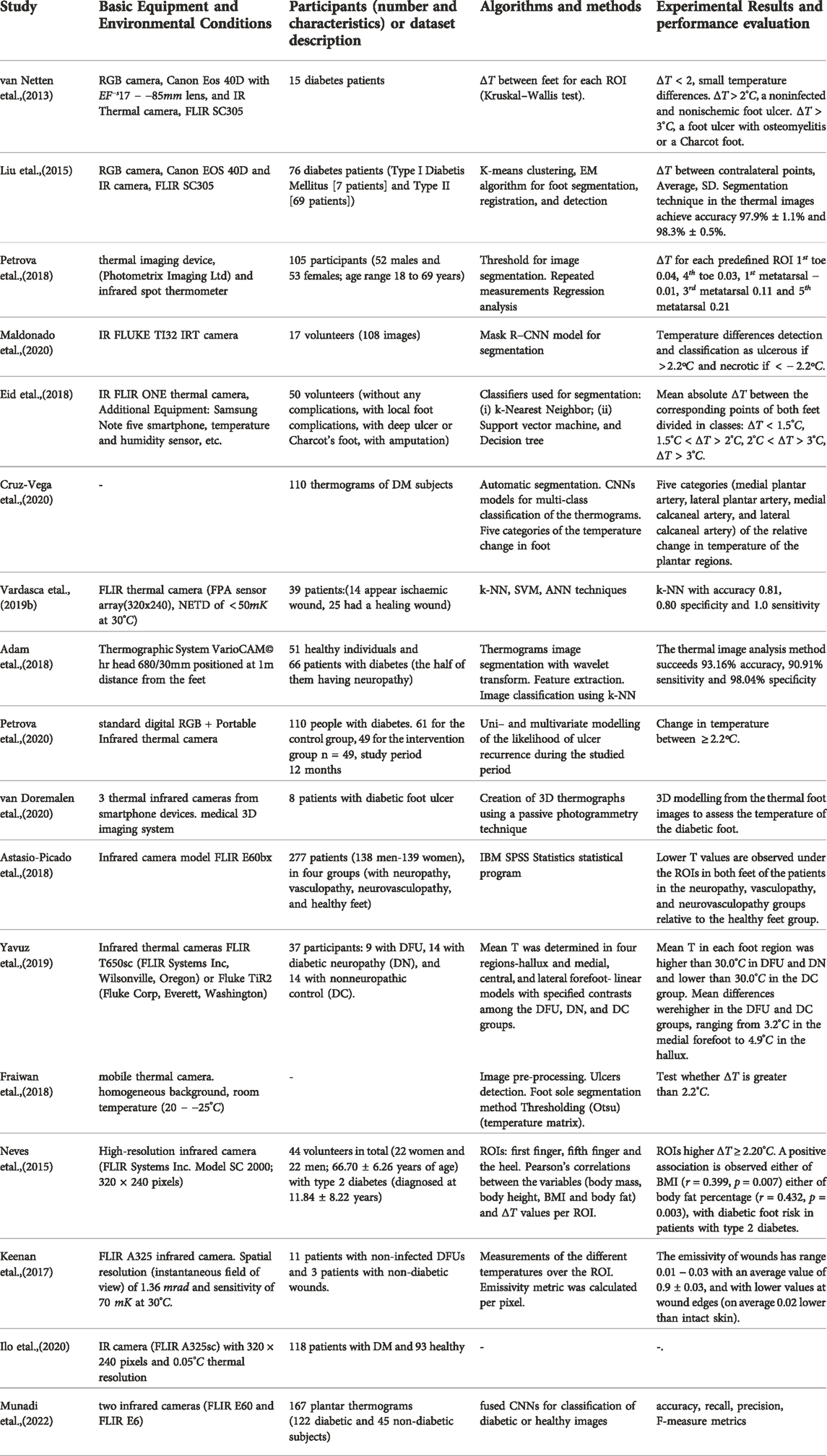
TABLE 6. Summary of the literature for diabetic foot monitoring using imagery data from thermal sensors.
6 Discussion
In this paper we focused on comparing noninvasive techniques and approaches for DFU monitoring, and to highlight their advantages and disadvantages. There are multiple sensors, which can support efficiently the monitoring process, resulting in an improvement of patient’s life. Yet, the type of raw data provided, including spectral range and resolution, remains a topic of debate, susceptible to limitations related to cost and portability of the monitoring devices. In total, four crucial monitoring factors have been identified namely: a) glucose levels, b) foot deformity/wounds, c) temperature, and d) hemoglobin concentration. All these factors can be currently monitored with the usage of various sensors. Currently, none of the existing sensors that are commercially available supports a holistic self monitoring, including measurements for all the above mentioned factors. In particularly, RGB sensors can be used to distinguish deformities on the skin, NIR spectroscopy (700nm − 1300 nm) can capture haemoglobin saturation, thermal infrared spectroscopy can detect hyperthermia (or hypothermia), among ROIs of a foot, and the Mid-IR absorbance (5.7 μm − 9.3 μm) spectrum provides rich information about the proteomics, lipidomics, and metabolomics (e.g., glucose).
6.1 Challenges on data acquisition
As regards the cost-effectiveness of the sensory systems, it heavily depends on the type of sensors that each system is equipped with. On the one hand, self-monitoring devices are usually equipped with RGB cameras or with sensors in thermal infrared spectrum González-Pérez et al. (2021) and both cases are considered low-cost. On the other hand, HSI equipment is more expensive than the traditional methods and it is not recommended for self-monitoring cases López-Moral et al. (2022).
High quality visible and infrared (thermography) images can be acquired through portable devices (e.g., cell-phones or even lightweight and low-cost equipment). As regards hyperspectral imagery data in DFU monitoring, the high volume of HSI images is an obstacle for applying these techniques to be used in conjunction with the smartphone applications. However, recently there are appeared relevant studies that propose solutions where acquiring HSI images through smart phone devices could be feasible Stuart et al. (2021). Hyperspectral images are capable of accurate spectral and spatial data collection and have the potential for future deployment combined with smartphones for DFU monitoring applications. Portability and user-friendly setup of this devices is of major concern, in order to make it a valuable instrument to assist the decision process of the doctors. However, these solutions are yet immature and future development and improvements in this area of research is necessary in order to provide commercial products and ready-to-use solutions.
6.2 Challenges on machine learning algorithms
Machine learning and computer vision methods, including detection, segmentation and classification, are used to analyse the diabetic foot ulcer images. Usually, DFU is addressed as a binary classification problem; we either have a DFU or not, or we have the appearance of a specific factor, correlated to DFU. To assess the usability of these models, it is important to incorporate them on mobile and cloud or even edge-based technologies. Given the significant and growing impact of DFU, mobile health solutions that target this condition could assist in improving patient quality of life (Cassidy et al., 2022). Taking this into consideration, trustworthiness in AI solutions for DFU monitoring is an important aspect.
The performance evaluation of the ML model aims to assess the method’s effectiveness in the accurate detection of DFU. There are various metrics for ML models, which can be often combined to evaluate a model (Zhang et al., 2022). Cross-validation technique is also an important part of the evaluation process, because it provides an insight into the model’s precision level and is a necessary part of the algorithmic process to ensure the models’ stability and to define the confidence intervals of the proposed method. The machine learning approaches should always keep the trade-off between the complexity of the model/architecture and the accuracy improvement. CNNs have had a great success in the recent past, due to the advent of faster GPUs and vast amount of memory access, however, deploying deep learning applications on the edge has constrains as regards the computational resources. Thus, it is important to make models feasible for constrained devices, such as mobile phones. In health care systems, deep-learning models largely rely on sufficient and diverse training data gathered from patients. However, leveraging AI-based technologies to improve the management of diabetic foot ulcers is usually challenging due to limits arising from the legislation on patient’s privacy and data security. Thus, safety and privacy issues may imply fewer data available; therefore, additional challenges for training supervised AI algorithms with good performance appear. However, recently, federated schemes have emerged as the state-of-the-art techniques in order to achieve personalized recommendations in health care systems with state-of-the-art accuracy, while ensuring privacy preservation for the patient (Rieke et al., 2020).
Author contributions
MK and EP were responsible for drafting this manuscript and oversaw the research adaptations discussed, in collaboration with ND and AD. All authors contributed to the article and approved the submitted version.
Funding
The work in this paper has been supported by the H2020 Phootonics project: “A Cost-Effective Photonics-based Device for Early Prediction, Monitoring and Management of Diabetic Foot Ulcers” funded under the ICT H2020 framework and the grand agreement no. 871908.
Conflict of interest
The authors declare that the research was conducted in the absence of any commercial or financial relationships that could be construed as a potential conflict of interest.
Publisher’s note
All claims expressed in this article are solely those of the authors and do not necessarily represent those of their affiliated organizations, or those of the publisher, the editors and the reviewers. Any product that may be evaluated in this article, or claim that may be made by its manufacturer, is not guaranteed or endorsed by the publisher.
Appendix A
Abbreviations
ANN, Artificial neural network; BMI; Body mass index; CCD, Charge-coupled device; CNN, Convolutional neural network; DFU, Diabetic foot ulcer; DM, Diabetes Mellitus; EC-QCL, External cavity-quantum cascade laser; EM, Expectation maximization; GAN, Generative Adverserial neural network; HSI, hyperspectral imaging; IRT; Infrared thermography, k-NN; k-nearest neighbours; LCTF, Liquid crystal tunable filter; MaP, Mean average precision; MIR, Mid - infrared; NIR, Near - infrared; PAD, Peripheral arterial disease; PCA, Principal component analysis; PSNR, Peak signal-to-noise-ratio; QCL, Quantum cascade lasers; RCNN, Region-based Convolutional Neural Network; RMSE, Root Mean Square Error; ROI, Region of interest; SSIM, Structural similarity index measure; SVM, Support vector machines; VSI, Visual spectrum imaging.
References
Adam M., Ng E. Y., Oh S. L., Heng M. L., Hagiwara Y., Tan J. H., et al. (2018). Automated detection of diabetic foot with and without neuropathy using double density-dual tree-complex wavelet transform on foot thermograms. Infrared Phys. Technol. 92, 270–279. doi:10.1016/j.infrared.2018.06.010
Aitchison R. T., Ward L., Kennedy G. J., Shu X., Mansfield D. C., Shahani U. (2018). Measuring visual cortical oxygenation in diabetes using functional near-infrared spectroscopy. Acta Diabetol. 55, 1181–1189. doi:10.1007/s00592-018-1200-5
Alatrany A. S., Hussain A., Alatrany S. S., Al-Jumaily D. (2022). “Application of deep learning autoencoders as features extractor of diabetic foot ulcer images,” in International conference on intelligent computing (Germany: Springer), 129–140.
Alzubaidi L., Al-Amidie M., Al-Asadi A., Humaidi A. J., Al-Shamma O., Fadhel M. A., et al. (2021). Novel transfer learning approach for medical imaging with limited labeled data. Cancers 13, 1590. doi:10.3390/cancers13071590
Armstrong D. G., Boulton A. J., Bus S. A. (2017). Diabetic foot ulcers and their recurrence. N. Engl. J. Med. 376, 2367–2375. doi:10.1056/NEJMra1615439
Astasio-Picado A., Martínez E. E., Nova A. M., Rodríguez R. S., Gómez-Martín B. (2018). Thermal map of the diabetic foot using infrared thermography. Infrared Phys. Technol. 93, 59–62. doi:10.1016/j.infrared.2018.07.008
Bus S. A., van Netten J. J. (2016). A shift in priority in diabetic foot care and research: 75% of foot ulcers are preventable. Diabetes. Metab. Res. Rev. 32, 195–200. doi:10.1002/dmrr.2738
Cai Z., Vasconcelos N. (2019). Cascade r-cnn: High quality object detection and instance segmentation. IEEE Trans. Pattern Anal. Mach. Intell. 43, 1483–1498. doi:10.1109/TPAMI.2019.2956516
Cassidy B., Reeves N. D., Pappachan J. M., Ahmad N., Haycocks S., Gillespie D., et al. (2022). A cloud-based deep learning framework for remote detection of diabetic foot ulcers. IEEE Pervasive Comput. 21, 78–86. doi:10.1109/mprv.2021.3135686
Cassidy B., Reeves N. D., Pappachan J. M., Gillespie D., O’Shea C., Rajbhandari S., et al. (2021). The dfuc 2020 dataset: Analysis towards diabetic foot ulcer detection. touchREV. Endocrinol. 17, 5–11. doi:10.17925/EE.2021.17.1.5
Cheng Y., Zu P., Zhao J., Shi L., Shi H., Zhang M., et al. (2021). Differences in initial versus recurrent diabetic foot ulcers at a specialized tertiary diabetic foot care center in China. J. Int. Med. Res. 49, 030006052098739. doi:10.1177/0300060520987398
Ciurczak E. W., Igne B. (2014). Pharmaceutical and medical applications of near-infrared spectroscopy. Florida: CRC Press.
Cousart T. H., Handley M. (2017). Implementing diabetic foot care in the primary care setting. J. Nurse Pract. 13, e129–e132. doi:10.1016/j.nurpra.2016.11.009
Cruz-Vega I., Hernandez-Contreras D., Peregrina-Barreto H., Rangel-Magdaleno J. d. J., Ramirez-Cortes J. M. (2020). Deep learning classification for diabetic foot thermograms. Sensors 20, 1762. doi:10.3390/s20061762
Cui C., Thurnhofer-Hemsi K., Soroushmehr R., Mishra A., Gryak J., Domínguez E., et al. (2019). Diabetic wound segmentation using convolutional neural networks. In 2019 41st Annual International Conference of the IEEE Engineering in Medicine and Biology Society (EMBC) (IEEE), 23-27 July 2019, Berlin, Germany, 1002–1005.
Das S. K., Roy P., Mishra A. K. (2022). Fusion of handcrafted and deep convolutional neural network features for effective identification of diabetic foot ulcer. Concurrency Comput. 34, e6690. doi:10.1002/cpe.6690
Davradou A., Protopapadakis E., Kaselimi M., Doulamis A., Doulamis N. (2022). “Diabetic foot ulcers monitoring by employing super resolution and noise reduction deep learning techniques,” in Proceedings of the 15th International Conference on PErvasive Technologies Related to Assistive Environments (New York, NY: Association for Computing Machinery), 83–88. doi:10.1145/3529190.3529214
Eid M. M., Yousef R. N., Mohamed M. A. (2018). A proposed automated system to classify diabetic foot from thermography. Int. J. Sci. Eng. Res. 9, 371–381.
Fei B. (2020). Hyperspectral imaging in medical applications. Data Handl. Sci. Technol. 32, 523–565. Elsevier. doi:10.1016/B978-0-444-63977-6.00021-3
Fraiwan L., Ninan J., Al-Khodari M. (2018). Mobile application for ulcer detection. Open Biomed. Eng. J. 12, 16–26. doi:10.2174/1874120701812010016
González-Pérez S., Perea Ström D., Arteaga-Marrero N., Luque C., Sidrach-Cardona I., Villa E., et al. (2021). Assessment of registration methods for thermal infrared and visible images for diabetic foot monitoring. Sensors 21, 2264. doi:10.3390/s21072264
Goyal M., Reeves N. D., Davison A. K., Rajbhandari S., Spragg J., Yap M. H. (2018). Dfunet: Convolutional neural networks for diabetic foot ulcer classification. IEEE Trans. Emerg. Top. Comput. Intell. 4, 728–739. doi:10.1109/tetci.2018.2866254
Goyal M., Reeves N. D., Rajbhandari S., Ahmad N., Wang C., Yap M. H. (2020). Recognition of ischaemia and infection in diabetic foot ulcers: Dataset and techniques. Comput. Biol. Med. 117, 103616. doi:10.1016/j.compbiomed.2020.103616
Greenman R. L., Panasyuk S., Wang X., Lyons T. E., Dinh T., Longoria L., et al. (2005). Early changes in the skin microcirculation and muscle metabolism of the diabetic foot. Lancet 366, 1711–1717. doi:10.1016/S0140-6736(05)67696-9
Gurjarpadhye A. A., Parekh M. B., Dubnika A., Rajadas J., Inayathullah M. (2015). Infrared imaging tools for diagnostic applications in dermatology. SM J. Clin. Med. Imaging 1, 1–5.
Hillen B., Pfirrmann D., Nägele M., Simon P. (2020). Infrared thermography in exercise physiology: The dawning of exercise radiomics. Sports Med. 50, 263–282. doi:10.1007/s40279-019-01210-w
Ilo A., Romsi P., Mäkelä J. (2020). Infrared thermography and vascular disorders in diabetic feet. J. Diabetes Sci. Technol. 14, 28–36. doi:10.1177/1932296819871270
Jaly I., Iyengar K., Bahl S., Hughes T., Vaishya R. (2020). Redefining diabetic foot disease management service during Covid-19 pandemic. Diabetes Metab. Syndr. 14, 833–838. doi:10.1016/j.dsx.2020.06.023
Jeffcoate W., Clark D., Savic N., Rodmell P., Hinchliffe R., Musgrove A., et al. (2015). Use of hsi to measure oxygen saturation in the lower limb and its correlation with healing of foot ulcers in diabetes. Diabet. Med. 32, 798–802. doi:10.1111/dme.12778
Ji X., Akiyarna Y., Yamada Y., Okamoto S., Hayashi H. (2020). Development of deep clustering model to stratify occurrence risk of diabetic foot ulcers based on foot pressure patterns and clinical indices. In 2020 IEEE International Joint Conference on Biometrics (IJCB) (IEEE), 01 October 2020, Houston, TX, USA, 1–8
Kateel R., Augustine A. J., Prabhu S., Ullal S., Pai M., Adhikari P. (2018). Clinical and microbiological profile of diabetic foot ulcer patients in a tertiary care hospital. Diabetes Metab. Syndr. 12, 27–30. doi:10.1016/j.dsx.2017.08.008
Keenan E., Gethin G., Flynn L., Watterson D., O’Connor G. M. (2017). Enhanced thermal imaging of wound tissue for better clinical decision making. Physiol. Meas. 38, 1104–1115. doi:10.1088/1361-6579/aa6ea0
Kendrick C., Cassidy B., Pappachan J. M., O’Shea C., Fernandez C. J., Chacko E., et al. (2022). Translating clinical delineation of diabetic foot ulcers into machine interpretable segmentation. arXiv preprint arXiv:2204.11618
Khagi B., Lee C. G., Kwon G.-R. (2018). Alzheimer’s disease classification from brain mri based on transfer learning from cnn. In 2018 11th biomedical engineering international conference (BMEiCON) (IEEE), 21-24 November 2018, Thailand,
Khan M. J., Khan H. S., Yousaf A., Khurshid K., Abbas A. (2018). Modern trends in hyperspectral image analysis: A review. Ieee Access 6, 14118–14129. doi:10.1109/access.2018.2812999
Khandakar A., Chowdhury M. E., Reaz M. B. I., Ali S. H. M., Kiranyaz S., Rahman T., et al. (2022). A novel machine learning approach for severity classification of diabetic foot complications using thermogram images. Sensors 22, 4249. doi:10.3390/s22114249
Khaodhiar L., Dinh T., Schomacker K. T., Panasyuk S. V., Freeman J. E., Lew R., et al. (2007). The use of medical hyperspectral technology to evaluate microcirculatory changes in diabetic foot ulcers and to predict clinical outcomes. Diabetes care 30, 903–910. doi:10.2337/dc06-2209
Kottmann J., Rey J. M., Luginbühl J., Reichmann E., Sigrist M. W. (2012). Glucose sensing in human epidermis using mid-infrared photoacoustic detection. Biomed. Opt. Express 3, 667–680. doi:10.1364/BOE.3.000667
Lim J. Z. M., Ng N. S. L., Thomas C. (2017). Prevention and treatment of diabetic foot ulcers. J. R. Soc. Med. 110, 104–109. doi:10.1177/0141076816688346
Liu C., van Netten J. J., Van Baal J. G., Bus S. A., van Der Heijden F. (2015). Automatic detection of diabetic foot complications with infrared thermography by asymmetric analysis. J. Biomed. Opt. 20, 026003. doi:10.1117/1.JBO.20.2.026003
López-Moral M., García-Álvarez Y., Molines-Barroso R. J., Tardáguila-García A., García-Madrid M., Lázaro-Martínez J. L. (2022). A comparison of hyperspectral imaging with routine vascular noninvasive techniques to assess the healing prognosis in patients with diabetic foot ulcers. J. Vasc. Surg. 75, 255–261. doi:10.1016/j.jvs.2021.07.123
Makantasis K., Georgogiannis A., Voulodimos A., Georgoulas I., Doulamis A., Doulamis N. (2021). Rank-r fnn: A tensor-based learning model for high-order data classification. IEEE Access 9, 58609–58620. doi:10.1109/access.2021.3072973
Maldonado H., Bayareh R., Torres I., Vera A., Gutiérrez J., Leija L. (2020). Automatic detection of risk zones in diabetic foot soles by processing thermographic images taken in an uncontrolled environment. Infrared Phys. Technol. 105, 103187. doi:10.1016/j.infrared.2020.103187
Mejaiti N., van Netten J. J., Dijkgraaf M. G., van Baal J. G., Busch-Westbroek T. E., Bus S. A., et al. (2018). The cost-effectiveness and cost-utility of at-home infrared temperature monitoring in reducing the incidence of foot ulcer recurrence in patients with diabetes (diatemp): Study protocol for a randomized controlled trial. Trials 19, 1–12. doi:10.1186/s13063-018-2890-2
Munadi K., Saddami K., Oktiana M., Roslidar R., Muchtar K., Melinda M., et al. (2022). A deep learning method for early detection of diabetic foot using decision fusion and thermal images. Appl. Sci. 12, 7524. doi:10.3390/app12157524
Najafi B., Mishra R. (2021). Harnessing digital health technologies to remotely manage diabetic foot syndrome: A narrative review. Medicina 57, 377. doi:10.3390/medicina57040377
Najafi B., Reeves N. D., Armstrong D. G. (2020). Leveraging smart technologies to improve the management of diabetic foot ulcers and extend ulcer-free days in remission. Diabetes. Metab. Res. Rev. 36, e3239. doi:10.1002/dmrr.3239
Nanda R., Nath A., Patel S., Mohapatra E. (2022). Machine learning algorithm to evaluate risk factors of diabetic foot ulcers and its severity. Med. Biol. Eng. Comput. 60, 2349–2357. doi:10.1007/s11517-022-02617-w
Neves E. B., Almeida A. J., Rosa C., Vilaça-Alves J., Reis V. M., Mendes R. (2015). Anthropometric profile and diabetic foot risk: A cross-sectional study using thermography. 2015 37th Annu. Int. Conf. IEEE Eng. Med. Biol. Soc. (EMBC) (IEEE) 2015, 1–3. doi:10.1109/EMBC.2015.7445519
Nouvong A., Hoogwerf B., Mohler E., Davis B., Tajaddini A., Medenilla E. (2009). Evaluation of diabetic foot ulcer healing with hyperspectral imaging of oxyhemoglobin and deoxyhemoglobin. Diabetes care 32, 2056–2061. doi:10.2337/dc08-2246
Ohura N., Mitsuno R., Sakisaka M., Terabe Y., Morishige Y., Uchiyama A., et al. (2019). Convolutional neural networks for wound detection: The role of artificial intelligence in wound care. J. Wound Care 28, S13–S24. doi:10.12968/jowc.2019.28.Sup10.S13
Pasquini C. (2003). Near infrared spectroscopy: Fundamentals, practical aspects and analytical applications. J. Braz. Chem. Soc. 14, 198–219. doi:10.1590/s0103-50532003000200006
Petrova N., Donaldson N., Tang W., MacDonald A., Allen J., Lomas C., et al. (2020). Infrared thermography and ulcer prevention in the high-risk diabetic foot: Data from a single-blind multicentre controlled clinical trial. Diabet. Med. 37, 95–104. doi:10.1111/dme.14152
Petrova N., Whittam A., MacDonald A., Ainarkar S., Donaldson A., Bevans J., et al. (2018). Reliability of a novel thermal imaging system for temperature assessment of healthy feet. J. Foot Ankle Res. 11, 22–26. doi:10.1186/s13047-018-0266-1
Protopapadakis E., Doulamis A., Doulamis N., Maltezos E. (2021). Stacked autoencoders driven by semi-supervised learning for building extraction from near infrared remote sensing imagery. Remote Sens. 13, 371. doi:10.3390/rs13030371
Rania N., Douzi H., Yves L., Sylvie T. (2020). “Semantic segmentation of diabetic foot ulcer images: Dealing with small dataset in dl approaches,” in International conference on image and signal processing (Germany: Springer), 162–169.
Rieke N., Hancox J., Li W., Milletari F., Roth H. R., Albarqouni S., et al. (2020). The future of digital health with federated learning. NPJ Digit. Med. 3, 1–7. doi:10.1038/s41746-020-00323-1
Rubins U., Marcinkevics Z., Cimurs J., Saknite I., Kviesis-Kipge E., Grabovskis A. (2019). Multimodal device for real-time monitoring of skin oxygen saturation and microcirculation function. Biosensors 9, 97. doi:10.3390/bios9030097
Saito H., Ishikawa T., Tanabe J., Kobayashi S., Moroi J. (2018). Bedside assessment of regional cerebral perfusion using near-infrared spectroscopy and indocyanine green in patients with atherosclerotic occlusive disease. Sci. Rep. 8, 1242–1248. doi:10.1038/s41598-018-19668-5
Salman I. N., Wadood S. A., Abualkasem B. A. (2017). Low hemoglobin levels in infected diabetic foot ulcer. Age (y) 36, 40–65.
Sarawade A. A., Charniya N. N. (2018). Infrared thermography and its applications: A review. In 2018 3rd International Conference on Communication and Electronics Systems (ICCES) (IEEE), 15-16 October 2018, Coimbatore, 280–285.
Schaper N., Van Netten J., Apelqvist J., Lipsky B., Bakker K., Diabetic I. (2016). Prevention and management of foot problems in diabetes: A summary guidance for daily practice 2015, based on the iwgdf guidance documents. Diabetes. Metab. Res. Rev. 32, 7–15. doi:10.1002/dmrr.2695
Stuart M. B., McGonigle A. J., Davies M., Hobbs M. J., Boone N. A., Stanger L. R., et al. (2021). Low-cost hyperspectral imaging with a smartphone. J. Imaging 7, 136. doi:10.3390/jimaging7080136
Tulloch J., Zamani R., Akrami M. (2020). Machine learning in the prevention, diagnosis and management of diabetic foot ulcers: A systematic review. IEEE Access 8, 198977–199000. doi:10.1109/access.2020.3035327
Tzortzis I. N., Davradou A., Protopapadakis E., Kaselimi M., Doulamis N., Angeli A., et al. (2022). Unsupervised diabetic foot monitoring techniques. In Proceedings of the 15th International Conference on PErvasive Technologies Related to Assistive Environments, March 10, 2022, Kerkira. 608–614.
van Doremalen R. F., van Netten J. J., van Baal J. G., Vollenbroek M. M., Heijden F. (2020). Infrared 3d thermography for inflammation detection in diabetic foot disease: A proof of concept. J. Diabetes Sci. Technol. 14, 46–54. doi:10.1177/1932296819854062
van Netten J. J., Clark D., Lazzarini P. A., Janda M., Reed L. F. (2017). The validity and reliability of remote diabetic foot ulcer assessment using mobile phone images. Sci. Rep. 7, 1–10. doi:10.1038/s41598-017-09828-4
[Dataset] van Netten J. J., van Baal J. G., Liu C., van Der Heijden F., Bus S. A. (2013). Infrared thermal imaging for automated detection of diabetic foot complications. J. Diabetes Sci. Technol. 7, 1122–1129. doi:10.1177/193229681300700504
Vardasca R., Magalhães C., Seixas A., Carvalho R., Mendes J. (2019b). Diabetic foot monitoring using dynamic thermography and ai classifiers. In Third Quantitative Infrared Thermography Asian Conference, July 2019: Tokyo, Japan
Vardasca R., Magalhaes C., Silva P., Abreu P., Mendes J., Restivo M. T. (2019a). Biomedical musculoskeletal applications of infrared thermal imaging on arm and forearm: A systematic review. J. Therm. Biol. 82, 164–177. doi:10.1016/j.jtherbio.2019.04.008
Voulodimos A., Doulamis N., Doulamis A., Protopapadakis E. (2018). Deep learning for computer vision: A brief review. Comput. Intell. Neurosci. 2018. doi:10.1155/2018/7068349
Wang L., Pedersen P. C., Agu E., Strong D. M., Tulu B. (2019). Boundary determination of foot ulcer images by applying the associative hierarchical random field framework. J. Med. Imaging 6, 024002. doi:10.1117/1.JMI.6.2.024002
Xie P., Li Y., Deng B., Du C., Rui S., Deng W., et al. (2021). An explainable machine learning model for predicting in-hospital amputation rate of patients with diabetic foot ulcer. Int. Wound J. 19, 910–918. doi:10.1111/iwj.13691
Xu Y., Han K., Zhou Y., Wu J., Xie X., Xiang W. (2021). Classification of diabetic foot ulcers using class knowledge banks. Front. Bioeng. Biotechnol. 9, 811028. doi:10.3389/fbioe.2021.811028
Yang Q., Sun S., Jeffcoate W. J., Clark D. J., Musgove A., Game F. L., et al. (2018). Investigation of the performance of hyperspectral imaging by principal component analysis in the prediction of healing of diabetic foot ulcers. J. Imaging 4, 144. doi:10.3390/jimaging4120144
Yap M. H., Chatwin K. E., Ng C.-C., Abbott C. A., Bowling F. L., Rajbhandari S., et al. (2018). A new mobile application for standardizing diabetic foot images. J. Diabetes Sci. Technol. 12, 169–173. doi:10.1177/1932296817713761
Yap M. H., Hachiuma R., Alavi A., Brüngel R., Cassidy B., Goyal M., et al. (2021). Deep learning in diabetic foot ulcers detection: A comprehensive evaluation. Comput. Biol. Med. 135, 104596. doi:10.1016/j.compbiomed.2021.104596
Yavuz M., Ersen A., Hartos J., Lavery L. A., Wukich D. K., Hirschman G. B., et al. (2019). Temperature as a causative factor in diabetic foot ulcers: A call to revisit ulceration pathomechanics. J. Am. Podiatr. Med. Assoc. 109, 345–350. doi:10.7547/17-131
Yazdanpanah L., Shahbazian H., Nazari I., Arti H. R., Ahmadi F., Mohammadianinejad S. E., et al. (2018). Incidence and risk factors of diabetic foot ulcer: A population-based diabetic foot cohort (adfc study)—two-year follow-up study. Int. J. Endocrinol. 2018, 7631659. doi:10.1155/2018/7631659
Yudovsky D., Nouvong A., Pilon L. (2010). Hyperspectral imaging in diabetic foot wound care. J. Diabetes Sci. Technol. 4, 1099–1113. doi:10.1177/193229681000400508
Zhang J., Qiu Y., Peng L., Zhou Q., Wang Z., Qi M. (2022). A comprehensive review of methods based on deep learning for diabetes-related foot ulcers. Front. Endocrinol. 13, 945020. doi:10.3389/fendo.2022.945020
Keywords: diabetic foot, artificial intelligence, review, sensors, hyperspectral imaging
Citation: Kaselimi M, Protopapadakis E, Doulamis A and Doulamis N (2022) A review of non-invasive sensors and artificial intelligence models for diabetic foot monitoring. Front. Physiol. 13:924546. doi: 10.3389/fphys.2022.924546
Received: 20 April 2022; Accepted: 05 October 2022;
Published: 21 October 2022.
Edited by:
Yi Jiang, Georgia State University, United StatesReviewed by:
Nazmi Yaraş, Akdeniz University, TurkeyAmin Zehtabian, Freie Universität Berlin, Germany
Copyright © 2022 Kaselimi, Protopapadakis, Doulamis and Doulamis. This is an open-access article distributed under the terms of the Creative Commons Attribution License (CC BY). The use, distribution or reproduction in other forums is permitted, provided the original author(s) and the copyright owner(s) are credited and that the original publication in this journal is cited, in accordance with accepted academic practice. No use, distribution or reproduction is permitted which does not comply with these terms.
*Correspondence: Maria Kaselimi, bWthc2VsaW1pQG1haWwubnR1YS5ncg==