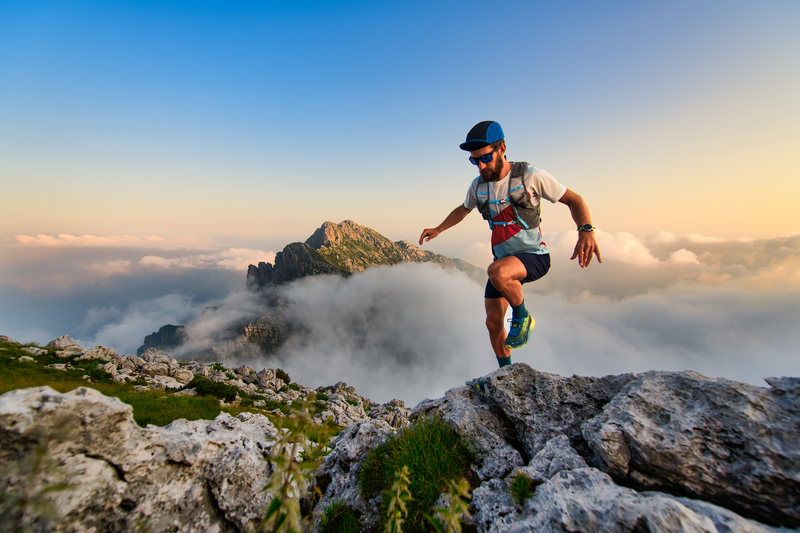
94% of researchers rate our articles as excellent or good
Learn more about the work of our research integrity team to safeguard the quality of each article we publish.
Find out more
ORIGINAL RESEARCH article
Front. Physiol. , 06 October 2022
Sec. Striated Muscle Physiology
Volume 13 - 2022 | https://doi.org/10.3389/fphys.2022.899784
This article is part of the Research Topic Molecular Physiology of Skeletal Muscle Cells View all 4 articles
Skeletal muscle adaptation is correlated to training exercise by triggering different signaling pathways that target many functions; in particular, the IGF1-AKT pathway controls protein synthesis and degradation. These two functions regulate the adaptation in size and strength of muscles. Computational models for muscle adaptation have focused on: the biochemical description of signaling pathways or the mechanical description of muscle function at organ scale; however, an interrelation between these two models should be considered to understand how an adaptation in muscle size affects the protein synthesis rate. In this research, a dynamical model for the IGF1-AKT signaling pathway is linked to a continuum-mechanical model describing the active and passive mechanical response of a muscle; this model is used to study the impact of the adaptive muscle geometry on the protein synthesis at the fiber scale. This new computational model links the signaling pathway to the mechanical response by introducing a growth tensor, and links the mechanical response to the signaling pathway through the evolution of the protein synthesis rate. The predicted increase in cross sectional area (CSA) due to an 8 weeks training protocol excellently agreed with experimental data. Further, our results show that muscle growth rate decreases, if the correlation between protein synthesis and CSA is negative. The outcome of this study suggests that multi-scale models coupling continuum mechanical properties and molecular functions may improve muscular therapies and training protocols.
Training exercise has an important effect on skeletal muscle anatomy and physiology by means of increasing the protein content. Whereas training exercise is related to the mechanical behavior of muscle, increasing protein content is related to its biological behavior.
From the biological perspective, protein synthesis is promoted by the IGF1-AKT signaling pathway (Sandri, 2008; Schiaffino and Mammucari, 2011). The most prominent relation in this pathway starts when insuline-like growth factor (IGF1) (Adams and McCue, 1998) promotes the serine/threonine kinase (AKT) (Song et al., 2005). After that, AKT promotes the mammalian target of rapamycin (mTOR) (Hay and Sonenberg, 2004) and inhibits the forkhead box transcription factor (FOXO) (Wang et al., 2014). Two outcomes can occur: hypertrophy or atrophy. If FOXO is inhibited, then mTOR activates protein synthesis to produce hypertrophy. If AKT is inhibited, then FOXO promotes protein degradation and the inhibition of mTOR resulting in muscle atrophy.
The regulation of protein content is the key to preserve or improve the ability to generate force. On a subcellular scale, force generation is closely related to its microstructure and can be explained by the sliding filament theory (Hanson and Huxley, 1953; Pollack, 1983; Huxley, 2000) and the cross-bridge theory (Huxley, 1957). According to the sliding filament theory, internal sarcomere constituents -actin and myosin- overlap and change the overlapping length while momentarily bound to one another; this short-lasting bound generates force and is known as cross-bridge (Huxley and Hanson, 1959; Randall et al., 2002; Gautel, 2008; Rui et al., 2010). The force generation process starts when the nervous system sends electrical signals to muscle fibers triggering a muscle contraction (Matthews, 1931; Kraus et al., 1994; De Deyne, 2001).
From a mechanical perspective, one can distinguish between a passive and an active response; the active response accounts for muscle contraction. The force generated during contraction is assumed to be a function of the fiber stretch that occurs during the actin and myosin overlap (Gordon et al., 1966; Flitney and Hirst, 1978; Stephenson and Williams, 1982). In contrast, a passive response occurs when a muscle is stretched without contracting.
In a continuum-mechanical setting, the active (Ramírez et al., 2010; Heidlauf et al., 2017) and passive (Takaza et al., 2013; Bleiler et al., 2019) responses of skeletal muscle tissue are modelled by appealing to hyperelastic transversely isotropic constitutive laws. Growth has been considered within continuum-mechanical models of cardiac tissue and to describe the longitudinal growth of skeletal muscles (Göktepe et al., 2010; Zöllner et al., 2012; Altan et al., 2016). Growth is typically based on a multiplicative decomposition (Rodriguez et al., 1994) of the deformation gradient into elastic and growth components. The elastic component requires the characteristic material response of the tissue (passive response), whereas the growth component requires further assumptions like growth multipliers (Göktepe et al., 2010). The continuum mechanical description of growth is a macroscopic and purely phenomenological process and lacks direct links to the underlying biochemical aspects.
Growth is a biochemical response that requires weeks to produce an increase in protein content, and is triggered by an intermittent but regular stimulus (training or physical activity). Although existing continuum-mechanical models of muscle tissue growth predict adaptation in the scale of weeks, those models require continuous stimulation (sustained strain or stress), and none of them considers biochemical processes to predict the resulting change in mass.
Given a training protocol, a model that predicts changes in muscle properties greatly benefits high performance athletes, recovery patients after injuries or illnesses, and the general public. Such model allows to fine tune the protocol (training frequency, intensity, physical restrictions etc) to efficiently target desired outcomes. The aim of this work is to present a multi-scale mechanobiological model for skeletal muscle adaptation. After briefly introducing the independent models for the IGF1-AKT signaling pathway (biochemical model) and fundamental equations of continuum-mechanical basis, i.e., how to model active and passive responses for muscle tissue (mechanical model), we propose a novel description to 1) couple the biochemical model to the mechanical model (by defining of a growth multiplier); 2) couple the mechanical model to the biochemical model (by defining an appropriate feedback function). To validate our new model, we predict the muscular changes due to a specific training protocol and compare the outcome with experimental data. We conclude by discussing our findings.
This section is divided into three parts: Section 2.1 (Independent models) describes the main characteristics of the IGF1-AKT signaling pathway model and the skeletal muscle passive and active hyperelastic material model; Section 2.2 (Mechanobiological model) presents the procedures to couple: first, the biochemical to the mechanical model, and second, the mechanical to the biochemical model; Section 2.3 (Numerical experiments) provides details for the computational implementation, the finite-elements structure, the exercise-training protocol, and the biochemical model parameters.
The independent models are introduced in this section. The biochemical model in Section 2.1.1 describes the dynamical system that explains the interaction between the variables of the IGF1-AKT signaling pathway. The mechanical model in Section 2.1.2 describes the characteristics of the continuum model for skeletal muscle mechanical response.
The IGF1-AKT signaling pathway is triggered by an external stimulus; as a result, the concentration of each molecule involved in this pathway evolves producing hypertrophy or atrophy. Based on the simplified mechanism of the IGF1-AKT signaling pathway reviewed by Schiaffino and Mammucari (2011), we developed the biochemical model presented in Villota-Narvaez et al. (2021). Our biochemical model produces the evolution of the cellular myofibril content, and it is suitable for atrophy scenarios, whereas the model presented in the present paper improves the hypertrophy results. We briefly summarize our biochemical model in this section.
We assume that the essential molecules of the pathway are IGF1, AKT, FOXO, and mTOR, and each molecule is represented by variables x1, x2, x3, and x4 respectively. The variables are treated as populations that interact in a Lotka-Volterra system.
Following the pathway in Figure 1, physical activity produces an increase in x1 that remains high as long as the activity is sustained. The increase in x1 produces an increase in x2 that persists for about 6 h after the activity is stopped (Bickel et al., 2005). The increase in x2 produces both an increase in x4 and a decrease in x3. An increased x4 correlates to increased protein synthesis and, in the long term, to hypertrophy. In contrast, drastically reduced physical activity produces a decrease in x1. The decrease in x1 produces a decrease in x2. The decrease in x2 causes both a decrease in x4 and an increase in x3. An increased x3 correlates to protein degradation and, in the long term, to atrophy. Figure 1 sketches the interaction between these variables.
FIGURE 1. Simplified signaling pathway for muscle adaptation presented by Schiaffino and Mammucari (2011). IGF1 activates AKT, AKT activates mTOR and inhibits FOXO, FOXO inhibits mTOR and promotes atrophy, mTOR promotes hypertrophy.
The coupled ordinary differential equation system is
Terms in Eqs 1a, 1b, 1c, 1d, 1e are: xi is the population of molecule i, coefficient ai is the intrinsic growth rate of the molecule i, and coefficient cij is the coupling factor between molecules i and j. Since population xi grows exponentially in the absence of interactions between species, the rate of change of xi increases at the intrinsic growth rate ai. Each population is limited by its own population at the self-inhibition rate bi; molecules xi and xj, interact with a coupling strength cij. In the Eq. 1e, z is the myofibril population, and its rate of change is given by:
which depends on 1) the difference between populations x3 and x4, and their respective thresholds
The main outcome of the dynamical model (Eqs 1a, 1b, 1c, 1d, 1e) is the rate of change of population z, i.e.,
The highly organized arrangement of muscle fibers explains the transversely isotropic mechanical behavior of skeletal muscle tissue (Van Loocke et al., 2008; Morrow et al., 2010; Takaza et al., 2013); therefore, the mechanical response is described using a hyperelastic, transversely isotropic constitutive model (Holzapfel, 2000). In this work we used a multiplicative decomposition approach of the deformation gradient F (Holzapfel, 2000); the decomposition splits the deformation gradient into volume-changing (volumetric) and volume-preserving (isochoric) parts; then, we used an additional multiplicative decomposition of the isochoric part during contraction (Grasa et al., 2012).
The first decomposition is
where λa characterizes the active stretch of muscle fibers during contraction, and m° is the direction of the muscle fibers.
The use of the multiplicative decomposition leads to consider three contributions to the strain energy function: a volumetric contribution to ensure the nearly incompressible material behavior of the tissue, a passive contribution to account for elastic deformations, and an active contribution to describe muscle contraction. Within this paper, we assume the following strain energy function (SEF):
where J and λa were defined previously;
Following Martins et al. (1998), the volumetric contribution is assumed to be
where D is a property of the material that controls the bulk modulus.
The passive contribution (Grasa et al., 2011) is:
with
where
Finally, the active contribution (Ramírez et al., 2010; Grasa et al., 2012) is:
where
FIGURE 2. Force-Stretch relation for a muscle. λopt is the stretch where the muscle exerts its maximum force, decreasing or increasing stretch will produce a drop in the generated force. During active response, the maximum isometric tension σo characterizes the maximum force, and the active stretch λa characterizes stretch; whereas during passive response, the stretch of collagen fibers
The adaptation process takes place in a time course much longer than the activation time course; therefore, the force-velocity relation is not considered relevant in our model. Following Grasa et al. (2012), the functions in Eq. 8 are defined as follows:
where λopt characterizes the optimal length where muscle fibers produce their maximum force, and α is a parameter that characterizes how fast the force decays around λopt.
The mechanical model allows the description of the deformations of muscle tissue. When we consider a change in size due to muscle hypertrophy or atrophy, it will affect the deformation gradient via the growth tensor that is described in the next section.
In the previous sections, the biochemical and mechanical models that compose our multi-scale mechanobiological model were described. In this section we introduce the concepts to interrelate those independent models.
To describe the coupling procedures between the independent models, we need two conceptual stages and two coupling functions. First, we define the two stages as follows: a training session that typically ranges from minutes to hours, occurs when external loading conditions stimulate the biochemical pathway, and allows the assessment of CSA and force according to the mechanical model; and a growth period that typically ranges from days to weeks, occurs after each training session, and promotes the evolution of the myofibril population z according to the biochemical model. The intention of the two functions, growth multiplier and force-activation relation, is to build a mathematical connection between the different scales of the independent models and will be briefly described in the paragraphs below. Details of the construction of the functions and the coupling procedures are presented in Supplementary Appendices SA, SB.
The growth of the muscle structure is characterized by the growth tensor Fg, which, following a multiplicative decomposition (Lee, 1969; Rodriguez et al., 1994; Stålhand et al., 2008), requires an elastic deformation Fe to ensure compatible configurations while the muscle grows:
The growth tensor Fg links the rate of change of myofibrils
where κ is a proportionality constant in myofibrils/cm2, and Δt is the time step of the evolution of the muscle structure during the growth period. Details about the construction of the growth multiplier are presented in Supplementary Appendix SA.1.
In our model, the growth tensor follows the description given by Göktepe et al. (2010), and in analogy to Eq. 3, we have,
where
The biochemical model is now coupled to the mechanical model by converting
Now that we linked the biochemical to the mechanical model, we need to close the feedback loop by linking the mechanical to the biochemical model. In this regard, the force-activation relation
The mechanical model is coupled to the biochemical model by modifying the protein synthesis rate (k1 in Eq. 2). This modification uses the function
In summary, the biochemical model is coupled to the mechanical model by means of the growth tensor, and the mechanical model is coupled to the biochemical model by means of the force-activation relation. A full algorithm of the computational model is shown in Figure 3.
FIGURE 3. Algorithm for the mechanobiological model for muscle adaptation. Each function outside a block is an output of the closer block and an input for the next block. f is the rate of change of the myofibril population, Fg is the growth tensor, F and
Table 1 shows the values of the equation parameters and initial conditions required for the implementation of the biochemical model. The training input (a1(t) function) was based on the experimental protocol given in DeFreitas et al. (2011). Subjects in that study performed a total of 24 1-hour sessions on days 1, 3, and 5 of every week. The intensity of training was approximately 80% of the one repetition maximum (1-RM) force. As defined in Section 2.2, the training session is the 1-hour training session, while the growth period is the time right after training. The training protocol was implemented by the following two-state function a1(t) that triggers the biochemical model:
Where β = 2/50 (hours*kgf)−1; I is the fraction of the maximum force (Fmax in kgf) that the muscle can produce; a10 is given in Table 1.
TABLE 1. Parameters and initial conditions for the biochemical model. For equation system 1: intrinsic growth rates (ai), self-inhibition rates (bi), coupling strengths between species (cij). For the rate of change of the myofibril population (Eq. 2): Minimum value (zmin); maximum value (zMax); threshold for x3
a2(t), required for the dynamics of x2, was defined as:
where τh = 6 h, t1 = 17 h, a20 is given in Table 1. In the case when no exercise signal is applied, a2(t) = a20.
Table 1 shows parameters and initial conditions for the biochemical model, those values were reported in Villota-Narvaez et al. (2021). In that study, homeostatic concentrations of the molecules involved in the pathway, taken from Léger et al. (2006) and Bickel et al. (2005), were normalized to the concentration of IGF1, and those values were used as initial conditions; the myofibril population was set to 1; parameters for the equation system 1 were fitted to experimental evidence on atrophy and used in this article for the hypertrophy case.
Equation system 1a, 1b, 1c, 1d, 1e was solved by means of a Runge-Kutta fourth-order method in a Fortran routine. The time step was dt = 0.05 h and the total time simulated was 8 weeks.
The difference in muscle size from t to t + dt is negligible; therefore, we use a much longer time step Δt = 1 h for the adaptation in size during the growth period. By solving equation system 1a, 1b, 1c, 1d, 1e, we have 1/dt data points for each simulated hour and for each biochemical variable. From all those data, we used the values of f(x3, x4) every Δt/dt steps to build the growth tensor.
Our FEM procedure uses an idealized muscle structure as shown in Figure 4. The initial structure was built from a cylindrical shape of 20 cm long, 2.34 cm diameter on the top and bottom, and 6.5 cm diameter half height. The geometry was discretized into a structured mesh of 832 8-noded brick elements with a total of 1107 nodes (see Figure 4). As boundary conditions, nodes at top and bottom surfaces were kept fixed for displacement and rotation. Table 2 shows the set of parameters for the implementation of the mechanical model.
The implementation of the mechanical model depends on the stage of the evolution. First, the implementation during the growth period assumes the growth multiplier given in Eq. 11, the growth tensor given by Eq. 12, the activation parameter β = 0, and Δt = 1 h. Second, during the training session, the growth tensor is equal to the identity tensor; the activation parameter increases from 0 to 1 and then decreases back to 0; and Δt only counts the activation steps (it does not have a physical meaning during the evaluation of force). The mechanical response of the tissue was simulated by means of a UEL subroutine, implemented in Fortran, linked to the solving procedures in the specialized software ABAQUS 3DEXPERIENCE R2017x (Dassault Systemes USA, Waltham, MA).
We will compare the CSA evolution from our mechanobiological model to the adaptation results obtained by DeFreitas et al. (2011). In their study, the CSA of the right thigh muscle was measured every week by using a peripheral quantitative computed tomography.
The applicability of our model is explored by simulating the hypertrophy results under different training frequencies, different values of the proportionality constant κ defined in Eq. 11, and variations of the modified protein synthesis rate.
For an estimation of the effect of parameter values of Eqs 1a, 1b, 1c, 1d, 1e, we tested variations of the parameters within 1% of the value presented in Table 1; we calculated the Root Mean Squared Error RMSE of the CSA relative to the hypertrophy results reported by DeFreitas et al. (2011), and the maximum increase in CSA. We varied one parameter while all the others remained fixed.
The mechanobiological model in Section 2.2 was tested under the numerical experiments described in Section 2.3. The main result of the numerical experiments was the increase in CSA of the muscle structure at the end of three different stages of the algorithm (see Figure 3). Relative to the experimental results, the CSA evolution improves after each stage: first, at the end of the biochemical model (myofibril population z); second, at the end of the mechanical model before the implementation of the feedback from the mechanical to the biochemical model (
The first two stages show that the CSA of the muscle structure differs from the myofibril population of the biochemical prediction, as seen in Figure 5. This difference is explained by the elastic response of the material that enforces compatible configurations. In this regard, since the mechanical properties of the muscle tissue do not change due to adaptation processes, the elastic deformation contribution of the deformation gradient (Eq. 10) does not depend on our biochemical parameters. Hence, the difference between CSA and biochemical prediction shown in Figure 5 cannot be avoided because the biochemical model only controls the growth tensor contribution of the deformation gradient.
FIGURE 5. Cross Sectional Area and myofibril population comparison. These results were obtained by considering full activation (β = 1) for the whole training protocol, and no feedback from the mechanical to the biochemical model.
Before the results of the third stage, we need to consider function
FIGURE 6. Activation level β as a function of the CSA
Assuming an increasing CSA, the following observations about function
Now, let us consider the CSA after stage three, which includes the function
where k10 is the k1 value used in the biochemical system without feedback, and dimensionless parameters d1 and d2 allow us to adjust the strength of the coupling; in our simulations: d1 = 20.40, and d2 = 18.907.
Figure 7 shows a comparison of the ratio k1/k10 of the biochemical system alone, and the same ratio using the coupling relation
FIGURE 7. Protein synthesis rate k1 given by Eq. 15, at parameters d1 = 20.40 and d2 = 18.907. Here, we compare the growth rate k1 of the original biochemical system Eqs 1a, 1b, 1c, 1d, 1e with the modified value of k1 using the feedback from the mechanical response.
FIGURE 8. Normalized CSA adaptation due to training. Experimental results compared to our mechanobiological model. The activation function shown in Figure 6 was used to feedback equation system Eqs 1a, 1b, 1c, 1d, 1e. The modified protein synthesis rate k1(β) was defined in Eq. 15, and replaces the constant value of the protein synthesis rate in function
FIGURE 9. Biochemical variables and protein synthesis rate during the CSA adaptation shown in Figure 8. Dashed lines represent threshold levels in figures (A,C); these figures show that periodic training leads to oscillations of x3 below its threshold, and x4 above its threshold; those levels favor hypertrophy according to Eq. 2. Figures (B,D) show that protein synthesis rate decreases as z increases. And figure (D) shows the results presented in Figure 5 after the use of function
Figure 8 shows our model results when the feedback is used in k1, and also a comparison with experiments. Our results show that muscle grows faster during the early days of the training period, and the growing speed decreases with time even when training is continued. This means that the protein synthesis rate decreases as the protein content increases until eventually a maximum muscle size is reached. In our model, the protein synthesis rate was initially set constant (before the feedback implementation); when we fed back the biochemical system directly in the protein synthesis rate (according to Eq. 15) our model matches in size and shape the experimental results. Therefore, we argue that the muscle adaptation feedback affects directly the protein synthesis level. In addition, our model shows that a muscle cannot grow indefinitely when driven only by exercise.
The evolution of x3 and x4 presented in Figures 9A,C is specific for the training protocol of DeFreitas et al. (2011) that consisted in three training sessions per week. Different training frequencies affect the oscillations of x3 and x4, whose values lead to different responses (according to Eq. 2) depending on how frequently x3 and x4 cross their thresholds. Figure 10A shows the results of the simulation under different protocols: training every day, every 2 days, every 3 days, and on days 1, 3, and 5 of every week (protocol used by DeFreitas et al. (2011)). Our results show that the maximum CSA increase is affected by training frequency: the more trainings per week, the higher the CSA increase. Our results also suggest that training three times per week slowly leads to a maximum CSA close to the results of training every 2 days, whereas training every 3 days leads to a significantly less CSA increase.
FIGURE 10. CSA increase under variation of: (A) training frequency, and (B) the proportionality constant κ defined in Supplementary Equation S16, with
Supplementary Equation S16 allows us to have
To evaluate variations of the modified protein synthesis rate in a simplified way, note that the results shown in Figure 7 suggest a straight line approximation to the numerical relation k1/k10. We tested such approximation as
FIGURE 11. (A) is the linear approximation of the k1/k10 numerical solution of Figure 7, the variation of the slope represents different protein synthesis responses. (B) Evolution of the CSA for the different variations presented in (A).
Parameters a10, b1, c21, and c32 have very little effect (RMSE changes less than 1%, and CSA changes less than 0.2%). Parameters a20, b2, c42, and c43 have a small effect (RMSE increases up to 3 times the best fit value, while CSA changes less than 2%). Parameters a3 and b3 have a considerable effect (RMSE increases up to 8 times the best fit value, while CSA changes about 4%). Parameters a4 and b4 have a big effect (RMSE increases up to 16 times the best fit value, while CSA changes up to 9%). None of the tested variations produced saturation in hypertrophy, but zero hypertrophy resulted by 1% smaller value of a4, and also by 1% larger value of b4. These results are consistent with the importance of x3 and x4, because these variables control the rate of change of z (Eq. 1e).
Regarding results shown in Figure 8. Experiments show that muscle grows even in the early days of a training period. According to Seynnes et al. (2007) and DeFreitas et al. (2011), the early increase in CSA can be considered as hypertrophy; but Damas et al. (2016) argue that a major contribution could be related to edema. To solve the edema observation, Stock et al. (2017) measured the CSA increase under a concentric-only training; they showed that hypertrophy is small but detectable during the first training sessions. We can see that the increase in CSA is similar in all cases, even when the training protocol avoids edema. Thus, we can argue that, although our model ignores muscle damage and considers isometric contraction, our results are in good agreement to regular and concentric-only training.
We found that training frequency has a big impact in CSA increase assuming a constant volume per training session. However, some authors suggest that training volume per week is a variable more important than frequency Figueiredo et al. (2018), Schoenfeld et al. (2017). Evidence shows that training once per week leads to the same hypertrophy results than training 2 or 3 times per week when training is volume equated Brigatto et al. (2019), Gentil et al. (2015), Grgic et al. (2019). Our model requires more details in the training signal to address training volume and other features of different training protocols.
Regarding the effect of κ, myofibril size increases during skeletal muscle growth, and, after reaching a threshold size, they split (Goldspink, 1970; Jorgenson et al., 2020). This evidence may explain our results in Figure 10B: the more myofibrils per unit area, the smaller their size; therefore, larger myofibrils increase the myofibril population sooner than smaller ones, because larger myofibrils split easier than smaller ones. In this sense, smaller values of κ (larger initial myofibril sizes) lead to larger hypertrophy results.
Our results on protein synthesis rate adaptation are consistent with evidence on training status. According to Phillips et al. (2002), untrained subjects show a higher protein synthesis than trained subjects. In our model, training status relates to the force-activation relation
We proposed a multi-scale mechanobiological model for muscle adaptation. Starting at the biochemical base of the IGF1-AKT signaling pathway to predict how the protein content inside a muscle fiber evolves, we defined a growth multiplier and subsequently a growth tensor that allowed us to connect the cellular scale of the adaptation to the organ scale by means of the mechanics of growing tissue. Furthermore, the characteristic adaptation in force allowed us to build a function that describes how the activation of a muscle changes during the adaptation process. We proposed that this function affects the protein synthesis rate, and in this way the function connects the organ scale to the cellular scale.
Our multi-scale mechanobiological model is triggered by an exercise training protocol, and allows to predict how the protein content of the muscle evolves. We found that the activation required to produce force changes during the training protocol, and we argue that this activation change can be considered as a quantification of common training recommendations regarding intensity and loading increase.
Although many important aspects of the muscle function, such as neural signaling, fatigue, and fiber differentiation were not taken into account, our results show that the methodology of the growth tensor and the feedback function, which are key in our mechanobiological model, is capable of producing remarkable agreement with experiments. Future work will address the inclusion of fiber differentiation and fatigability.
The original contributions presented in the study are included in the article/Supplementary Material, further inquiries can be directed to the corresponding author.
YV-N, DG-A, and AR-M contributed to the conception and mathematical formulation of the model; YV-N and DG-A contributed to the implementation of the computational model; YV-N, DG-A, and OR contributed to the interpretation and analysis of the numerical results; YV-N drafted the manuscript; DG-A, AR-M, and OR critically revised the manuscript for intellectual content.
This work received financial support from: Ministerio de Ciencia Tecnología e Innovación, Colombia, under announcement 647/2015; project number INVING3505 of Universidad Militar Nueva Granada sede Campus; Federal Ministry of Education and Research (BMBF) research project “3DFoot” under contract number 01EC1907B; and Deutsche Forschungsgemeinschaft (DFG, German Research Council) SPP 2311 under Grant Number RO 4019/5-1.
YV-N warmly thanks Hugo Novoa-Losada for his careful language editing and proofreading of the text.
The authors declare that the research was conducted in the absence of any commercial or financial relationships that could be construed as a potential conflict of interest.
All claims expressed in this article are solely those of the authors and do not necessarily represent those of their affiliated organizations, or those of the publisher, the editors and the reviewers. Any product that may be evaluated in this article, or claim that may be made by its manufacturer, is not guaranteed or endorsed by the publisher.
The Supplementary Material for this article can be found online at: https://www.frontiersin.org/articles/10.3389/fphys.2022.899784/full#supplementary-material
Adams G. R., McCue S. A. (1998). Localized infusion of igf-i results in skeletal muscle hypertrophy in rats. J. Appl. Physiol. 84, 1716–1722. doi:10.1152/jappl.1998.84.5.1716
Altan E., Zöllner A., Avcı O., Röhrle O. (2016). Towards modelling skeletal muscle growth and adaptation. Proc. Appl. Math. Mech. 16, 921–924. doi:10.1002/pamm.201610448
Bickel C. S., Bickel C. S., Slade J., Slade J., Mahoney E., Mahoney E., et al. (2005). Time course of molecular responses of human skeletal muscle to acute bouts of resistance exercise. J. Appl. Physiol. 4560, 482–488. doi:10.1152/japplphysiol.00895.2004
Bleiler C., Castañeda P. P., Roehrle O. (2019). A microstructurally-based, multi-scale, continuum-mechanical model for the passive behaviour of skeletal muscle tissue. J. Mech. Behav. Biomed. Mat. 97, 171–186. doi:10.1016/j.jmbbm.2019.05.012
Brigatto F. A., Braz T. V., da Costa Zanini T. C., Germano M. D., Aoki M. S., Schoenfeld B. J., et al. (2019). Effect of resistance training frequency on neuromuscular performance and muscle morphology after 8 weeks in trained men. J. Strength Cond. Res. 33, 2104–2116. doi:10.1519/JSC.0000000000002563
Calvo B., Ramírez A., Alonso A., Grasa J., Soteras F., Osta R., et al. (2010). Passive nonlinear elastic behaviour of skeletal muscle: Experimental results and model formulation. J. Biomech. 43, 318–325. doi:10.1016/j.jbiomech.2009.08.032
Damas F., Phillips S., Vechin F. C., Ugrinowitsch C. (2015). A review of resistance training-induced changes in skeletal muscle protein synthesis and their contribution to hypertrophy. Sports Med. 45, 801–807. doi:10.1007/s40279-015-0320-0
Damas F., Phillips S. M., Lixandrão M. E., Vechin F. C., Libardi C. A., Roschel H., et al. (2016). Early resistance training-induced increases in muscle cross-sectional area are concomitant with edema-induced muscle swelling. Eur. J. Appl. Physiol. 116, 49–56. doi:10.1007/s00421-015-3243-4
De Deyne P. G. (2001). Application of passive stretch and its implications for muscle fibers. Phys. Ther. 81, 819–827. doi:10.1093/ptj/81.2.819
DeFreitas J. M., Beck T. W., Stock M. S., Dillon M. A., Kasishke P. R. (2011). An examination of the time course of training-induced skeletal muscle hypertrophy. Eur. J. Appl. Physiol. 111, 2785–2790. doi:10.1007/s00421-011-1905-4
Figueiredo V. C., de Salles B. F., Trajano G. S. (2018). Volume for muscle hypertrophy and health outcomes: The most effective variable in resistance training. Sports Med. 48, 499–505. doi:10.1007/s40279-017-0793-0
Flitney F., Hirst D. (1978). Cross-bridge detachment and sarcomere ‘give’ during stretch of active frog’s muscle. J. Physiol. 276, 449–465. doi:10.1113/jphysiol.1978.sp012246
Gautel M. (2008). “The sarcomere and skeletal muscle disease,” in The sarcomere and the nucleus: Functional links to hypertrophy, atrophy and sarcopenia (New York: Springer). chap. 13. 176–191.
Gentil P., Fischer B., Martorelli A., Lima R., Bottaro M. (2015). Effects of equal-volume resistance training performed one or two times a week in upper body muscle size and strength of untrained young men. J. Sports Med. Phys. Fit. 55, 144–149.
Göktepe S., Abilez O. J., Kuhl E. (2010). A generic approach towards finite growth with examples of athlete’s heart, cardiac dilation, and cardiac wall thickening. J. Mech. Phys. Solids 58, 1661–1680. doi:10.1016/j.jmps.2010.07.003
Goldspink G. (1970). The proliferation of myofibrils during muscle fibre growth. J. Cell Sci. 6, 593–603. doi:10.1242/jcs.6.2.593
Gordon A., Huxley A. F., Julian F. (1966). The variation in isometric tension with sarcomere length in vertebrate muscle fibres. J. Physiol. 184, 170–192. doi:10.1113/jphysiol.1966.sp007909
Grasa J., Ramirez A., Osta R., Munoz M. J., Soteras F., Calvo B. (2011). A 3D active-passive numerical skeletal muscle model incorporating initial tissue strains. Validation with experimental results on rat tibialis anterior muscle. Biomech. Model. Mechanobiol. 10, 779–787. doi:10.1007/s10237-010-0273-z
Grasa J., Hernández-Gascón B., Ramírez A., Rodríguez J. F., Calvo B. (2012). Modelado numérico del comportamiento del tejido músculo-esquelético. Rev. Int. Métodos Numéricos Cálculo Diseño Ing. 28, 177–186. doi:10.1016/j.rimni.2012.03.005
Grgic J., Schoenfeld B. J., Latella C. (2019). Resistance training frequency and skeletal muscle hypertrophy: A review of available evidence. J. Sci. Med. Sport 22, 361–370. doi:10.1016/j.jsams.2018.09.223
Hanson J., Huxley H. E. (1953). Structural basis of the cross-striations in muscle. Nature 172, 530–532. doi:10.1038/172530b0
Hay N., Sonenberg N. (2004). Upstream and downstream of mTOR. Genes Dev. 18, 1926–1945. doi:10.1101/gad.1212704
Heidlauf T., Klotz T., Rode C., Siebert T., Röhrle O. (2017). A continuum-mechanical skeletal muscle model including actin-titin interaction predicts stable contractions on the descending limb of the force-length relation. PLoS Comput. Biol. 13, e1005773. doi:10.1371/journal.pcbi.1005773
Hernández-Gascón B., Grasa J., Calvo B., Rodríguez J. (2013). A 3d electro-mechanical continuum model for simulating skeletal muscle contraction. J. Theor. Biol. 335, 108–118. doi:10.1016/j.jtbi.2013.06.029
Holzapfel G. A. (2000). Nonlinear solid mechanics: A continuum approach for engineering. Chichester: Wiley.
Huxley H., Hanson J. (1959). The structural basis of the contraction mechanism in striated muscle. Ann. N. Y. Acad. Sci. 81, 403–408. doi:10.1111/j.1749-6632.1959.tb49323.x
Huxley H. (1957). The double array of filaments in cross-striated muscle. J. Biophys. Biochem. Cytol. 3, 631–648. doi:10.1083/jcb.3.5.631
Huxley A. (2000). Cross-bridge action: Present views, prospects, and unknowns. J. Biomech. 33, 1189–1195. doi:10.1016/s0021-9290(00)00060-9
Jorgenson K. W., Phillips S. M., Hornberger T. A. (2020). Identifying the structural adaptations that drive the mechanical load-induced growth of skeletal muscle: A scoping review. Cells 9, 1658. doi:10.3390/cells9071658
Kraus W. E., Torgan C. E., Taylor D. A. (1994). Skeletal muscle adaptation to chronic low-frequency motor nerve stimulation. Exerc. Sport Sci. Rev. 22, 313–360. doi:10.1249/00003677-199401000-00014
Lee E. H. (1969). Elastic-plastic deformation at finite strains. J. Appl. Mech. 36, 1–6. doi:10.1115/1.3564580
Léger B., Cartoni R., Praz M., Lamon S., Dériaz O., Crettenand A., et al. (2006). Akt signalling through GSK-3beta, mTOR and Foxo1 is involved in human skeletal muscle hypertrophy and atrophy. J. Physiol. 576, 923–933. doi:10.1113/jphysiol.2006.116715
Martins J., Pires E., Salvado R., Dinis P. (1998). A numerical model of passive and active behavior of skeletal muscles. Comput. methods Appl. Mech. Eng. 151, 419–433. doi:10.1016/s0045-7825(97)00162-x
Matthews B. H. (1931). The response of a muscle spindle during active contraction of a muscle. J. Physiol. 72, 153–174. doi:10.1113/jphysiol.1931.sp002768
Miller M. S., Bedrin N. G., Ades P. A., Palmer B. M., Toth M. J. (2015). Molecular determinants of force production in human skeletal muscle fibers: Effects of myosin isoform expression and cross-sectional area. Am. J. Physiol. Cell Physiol. 308, C473–C484. doi:10.1152/ajpcell.00158.2014
Morrow D. A., Donahue T. L. H., Odegard G. M., Kaufman K. R. (2010). Transversely isotropic tensile material properties of skeletal muscle tissue. J. Mech. Behav. Biomed. Mat. 3, 124–129. doi:10.1016/j.jmbbm.2009.03.004
Phillips S., Parise G., Roy B., Tipton K., Wolfe R., Tarnopolsky M. (2002). Resistance-training-induced adaptations in skeletal muscle protein turnover in the fed state. Can. J. Physiol. Pharmacol. 80, 1045–1053. doi:10.1139/y02-134
Pollack G. H. (1983). The cross-bridge theory. Physiol. Rev. 63, 1049–1113. doi:10.1152/physrev.1983.63.3.1049
Ramírez A., Grasa J., Alonso A., Soteras F., Osta R., Muñoz M., et al. (2010). Active response of skeletal muscle: In vivo experimental results and model formulation. J. Theor. Biol. 267, 546–553. doi:10.1016/j.jtbi.2010.09.018
Randall D., Burggren W., French K. (2002). ckert animal physiology: Mechanisms and adaptations. New York, NY: WH Freeman.
Rodriguez E. K., Hoger A., McCulloch A. D. (1994). Stress-dependent finite growth in soft elastic tissues. J. Biomech. 27, 455–467. doi:10.1016/0021-9290(94)90021-3
Rui Y., Bai J., Perrimon N. (2010). Sarcomere formation occurs by the assembly of multiple latent protein complexes. PLoS Genet. 6, e1001208. doi:10.1371/journal.pgen.1001208
Sandri M. (2008). Signaling in muscle atrophy and hypertrophy. Physiology 23, 160–170. doi:10.1152/physiol.00041.2007
Schiaffino S., Mammucari C. (2011). Regulation of skeletal muscle growth by the igf1-akt/pkb pathway: Insights from genetic models. Skelet. Muscle 1, 4. doi:10.1186/2044-5040-1-4
Schoenfeld B. J., Ogborn D., Krieger J. W. (2017). Dose-response relationship between weekly resistance training volume and increases in muscle mass: A systematic review and meta-analysis. J. Sports Sci. 35, 1073–1082. doi:10.1080/02640414.2016.1210197
Seynnes O. R., Boer M. D., Narici M. V. (2007). Early skeletal muscle hypertrophy and architectural changes in response to high-intensity resistance training. J. Appl. Physiol. 368, 368–373. doi:10.1152/japplphysiol.00789.2006
Song G., Ouyang G., Bao S. (2005). The activation of Akt/PKB signaling pathway and cell survival. J. Cell. Mol. Med. 9, 59–71. doi:10.1111/j.1582-4934.2005.tb00337.x
Stålhand J., Klarbring A., Holzapfel G. A. (2008). Smooth muscle contraction : Mechanochemical formulation for homogeneous finite strains. Prog. Biophys. Mol. Biol. 96, 465–481. doi:10.1016/j.pbiomolbio.2007.07.025
Stålhand J., Klarbring A., Holzapfel G. A. (2011). A mechanochemical 3d continuum model for smooth muscle contraction under finite strains. J. Theor. Biol. 268, 120–130. doi:10.1016/j.jtbi.2010.10.008
Stephenson D., Williams D. (1982). Effects of sarcomere length on the force—Pca relation in fast-and slow-twitch skinned muscle fibres from the rat. J. Physiol. 333, 637–653. doi:10.1113/jphysiol.1982.sp014473
Stock M. S., Mota J. A., DeFranco R. N., Grue K. A., Jacobo A. U., Chung E., et al. (2017). The time course of short-term hypertrophy in the absence of eccentric muscle damage. Eur. J. Appl. Physiol. 117, 989–1004. doi:10.1007/s00421-017-3587-z
Takaza M., Moerman K. M., Gindre J., Lyons G., Simms C. K. (2013). The anisotropic mechanical behaviour of passive skeletal muscle tissue subjected to large tensile strain. J. Mech. Behav. Biomed. Mat. 17, 209–220. doi:10.1016/j.jmbbm.2012.09.001
Van Loocke M., Lyons C., Simms C. (2008). Viscoelastic properties of passive skeletal muscle in compression: Stress-relaxation behaviour and constitutive modelling. J. Biomech. 41, 1555–1566. doi:10.1016/j.jbiomech.2008.02.007
Villota-Narvaez Y., Garzon-Alvarado D. A., Ramirez-Martinez A. M. (2021). A dynamical system for the igf1-akt signaling pathway in skeletal muscle adaptation. Biosystems. 202, 104355. doi:10.1016/j.biosystems.2021.104355
Wang Y., Zhou Y., Graves D. T., Wang Y., Zhou Y., Graves D. T. (2014). FOXO transcription factors: Their clinical significance and regulation. Biomed. Res. Int. 2014, 925350. doi:10.1155/2014/925350
Keywords: dynamical systems, population dynamics, cellular signaling pathways, biochemical modeling, muscle adaptation, mechanobiology, biomechanics
Citation: Villota-Narvaez Y, Garzón-Alvarado DA, Röhrle O and Ramírez-Martínez AM (2022) Multi-scale mechanobiological model for skeletal muscle hypertrophy. Front. Physiol. 13:899784. doi: 10.3389/fphys.2022.899784
Received: 19 March 2022; Accepted: 23 August 2022;
Published: 06 October 2022.
Edited by:
Paul M. L. Janssen, The Ohio State University, United StatesReviewed by:
Sandra Shefelbine, Northeastern University, United StatesCopyright © 2022 Villota-Narvaez, Garzón-Alvarado, Röhrle and Ramírez-Martínez. This is an open-access article distributed under the terms of the Creative Commons Attribution License (CC BY). The use, distribution or reproduction in other forums is permitted, provided the original author(s) and the copyright owner(s) are credited and that the original publication in this journal is cited, in accordance with accepted academic practice. No use, distribution or reproduction is permitted which does not comply with these terms.
*Correspondence: Yesid Villota-Narvaez , eWF2aWxsb3RhbkB1bmFsLmVkdS5jbw==
Disclaimer: All claims expressed in this article are solely those of the authors and do not necessarily represent those of their affiliated organizations, or those of the publisher, the editors and the reviewers. Any product that may be evaluated in this article or claim that may be made by its manufacturer is not guaranteed or endorsed by the publisher.
Research integrity at Frontiers
Learn more about the work of our research integrity team to safeguard the quality of each article we publish.