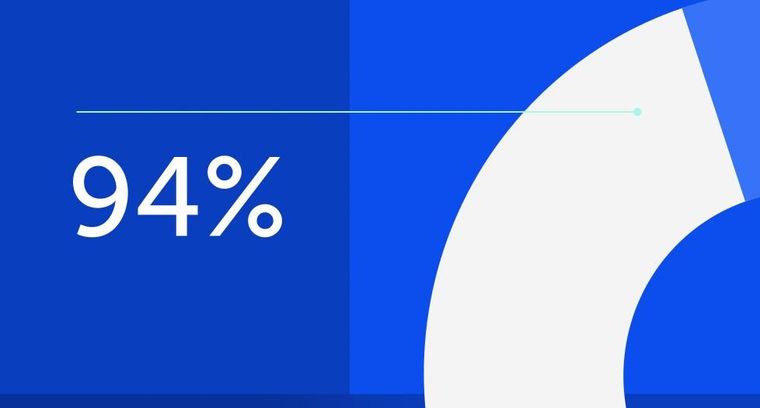
94% of researchers rate our articles as excellent or good
Learn more about the work of our research integrity team to safeguard the quality of each article we publish.
Find out more
METHODS article
Front. Physiol., 20 June 2022
Sec. Developmental Physiology
Volume 13 - 2022 | https://doi.org/10.3389/fphys.2022.881929
This article is part of the Research TopicAdvances in Techniques for Measurement and Assessment of Physiological Processes in Developing AnimalsView all 9 articles
Mechanical forces from blood flow and pressure (hemodynamic forces) contribute to the formation and shaping of the blood vascular network during embryonic development. Previous studies have demonstrated that hemodynamic forces regulate signaling and gene expression in endothelial cells that line the inner surface of vascular tubes, thereby modifying their cellular state and behavior. Given its important role in vascular development, we still know very little about the quantitative aspects of hemodynamics that endothelial cells experience due to the difficulty in measuring forces in vivo. In this study, we sought to determine the magnitude of wall shear stress (WSS) exerted on ECs by blood flow in different vessel types and how it evolves during development. Utilizing the zebrafish as a vertebrate model system, we have established a semi-automated high-throughput fluorescent imaging system to capture the flow of red blood cells in an entire zebrafish between 2- and 6-day post-fertilization (dpf). This system is capable of imaging up to 50 zebrafish at a time. A semi-automated analysis method was developed to calculate WSS in zebrafish trunk vessels. This was achieved by measuring red blood cell flow using particle tracking velocimetry analysis, generating a custom-made script to measure lumen diameter, and measuring local tube hematocrit levels to calculate the effective blood viscosity at each developmental stage. With this methodology, we were able to determine WSS magnitude in different vessels at different stages of embryonic and larvae growth and identified developmental changes in WSS, with absolute levels of peak WSS in all vessel types falling to levels below 0.3 Pa at 6 dpf. Additionally, we discovered that zebrafish display an anterior-to-posterior trend in WSS at each developmental stage.
The establishment of a blood circulatory system is essential for the efficient transport of oxygen, metabolites and cells to all tissues of the body. Blood is pumped by the heart under high pressure and distributed through a network of tubular blood vessels comprised of arteries, capillaries and veins. The flow of viscous blood inflicts different mechanical stresses (hemodynamic forces) on endothelial cells (ECs) lining the inner surface of blood vessels. Blood flow imparts fluid shear stress, which is the tangential frictional force per unit area on ECs, while blood pressure exerts a normal force that compresses the EC apical surface (Dessalles et al., 2021). Besides driving the exchange of gases and solutes between the endothelium and surrounding tissues, the mechanical forces of blood have fundamental roles in the initial development of blood vessels and their subsequent remodeling by modulating EC behaviors through the mechanical forces imparted. During angiogenesis, pressurized blood flow is required for the formation of new vascular sprouts in certain vascular beds (Nicoli et al., 2010; Goetz et al., 2014; Ghaffari et al., 2015), the expansion and fusion of apical membranes in vessels undergoing transcellular lumen formation (Lenard et al., 2013; Gebala et al., 2016) and in maintaining vessel diameter (Bussmann et al., 2011; Baeyens et al., 2015; Nakajima et al., 2017). After the formation of a primitive vascular plexus, hemodynamics further regulate vessel pruning (Chen et al., 2012; Kochhan et al., 2013; Lenard et al., 2015) and vessel diameter (Udan et al., 2013; Sugden et al., 2017) to remodel the primitive vascular plexus into a hierarchical network of larger arteries and veins and smaller capillaries with optimal connections. Haemodynamic forces therefore have a major influence on the generation and shaping of the vascular tree (reviewed in Campinho et al., 2020; Phng and Belting, 2021). However, little is known about the magnitude of hemodynamic forces ECs are exposed to and how they vary spatiotemporally within a vascular network during different stages of development and growth.
In this study, we sought to determine how hemodynamics evolve during blood vessel development and remodeling using the zebrafish as a vertebrate model system. The zebrafish offers many advantages for several reasons: many eggs can be obtained from a single female zebrafish, eggs are rapidly and externally fertilized, zebrafish in early developmental stages are optically transparent and transgenic lines with specific fluorescently labelled cellular compartments exist (Streisinger et al., 1981; Weinstein, 2002). These features therefore permit live observation of many zebrafish throughout development. However, in practice, only a small fraction of zebrafish is analyzed due to the time-consuming and laborious nature of handling zebrafish specimens. As such, in previous studies that have investigated blood flow, the sample size of zebrafish analyzed has been small, ranging from 1 to 21 zebrafish and limited to a few vessel types and developmental stages (Malone et al., 2007; Chen et al., 2011; Watkins et al., 2012; Anton et al., 2013; Choi et al., 2017; Sugden et al., 2017; Follain et al., 2018; Santoso et al., 2019). Therefore, to reliably determine hemodynamic quantities over development, we aimed to increase the number of zebrafish that can be imaged and analyzed from 2 to 6 dpf. To achieve this, we established a semi-automated high-throughput imaging system to image red blood cell (RBC) flow in the entire zebrafish and an analysis pipeline to calculate RBC flow speed, lumen diameter, hematocrit, blood viscosity, pseudo shear rate, and wall shear stress in multiple vessel types of the zebrafish trunk.
Zebrafish (Danio rerio) were raised and staged according to established protocols (Kimmel et al., 1995). Red blood cells were visualized using the transgenic line, Tg(gata1:dsRed)sd2 (Traver et al., 2003). Endothelial cells were visualized using Tg(kdrl:EGFP)s843 (Jin et al., 2005). 0–6 days post-fertilization (dpf) zebrafish were maintained at 28°C in E3 medium containing 0.003% phenylthiourea to inhibit melanogenesis. For imaging, 2–6 dpf zebrafish were anaesthetized in E3 medium containing 0.16 mg/ml Tricaine (Sigma-Aldrich). An agarose-based imaging chamber consisting of six grooved-lanes (similar to a microinjection plate) was used to mount dechorionated zebrafish. Zebrafish were placed in the lanes and fixed at their positions by pipetting 1% low-melting agarose (Bio-Rad) containing 0.16 mg/ml Tricaine until zebrafish and grooves were entirely covered and filled, respectively. The zebrafish were quickly aligned and positioned on their sides before the low-melting agarose has set. Once set, the mounting chamber was covered with E3 medium containing 0.16 mg/ml Tricaine.
We built a semi-automated, high-throughput imaging system consisting of an imaging chamber capable of mounting 50 zebrafish at a time, a robotic XY-stage scanning over 50 × 50 mm2 area, and a high-performance sCMOS camera (PCO, pco.edge 4.2 CL) mounted on a fluorescent stereomicroscope (Leica, M205FA). The sCMOS camera can capture images at 100 frames per second (fps) at 2048 x 2048 pixels full resolution. A stage-top incubator (Live Cell Instrument, Chamlide) was used to keep 2–6 dpf zebrafish at 27–28°C. We synchronized the robotic stages with the sCMOS camera to image many zebrafish for each stage movement. The positions of the zebrafish in the sample mounting chamber were pre-registered manually before imaging. Two actuators that were connected to each stage and controlled by a multi-dimensional acquisition option at the Micro-Manager were set up to move to the pre-registered positions throughout whole scanning area. The smallest distance that can be moved by the actuator was 0.0476 µm. A TTL (Transistor Transistor Logic) signal triggered the sCMOS camera with a time delay of half a second in response to a typical pulse waveform generated at the movement of the actuator. After the completion of each stage movement, a stack of 1,000 images per zebrafish was acquired at the frame rate of 100–180 fps and stored in an automatically generated folder. The size of one image stack was ∼1.3 GB.
RBC flow was imaged for 1,000 frames at 180 fps for zebrafish at 2 and 3 dpf while lower imaging rates of 100 and 120 fps were employed for zebrafish at 4, 5, and 6 dpf. Lower imaging rates were used for the later stages (4–6 dpf) to achieve longer observation time to capture sufficient network flow while maintaining manageable data volume as the RBCs flowed significantly slower than at 2 and 3 dpf stages.
We used the linear motion tracker in Image J software plug-in TrackMate (Tinevez et al., 2017) for particle tracking velocimetry (PTV) analysis. In order to obtain RBC velocities, the linear motion tracker algorithm performs pairing identification of the same RBC across two or more image frames. Newly appearing RBCs are paired with their previous position by searching for unpaired candidates from the previous image frame within an initial search radius (ISR). Previously tracked RBCs are paired with their new position using the expected position given by the previously predicted velocity, the highest correlated candidate for pairing is searched for within the maximum search radius (MSR) around that expected position. We optimized the tracker with an ISR of 25 µm and a MSR of 10 µm for extracting reliable trajectories from 1,000 images per zebrafish (Supplementary Videos S1–S5).
The TrackMate software for measuring RBC flow speeds was evaluated to be comparable with manual tracking of RBC trajectories. These validation results are shown in Supplementary Figure S1, where a single RBC was followed along its trajectory using both TrackMate and manual tracking (Supplementay Figure S1A). The graph of RBC speed against time obtained via both methods showed good correspondence between the two approaches (Supplementary Figure S1B), thus indicating the robustness of the TrackMate algorithm for RBC speed measurement even under high hematocrit conditions.
First, all zebrafish images were aligned such that the anterior to posterior (head to tail) axis was horizontally oriented from left to right while the ventral to dorsal axis was vertically oriented from bottom to top of the image. RBC trajectories from TrackMate were separated for vessel type according to the overall track direction (straight line path from the full trajectory of an RBC from start point to end point in the image time series). The dorsal longitudinal anastomotic vessel (DLAV) segments were identified by their dorsal location in the image and the rightward or leftward travelling track directions of RBCs. In the mid dorsal-ventral region of the image, rightwards travelling tracks corresponded to the dorsal aorta/caudal artery (DA/CA) segments while leftwards travelling tracks were assigned as posterior cardinal vein/caudal vein (PCV/CV) segments. Upwards travelling tracks were recognized as arterial intersegmental vessel (aISV) segments and downwards travelling tracks were assigned as venous intersegmental vessel (vISV) segments.
Using this assignment, we generated preliminary masks for each vessel type by stacking the corresponding TrackMate-identified RBC spots across the 1,000 time series images (Figures 1A,Bi). These masks were then skeletonized to provide skeletal connectivity maps of the vessel types (Figures 1Bii,iii). Next, we filtered the vessel-type-assigned data for quality and topological uniqueness. As our imaging technique superimposes data from multiple focal depth into a two-dimensional projection, vessel lumen diameter was impossible to measure when multiple regions merged in the projection to provide a combined luminal width. Hence, we rejected data from vessel locations where the skeletal maps coincided as this indicated topological ambiguity. This rejection criteria applied to regions where the ISVs joined with the DA/CA, PCV/CV or DLAV (* regions in Figure 1Biv) and most often in ISV locations where the imaging captured ISVs located on a higher focal plane running across or positioned very close to ISVs on a lower focal plane (** regions in Figure 1Biv). Finally, the topologically unique vessel segments were fitted to splines and smoothed with a Savitzky-Golay filter to determine spline coordinates (
where
FIGURE 1. Schematic diagrams of methods used for automated vessel labeling, data filtering, and vessel diameter and hematocrit calculation. (A) High speed image sequence of dsRed intensity was the only collected data from experiments. (B) Series of steps using TrackMate data (i–iii) to obtain vessel spline segment points and directions
At vessel segments where the SG fit was poor (
where
Next, the lumen diameter (
where
A key consideration in our wall shear stress (WSS) calculation is the RBC phase contribution to apparent blood viscosity (
where
where
For calculating WSS we used the Hagen–Poiseuille formulation with the assumption of parabolic blood flow velocity profile in the lumen cross-section. Using the apparent blood viscosity (
From the high-speed image acquisition of RBC flow, we obtained the RBC flow velocity (
FIGURE 2. Series of script-automated steps to obtain peak (systolic) RBC velocity from TrackMate data, demonstrated using data from zebrafish 28 of the 2 dpf data set. (A) Velocity sampling within spatial bins of controlled intervals in different vessel types: shown in bold line black boxes are the 60-µm intervals for (i) a region in the anterior DA/CA, (ii) a region in the posterior DA/CA and ventral-to-dorsal intervals of filtered data (see text) for aISVs at the (iii) anterior and (iv) posterior regions of the trunk vasculature. (B) Example of TrackMate particle tracking algorithm performed in the anterior DA/CA region (i) shown in (A). (C) Contiguous velocity against time curves and the application of peak scanning and outlier filtering to obtain the average peak (systolic) velocities in regions i, ii, iii and iv shown in (A). (D) The anterior-to-posterior distribution of average peak velocities in the DA/CA (left) and aISVs (right) with the values for the four locations (i–iv) highlighted in (A,C) shown in boxes.
It is important to note that the apparent viscosity of blood (
In addition to the WSS, we also calculated the peak pseudo shear rate
Unlike the WSS, the
In order to standardize the anterior to posterior (AP) trend comparison between zebrafish of varying sizes in our experiment, the AP axis was normalized for all our observations. The normalized AP coordinate,
To measure the temporal fluctuation of RBC flow velocity (and its WSS derivative) at various positions in the vascular network, we had to optimize a spatial sampling window that was large enough to contain a sufficient number of tracked RBCs in all image frame sequences. When the spatial sampling window was too narrow then a contiguous velocity fluctuation against time signal could not be constructed for that particular spatial position. We found that for DA/CA and PCV/CV, sampling windows of 60 µm intervals provided good velocity against time signals for analysis. Figures 2Ai,ii show two such intervals in the DA/CA taken at anterior and posterior locations and the resulting velocity against time signals are shown in Figure 2Ci,ii. In the DLAV, each interval was the available segment (after rejection discussed in Section 3.2) between two co-parallel ISVs. In aISVs and vISVs, we took available ISV segments (after rejection for poor imaging quality and topological non-uniqueness discussed in Section 3.2) separated by the DLAV segments as the sampling window. Figures 2Aiii,2Aiv show two such intervals for the aISVs and the resulting velocity against time signals are shown in Figures 2Ciii,iv. The aISVs show less regularity in the RBC velocity pulsation as compared to the DA/CA due to the sporadic nature of RBC flow into these vessels, unlike the DA/CA which is the major conduit for the RBC flow. Due to the regularity of the velocity pulsation in the anterior region of the DA/CA (Figure 2Ci), the velocity pulsation frequency was used to calculate the heartbeat in the zebrafish (heartbeats shown in Supplementary Figure S3). We validated this assumption in validation experiments detailed in methods Section 3.5.
From the temporal signals of velocity fluctuations at each spatial window, we identified the signal peaks in velocities (green circles in Figure 2C) and removed outlier peaks (peaks larger or smaller than the average peak by one standard deviation, shown by the dashed lines in Figure 2C) assuming that they were noise-contributed errors in the particle tracking algorithm. Finally, we applied ensemble averaging of the filtered peak velocities (red circles in Figure 2C) and took that value to be the representative peak velocity of RBC flow at that particular AP location. Figure 2D shows graphs of the average peak velocity against
We performed an additional set of experiments using the double transgenic zebrafish line, Tg(gata1:dsRed);Tg(kdrl:EGFP), where RBCs and ECs, respectively, can be simultaneously visualized. For this, we examined seven zebrafish at 2 dpf with varying degrees of hematocrit by injecting 1 nl of 0.1 mM Gata1 morpholino (Galloway et al., 2005). As the endothelial EGFP signal was not sharp under whole-zebrafish imaging conditions, we had to focus on the mid-trunk to tail region to increase the pixel resolution of the resulting image in the validation experiment (× 80 magnification) as compared to the main experiment (× 40). As presented in Supplementary Figure S4, two zebrafish injected with control morpholino (fish C1 and C2 in Supplementary Figures S4A,B and Supplementary Videos S6, S7) displayed typical levels of hematocrit. Among the Gata1 morphants, 3 zebrafish had moderate levels of hematocrit reduction (fish M1, M2, M3 in Supplementary Figures S4C–E and Supplementary Videos S8–S10) and 2 zebrafish had almost vanishing hematocrit levels (fish M4 and M5 in Supplementary Figures S4F,G and Supplementary Videos S11, S12). Compared against manual counting of RBCs, our method of using the average intensity correlation corroborated the trend of decreasing hematocrit in the DA/CA, PCV/CV and ISV (Supplementary Figures S4H–J) across the three hematocrit group ranges. Both approaches showed qualitative trend of decreasing hematocrit.
Using the same seven fish, we examined lumen diameters using the method in Section 3.2 and compared its results against a peak-to-peak distance approach using endothelial EGFP signal. Compared to diameters measured using the endothelial marker, diameters obtained using the method in 3.2 had average discrepancy levels of ± 17% in the CA, ± 11% in the CV and ± 22% in the smaller vessels like the ISVs (Supplementary Figure S5). We noted that the discrepancy tended to increase for smaller vessels and for vessels with very low local hematocrit (<0.01). Graphs of the dsRed maximum projection and super-gaussian fitting performed for this data set can be seen in Supplementary Figures S6–S8.
Validation of the heartbeat measurement was performed using zebrafish C2. We compared the velocity pulsation frequency at the anterior region of the DA (Supplementary Figures S9Ai,ii) against the heart wall displacement cycle (Supplementary Figures S9Bi,ii). Both methods indicated the same 180 bpm for zebrafish C2, thus indicating the direct correlation between velocity pulsation frequency in the DA and the zebrafish heartbeat.
WSS is linearly proportional to blood velocity and viscosity but inversely proportional to the lumen diameter (Eq. 12). Using this relation, we sought to acquire coherent appreciation of the microhemodynamics at play and the developmental trends of these related quantities were analyzed in tandem for each vessel type.
We plotted the colorized magnitudes of hemodynamic quantities with respect to the spatial bins (defined in Section 3.4) for each vessel type in zebrafish 28 from the 2 dpf data set (Figure 3A) to provide a spatial distribution map of quantity levels in the zebrafish trunk network. There was a reduction in peak blood velocity towards the tail of the zebrafish in the main vessels (DA/CA and PCV/CV) and in the aISVs closer towards the tail (Figure 3B). PCV/CV showed reduction in diameters towards the tail while the DA/CA showed a similar but milder trend (Figure 3C). ISVs and DLAV segments were significantly smaller in diameter than the main vessels (Figure 3C) and a lower discharge hematocrit (RBC flow concentration) was observed in these smaller vessel types (Figure 3D). As a result of their lower discharge hematocrit, local viscosity of blood in the ISVs and DLAV was lower than the blood viscosity in main axial vessels (Figure 3E). The peak WSS trend in the DA/CA and aISVs showed a reduction in levels for vessel segments closer to the tail (Figure 3F), which closely correlated with the peak velocity trends for the two respective vessel types (Figure 3B). Interestingly, the peak WSS for PCV/CV showed a slight increase in levels accompanied by increasing spatial fluctuation in levels towards the tail (Figure 3F) despite falling peak velocities tailward in this vessel type (Figure 3B)—this was likely due to the significant diameter reduction tailward and the increasing spatial fluctuations of diameter in the CVP region (Figure 3C).
FIGURE 3. Spatial distribution map of data in zebrafish 28 of the 2 dpf data set after automated spatial bin averaging: (A) vessel type, (B) average velocity peak (
The trends in zebrafish 28 show the causal relationships between lumen diameter and discharge hematocrit; discharge hematocrit and blood viscosity; blood flow velocity, lumen diameter and WSS. However, since biological variations among zebrafish can be large (as shown in the individual zebrafish trends for hemodynamic quantities in Supplementary Figures S10–S34), the trends described for a single zebrafish may not be representative for an entire population. Hence, in order to study the developmental and spatial trends in the early development of zebrafish, we pooled the data from zebrafish at each stage of development. At 2, 3, 4, 5, and 6 dpf, we pooled data from 30, 32, 38, 35, and 29 zebrafish, respectively, for the spatial analysis of vessel morphology and hemodynamic trends (Supplementary Figure S35). Different zebrafish were used at each developmental stage.
Two trend categories were summarized for all hemodynamic quantities analyzed. The first was the changes in magnitudes of quantities in each vessel type for zebrafish across developmental stages at four anterior-to-posterior (AP) group locations (Figures 4Ai–8Ai,Bi,Ci,Di,Ei,Fi) where the data was pooled along the AP axis: AP1 (pooling within 0.2 ≤
FIGURE 4. Developmental trends of morphological and hemodynamic quantities in the dorsal aorta/caudal artery (DA/CA). (A) The changes in
FIGURE 5. Developmental trends of morphological and hemodynamic quantities in the posterior cardinal vein/caudal vein plexus (PCV/CVP). (A) The changes in
FIGURE 6. Developmental trends of morphological and hemodynamic quantities in the arterial intersegmental vessels (aISVs). (A) The changes in
FIGURE 7. Developmental trends of morphological and hemodynamic quantities in the venous intersegmental vessels (vISVs). (A) The changes in
FIGURE 8. Developmental trends of morphological and hemodynamic quantities in the dorsal longitudinal anastomotic vessel (DLAV). (A) The changes in
Developmental trends for hemodynamic quantity levels in DA/CA showed a general reduction in median peak velocity (
Zebrafish also showed decreases in median diameter (
In terms of spatial trends, we observed a consistent negative AP trend of decreasing quantity levels in the DA/CA for peak velocity (
Developmental trends for hemodynamic quantity levels in PCV/CV were generally similar to those observed in the DA/CA. Results showed a general reduction in median
Zebrafish showed decreases in median
With regards to spatial distribution trends in the PCV/CV, we observed consistent negative AP gradients for
Median levels of
One developmental trend distinctly different than those from DA/CA and PCV/CV was the diameter evolution. Zebrafish showed rising
For spatial trends in quantity distribution, we observed consistent negative AP gradients in the aISVs for
Results for the vISVs showed a general reduction in median levels of
Zebrafish showed a sharply rising median levels of
For spatial trends in quantity distribution, we observed negative AP gradients in the vISVs for
DLAV segments exhibited a general reduction in median
Diameters exhibited a dynamic trend that was oscillatory in all four AP regions in the DLAV segments and there was no clear directionality in the developmental trend for median levels of
Spatial trends for DLAV segments saw negative AP gradients for
The
We summarized the changes in AP gradients across development time in Figure 9 for quantities (A)
FIGURE 9. Developmental patterns of the anterior-to-posterior (AP) gradients in morphological and hemodynamic quantities in the zebrafish trunk vasculature. Circles indicate the hemodynamic AP gradient obtained from linear regression fitting of the pooled AP data, whisker bars indicate the standard error of the regression and * symbols denote statistically significant gradients from the slope T-test (p < 0.05). (A) AP gradients for
For the case of peak WSS comparison between vessel types, we observed that the median levels of
FIGURE 10. Developmental changes in magnitude levels of shear rate related quantities. The
In this study, we have developed a high-throughput protocol to image and analyze blood flow in order to estimate systolic wall shear stress (WSS) in the zebrafish trunk network. The aim of our study was to investigate the spatial and developmental trends in WSS distribution and vascular diameters in a developing microvascular network using the zebrafish trunk network as our animal model. To achieve the high-throughput imaging, we implemented a semi-automated zebrafish mounting and imaging protocol that can image RBC flow up to 50 zebrafish in one imaging sequence. We imaged zebrafish at 2, 3, 4, 5, and 6 days post-fertilization (dpf) for spatial and developmental trends in hemodynamics. To achieve high-throughput data analysis, lumen diameter, hemodynamic quantity calculation and data filtering were automated using our custom-written Python and C scripts. A key point to note is that our approach required only fluorescent labelling of RBCs and evaluation of their trajectories from the imaging to perform all the analyses in our study.
One limitation with our current protocol was the low imaging resolution, which was 1.625 µm for 2 dpf images and 1.85 µm for 3 dpf onwards. This setting was a consequence of prioritizing whole zebrafish imaging over high-resolution imaging in order to facilitate high-throughput imaging protocol since our robotic stage for ROI scanning required manual pre-registration of the imaging positions (which we aimed to minimize) on the mounting chamber. Further improvement to the protocol can be achieved with higher automation in the ROI registration process and image post-processing in order to obtain higher resolution images of the zebrafish trunk vasculature. We found that the levels of changes in median
With regards to our WSS calculation, a key component is the evaluation of the apparent blood viscosity in micron-sized vessels (Eqs 13–17). In this respect, it is important to consider the Fåhræus–Lindqvist (FL) effect that states the dependence of apparent blood viscosity on the holding vessel inner diameter for micron-sized vessels (Pries et al., 1992). Specifically, the apparent blood viscosity reduces with diameter reduction for microvessels with diameters below 300 µm before the trend inverts to sharp increases in viscosity with further diameter reduction at capillary scales (3–10 µm). The inversion point for this biphasic trend is dependent on the vessel hematocrit but for most of the diameter range below 300 µm, the blood viscosity reduces in response to vessel diameter reduction (Supplementary Figure S2). Mechanistically, the FL effect is due to the increasing prominence of the hydrodynamics of the cell-free plasma layer (CFL) as vessel sizes reduce. The CFL provides a lubricating buffer for RBC-flow in the center of the lumen and progressively increases in effective lubrication as its relative size increases as vessels reduce in diameter (Pries and Secomb, 2005; Fedosov et al., 2010). The FL trend inversion occurs when further lumen reductions in capillary-sized vessels diminishes the CFL to the extent that flowing RBCs now experience higher frequency of frictional contact with vessel walls, thus heightening apparent blood viscosity. To the best of our knowledge no study has measured zebrafish blood viscosity using microfluidic devices with geometries in 5–40 µm range relevant to the zebrafish trunk vascular network. Only the empirical observations of mammalian blood behavior in glass microcapillary tubes such as ones summarized by Pries’ model (Pries et al., 1992) provide a reference for the blood viscosity estimation in small microvessels. Thus, it should be noted that the commonly reported zebrafish blood viscosity has been measured at macroscopic scales. For example, calculation of WSS in the zebrafish heart has been presented with assumptions of a macroscopic blood viscosity between 0.003–0.005 Pa s in the work by (Jamison et al., 2013) and this is perfectly valid due to the size of the heart chamber exceeding 100 µm. When measured using a device with 240 µm width, the macroscopic scale blood viscosity was reported to be 4.2 cP (Lee et al., 2017) at 0.4 hematocrit (
We would like to provide some discussion on alternative methods for obtaining WSS that exist in comparison to our coarse-grained approach. In formulating Eq. 12 for the evaluation of WSS, we have made the assumption that blood flow velocity profile in the lumen cross section is parabolic. Contrary to this, the lumen velocity profile in zebrafish vessels under physiological hematocrit has been reported (Choi et al., 2017) to be blunted and non-parabolic. Two-phase blood flow models can account for non-parabolic velocity profiles (Namgung et al., 2013) but application of such models require calculation of the mass and momentum balance through iterative optimization of the best-fit cross-sectional velocity profile model in the vessel lumen, thus making their usage complicated. Applying this technique, our WSS evaluation can double from current calculations in vessel regions with high hematocrit. Given that this error is systematic, it is expected to only affect the absolute values of our WSS calculations but not affect the spatial trends discussed here. Hence, our significant findings are not diminished by this methodology limitation. Instead of theoretically calculating the velocity profile, another approach to calculating WSS is by directly measuring the RBC velocity distribution radially along the lumen cross-section. In this approach, the edge velocity of the moving RBC core is identified and by assuming the plasma velocity to decay linearly from the edge velocity at the fringe of the RBC core to zero at the lumen wall, the WSS is estimated from the plasma shear rate (
As shown in Eq. 19, the evaluation of
Our analyses revealed two interesting hemodynamic features during the growth and development of the zebrafish. The first is developmental changes in the systolic WSS (
It has been reported that the endothelial junctional mechanosensory complex regulate vascular diameters to maintain a shear stress setpoint (Baeyens et al., 2015) by influencing EC behaviors. Studies have explored the role of VEGF receptors in modulating the mechanosensory complexes (Coon et al., 2015) but few studies have provided empirical data for crafting mechanistic understanding of the vascular morphogenesis and EC rearrangement trends in accordance with fluid shear stress levels as described in the theoretical discussions of this topic by Roux and colleagues (Roux et al., 2020). We believe that our high throughput approach to estimating WSS distribution in developing zebrafish can provide empirical data to further explore the fluid shear stress setpoint concept from an empirical perspective.
In conclusion, our current study represents the first in-depth comprehensive analysis of hemodynamics during the development of the zebrafish. Using a high-throughput semi-automated imaging and analyses protocol we presented a new finding that anterior-to-posterior (AP) gradients of hemodynamic quantities exist in the zebrafish trunk vasculature and evolve with development. We believe this previously unreported developmental trend can increment the current understanding of zebrafish vascular development and physiology with follow up studies that elucidate the correlation between EC rearrangements and the developmental changes in AP gradients. Furthermore, our method can be applied to investigate systemic changes in hemodynamics in zebrafish models of vessel malformations under pathological conditions and can be of significant relevance to diverse research fields such as tumor angiogenesis and organoid vascularization research.
The original contributions presented in the study are included in the article/Supplementary Material, further inquiries can be directed to the corresponding author
The animal study was reviewed and approved by the Safety Management Regulations for Genetic Modification Experiments of RIKEN.
SSMY performed validation experiments, analyzed data, made the figures and wrote the manuscript. JKK performed experiments. NTC helped analyze data. LKP conceptualized the project and wrote the manuscript.
This work was supported by RIKEN BDR core funding and BDR Research Automation Project. JKK was supported by JSPS Invitational Fellowship for Research in Japan (L20515); SSMY is supported by RIKEN Special Postdoctoral Researcher Program and JSPS KAKENHI (JP20K20190); LKP was supported by the Naito Foundation and the JSPS KAKENHI (19K06651).
The authors declare that the research was conducted in the absence of any commercial or financial relationships that could be construed as a potential conflict of interest.
All claims expressed in this article are solely those of the authors and do not necessarily represent those of their affiliated organizations, or those of the publisher, the editors and the reviewers. Any product that may be evaluated in this article, or claim that may be made by its manufacturer, is not guaranteed or endorsed by the publisher.
The Supplementary Material for this article can be found online at: https://www.frontiersin.org/articles/10.3389/fphys.2022.881929/full#supplementary-material
Anton H., Harlepp S., Ramspacher C., Wu D., Monduc F., Bhat S., et al. (2013). Pulse Propagation by a Capacitive Mechanism Drives Embryonic Blood Flow. Development 140, 4426–4434. doi:10.1242/dev.096768
Baeyens N., Nicoli S., Coon B. G., Ross T. D., Van den Dries K., Han J., et al. (2015). Vascular Remodeling Is Governed by a VEGFR3-dependent Fluid Shear Stress Set Point. Elife 4, e04645. doi:10.7554/elife.04645
Bussmann J., Wolfe S. A., Siekmann A. F. (2011). Arterial-venous Network Formation during Brain Vascularization Involves Hemodynamic Regulation of Chemokine Signaling. Development 138, 1717–1726. doi:10.1242/dev.059881
Campinho P., Vilfan A., Vermot J. (2020). Blood Flow Forces in Shaping the Vascular System: A Focus on Endothelial Cell Behavior. Front. Physiol. 11, 552. doi:10.3389/fphys.2020.00552
Chen C.-Y., Patrick M. J., Corti P., Kowalski W., Roman B. L., Pekkan K. (2011). Analysis of Early Embryonic Great-Vessel Microcirculation in Zebrafish Using High-Speed Confocal μPIV. Biorheology 48, 305–321. doi:10.3233/bir-2012-0600
Chen Q., Jiang L., Li C., Hu D., Bu J.-w., Cai D., et al. (2012). Haemodynamics-driven Developmental Pruning of Brain Vasculature in Zebrafish. Plos Biol. 10, e1001374. doi:10.1371/journal.pbio.1001374
Choi W., Kim H. M., Park S., Yeom E., Doh J., Lee S. J. (2017). Variation in Wall Shear Stress in Channel Networks of Zebrafish Models. J. R. Soc. Interface. 14, 20160900. doi:10.1098/rsif.2016.0900
Coon B. G., Baeyens N., Han J., Budatha M., Ross T. D., Fang J. S., et al. (2015). Intramembrane Binding of VE-Cadherin to VEGFR2 and VEGFR3 Assembles the Endothelial Mechanosensory Complex. J. Cell Biol. 208, 975–986. doi:10.1083/jcb.201408103
Dessalles C. A., Leclech C., Castagnino A., Barakat A. I. (2021). Integration of Substrate- and Flow-Derived Stresses in Endothelial Cell Mechanobiology. Commun. Biol. 4, 764. doi:10.1038/s42003-021-02285-w
Fåhræus R., Lindqvist T. (1931). The Viscosity of the Blood in Narrow Capillary Tubes. Am. J. Physiology-Legacy Content. 96, 562–568. doi:10.1152/ajplegacy.1931.96.3.562
Fedosov D. A., Caswell B., Popel A. S., Karniadakis G. E. (2010). Blood Flow and Cell-Free Layer in Microvessels. Microcirculation 17, 615–628. doi:10.1111/j.1549-8719.2010.00056.x
Fedosov D. A., Pan W., Caswell B., Gompper G., Karniadakis G. E. (2011). Predicting Human Blood Viscosity In Silico. Proc. Natl. Acad. Sci. U.S.A. 108, 11772–11777. doi:10.1073/pnas.1101210108
Follain G., Osmani N., Azevedo A. S., Allio G., Mercier L., Karreman M. A., et al. (2018). Hemodynamic Forces Tune the Arrest, Adhesion, and Extravasation of Circulating Tumor Cells. Dev. Cell. 45, 33–52. doi:10.1016/j.devcel.2018.02.015
Galloway J. L., Wingert R. A., Thisse C., Thisse B., Zon L. I. (2005). Loss of Gata1 but Not Gata2 Converts Erythropoiesis to Myelopoiesis in Zebrafish Embryos. Dev. Cell. 8, 109–116. doi:10.1016/j.devcel.2004.12.001
Gebala V., Collins R., Geudens I., Phng L.-K., Gerhardt H. (2016). Blood Flow Drives Lumen Formation by Inverse Membrane Blebbing during Angiogenesis In Vivo. Nat. Cell Biol. 18, 443–450. doi:10.1038/ncb3320
Ghaffari S., Leask R. L., Jones E. A. V. (2015). Flow Dynamics Control the Location of Sprouting and Direct Elongation during Developmental Angiogenesis. Development 142, 4151–4157. doi:10.1242/dev.128058
Goetz J. G., Steed E., Ferreira R. R., Roth S., Ramspacher C., Boselli F., et al. (2014). Endothelial Cilia Mediate Low Flow Sensing During Zebrafish Vascular Development. Cell Rep. 6, 799–808. doi:10.1016/j.celrep.2014.01.032
Jamison R. A., Samarage C. R., Bryson-Richardson R. J., Fouras A. (2013). In Vivo Wall Shear Measurements within the Developing Zebrafish Heart. Plos One 8, e75722. doi:10.1371/journal.pone.0075722
Jin S.-W., Beis D., Mitchell T., Chen J.-N., Stainier D. Y. (2005). Cellular and Molecular Analyses of Vascular Tube and Lumen Formation in Zebrafish. Development 132, 5199. doi:10.1242/dev.02087
Karthik S., Djukic T., Kim J.-D., Zuber B., Makanya A., Odriozola A., et al. (2018). Synergistic Interaction of Sprouting and Intussusceptive Angiogenesis During Zebrafish Caudal Vein Plexus Development. Sci. Rep. 8, 9840. doi:10.1038/s41598-018-27791-6
Kim S., Kong R. L., Popel A. S., Intaglietta M., Johnson P. C. (2007). Temporal and Spatial Variations of Cell-Free Layer Width in Arterioles. Am. J. Physiology-Heart Circulatory Physiology 293, H1526–H1535. doi:10.1152/ajpheart.01090.2006
Kimmel C. B., Ballard W. W., Kimmel S. R., Ullmann B., Schilling T. F. (1995). Stages of Embryonic Development of the Zebrafish. Dev. Dyn. 203, 253–310. doi:10.1002/aja.1002030302
Kochhan E., Lenard A., Ellertsdottir E., Herwig L., Affolter M., Belting H.-G., et al. (2013). Blood Flow Changes Coincide with Cellular Rearrangements During Blood Vessel Pruning in Zebrafish Embryos. Plos One 8, e75060. doi:10.1371/journal.pone.0075060
Langille B. L., O'Donnell F. (1986). Reductions in Arterial Diameter Produced by Chronic Decreases in Blood Flow Are Endothelium-dependent. Science 231, 405–407. doi:10.1126/science.3941904
Lee J., Chou T.-C., Kang D., Kang H., Chen J., Baek K. I., et al. (2017). A Rapid Capillary-Pressure Driven Micro-channel to Demonstrate Newtonian Fluid Behavior of Zebrafish Blood at High Shear Rates. Sci. Rep. 7, 1980. doi:10.1038/s41598-017-02253-7
Lenard A., Daetwyler S., Betz C., Ellertsdottir E., Belting H.-G., Huisken J., et al. (2015). Endothelial Cell Self-Fusion During Vascular Pruning. Plos Biol. 13, e1002126. doi:10.1371/journal.pbio.1002126
Lenard A., Ellertsdottir E., Herwig L., Krudewig A., Sauteur L., Belting H.-G., et al. (2013). In Vivo Analysis Reveals a Highly Stereotypic Morphogenetic Pathway of Vascular Anastomosis. Dev. Cell. 25, 492. doi:10.1016/j.devcel.2013.05.010
Malone M. H., Sciaky N., Stalheim L., Hahn K. M., Linney E., Johnson G. L. (2007). Laser-Scanning Velocimetry: a Confocal Microscopy Method for Quantitative Measurement of Cardiovascular Performance in Zebrafish Embryos and Larvae. BMC Biotechnol. 7, 40–11. doi:10.1186/1472-6750-7-40
Nakajima H., Yamamoto K., Agarwala S., Terai K., Fukui H., Fukuhara S., et al. (2017). Flow-Dependent Endothelial YAP Regulation Contributes to Vessel Maintenance. Dev. Cell. 40, 523. doi:10.1016/j.devcel.2017.02.019
Namgung B., Ju M., Cabrales P., Kim S. (2013). Two-Phase Model for Prediction of Cell-Free Layer Width in Blood Flow. Microvasc. Res. 85, 68–76. doi:10.1016/j.mvr.2012.10.006
Namgung B., Ong P. K., Johnson P. C., Kim S. (2011). Effect of Cell-Free Layer Variation on Arteriolar Wall Shear Stress. Ann. Biomed. Eng. 39, 359–366. doi:10.1007/s10439-010-0130-3
Nicoli S., Standley C., Walker P., Hurlstone A., Fogarty K. E., Lawson N. D. (2010). MicroRNA-Mediated Integration of Haemodynamics and Vegf Signalling During Angiogenesis. Nature 464, 1196–1200. doi:10.1038/nature08889
Phng L.-K., Belting H.-G. (2021). Endothelial Cell Mechanics and Blood Flow Forces in Vascular Morphogenesis. Seminars Cell and Dev. Biol. 120, 32–43. doi:10.1016/j.semcdb.2021.06.005
Pries A. R., Neuhaus D., Gaehtgens P. (1992). Blood Viscosity in Tube Flow: Dependence on Diameter and Hematocrit. Am. J. Physiology-Heart Circulatory Physiology 263, H1770–H1778. doi:10.1152/ajpheart.1992.263.6.h1770
Pries A. R., Secomb T. W. (2005). Microvascular Blood Viscosity In Vivo and the Endothelial Surface Layer. Am. J. Physiology-Heart Circulatory Physiology 289, H2657–H2664. doi:10.1152/ajpheart.00297.2005
Roustaei M., In Baek K., Wang Z., Cavallero S., Satta S., Lai A., et al. (2022). Computational Simulations of the 4D Micro-Circulatory Network in Zebrafish Tail Amputation and Regeneration. J. R. Soc. Interface. 19, 20210898. doi:10.1098/rsif.2021.0898
Roux E., Bougaran P., Dufourcq P., Couffinhal T. (2020). Fluid Shear Stress Sensing by the Endothelial Layer. Front. Physiol. 11, 861. doi:10.3389/fphys.2020.00861
Santoso F., Sampurna B. P., Lai Y.-H., Liang S.-T., Hao E., Chen J.-R., et al. (2019). Development of a Simple ImageJ-Based Method for Dynamic Blood Flow Tracking in Zebrafish Embryos and its Application in Drug Toxicity Evaluation. Inventions 4, 65–14. doi:10.3390/inventions4040065
Stratman A. N., Pezoa S. A., Farrelly O. M., Castranova D., Dye L. E., Butler M. G., et al. (2016). Mural-Endothelial Cell-Cell Interactions Stabilize the Developing Zebrafish Dorsal Aorta. Development 144, 115–127. doi:10.1242/dev.143131
Streisinger G., Walker C., Dower N., Knauber D., Singer F. (1981). Production of Clones of Homozygous Diploid Zebra Fish (Brachydanio Rerio). Nature 291, 293–296. doi:10.1038/291293a0
Sugden W. W., Meissner R., Aegerter-Wilmsen T., Tsaryk R., Leonard E. V., Bussmann J., et al. (2017). Endoglin Controls Blood Vessel Diameter through Endothelial Cell Shape Changes in Response to Haemodynamic Cues. Nat. Cell Biol. 19, 653–665. doi:10.1109/34.103273
Tinevez J.-Y., Perry N., Schindelin J., Hoopes G. M., Reynolds G. D., Laplantine E., et al. (2017). TrackMate: An Open and Extensible Platform for Single-Particle Tracking. Methods 115, 80–90. doi:10.1016/j.ymeth.2016.09.016
Traver D., Paw B. H., Poss K. D., Penberthy W. T., Lin S., Zon L. I. (2003). Transplantation and In Vivo Imaging of Multilineage Engraftment in Zebrafish Bloodless Mutants. Nat. Immunol. 4, 1238–1246. doi:10.1038/ni1007
Udan R. S., Vadakkan T. J., Dickinson M. E. (2013). Dynamic Responses of Endothelial Cells to Changes in Blood Flow during Vascular Remodeling of the Mouse Yolk Sac. Development 140, 4041–4050. doi:10.1242/dev.096255
Vedula V., Lee J., Xu H., Kuo C.-C. J., Hsiai T. K., Marsden A. L. (2017). A Method to Quantify Mechanobiologic Forces during Zebrafish Cardiac Development Using 4-D Light Sheet Imaging and Computational Modeling. Plos Comput. Biol. 13, e1005828. doi:10.1371/journal.pcbi.1005828
Watkins S. C., Maniar S., Mosher M., Roman B. L., Tsang M., St Croix C. M. (2012). High Resolution Imaging of Vascular Function in Zebrafish. Plos One 7, e44018. doi:10.1371/journal.pone.0044018
Keywords: zebrafish, development, live imaging, blood flow, blood viscosity, wall shear stress
Citation: Maung Ye SS, Kim JK, Carretero NT and Phng L-K (2022) High-Throughput Imaging of Blood Flow Reveals Developmental Changes in Distribution Patterns of Hemodynamic Quantities in Developing Zebrafish. Front. Physiol. 13:881929. doi: 10.3389/fphys.2022.881929
Received: 23 February 2022; Accepted: 23 May 2022;
Published: 20 June 2022.
Edited by:
Koichi Kawakami, National Institute of Genetics, JapanReviewed by:
Jovana Serbanovic-Canic, The University of Sheffield, United KingdomCopyright © 2022 Maung Ye, Kim, Carretero and Phng. This is an open-access article distributed under the terms of the Creative Commons Attribution License (CC BY). The use, distribution or reproduction in other forums is permitted, provided the original author(s) and the copyright owner(s) are credited and that the original publication in this journal is cited, in accordance with accepted academic practice. No use, distribution or reproduction is permitted which does not comply with these terms.
*Correspondence: Li-Kun Phng, bGlrdW4ucGhuZ0ByaWtlbi5qcA==
†These authors have contributed equally to this work and share first authorship
Disclaimer: All claims expressed in this article are solely those of the authors and do not necessarily represent those of their affiliated organizations, or those of the publisher, the editors and the reviewers. Any product that may be evaluated in this article or claim that may be made by its manufacturer is not guaranteed or endorsed by the publisher.
Research integrity at Frontiers
Learn more about the work of our research integrity team to safeguard the quality of each article we publish.