- 1Department of General Psychology, University of Padova, Padova, Italy
- 2Institut des Neurosciences Paris-Saclay, Université Paris-Saclay, CNRS, Saclay, France
- 3Department of Biology, University of Padova, Padova, Italy
- 4Padova Neuroscience Center, University of Padova, Padova, Italy
- 5Department of Biomedical Sciences, University of Padova, Padova, Italy
The central complex (CX) is a neural structure located on the midline of the insect brain that has been widely studied in the last few years. Its role in navigation and goal-oriented behaviors resembles those played by the basal ganglia in mammals. However, the neural mechanisms and the neurotransmitters involved in these processes remain unclear. Here, we exploited an in vivo bioluminescence Ca2+ imaging technique to record the activity in targeted neurons of the ellipsoid body (EB). We used different drugs to evoke excitatory Ca2+-responses, depending on the putative neurotransmitter released by their presynaptic inputs, while concomitant dopamine administration was employed to modulate those excitations. By using a genetic approach to knockdown the dopamine 1-like receptors, we showed that different dopamine modulatory effects are likely due to specific receptors expressed by the targeted population of neurons. Altogether, these results provide new data concerning how dopamine modulates and shapes the response of the ellipsoid body neurons. Moreover, they provide important insights regarding the similitude with mammals as far as the role played by dopamine in increasing and stabilizing the response of goal-related information.
Introduction
The insect central complex (CX) represents an integrative structure which provides spatial representation for locomotor control (Pfeiffer and Homberg, 2014). It has been an intriguing neural structure for neuroscientists since several decades. Lately, it has become a highly debated topic where several research groups have thoroughly investigated its connectome, transcriptome and shown its functional role in navigation and goal-oriented behaviors (Seelig and Jayaraman, 2015; Green et al., 2019; Turner-Evans et al., 2020; Hulse et al., 2021).
The CX is an ensemble of four neuropils located along the midline of the protocerebrum (Figure 1A). Two main types of neurons innervate the CX (Figure 1A): tangential neurons, which form strata within one neuropil, and columnar neurons, which connect different neuropils perpendicularly (Hanesch et al., 1989). Among the four neuropils the lower division of the central body, also known as ellipsoid body (EB), constitutes the entry point of visual inputs to the CX through the anterior optic tubercle (AOTU) (Seelig and Jayaraman, 2013; Omoto et al., 2017; Hardcastle et al., 2021). Visual information is conveyed by the tubercular-bulbar neurons (TuBu) to the bulb (BU), towards which the tangential neurons of the EB project their dendrites (Shiozaki and Kazama, 2017; Sun et al., 2017). In flies, these tangential neurons send their axons to different layers of the EB forming a densely packed toroid of synapses (Figure 1B). Because of their ringlike shape, they are called ring neurons (R-neurons) and different subclasses have been defined on the basis of the layer (i.e., R1-6) and the domains innervated in the EB (Omoto et al., 2018). The columnar neurons innervating the EB arborize in different circular sectors along the coronal plane and they maintain a segregated organization projecting to the protocerebral bridge (PB) and to other compartments of the CX, such as gall (GA)—small regions on the “shoulder” of the lateral accessory lobe (LAL)—or noduli (Hanesch et al., 1989). Besides the innervation pattern, different types of columnar neurons have been classified depending on the radial morphology of their circular sectors: the wedge-shaped and the tile-shaped arborizations (Wolff et al., 2015).
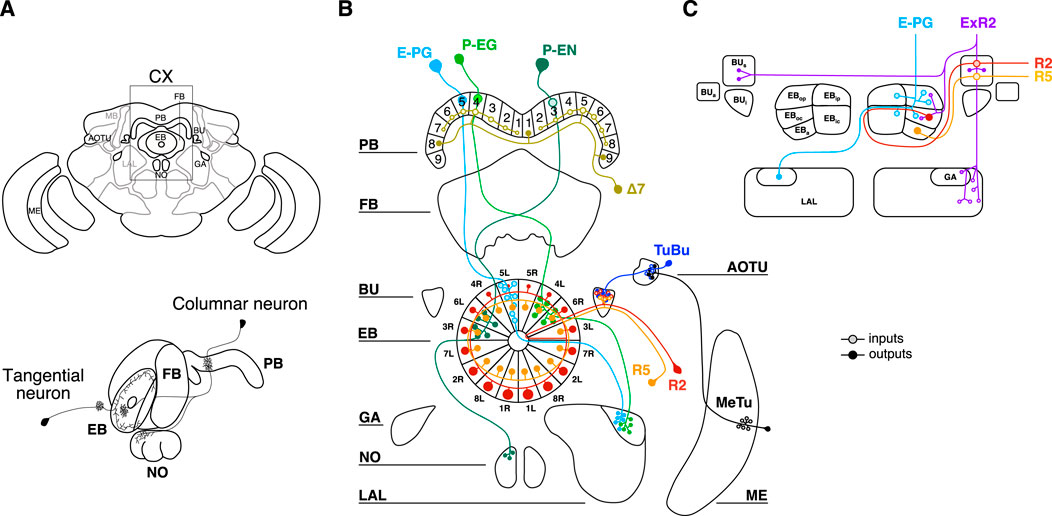
FIGURE 1. Central complex and type of neurons innervating the ellipsoid body. (A) On the top, cartoon of the fly brain with a window framing the central complex (CX). Four different neuropils composed the CX: protocerebral bridge (PB); fan-shaped body (FB); ellipsoid body (EB) and noduli (NO). Other brain regions represent important hubs for the information transmitted to/from the CX: medulla (ME); anterior optic tubercle (AOTU); bulb (BU); gall (GA) and lateral accessory lobe (LAL). On the bottom, example of two different types of neurons innervating the CX: tangential and columnar neurons. (B) Circuit diagram of the columnar neurons involved in encoding the visual inputs and in translating them to information for navigation and goal-oriented behaviors. E-PG neurons are columnar neurons receiving inputs in the EB and sending outputs to PB and GA. P-EG and P-EN are columnar neurons receiving inputs in the PB and sending outputs to the EB. Δ7 neurons receive inputs from seven glomeruli in the PB and send output to three glomeruli spaced out by seven glomeruli. R2 and R5 are tangential neurons innervating similar regions of the EB and BU. MeTu neurons, relaying visual information to the AOTU, are also depicted. (C) EB domains innervated by the neurons object of our study: E-PG, R2 and R5. ExR2 (also known as PPM-EB) dopaminergic neurons are also depicted (image adapted from Omoto et al., 2018).
The most famous wedge-shaped neurons are probably the E-PG (Figure 1B), where the first letter stands for the region receiving mainly inputs, that is the EB, and the ones after the hyphen stand for the output regions, that is the PB and the GA (Wolff and Rubin, 2018). These neurons, which segment the toroid in a way that resembles the slices of a pie (i.e., wedges), have been shown to maintain a representation of the fly’s heading direction also in the absence of direct sensory inputs through attractor-like dynamics (Seelig and Jayaraman, 2015; Green et al., 2017; Turner-Evans et al., 2017). More generally, the E-PG neurons may reflect one component of a complex interface between heading and action selection which in turn underlies complex abilities such as navigation (Green et al., 2019; Dan et al., 2021). On the other hand, tile-shaped neurons such as the P-EG instead (Figure 1B), occupy the surface volume of the caudal EB and they innervate larger circular sectors (i.e., tiles) than the wedges (Wolff et al., 2015).
Finally, another subclass of ring neurons, called ExR-neurons (extrinsic ring neurons), arborize in the EB but also outside of it (Hanesch et al., 1989). The ExR2 neurons correspond to the PPM3-EB dopaminergic neurons innervating EB, BU and the LAL (Figure 1C) (Omoto et al., 2018; Hulse et al., 2021).
Remarkably, extensive correspondences in heritable ontogeny, neuroanatomical organization and function between the vertebrate basal ganglia and the insect central complex (CX) have been put forward. Specifically, similarities have been found regarding the embryological derivation, orthologous genetic specification, neurochemicals and physiological properties (Strausfeld and Hirth, 2013a; Strausfeld and Hirth, 2013b). Furthermore, correspondences of computational mechanisms mediated by dopamine, which subtend the selection and maintenance of actions in vertebrates and insects, have been proposed (Fiore et al., 2015). In a similar manner, the dopaminergic fluctuations in the basal ganglia and the CX might mediate the switch from stable to unstable patterns of selection and vice versa through the differential effects of the direct and indirect pathways (Humphries et al., 2012; Fiore et al., 2015).
Whereas some behavioral effects of dopamine in the CX have already been shown (Lebestky et al., 2009; Kong et al., 2010; Kottler et al., 2019), no data regarding its role in the neurophysiological modulation of CX and even less supporting the existence of distinct pathways—based on different type of receptors—are available so far.
Here, we asked whether dopamine modulates EB neurons and whether different types of receptors could differentially mediate their activation levels. The aim of this study was to understand whether neural circuits involved in goal-oriented behaviors in flies respond to dopamine in a way that is similar to the mammalian basal ganglia (Green et al., 2019; Kottler et al., 2019; Dan et al., 2021). We studied the effects of dopamine on two tangential (i.e., R2 and R5) and one columnar (i.e., E-PG) neurons (Wolff et al., 2015; Omoto et al., 2018). By taking advantage of a functional in vivo bioluminescence Ca2+ imaging technique (Martin et al., 2007), we recorded the activity of specific neural populations in response to excitatory drugs with and without a previous dopamine application.
Our results show that: 1) the E-PG neurons are modulated by dopamine and two subtypes of dopamine 1-like receptors, Dop1R1 and Dop1R2, influence the state of their excitability in opposite ways; 2) two populations of R-neurons, R2 and R5,—likely expressing Dop1R1 and dopamine 2-like receptors (D2R) respectively—are modulated by dopamine, which increases the response in R2 and decreases the response in R5 neurons.
These results suggest that dopamine affects the excitability state of the EB neurons and that different pathways, involving different type of dopamine receptors—as seen in vertebrate basal ganglia—modulate their responses. We propose that dopamine might contribute to the selection of goal-directed behaviors by exciting and inhibiting different subsets of tangential and columnar neurons.
Materials and Methods
Fly Stocks
Flies were maintained on standard medium at room temperature (24°C). Five to ten newly enclosed males and females per vial were kept for mating. A new version of the responder GFP-aequorin (G5A) placed downstream of 20 UAS repetitions (w1118;P{y+t7.7 w+mC = 20xUAS-G5A}attP2) (a courtesy of Barret D. Pfeiffer, Janelia Research Campus, Ashburn, VA, United States) was used for crossings with the driver lines targeting tangential and columnar neurons (Pfeiffer et al., 2010; Jenett et al., 2012). To target the R2 neurons we used the w1118; P{y+t7.7 w+mC = GMR20D01-GAL4}attP2 (BDSC #48889), while for the R5 neurons we used the w1118; P{y+t7.7 w+mC = GMR72D06-GAL4}attP2 (BDSC #39769). The columnar E-PG neurons were targeted by using the w1118; P{y+t7.7 w+mC = GMR70G12-GAL4}attP2 (BDSC #39552). Knockdown of dopamine 1-like receptors was performed by means of RNAi on Dop1R1 w1118; P{w+mC = UAS-Dop1R1 RNAi}pKC43; (VDRC #107058/KK) and Dop1R2 w1118;P{w+mC = UAS-Dop1R2 RNAi}pMF3; (VDRC #3392/GD) (Dietzl et al., 2007; Green et al., 2014). Therefore, trans-heterozygous lines bearing GAL4, 20xUAS-G5A and UAS-RNAi were also used (Figure 2A). Imaging experiments were performed on 4–5 days old mated females.
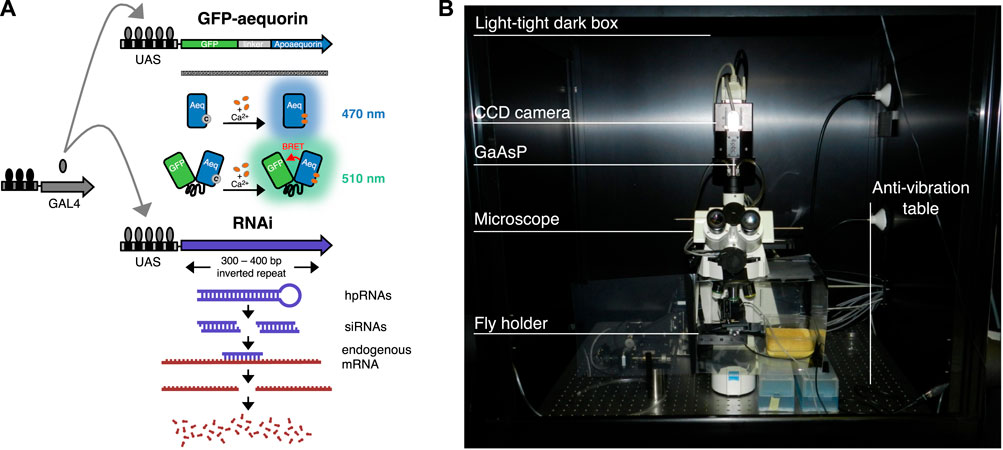
FIGURE 2. Genetic techniques and Ca2+ imaging setup. (A) GFP-aequorin responders activated by the driver GAL4 used in this study. On the top, schematic of GFP and aequorin fusion gene with upstream activation sequence (UAS). Models of blue light emission by aequorin (grey dot represents coelenterazine), and of green light emission by GFP-aequorin in response to high levels of Ca2+ (orange dots) (image adapted from Xiong et al., 2014). On the bottom, schematic of the RNAi technique in which a double-stranded RNA (i.e., hairpin RNA, hpRNA) is expressed under the control of UAS, as a complementary sequence to the gene of interest. The dsRNA is then processed by Dicer-2 into siRNA which leads to sequence-specific degradation of the mRNA related to the gene of interest (image adapted from https://stockcenter.vdrc.at/control/library_rnai). (B) Image of the setup used for in vivo Ca2+ brain imaging based on the bioluminescence technique.
Preparation of Flies
Flies ready to be tested were prepared for in vivo brain imaging experiments as described by Martin et al. (2007). Briefly, an offspring collected from food vials was ice-anesthetized and then, by gently grabbing its wings with a forceps, was positioned upside down on a plastic coverslip (22 × 22 × 0.157 mm) specifically designed to accommodate the upper half of its head inside a hole (BAH446900000-1 PK, Sigma-Aldrich, St. Louis, MO, United States). A drop of dental glue between the coverslip and the dorsal part of the fly’s thorax guaranteed the binding (Protemp 4®, 3M ESPE™, Seefeld, Germany, EU). The coverslip was previously prepared so as to have a hole of approximately 0.6 mm diameter drilled at the center by using an awl. Moreover, a thinner edge around this hole was made by using a cutting burr bit mounted on a rotary machine tool (Dremel 3,000, Dremel®, Mount Prospect, IL, United States). This latter operation was necessary in order to make room for the dissection and, at the same time, to leave the fly’s visual field intact in the lower half as much as possible. Once the fly was glued to the coverslip, its head was pushed through the hole in such a way that the antennae were below the horizontal plane of the coverslip and the rest of the head capsule above it. Then, the free space around the fly’s head was sealed using a special bio-compatible silicon (Kwik-Sil™, WPI, Sarasota, FL, United States). The entire preparation was fixed on an acrylic block with two small strips of adhesive tape whereby the fly was tethered but free to move its legs. Ringer’s solution with pH = 7.3 containing 130 mM NaCl, 5 mM KCl, 2 mM MgCl2, 2 mM CaCl2, 36 mM sucrose and 5 mM HEPES-NaOH (Martin et al., 2007), was dropped over the upper half of the head and a tiny window between the eyes and just above the antennae was opened in the head capsule using a micro knife (#10315-12, Fine Science Tools GmbH, Heidelberg, Germany, EU) to expose the brain. The underlying neural sheath was also gently removed with forceps (Dumont #5SF, Fine Science Tools GmbH, Heidelberg, Germany, EU) in order to improve the exposure of the outer brain surface. Extreme care was taken to avoid damaging the fly brain structures. The dissection procedure was performed under a fluorescence stereomicroscope (Leica MZ FLIII, Leica Microsystems GmbH, Wetzlar, DE, EU). After the dissection, the fly was free to recover for 2 h while its exposed brain was incubated in 100 μl of Ringer’s solution containing 25 μM native water-soluble coelenterazine (NanoLight®, Prolume Ltd., Pinetop, AZ, United States). Subsequent to the incubation, the preparation solution was replaced with 100 μl of fresh Ringer’s solution and the fly was ready to be imaged. In this condition, the fly was able to breathe via the tracheal system and could be maintained alive for more than 24 h. However, before starting the recording an air puff was delivered with a mouth aspirator onto the fly’s legs to stimulate a locomotor reflex. If the fly did not show any response, then it was not further considered for the experiment.
In Vivo Brain Imaging
Bioluminescence signals (i.e., Ca2+-response) in tangential and columnar neurons were recorded using an intensified CCD camera with a cooled (at −20°C) GaAsP photocathode (Turbo-Z™, Stanford Photonics Inc., Palo Alto, CA, United States) fitted onto a direct microscope (Axioplan 2, Carl Zeiss GmbH, Jena, Germany, EU) (Figure 2B). The entire system was positioned on an anti-vibration table and housed inside a light-tight dark box (Science Wares Inc., Falmouth, MA, United States). Using a 20x water immersive objective lens (Zeiss N-Achroplan, N.A. 0.5) the spatial resolution was 480 × 360 μm (640 × 480 pixels), while using a 40x objective lens (Zeiss N-Achroplan, N.A. 0.75) it was 240 × 180 μm (1 pixels = 0.375 × 0.375 μm). To acquire and store data, each detected photon was assigned an x and y-coordinate and a time point (i.e., x, y, t). Photon acquisition was carried out at 120 frames s−1, providing 8.3 ms time resolution with an extremely low background signal. The Photon Imager software (Science Wares Inc., Falmouth, MA, United States) written in LabView 2010 (National Instruments™, Austin, TX, United States) was used for this purpose. Image recordings were obtained from 10 to 20 flies per each genotype. Differently to other fluorescence-based imaging approaches such as those which rely on GCaMP, bioluminescent GFP-aequorin does not require (and should not undergo) light excitation. This provides some advantages while avoiding some of the disadvantages associated with light excitable fluorophores. Specifically, the GFP-aequorin signal is not subject to disturbances from autofluorescence, phototoxicity and photobleaching. Consequently, it allows continuous real-time recordings, which in our case were performed with a 8.3 ms temporal resolution, during extended periods of time, in relatively deeply located structures (since it has no autofluorescence). However, since this approach does not rely on fluorophore excitation, it is not possible to measure ΔF/F, but rather we directly measure the number of emitted photons (Martin, 2008).
Pharmacology
To stimulate the targeted neurons in flies we used either nicotine or picrotoxin. Depending on the putative nature of the presynaptic neurons in input to the circuit under investigation we applied these two drugs as stimulants for eliciting a Ca2+-response following activation of the excitatory nicotinic acetylcholine receptors, or blocking the GABAA receptors, respectively. Nicotine (N3876, Sigma-Aldrich, St. Louis, MO, United States) was prepared as a 10 mM stock solution in H2O and diluted to 100 μM in Ringer’s solution as final concentration reached during the experiment (i.e., 1 μl application). Picrotoxin (P1675, Sigma-Aldrich, St. Louis, MO, United States) was prepared as a 25 mM stock solution in H2O and then dissolved in Ringer’s solution to 250 μM as final concentration reached during the experiment (i.e., 1 μl application). Dopamine (H8502, Sigma-Aldrich, St. Louis, MO, United States) was dissolved directly in Ringer’s solution prepared without sucrose at 1 mM and diluted to 100 μM as the result of a 10 μl application during the recording. Accordingly, we used Ringer’s solution instead of dopamine in the control samples. KCl application was also used at the end of each trial to evoke a strong Ca2+-response in order to check that the preparation was in good condition. KCl (P9333, Sigma-Aldrich, St. Louis, MO, United States) was prepared as a 3 M stock solution in H2O and diluted to 100 mM in the bathing solution during the experiment (i.e., 30 μl application). All drugs were applied to the bath solution using a pipette directly positioned on top of the brain.
Data Processing
Pre-processing of imaging data was performed using the Photon Viewer (2.1) software (Science Wares Inc., Falmouth, MA, United States). Bioluminescence signals are presented as the total amount of emitted photons within a selected region of interest (ROI). Using GFP images of individual expression patterns, collected before the beginning of the experiments, we identified the ROIs and confirmed them by visual verification of the coverage of the subsequent response area. Different ROIs were manually drawn depending on the subclass of neurons investigated and the same shapes and sizes were kept constant among flies. To improve the signal-to-noise ratio, data were subjected to 1 s integration time (1 Hz) without applying any binning of pixels. The data frames were exported as “.csv” files and then imported into RStudio Team (2017) for data processing and subsequent statistical analysis. Duration, latency and total photons of the Ca2+-response were automatically computed for each ROI using a custom R script. We considered a response onset as a 10% increase in the number of photons s−1 with respect to a normalization performed on the basis of the maximum number of photons s−1 collected (i.e., the response peak). Moreover, to avoid the detection of unrelated activity such as the triggering of spots of increased photons s−1 on the part of rare cosmic rays, the response above the 10% threshold had to be sustained for at least 2 s. Accordingly, the end of the response was defined as the decrease in the photons s−1 below the 10% threshold. For the average profile, the alignment was performed on the response peak and the time window set to 200 s around the peak (i.e., 100 s before and 100 s after the peak).
Statistical Approach
We adopted a Bayesian approach instead of a more “traditional” frequentist approach to analyze our data in order to accommodate the nature and structure of the data and to move forward with an approach that will probably contribute to improve the generality of the inferences, given the fact these data are not treatable (or poorly so) with the frequentist approach (Baker, 2016; Saravanan et al., 2020; DeBruine and Barr, 2021). A linear mixed effects (LME) model consists in a regression in which the parameters (i.e., the regression coefficients) are assigned to a probability model that is in turn estimated from the data (Gelman and Hill, 2007). In a LME model at least one predictor is categorical (e.g., experimental units such as the flies in our study) and defined by a set of discrete levels. The parameters associated to the specific levels of a predictor are called effects. They are called fixed effects when the set of levels is fixed and reproducible and they are called random effects when the levels constitute a random sample from the set of all possible levels (Bates, 2010). It is important to notice that the fixed effects parameters are actual parameters of the statistical model while the random effects are technically not parameters, but unobserved random variables (Bates, 2010). Mixed effects models are statistical models which include both fixed and random effects and the LME models are a specific class of these. They are also called hierarchical or multilevel models both because of the characteristics of the data structures they can handle and because of the hierarchy defined by the parameters of the model (Gelman and Hill, 2007). LME models allow the adjustment of estimates for repeated sampling and for imbalance in sampling (i.e., some groups with more individuals than others). Also, they take into account experimental variation (i.e., variation among flies or among other grouping variables) avoiding the harmful effects of averaging which very often reduce the statistical power of the analysis. For these reasons, LME models represent a reasonable way to strike a good balance between Type I error and power. We fitted the data with different LME models with the R package lme4 (Bates et al., 2014). The models were then compared by using the Bayesian Information Criterion (BIC) in order to select the model with the greatest predictive power, given the experimental data against which it is tested (Schwarz, 1978). BIC is an index that measures the efficiency of the model in terms of data prediction. Furthermore, an approximation of the Bayes Factor (BF), calculated as
where
Results
Evoked Ca2+-Activity in the EB Neurons
To test if bath-applied drugs excited the EB neurons, we used an in vivo bioluminescence Ca2+ imaging technique as previously described (Martin et al., 2007; Pavot et al., 2015; Lark et al., 2016). By means of the GAL4-UAS binary system we expressed the bioluminescent molecule GFP-aequorin (G5A) in different EB neurons (Baubet et al., 2000; Martin et al., 2007). In order to narrow down the investigation to a few driver lines expressing GAL4 in selected EB neurons which were also deemed more likely to be modulated by dopamine, we screened the expression patterns of a number of GAL4 lines from the FlyLight database (Jenett et al., 2012). The prerequisites for a driver line to be included were the pattern of innervation at the level of the EB and concurrently that the fragment of DNA serving as transcriptional enhancer for GAL4 belonged to a dopamine receptor. Alternatively, the driver line had to show an expression pattern which highlighted innervation of the EB and which overlapped with the pattern of a GAL4 line, the transcriptional enhancer of which belonged to a dopamine receptor. In this way, three main GAL4 lines were selected for the experiments: two lines expressing in two different subclasses of R-neurons and one line expressing in the E-PG neurons.
The first driver line targeting tangential neurons, the R20D01 (Figure 3A, top), is selective for the R2 neurons as defined by Omoto et al. (2018). This line actually has the transcriptional enhancer corresponding to a DNA fragment of the nicotinic acetylcholine receptor (nAChR) α3 subunit. Therefore, it is not directly a putative dopamine receptor-expressing line. However, it was selected because of the overlap in the expression pattern with another line targeting the R2 neurons (R72B07) which does have the putative enhancer fragment of the Dop1R1 receptor (Figure 3B). The latter driver line was not selected because its pattern includes TuBu neurons. Therefore, we surmised that the R2 neurons targeted by R20D01 express Dop1R1 besides nAChR and we pharmacologically stimulated them (R2 > G5A flies) using nicotine as the excitatory drug.
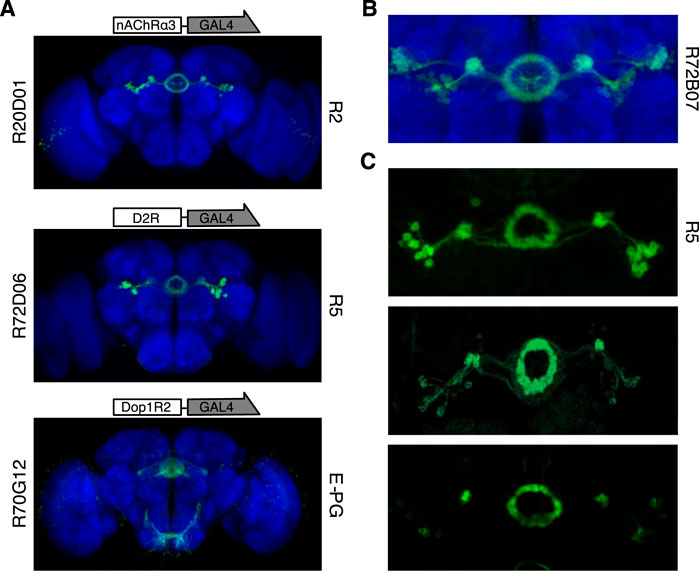
FIGURE 3. Driver lines selected and neurons targeted. (A) Images of the pattern expressed by the GAL4 lines selected from the Janelia FlyLight Project database (https://flweb.janelia.org/cgi-bin/flew.cgi) (Jenett et al., 2012). Starting from the top: the R20D01, with the promoter sequence corresponding to the putative enhancer sequence of the nAChRα3 gene, targets the R2 neurons; in the middle the R72D06, with the promoter sequence of the D2R gene, targets the R5 neurons; and at the bottom the R70G12, with the promoter associated to Dop1R2 gene, targets the E-PG neurons. (B) Expression pattern of R72B07-GAL4 (image from https://flweb.janelia.org/cgi-bin/flew.cgi) which has the promoter sequence associated to the Dop1R1 gene. As can clearly be appreciated, its pattern is widely superimposable with that of R20D01, apart from the TuBu neurons. (C) Expression patterns of three different driver lines targeting the same subclass of neurons (i.e., R5). Starting from the top: image of the R58H05-GAL4 line expressing GFP (10xUAS-IVS-mCD8:GFP) which was considered by Omoto et al. (2018) to target the same neurons considered as R2 by Liu et al. (2016) and recently defined as R5 neurons (image from https://flweb.janelia.org/cgi-bin/flew.cgi); in the middle, pattern of the nv45-LexA:VP16 driver line expressing GFP (LexAop-mCD8:GFP) which was considered to target R3 neurons (image taken with permission from Kottler et al., 2017); at the bottom, image of the R69F08-GAL4 line (used by Liu et al., 2016) expressing GFP (10xUAS-IVS-mCD8:GFP) that was considered to target R2 neurons (image from https://flweb.janelia.org/cgi-bin/flew.cgi).
The second driver line targeting tangential neurons, the R72D06 (Figure 3A, middle), strongly labels what have recently been named R5 (Figure 3C, top) neurons (Omoto et al., 2018). These neurons seem to correspond quite closely to the neurons targeted by the nv45-LexA driver line which Kottler and coworkers have previously defined as R3 (Figure 3C, middle) neurons (Kottler et al., 2017). Furthermore, according to Omoto et al. (2018), R5 neurons well described those which in previous papers have been referred to as the R2 (Figure 3C, bottom) subclass (Liu et al., 2016; Donlea et al., 2018). The R72D06 driver line is characterized by a putative enhancer fragment corresponding to D2R. Since this line projects axons over the anterior surface of the EB, then into the canal and these then spread centrifugally, other neurons, probably R3w, may be targeted as well (Omoto et al., 2018). The expression pattern of another driver line (R70F01) (Jenett et al., 2012) with a putative enhancer fragment from the resistant to dieldrin (Rdl) gene, which encodes for the GABAA receptor, targets a wide range of R-neurons (among them R5 and R3w) but substantially spares R2 neurons. For this reason, we pharmacologically disinhibited the R5 neurons (R5 > G5A flies) using picrotoxin, a non-competitive blocker of GABAA receptor chloride channels.
Finally, the E-PG neurons were targeted by the driver line R70G12 which was characterized by a putative enhancer fragment from the Dop1R2 (Figure 3A, bottom). It is worth noting that, a recent cell-type-specific RNA sequencing (RNA-seq) of the E-PG neurons confirmed the expression of Dop1R2 and Dop1R1 (Turner-Evans et al., 2020). Moreover, since the columnar neurons have recently been proposed to be cholinergic (Franconville et al., 2018; Turner-Evans et al., 2020) and are characterized by an extensive recurrent networking to update the fly’s heading (Green et al., 2017; Turner-Evans et al., 2017), we stimulated the E-PG neurons (E-PG > G5A flies) using nicotine. Also in this case the expression of nAChR and metabotropic acetylcholine receptor (mAChR) in E-PG have been revealed in RNA-seq experiments (Turner-Evans et al., 2020).
To test whether the stimulant drugs were able to excite the cells under investigation, we applied the drugs during the Ca2+ imaging recordings (Figure 4A). After 10 min of baseline recording, the drug was applied to the preparation and the evoked response was recorded over a period of 10 min. At the end of this period, 10 μl of KCl was applied as a stimulus control to verify the integrity of the brain preparation (Figure 4B).
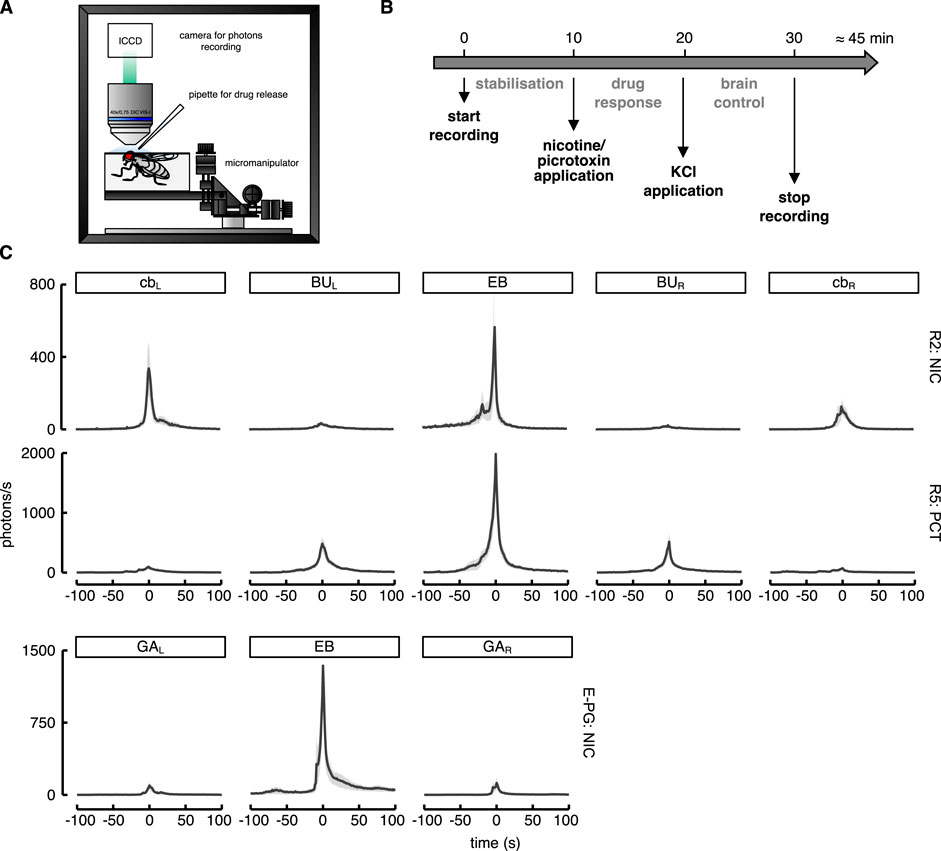
FIGURE 4. Experimental procedure and Ca2+-response to drugs application. (A) Cartoon of the fly preparation depicting the main components which are not drawn to scale. (B) Schematic drawing of the protocol used to stimulate the neurons. (C) Ca2+-response profiles to drug application in the selected ROIs (cbL-R: left and right cell bodies; BUL-R: left and right bulb; EB: ellipsoid body; GAL-R: gall region). Starting from the top: nicotine-evoked (NIC) activity of the R2 neurons (R20D01 driver) in the five ROIs drawn around cb, BU and EB (see also Supplementary Movie S1 for a representative brain response); in the middle picrotoxin-evoked (PCT) activity of the R5 neurons (R72D06 driver) in the five ROIs drawn as in the previous neurons (see also Supplementary Movie S2 for a representative brain response); at the bottom, nicotine-evoked (NIC) activity of the E-PG neurons (R70G12 driver) in the three ROIs drawn around the 2 GA regions and EB (see also Supplementary Movie S3 for a representative brain response).
The results confirmed our expectations regarding the stimulating properties of the drugs used. In all genotypes, a Ca2+-response was evoked as a consequence of the application (Figure 4C) (see also Supplementary Movies S1–3 for a representative brain response for each driver line). Compared to the baseline activity, drug-evoked responses in the EB reached thousands of photons s−1 within a few seconds. Although a pharmacological approach makes it almost impossible to rule out indirect responses caused by the activation of neurons other than those specifically targeted, we believe that the bulk of these responses was likely determined by direct stimulation.
Dopamine Modulation of Ca2+-Activity of the EB Neurons
To test the hypothesis that these neurons could be subject to dopamine modulation, we modified the previous protocol by adding a dopamine application 10 min before the stimulation (Figure 5A). The R2 neurons showed an enhanced Ca2+-response to nicotine after dopamine application compared to the condition without dopamine (Figure 5B). However, to better understand the extent of the dopamine modulation, we fitted the data with different LME models considering several predictors: “condition” that distinguishes the responses with or without dopamine application; “ROIs” that refers to the different regions innervated by the driver line (e.g., cell body, EB and BU); and “time” that refers to the time window during which the responses were unfolded. For the R2 neurons we defined five ROIs encompassing all the EB, the two lateral superior BU and the two lateral groups of cell bodies (cb). We computed the BIC for the LME model comparisons and the best one took into account the interaction between “condition” and ROIs as fixed effects (Table 1).
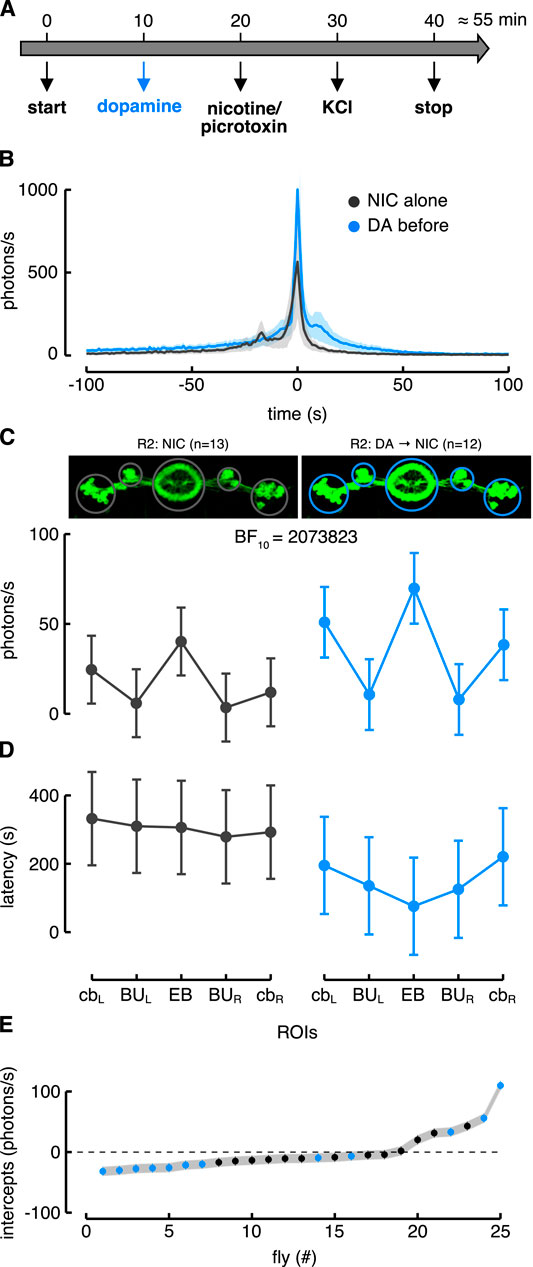
FIGURE 5. Dopamine modulation in R2 > G5A flies. (A) Image of the protocol used to modulate the neurons before the drug application (nicotine or picrotoxin). (B) Ca2+-response profiles of the R2 neurons in the EB ROI. In black is depicted the condition with the nicotine alone while in blue the condition with dopamine application before nicotine. (C) Estimated parameters of the Ca2+-response referred to the interaction between condition and ROIs (i.e., fixed effect). The dots represent the estimated values while the error bars correspond to the 97.5% confidence intervals (CI) computed with parametric bootstrap of 10,000 simulations. On the left is represented the Ca2+-response to nicotine (NIC) alone in the five ROIs of the R2 neurons (n = 13), while on the right is represented their Ca2+-response to nicotine after dopamine (DA) application (n = 12) (see also Supplementary Movie S4 for a representative brain response). (D) Estimated parameters of the response latency referred to the interaction between condition and ROIs (i.e., fixed effect) with corresponding 97.5% CI (computed as in Figure 5C). On the left is represented the latency response to nicotine alone in the five ROIs of the R2 neurons while on the right is represented their latency response to nicotine after dopamine application. (E) Plot of random effect referred to Ca2+-response (i.e., random fly intercept). Dots represent each fly (known as BLUPs, Best Linear Unbiased Predictions) while the horizontal lines crossing dots (i.e., error bars) correspond to the standard deviation (SD).
This model showed a higher likelihood than the one that considered only the ROIs as a fixed effect (BF
On the contrary, the other subclass of R-neurons, the R5, showed a clear reduction of the Ca2+-response to picrotoxin after dopamine application. As in the case of the R2 neurons, we tested and compared the same LME models. The ROIs for these neurons corresponded roughly to the ones of the R2, apart from the BU which were encompassed by larger ROIs because of the particular ramifications projecting to the EB. Again, the best model was the one that included the interaction between “condition” and ROIs. It showed a higher likelihood than the one with only ROIs as the fixed effect (BF
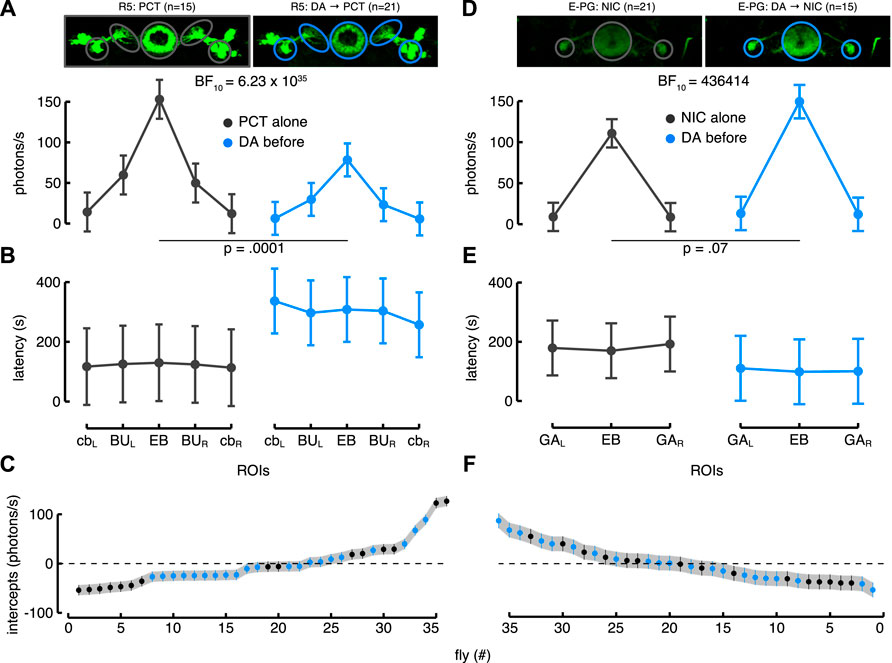
FIGURE 6. Dopamine modulation of R5 > G5A (A–C) and E-PG > G5A flies (D–F). (A) Estimated parameters of the Ca2+-response referred to the interaction between condition and ROIs (i.e., fixed effect) with corresponding CI (computed as in Figure 5C). On the left is represented the Ca2+-response to picrotoxin (PCT) alone in the five ROIs of the R5 neurons (back, n = 15), while on the right is represented their Ca2+-response to picrotoxin after dopamine (DA) application (blue, n = 21) (see also Supplementary Movie S5 for a representative brain response). (B) Estimated parameters of the response latency referred to the interaction between condition and ROIs (computed as in Figure 5D). On the left is represented the latency response to picrotoxin alone in the five ROIs of the R5 neurons (black) while on the right is represented their latency response to picrotoxin after dopamine application (blue). (C) Plot of random effect referred to Ca2+-response of R5 neurons (as in Figure 5E). (D) Estimated parameters of the Ca2+-response referred to the interaction between condition and ROIs (as in Figure 5C). On the left is represented the Ca2+-response to nicotine (NIC) alone in the three ROIs of the E-PG neurons (black, n = 21), while on the right is represented their Ca2+-response to nicotine after dopamine (DA) application (blue, n = 15) (see also Supplementary Movie S6 for a representative brain response). (E) Estimated parameters of the response latency referred to the interaction between condition and ROIs (computed as in Figure 5D). On the left is represented the latency response to nicotine alone in the three ROIs of the E-PG neurons (black) while on the right is represented their latency response to picrotoxin after dopamine application (blue). (F) Plot of random effect referred to Ca2+-response of E-PG neurons (as in Figure 5E).
Dopamine application on the E-PG neurons moderately increased the Ca2+-response. Three ROIs were drawn, one encompassed the EB and two the GA regions. The deeper PB glomeruli innervated by these neurons and their perikarya were not considered because of difficulties in imaging the PB from above and to standardize a common ROI dimension for the cell bodies. Among the LME models tested, the best one confirmed the interaction between condition and ROIs as the fixed effect. This model showed a higher likelihood than the simpler model which did not consider the “condition” (BF
Knockdown of Dopamine Receptors in R2 Neurons
Since the targeted R2 neurons expressed GAL4 under the control of an enhancer fragment which was not consistent with a dopamine receptor, we directly tested whether the Dop1R1 (likely expressed by these neurons) affected their nicotine-evoked response and if dopamine increased their excitability via those specific receptors. For this purpose, we knocked down the Dop1R1 in R2 neurons by using RNAi (R2 > G5A + Dop1R1RNAi). Flies with downregulation of Dop1R1 in the R2 neurons showed a Ca2+-response to nicotine similar to the one of control flies (Figure 7A, inset) as shown by the best model considering only ROIs as fixed effect (BF
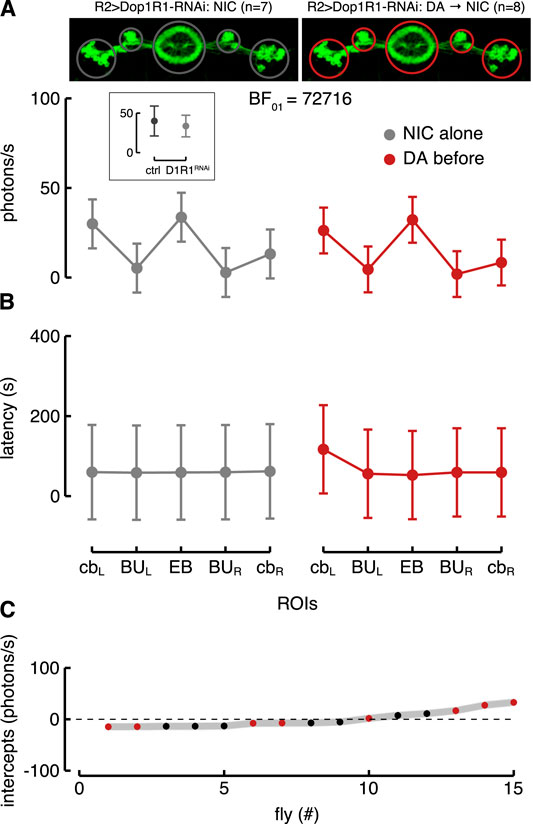
FIGURE 7. Knockdown of Dop1R1 in R2>G5A flies. (A) Estimated parameters of the Ca2+-response referred to the interaction between condition and ROIs with corresponding CI (computed as in Figure 5C). The inset represents the comparison of the estimated interaction parameters (i.e., between condition and ROIs) referred to EB in the R2 normal (ctrl) and R2 >Dop1R1-RNAi (D1R1RNAi) flies in response to nicotine. On the left is represented the Ca2+-response to nicotine (NIC) alone in the five ROIs of the R2 neurons with knockdown of Dop1R1 (R2 > Dop1R1-RNAi; grey, n = 7), while on the right is represented their Ca2+-response to nicotine after dopamine (DA) application (red, n = 8). (B) Estimated parameters of the response latency referred to the interaction between condition and ROIs (computed as in Figure 5D). On the left is represented the latency response to nicotine alone in the five ROIs of the R2 neurons with knockdown of Dop1R1 (grey) while on the right is represented their latency response to nicotine after dopamine application (red). (C) Plot of random effect referred to Ca2+-response (as in Figure 5E).
Opposite Modulation Operated by Dop1R1 and Dop1R2 in E-PG Neurons
The same approach was used to clarify the neural response of the E-PG neurons. Inasmuch as the driver we used has an enhancer fragment relating to Dop1R2 and previous electrophysiological data have shown an inhibitory role carried out by Dop1R2 in sleep-promoting neurons (Pimentel et al., 2016), we expected a downward modulation. Surprisingly, our results showed an enhancement of the Ca2+-response after dopamine application (Figure 6D). Thus, to better understand which were the dopamine receptors involved, we knocked down the Dop1R2 with RNAi (E-PG > G5A + Dop1R2RNAi). The downregulation of the Dop1R2 in the E-PG neurons determined an increase in the Ca2+-response to nicotine compared to the non-compromised E-PG (Figure 8A, inset). The best model confirmed the interaction between “condition” and ROIs, meaning that the reduction of the Dop1R2 (as well as Dop1R1) took the E-PG neurons to a higher level of excitability within the EB (BF
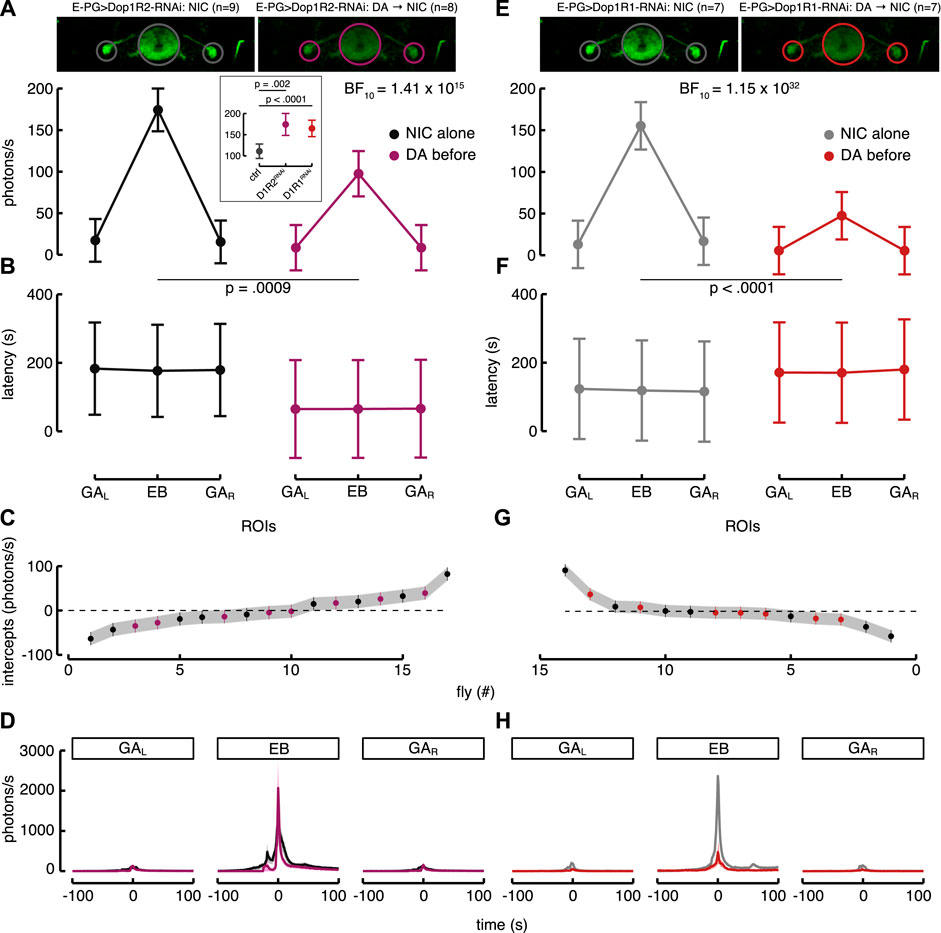
FIGURE 8. Knockdown of Dop1R2 (A–D) and Dop1R1 (E–H) in E-PG > G5A flies. (A) Estimated parameters of the Ca2+-response referred to the interaction between condition and ROIs (as in Figure 5C). The inset at the center represents the comparisons of the estimated interaction parameters (i.e., between condition and ROIs) referred to EB in the E-PG normal (ctrl), E-PG > Dop1R2-RNAi (D1R2RNAi) and E-PG > Dop1R1-RNAi (D1R1RNAi) flies in response to nicotine (post hoc comparisons adjusted with Bonferroni correction). On the left of the inset is represented the Ca2+-response to nicotine (NIC) alone in the three ROIs of the E-PG neurons with knockdown of Dop1R2 (E-PG > Dop1R2-RNAi; black, n = 9), while on the right is represented their Ca2+-response to nicotine after dopamine (DA) application (magenta, n = 8). (B) Estimated parameters of the response latency referred to the interaction between condition and ROIs in E-PG > Dop1R2-RNAi flies (computed as in Figure 5D). On the left is represented the latency response to nicotine alone in the three ROIs of the E-PG neurons with knockdown of Dop1R2 (black) while on the right is represented their latency response to nicotine after dopamine application (magenta). (C) Plot of random effect referred to Ca2+-response of E-PG neurons with Dop1R2 knockdown (as in Figure 5E). (D) Ca2+-response profiles to nicotine of the E-PG neurons with Dop1R2 knockdown in the three ROIs with (magenta) or without (black) dopamine application before. Starting from the left: left GA region, EB and right GA region. (E) Estimated parameters of the Ca2+-response in E-PG neurons with knockdown of Dop1R1 referred to the interaction between condition and ROIs. On the left the condition with nicotine (NIC) alone (E-PG > Dop1R1-RNAi; grey, n = 7) while on the right the condition with dopamine (DA) application before nicotine (red, n = 7). (F) Estimated parameters of the response latency in E-PG neurons with knockdown of Dop1R1 referred to the interaction between condition and ROIs (computed as in Figure 5D). On the left the condition with nicotine alone (grey) while on the right the condition with dopamine application before nicotine (red). (G) Plot of random effect referred to Ca2+-response of E-PG neurons with Dop1R1 knockdown. (H) Ca2+-response profiles to nicotine of the E-PG neurons with Dop1R1 knockdown in the three ROIs defined as in G with (red) or without dopamine application before (grey).
Discussion
In primates action selection is mainly dependent on a group of subcortical nuclei called the basal ganglia, the functions of which are critically dependent on dopamine (DeLong, 1990; Redgrave et al., 1999). Dysfunction of these nuclei may result in several pathological conditions related to motor control such as Parkinson’s or Huntington’s diseases (Redgrave et al., 2010; Nelson and Kreitzer, 2014). Two main pathways, which consist of striatal projections to the basal ganglia output nuclei, control the selection process: the direct and the indirect pathway. These two pathways act together to perform action selection by disinhibiting a selected motor program while inhibiting all the other competing ones (Grillner et al., 2005).
The direct pathway is characterized by GABAergic striatal projection neurons—expressing excitatory dopamine D1 receptors—which inhibit, upon stimulation, the tonically active GABAergic output neurons that keep the brainstem motor centers inhibited (Grillner and Robertson, 2016). On the other hand, the indirect pathway is characterized by GABAergic striatal projection neurons—expressing inhibitory dopamine D2 receptors—which inhibit the GABAergic neurons of the globus pallidus externa that in turn inhibit the GABAergic output neurons (Grillner and Robertson, 2016).
The same architecture has also been identified in an organism belonging to the phylogenetically oldest group of vertebrates, the lamprey, which diverged from the evolutionary line leading to primates some 560 million years ago (Ericsson et al., 2011; Stephenson-Jones et al., 2012). This suggests that the mammalian basal ganglia evolved through a functional replication of these circuits rather than by means of a sequential adaptation of this ancestral architecture (Stephenson-Jones et al., 2011). Moreover, the striking conservation between such evolutionary distant organisms has also been demonstrated with respect to dopamine modulation, suggesting a truly common blueprint for the evolution of the basal ganglia (Ericsson et al., 2013; Stephenson-Jones et al., 2013).
Extending the comparison to invertebrates, fly dopaminergic PPM3 neurons have been compared to the mammalian pars compacta nucleus of the substantia nigra (Strausfeld and Hirth, 2013a). This similarity has been considered to be due to homology. Nonetheless, the speculative extension of this claim remains quite large and still under discussion (Farries, 2013). Indeed, it might be due to convergent or parallel evolution rather than to homology. In any case, regardless of these intriguing evolutionary issues, the main correspondence between CX and basal ganglia—that is the presence of pathways differently modulated by dopamine (Figure 9) has remained elusive up to now.
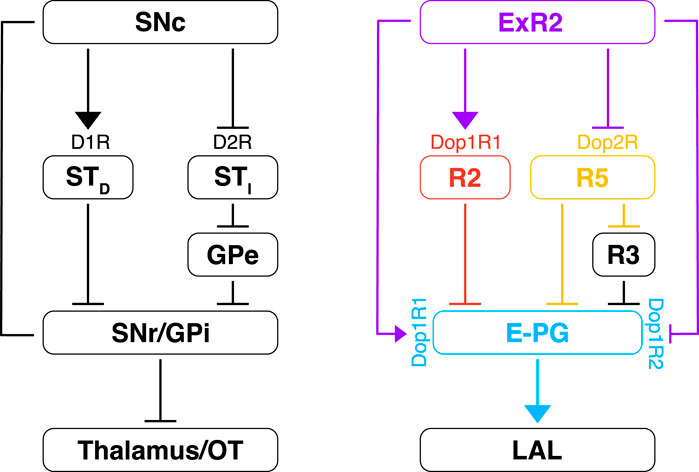
FIGURE 9. Dopamine modulation of the EB and comparison with the vertebrate basal ganglia. On the left is depicted the dopamine circuit involved in the vertebrate basal ganglia. The dopamine release from the substantia nigra pars compacta (SNc) modulates the direct and the indirect pathway. The striatal neurons (STD) of the direct pathway express the D1R (excitatory) and they inhibit the GABAergic neurons of the substantia nigra pars reticulata (SNr) and globus pallidus interna (GPi). In the indirect pathway, the striatal neurons express the D2R (inhibitory) and they inhibit the GABAergic neurons of the globus pallidus externa (GPe) which, in turn, inhibit the GPi. Both these pathways converge back to the thalamus (with efference copies) and to motor command regions like the optic tectum (OT). On the right is proposed a simple model about the dopamine modulation of the CX. As for the vertebrate basal ganglia, dopamine might excite (via Dop1R1) and inhibit (via Dop2R) specific R-neurons, R2 and R5, respectively, which synapse directly and/or indirectly to the E-PG neurons. A combination of excitatory (Dop1R1) and inhibitory (Dop1R2) dopamine receptors would be expressed by the E-PG neurons. The overall computation could serve the selection of goal-directed behaviors that is subsequently passed to the LAL for motor control. T-shaped bars mean inhibitory connections while arrows mean excitatory connections.
Kong et al. (2010) showed that PPM3 stimulation increased locomotor activity levels and that the Dop1R1 receptors in the R2 neurons are essential for ethanol-induced hyperactivity. Accordingly, a reduction of Dop1R1 in R2 neurons resulted in decreased ethanol-induced hyperactivity. This resembles the vertebrate direct pathway in which the D1 receptors act by increasing the response of the striatal projection neurons. Here, through a neurophysiological approach we showed the existence of a similar mechanism in which dopamine release on the R2 neurons modulates their response by increasing the Ca2+-activity via Dop1R1. Noteworthy, R2 neurons have been shown to respond to visual properties and to increase the walking probability (Seelig and Jayaraman, 2013; Robie et al., 2017).
On the contrary, a homologous indirect pathway in flies has never been proposed. Although Draper et al. (2007) showed that D2R plays a critical role in modulating locomotion and that reduced expression of this receptor resulted in decreased locomotor activity, no specific neuroanatomical structure or neuronal pathways have been identified at the core of this behavior. Administration of the synthetic D2 agonist bromocriptine, a well-established human anti-Parkinson drug, was also able to restore the deficit determined by the D2R knockdown (Draper et al., 2007). Strikingly, we identified a similar D2-based modulatory pathway involving R5 neurons. Our data suggest that dopamine release on the R5 neurons would act by inhibiting their activity very likely via D2R. A recent connectome analysis of the CX has highlighted that the PPM3-EB neurons (also known as ExR2) send outputs mainly to the superior BU where R2, R3w and R5 receive inputs, while a combination of input-output connections innervate the EB sending inputs to the E-PG neurons (Hulse et al., 2021). In this respect, Liang et al. (2019) have recently shown how the circadian M- and E-cells regulate the dawn and dusk peaks in locomotor activity by activating R5 neurons through the PPM3-EB. Furthermore, they showed an impaired locomotor activity in flies with Dop1R2 and D2R knock down in R-neurons (Liang et al., 2019).
Previous reports have shown that the vast majority of the R-neurons are GABAergic (Hanesch et al., 1989; Zhang et al., 2013; Fisher et al., 2019; Kim et al., 2019) and particularly the R2 neurons (Isaacman-Beck et al., 2020). By using the EB1 driver line which targets what has previously been referred to as R2/R4m, 94% of them were estimated to be GABAergic (Kottler et al., 2017). Moreover, an inter-R-neurons connectivity between R5 and R3 (ER3a specifically) has recently been shown in an electron microscopy (EM) reconstruction of the CX. Considering this connectivity and the fact that mainly the R5 neurons synapse onto the R3 neurons, these last neurons may be inhibited by the former ones via GABA. A structural connectivity between R2 and E-PG neurons has been shown in GRASP, trans-Tango and in EM reconstructions as well (Omoto et al., 2018; Kottler et al., 2019; Hulse et al., 2021). The R2 neurons would inhibit the E-PG neurons via GABAA receptor. On this matter, the R70F05 line (Jenett et al., 2012) has the putative enhancer fragment corresponding to Rdl and it targets the E-PG neurons. A corroboration of the fact that E-PG neurons express Rdl receptors comes from RNA-seq experiments (Turner-Evans et al., 2020). In this sense, R2 neurons, after being activated by the visual system via nAChR, would globally inhibit the E-PG neurons but not uniformly so as to push the bump of activity towards the E-PG neurons that are the least inhibited (Fisher et al., 2019; Kim et al., 2019). In other words, since the inhibition of the R2 neurons towards the E-PG neurons is not equally distributed around the toroid, the excitatory drive arriving to the E-PG neurons would activate the less inhibited ones. In parallel, the E-PG neurons would combine the visual information with proprioceptive inputs arriving onto the PB and, in turn, sent to the EB through the P-EN neurons which update the fly’s heading (Green and Maimon, 2018). Similarly, the P-EG neurons, which may receive motor efference copies or proprioceptive inputs in the PB from other neurons, inhibit the E-PG neurons by passing through a class of GABAergic interneurons defined GB-Eo (GA-BU-outer EB) which receive inputs in the GA region and send outputs in the EB (Franconville et al., 2018). An important bottleneck of this complex circuit is represented by the Δ7 neurons which receive inputs and relay them within the PB (Wolff et al., 2015). These glutamatergic neurons receive activating inputs from the E-PG neurons and their activation stabilizes the heading representation by inhibiting—likely through GluC1α channels—only the subset of E-PG within one column in the PB (Franconville et al., 2018; Turner-Evans et al., 2020). This means that the bump of activity in Δ7 neurons has exactly the opposite angular orientation as the E-PG bump.
In our study, the E-PG neurons have shown to be modulated by dopamine which acts on two different subtypes of dopamine 1-like receptors, Dop1R1 and Dop1R2. Although an indirect dopamine modulation of the E-PG neurons remains a possible confounding effect, our data suggest that the Dop1R1 may work by shaping a sharp response in the E-PG neurons. Whereas, the Dop1R2 may work by decreasing the overall response. A dopamine modulation of E-PG neurons via Dop1R1 has also been demonstrated in behavioral experiments (Kottler et al., 2019). The functional role played by the dopamine in the EB might be to improve the signal-to-noise ratio in order to increase the robustness of the activity with respect to interfering input as shown in a network model of the human prefrontal cortex (Durstewitz et al., 2000). In the context of goal-directed behaviors, in order to accomplish the action selected, flies committed to reach a specific visual target might be induced to ignore distracting visual stimuli, through dopamine modulation of the EB (Frighetto et al., 2019). In patients with Parkinson’s disease, dopaminergic medication has shown to reduce interference effects on the motor program elicited by a target object when distracter objects evoke competitive motor programs (Castiello et al., 2000). The dopaminergic neurons would gate and invigorate movements that are planned somewhere else, likely in the cortex (Klaus et al., 2019). In primates, basal ganglia have shown to confirm the commitment to the decision by providing a signal that modulates the gain of how sensory evidence influences the cortex (Thura and Cisek, 2017). Therefore, even though the basal ganglia may not be the place where a specific motor plan is selected, they would still be needed for initiating it and for committing to it.
In flies, it is difficult to discretely localize the locus in which action selection may be implemented. However, the structure in which the vast majority of different inputs converges—including the ones from the mushroom-bodies (Zhang et al., 2013)—and that recursively interacts with them, is the EB. A recurrent networking among different sensory inputs at the basis of the integration would produce a sort of attentional focus as a byproduct, and this would serve the selection of action (Krauzlis et al., 2014; de Bivort and van Swinderen, 2016). Future work combining Ca2+ imaging and optogenetic manipulation in behaving flies, will probably disentangle this complex and fascinating issue.
Data Availability Statement
The original contributions presented in the study are included in the article/Supplementary Material, further inquiries can be directed to the corresponding author.
Author Contributions
GF, MZ, UC, AM, and J-RM designed the study. GF performed the experiments and analyzed the data. GF and J-RM wrote the manuscript. J-RM supervised the whole study. All authors contributed to the final version of the manuscript, and approved the submitted version.
Funding
The present work was carried out within the scope of the research program Dipartimenti di Eccellenza (art.1, commi 314-337 legge 232/2016), which was supported by a grant from MIUR to the Department of General Psychology, University of Padova. It was also supported by the Brainscope Program (ANR) to J-RM, Université Paris-Saclay, CNRS, Institut des Neurosciences Paris-Saclay (NeuroPSI, UMR-9197), Saclay, France.
Conflict of Interest
The authors declare that the research was conducted in the absence of any commercial or financial relationships that could be construed as a potential conflict of interest.
Publisher’s Note
All claims expressed in this article are solely those of the authors and do not necessarily represent those of their affiliated organizations, or those of the publisher, the editors and the reviewers. Any product that may be evaluated in this article, or claim that may be made by its manufacturer, is not guaranteed or endorsed by the publisher.
Acknowledgments
We thank Paola Cisotto for the technical preparation of genetic crossings and Lesly Palacios Castillo for language editing and helpful comments on the manuscript.
Supplementary Material
The Supplementary Material for this article can be found online at: https://www.frontiersin.org/articles/10.3389/fphys.2022.849142/full#supplementary-material
References
Baker M. (2016). 1,500 Scientists Lift the Lid on Reproducibility. Nature 533, 452–454. doi:10.1038/533452a
Bates D., Mächler M., Bolker B., Walker S. (2014). Fitting Linear Mixed-Effects Models Using Lme4. ArXiv14065823 Stat. Available at: http://arxiv.org/abs/1406.5823 Accessed February 21, 2022.
Bates D. (2010). lme4: Mixed-Effects Modeling with R. Available at: https://lme4.r-forge.r-project.org/book/ Accessed February 21, 2022.
Baubet V., Le Mouellic H., Campbell A. K., Lucas-Meunier E., Fossier P., Brulet P. (2000). Chimeric green Fluorescent Protein-Aequorin as Bioluminescent Ca2+ Reporters at the Single-Cell Level. Proc. Natl. Acad. Sci. 97, 7260–7265. doi:10.1073/pnas.97.13.7260
Castiello U., Bonfiglioli C., Peppard R. F. (2000). Dopaminergic Effects on the Implicit Processing of Distractor Objects in Parkinson's Disease. Exp. Brain Res. 135, 251–258. doi:10.1007/s002210000510
Dan C., Hulse B. K., Jayaraman V., Hermundstad A. M. (2021). Flexible Control of Behavioral Variability Mediated by an Internal Representation of Head Direction. bioRxiv. doi:10.1101/2021.08.18.456004
de Bivort B. L., van Swinderen B. (2016). Evidence for Selective Attention in the Insect Brain. Curr. Opin. Insect Sci. 15, 9–15. doi:10.1016/j.cois.2016.02.007
DeBruine L. M., Barr D. J. (2021). Understanding Mixed-Effects Models through Data Simulation. Adv. Methods Practices Psychol. Sci. 4, 251524592096511. doi:10.1177/2515245920965119
DeLong M. R. (1990). Primate Models of Movement Disorders of Basal Ganglia Origin. Trends Neurosci. 13, 281–285. doi:10.1016/0166-2236(90)90110-v
Dietzl G., Chen D., Schnorrer F., Su K.-C., Barinova Y., Fellner M., et al. (2007). A Genome-wide Transgenic RNAi Library for Conditional Gene Inactivation in Drosophila. Nature 448, 151–156. doi:10.1038/nature05954
Donlea J. M., Pimentel D., Talbot C. B., Kempf A., Omoto J. J., Hartenstein V., et al. (2018). Recurrent Circuitry for Balancing Sleep Need and Sleep. Neuron 97, 378–389. doi:10.1016/j.neuron.2017.12.016
Draper I., Kurshan P. T., McBride E., Jackson F. R., Kopin A. S. (2007). Locomotor Activity Is Regulated by D2-like Receptors in Drosophila: an Anatomic and Functional Analysis. Devel. Neurobio. 67, 378–393. doi:10.1002/dneu.20355
Durstewitz D., Seamans J. K., Sejnowski T. J. (2000). Dopamine-mediated Stabilization of Delay-Period Activity in a Network Model of Prefrontal Cortex. J. Neurophysiol. 83, 1733–1750. doi:10.1152/jn.2000.83.3.1733
Ericsson J., Silberberg G., Robertson B., Wikström M. A., Grillner S. (2011). Striatal Cellular Properties Conserved from Lampreys to Mammals. J. Physiol. 589, 2979–2992. doi:10.1113/jphysiol.2011.209643
Ericsson J., Stephenson-Jones M., Perez-Fernandez J., Robertson B., Silberberg G., Grillner S. (2013). Dopamine Differentially Modulates the Excitability of Striatal Neurons of the Direct and Indirect Pathways in Lamprey. J. Neurosci. 33, 8045–8054. doi:10.1523/JNEUROSCI.5881-12.2013
Farries M. A. (2013). How 'Basal' Are the Basal Ganglia? Brain Behav. Evol. 82, 211–214. doi:10.1159/000356101
Fiore V. G., Dolan R. J., Strausfeld N. J., Hirth F. (2015). Evolutionarily Conserved Mechanisms for the Selection and Maintenance of Behavioural Activity. Phil. Trans. R. Soc. B 370, 20150053. doi:10.1098/rstb.2015.0053
Fisher Y. E., Lu J., D’Alessandro I., Wilson R. I. (2019). Sensorimotor Experience Remaps Visual Input to a Heading-Direction Network. Nature 576, 121–125. doi:10.1038/s41586-019-1772-4
Franconville R., Beron C., Jayaraman V. (2018). Building a Functional Connectome of the Drosophila central Complex. eLife 7, e37017. doi:10.7554/eLife.37017
Frighetto G., Zordan M. A., Castiello U., Megighian A. (2019). Action-based Attention in Drosophila melanogaster. J. Neurophysiol. 121, 2428–2432. doi:10.1152/jn.00164.2019
Gelman A., Hill J. (2007). Data Analysis Using Regression and Multilevel/Hierarchical Models. New York, NY: Cambridge University Press.
Green J., Maimon G. (2018). Building a Heading Signal from Anatomically Defined Neuron Types in the Drosophila central Complex. Curr. Opin. Neurobiol. 52, 156–164. doi:10.1016/j.conb.2018.06.010
Green E. W., Fedele G., Giorgini F., Kyriacou C. P. (2014). A Drosophila RNAi Collection Is Subject to Dominant Phenotypic Effects. Nat. Methods 11, 222–223. doi:10.1038/nmeth.2856
Green J., Adachi A., Shah K. K., Hirokawa J. D., Magani P. S., Maimon G. (2017). A Neural Circuit Architecture for Angular Integration in Drosophila. Nature 546, 101–106. doi:10.1038/nature22343
Green J., Vijayan V., Mussells Pires P., Adachi A., Maimon G. (2019). A Neural Heading Estimate Is Compared with an Internal Goal to Guide Oriented Navigation. Nat. Neurosci. 22, 1460–1468. doi:10.1038/s41593-019-0444-x
Grillner S., Robertson B. (2016). The Basal Ganglia over 500 Million Years. Curr. Biol. 26, R1088–R1100. doi:10.1016/j.cub.2016.06.041
Grillner S., Hellgren J., Menard A., Saitoh K., Wikstrom M. (2005). Mechanisms for Selection of Basic Motor Programs - Roles for the Striatum and Pallidum. Trends Neurosci. 28, 364–370. doi:10.1016/j.tins.2005.05.004
Hanesch U., Fischbach K.-F., Heisenberg M. (1989). Neuronal Architecture of the central Complex in Drosophila melanogaster. Cell Tissue Res. 257, 343–366. doi:10.1007/BF00261838
Hardcastle B. J., Omoto J. J., Kandimalla P., Nguyen B.-C. M., Keleş M. F., Boyd N. K., et al. (2021). A Visual Pathway for Skylight Polarization Processing in Drosophila. eLife 10, e63225. doi:10.7554/eLife.63225
Hulse B. K., Haberkern H., Franconville R., Turner-Evans D. B., Takemura S.-Y., Wolff T., et al. (2021). A Connectome of the Drosophila central Complex Reveals Network Motifs Suitable for Flexible Navigation and Context-dependent Action Selection. eLife 10, e66039. doi:10.7554/eLife.66039
Humphries M., Khamassi M., Gurney K. (2012). Dopaminergic Control of the Exploration-Exploitation Trade-Off via the Basal Ganglia. Front. Neurosci. 6, 9. doi:10.3389/fnins.2012.00009
Isaacman-Beck J., Paik K. C., Wienecke C. F. R., Yang H. H., Fisher Y. E., Wang I. E., et al. (2020). SPARC Enables Genetic Manipulation of Precise Proportions of Cells. Nat. Neurosci. 23, 1168–1175. doi:10.1038/s41593-020-0668-9
Jenett A., Rubin G. M., Ngo T.-T. B., Shepherd D., Murphy C., Dionne H., et al. (2012). A GAL4-Driver Line Resource for Drosophila Neurobiology. Cel Rep. 2, 991–1001. doi:10.1016/j.celrep.2012.09.011
Kim S. S., Hermundstad A. M., Romani S., Abbott L. F., Jayaraman V. (2019). Generation of Stable Heading Representations in Diverse Visual Scenes. Nature 576, 126–131. doi:10.1038/s41586-019-1767-1
Klaus A., Alves da Silva J., Costa R. M. (2019). What, if, and when to Move: Basal Ganglia Circuits and Self-Paced Action Initiation. Annu. Rev. Neurosci. 42, 459–483. doi:10.1146/annurev-neuro-072116-031033
Kong E. C., Woo K., Li H., Lebestky T., Mayer N., Sniffen M. R., et al. (2010). A Pair of Dopamine Neurons Target the D1-like Dopamine Receptor DopR in the central Complex to Promote Ethanol-Stimulated Locomotion in Drosophila. PLoS One 5, e9954. doi:10.1371/journal.pone.0009954
Kottler B., Fiore V. G., Ludlow Z. N., Buhl E., Vinatier G., Faville R., et al. (2017). A Lineage-Related Reciprocal Inhibition Circuitry for Sensory-Motor Action Selection. bioRxiv, 100420. doi:10.1101/100420
Kottler B., Faville R., Bridi J. C., Hirth F. (2019). Inverse Control of Turning Behavior by Dopamine D1 Receptor Signaling in Columnar and Ring Neurons of the Central Complex in Drosophila. Curr. Biol. 29, 567–577. doi:10.1016/j.cub.2019.01.017
Krauzlis R. J., Bollimunta A., Arcizet F., Wang L. (2014). Attention as an Effect Not a Cause. Trends Cogn. Sci. 18, 457–464. doi:10.1016/j.tics.2014.05.008
Lark A. R., Kitamoto T., Martin J.-R. (2016). In Vivo Functional Brain Imaging Approach Based on Bioluminescent Calcium Indicator GFP-Aequorin. JoVE (107), 53705. doi:10.3791/53705
Lebestky T., Chang J.-S. C., Dankert H., Zelnik L., Kim Y.-C., Han K.-A., et al. (2009). Two Different Forms of Arousal in Drosophila Are Oppositely Regulated by the Dopamine D1 Receptor Ortholog DopR via Distinct Neural Circuits. Neuron 64, 522–536. doi:10.1016/j.neuron.2009.09.031
Lenth R. V., Buerkner P., Herve M., Love J., Miguez F., Riebl H., et al. (2022). Emmeans: Estimated Marginal Means, Aka Least-Squares Means. Available at: https://CRAN.R-project.org/package=emmeans Accessed February 21, 2022.
Liang X., Ho M. C. W., Zhang Y., Li Y., Wu M. N., Holy T. E., et al. (2019). Morning and Evening Circadian Pacemakers Independently Drive Premotor Centers via a Specific Dopamine Relay. Neuron 102, 843–857. doi:10.1016/j.neuron.2019.03.028
Liu S., Liu Q., Tabuchi M., Wu M. N. (2016). Sleep Drive Is Encoded by Neural Plastic Changes in a Dedicated Circuit. Cell 165, 1347–1360. doi:10.1016/j.cell.2016.04.013
Martin J.-R., Rogers K. L., Chagneau C., Brûlet P. (2007). In Vivo Bioluminescence Imaging of Ca2+ Signalling in the Brain of Drosophila. PLoS One 2, e275. doi:10.1371/journal.pone.0000275
Martin J.-R. (2008). In VivoBrain Imaging: Fluorescence or Bioluminescence, Which to Choose? J. Neurogenet. 22, 285–307. doi:10.1080/01677060802298517
Nelson A. B., Kreitzer A. C. (2014). Reassessing Models of Basal Ganglia Function and Dysfunction. Annu. Rev. Neurosci. 37, 117–135. doi:10.1146/annurev-neuro-071013-013916
Omoto J. J., Keleş M. F., Nguyen B.-C. M., Bolanos C., Lovick J. K., Frye M. A., et al. (2017). Visual Input to the Drosophila Central Complex by Developmentally and Functionally Distinct Neuronal Populations. Curr. Biol. 27, 1098–1110. doi:10.1016/j.cub.2017.02.063
Omoto J. J., Nguyen B.-C. M., Kandimalla P., Lovick J. K., Donlea J. M., Hartenstein V. (2018). Neuronal Constituents and Putative Interactions within the Drosophila Ellipsoid Body Neuropil. Front. Neural Circuits 12, 103. doi:10.3389/fncir.2018.00103
Pavot P., Carbognin E., Martin J.-R. (2015). PKA and cAMP/CNG Channels Independently Regulate the Cholinergic Ca2+-Response of Drosophila Mushroom Body Neurons. eNeuro 2, 0054–114. doi:10.1523/ENEURO.0054-14.2015
Pfeiffer K., Homberg U. (2014). Organization and Functional Roles of the central Complex in the Insect Brain. Annu. Rev. Entomol. 59, 165–184. doi:10.1146/annurev-ento-011613-162031
Pfeiffer B. D., Ngo T.-T. B., Hibbard K. L., Murphy C., Jenett A., Truman J. W., et al. (2010). Refinement of Tools for Targeted Gene Expression in Drosophila. Genetics 186, 735–755. doi:10.1534/genetics.110.119917
Pimentel D., Donlea J. M., Talbot C. B., Song S. M., Thurston A. J. F., Miesenböck G. (2016). Operation of a Homeostatic Sleep Switch. Nature 536, 333–337. doi:10.1038/nature19055
Raftery A. E. (1995). Bayesian Model Selection in Social Research. Sociol. Methodol. 25, 111–163. doi:10.2307/271063
Redgrave P., Prescott T. J., Gurney K. (1999). The Basal Ganglia: a Vertebrate Solution to the Selection Problem? Neuroscience 89, 1009–1023. doi:10.1016/s0306-4522(98)00319-4
Redgrave P., Rodriguez M., Smith Y., Rodriguez-Oroz M. C., Lehericy S., Bergman H., et al. (2010). Goal-directed and Habitual Control in the Basal Ganglia: Implications for Parkinson's Disease. Nat. Rev. Neurosci. 11, 760–772. doi:10.1038/nrn2915
Robie A. A., Hirokawa J., Edwards A. W., Umayam L. A., Lee A., Phillips M. L., et al. (2017). Mapping the Neural Substrates of Behavior. Cell 170, 393–406. doi:10.1016/j.cell.2017.06.032
RStudio Team (2017). RStudio: Integrated Development for R. Boston, MA, USA: RStudio, Inc. Available at: https://www.rstudio.com/home/.
Saravanan V., Berman G. J., Sober S. J. (2020). Application of the Hierarchical Bootstrap to Multi-Level Data in Neuroscience. Neurons Behav. Data Anal. Theor. 3. Available at: https://nbdt.scholasticahq.com/article/13927-application-of-the-hierarchical-bootstrap-to-multi-level-data-in-neuroscience. doi:10.48550/arXiv.2007.07797
Schwarz G. (1978). Estimating the Dimension of a Model. Ann. Stat. 6, 461–464. doi:10.1214/aos/1176344136
Seelig J. D., Jayaraman V. (2013). Feature Detection and Orientation Tuning in the Drosophila central Complex. Nature 503, 262–266. doi:10.1038/nature12601
Seelig J. D., Jayaraman V. (2015). Neural Dynamics for Landmark Orientation and Angular Path Integration. Nature 521, 186–191. doi:10.1038/nature14446
Shiozaki H. M., Kazama H. (2017). Parallel Encoding of Recent Visual Experience and Self-Motion during Navigation in Drosophila. Nat. Neurosci. 20, 1395–1403. doi:10.1038/nn.4628
Stephenson-Jones M., Samuelsson E., Ericsson J., Robertson B., Grillner S. (2011). Evolutionary Conservation of the Basal Ganglia as a Common Vertebrate Mechanism for Action Selection. Curr. Biol. 21, 1081–1091. doi:10.1016/j.cub.2011.05.001
Stephenson-Jones M., Ericsson J., Robertson B., Grillner S. (2012). Evolution of the Basal Ganglia: Dual-Output Pathways Conserved throughout Vertebrate Phylogeny. J. Comp. Neurol. 520, 2957–2973. doi:10.1002/cne.23087
Stephenson-Jones M., Kardamakis A. A., Robertson B., Grillner S. (2013). Independent Circuits in the Basal Ganglia for the Evaluation and Selection of Actions. Proc. Natl. Acad. Sci. U.S.A. 110, E3670–E3679. doi:10.1073/pnas.1314815110
Strausfeld N. J., Hirth F. (2013a). Deep Homology of Arthropod central Complex and Vertebrate Basal Ganglia. Science 340, 157–161. doi:10.1126/science.1231828
Strausfeld N. J., Hirth F. (2013b). Homology versus Convergence in Resolving Transphyletic Correspondences of Brain Organization. Brain Behav. Evol. 82, 215–219. doi:10.1159/000356102
Sun Y., Nern A., Franconville R., Dana H., Schreiter E. R., Looger L. L., et al. (2017). Neural Signatures of Dynamic Stimulus Selection in Drosophila. Nat. Neurosci. 20, 1104–1113. doi:10.1038/nn.4581
Thura D., Cisek P. (2017). The Basal Ganglia Do Not Select Reach Targets but Control the Urgency of Commitment. Neuron 95, 1160–1170. doi:10.1016/j.neuron.2017.07.039
Turner-Evans D., Wegener S., Rouault H., Franconville R., Wolff T., Seelig J. D., et al. (2017). Angular Velocity Integration in a Fly Heading Circuit. eLife 6, e23496. doi:10.7554/eLife.23496
Turner-Evans D. B., Jensen K. T., Ali S., Paterson T., Sheridan A., Ray R. P., et al. (2020). The Neuroanatomical Ultrastructure and Function of a Biological Ring Attractor. Neuron 108, 145–163. doi:10.1016/j.neuron.2020.08.006
Wolff T., Rubin G. M. (2018). Neuroarchitecture of the Drosophila central Complex: A Catalog of Nodulus and Asymmetrical Body Neurons and a Revision of the Protocerebral Bridge Catalog. J. Comp. Neurol. 526, 2585–2611. doi:10.1002/cne.24512
Wolff T., Iyer N. A., Rubin G. M. (2015). Neuroarchitecture and Neuroanatomy of the Drosophila central Complex: A GAL4‐based Dissection of Protocerebral Bridge Neurons and Circuits. J. Comp. Neurol. 523, 997–1037. doi:10.1002/cne.23705
Xiong T. C., Ronzier E., Sanchez F., Corratgé-Faillie C., Mazars C., Thibaud J.-B. (2014). Imaging Long Distance Propagating Calcium Signals in Intact Plant Leaves with the BRET-Based GFP-Aequorin Reporter. Front. Plant Sci. 5, 43. doi:10.3389/fpls.2014.00043
Keywords: central complex, functional calcium brain imaging, dopamine 1-like receptors, action selection, nicotine response, basal ganglia
Citation: Frighetto G, Zordan MA, Castiello U, Megighian A and Martin J-R (2022) Dopamine Modulation of Drosophila Ellipsoid Body Neurons, a Nod to the Mammalian Basal Ganglia. Front. Physiol. 13:849142. doi: 10.3389/fphys.2022.849142
Received: 05 January 2022; Accepted: 10 March 2022;
Published: 14 April 2022.
Edited by:
Jose Eduardo Serrão, Universidade Federal de Viçosa, BrazilReviewed by:
Andrew Dacks, West Virginia University, United StatesAngelique Christine Paulk, Massachusetts General Hospital and Harvard Medical School, United States
Copyright © 2022 Frighetto, Zordan, Castiello, Megighian and Martin. This is an open-access article distributed under the terms of the Creative Commons Attribution License (CC BY). The use, distribution or reproduction in other forums is permitted, provided the original author(s) and the copyright owner(s) are credited and that the original publication in this journal is cited, in accordance with accepted academic practice. No use, distribution or reproduction is permitted which does not comply with these terms.
*Correspondence: Jean-René Martin, amVhbi1yZW5lLm1hcnRpbkBjbnJzLmZy
†Present address: Giovanni Frighetto, Department of Integrative Biology and Physiology, University of California, Los Angeles, Los Angeles, CA, United States