- 1Department of Genetic Medicine, King Abdulaziz University, Jeddah, Saudi Arabia
- 2Department of Laboratory Medicine, Faculty of Applied Medical Sciences, Umm Al-Qura University, Makkah, Saudi Arabia
- 3Princess Al-Jawhara Center of Excellence in Research of Hereditary Disorders (PACER-HD), King Abdulaziz University, Jeddah, Saudi Arabia
- 4Department of Biology, Faculty of Science, University of Hafr Al Batin, Hafar Al-Batin, Saudi Arabia
- 5Department of Medical Laboratory Sciences, Faculty of Applied Medical Sciences, King Abdulaziz University, Jeddah, Saudi Arabia
Background: Coronavirus disease (COVID-19) infection is known for its severe clinical pathogenesis among individuals with pre-existing comorbidities. However, the molecular basis of this observation remains elusive. Thus, this study aimed to map key genes and pathway alterations in patients with COVID-19 and comorbidities using robust systems biology approaches.
Methods: The publicly available genome-wide transcriptomic datasets from 120 COVID-19 patients, 281 patients suffering from different comorbidities (like cardiovascular diseases, atherosclerosis, diabetes, and obesity), and 252 patients with different infectious diseases of the lung (respiratory syncytial virus, influenza, and MERS) were studied using a range of systems biology approaches like differential gene expression, gene ontology (GO), pathway enrichment, functional similarity, mouse phenotypic analysis and drug target identification.
Results: By cross-mapping the differentially expressed genes (DEGs) across different datasets, we mapped 274 shared genes to severe symptoms of COVID-19 patients or with comorbidities alone. GO terms and functional pathway analysis highlighted genes in dysregulated pathways of immune response, interleukin signaling, FCGR activation, regulation of cytokines, chemokines secretion, and leukocyte migration. Using network topology parameters, phenotype associations, and functional similarity analysis with ACE2 and TMPRSS2—two key receptors for this virus-we identified 17 genes with high connectivity (CXCL10, IDO1, LEPR, MME, PTAFR, PTGS2, MAOB, PDE4B, PLA2G2A, COL5A1, ICAM1, SERPINE1, ABCB1, IL1R1, ITGAL, NCAM1 and PRKD1) potentially contributing to the clinical severity of COVID-19 infection in patients with comorbidities. These genes are predicted to be tractable and/or with many existing approved inhibitors, modulators, and enzymes as drugs.
Conclusion: By systemic implementation of computational methods, this study identified potential candidate genes and pathways likely to confer disease severity in COVID-19 patients with pre-existing comorbidities. Our findings pave the way to develop targeted repurposed therapies in COVID-19 patients.
Introduction
Severe Acute Respiratory Syndrome Coronavirus 2 (SARS-CoV-2), commonly known as coronavirus disease (COVID-19) imposed an unprecedented challenge to the global economy, lifestyle and public healthcare. COVID-19 patients, in general, show a variable spectrum of clinical phenotypes, ranging from asymptomatic to symptomatic with mild to severe acute respiratory distress syndrome (ARDS). SARS-CoV-2 virus infects all age groups, but those over 60 years of age, especially those with a history of cardiovascular disease (CVD), obesity, diabetes, or respiratory diseases, are at an increased risk of developing severe clinical complications (Goyal et al., 2020; Grasselli et al., 2020; Holman et al., 2020; Zhou et al., 2020).
CVD is strongly linked to higher SARS-CoV-1, SARS-CoV-2, and MERS infection mortality rates across many countries (Madjid et al., 2020; Zhou et al., 2020). Several studies have found that COVID-19 patients with CVD have not only severe infection-related symptoms but also myocardial damage and arrhythmia (Madjid et al., 2020; Shi et al., 2020). Interestingly, some reports also suggest the association of cardiovascular complications like thromboembolic events, myocarditis, acute coronary syndrome, and heart failure in COVID-19 patients with no prior CVD (Wu and McGoogan, 2020).
Diabetes is another comorbidity strongly associated with the severity of COVID-19 infection. It is known that people with diabetes are inclined to contract viral infections due to impaired immune responses. Further, impaired T cell function, increased release of inflammatory mediators, especially TNFα and IL-1β, and elevated levels of ACE2 also played a crucial role in developing severe COVID-19 symptoms and predisposed to higher mortality risk (Fang et al., 2020; Fox et al., 2021). Both CVD and diabetes are strongly linked to obesity, a risk factor that determines the clinical complications in COVID-19 patients (Malik et al., 2021). Thus, all these risk factors modulate the severity of COVID-19 and drastically reduce the patient survival rate (Hernandez-Garduno, 2020).
The pro-inflammatory cytokine status compromises innate immune response, and increased expression of the ACE2 receptor is the key molecular feature of COVID-19 and other chronic morbidity outcomes. Other molecular factors that determine the risk and severity of COVID-19 infection are frequently mentioned (Dolan et al., 2020; Facchiano et al., 2020; Singh et al., 2021). However, biological mechanisms and specific biomarkers underlying COVID-19 with associated comorbidities are poorly understood and are still being investigated. Understanding these biological mechanisms may help in developing preventive and therapeutic strategies against COVID-19 infection in patients with such comorbidities.
The unrestricted access to genome-wide transcriptome and viral genome data has accelerated the novel scientific discoveries related to COVID-19 infection and treatments. However, this exponential growth of gene expression datasets, which largely varies in experimental designs, sample sizes, array platforms, statistical methods, and analytic findings, presents both opportunities as well as challenges to biologists in identifying novel molecular drug targets. In recent years, multidimensional computational approaches have proven their robustness, efficiency, and applicability in exploring diverse large-scale biological datasets (Banaganapalli et al., 2020; Mujalli et al., 2020). These computational methods were developed using machine learning techniques and were trained on massive amounts of multi-omic (genomics, transcriptomics, proteomics, and metabolomics) datasets. Using comprehensive computational methods, our gene expression meta-analysis aims to map the unique and shared genes and pathways from transcriptomic datasets of COVID-19 patients (with severe or fatal phenotype) suffering from chronic diseases (CVD, diabetes, obesity, and lung diseases). This study may provide a novel insight into a better understanding of why COVID-19 patients with pre-existing comorbidities are more likely to develop severe clinical complications as well.
Methods
Datasets selection
Microarray transcriptome data from 120 COVID-19 (critical, severe, and deceased status) and from 281 patients with chronic morbidities (153 CVD, 64 atherosclerosis, 33 diabetes, and 31 obesity) were extracted from the Gene Expression Omnibus (GEO) (https://www.ncbi.nlm.nih.gov/geo/) and China National GeneBank databases (https://db.cngb.org/). Datasets from other lung viral infections such as respiratory syncytial virus (RSV, 145), influenza (95), and MERS (12) were also compared with COVID-19 in order to reduce the background noise signals by excluding common viral infection biomarkers. The full details of biological specimens, RNA isolations, hybridizations, and data analysis methods are mentioned in their corresponding publications. The full details of the gene expression datasets used in this study are shown in Supplementary Table S1.
Raw CEL files processing, normalization, filtration and differentially expressed genes identification
Each gene expression dataset initially underwent preprocessing, normalization, and filtration separately using the robust multiarray average algorithm in R package software version 4; (http://www.R-project.org/). The robust multiarray average (RMA) in R-affy and Lumi packages, exclusively built for the Illumina microarray platform, were used to perform background data correction and normalization. The RMA technique was chosen over other packages due to its power to detect little differential change, stable variance on a log scale, and minimize false-positive results. Then the limma package was used to select DEGs and paired t-test to analyze the statistical significance of the observed DEG patterns. The Benjamini–Hochberg correction method was used for p-value adjustment to the false discovery rate (FDR). The cutoff point for DEG selection was set at FDR 0.05 and |log2FC| > 1.5. The non-variant probes, the housekeeping probes, and those that are not assigned to any gene were filtered out, leaving known curated gene sets with the variant probes for further analysis.
Identification of differentially expressed genes which are unique and/or shared across different categories of the diseases
To identify both unique and shared genes in comorbidities and COVID-19, all DEGs, including upregulated and downregulated genes, were assigned to their respective category. Shared genes were then identified using the VENN tool, available in VENNY 2.1 (https://bioinfogp.cnb.csic.es/tools/venny/). At first, the “unique DEGs for comorbidities group” were identified by intersecting genes between comorbidities and respiratory viral infections like RSV, MERS, etc. Then, the “unique DEGs to COVID-19” were identified by intersecting genes between COVID-19 and other viral lung infections. Finally, unique DEGs from both groups were compared against each other to identify shared genes between the comorbidities set and COVID-19 sets (Banaganapalli et al., 2021).
Functional analysis of category-specific differentially expressed genes (DEGs)
To explore the pathways shared between DEGs, all the genes were first grouped into three categories: COVID-19, chronic comorbidities, and lung infections. Further Enriched Clustering Ontology analysis of each DEG group was performed using the web-based tool Metascape (https://metascape.org/).
Cell/tissue-specific expression of the identified genes
In order to determine cell and tissue distribution of the identified genes and also to generate visualization summaries of gene functions, all the query gene lists were uploaded to three different online web tools. ARCHS4 web-tool resource in EnrichR (http://amp.pharm.mssm.edu/Enrichr) contains most published RNA-seq data from humans and mice. TSEA web tool (http://genetics.wustl.edu/jdlab/tsea/) uses RNA-seq data from 45 tissues collected from 189 individuals, and the PaGenBase database in Metascape (http://metascape.org) combines gene expression patterns from literature and data mining.
Target genes prediction and ACE2/TMPRSS2 similarity analysis
The Open Target Platform is an online portal (https://www.opentargets.org/) that was used to retrieve associated drug targets. This platform contains information about the pharmacological targets of drugs used in clinical practice. Measuring gene functional similarity is an essential tool for gene clustering, function prediction, and disease identification. We performed the correlation between gene expression and gene functional similarity of the query genes as a group with ACE2 and TMPRSS2 using the open-source tool eXploring-Genomic Relations for enhanced interpretation web tool (XGR) (http://galahad.well.ox.ac.uk:3040/). We considered the best-match score of 0.5 or above for selecting the gene-drug association.
Causal signaling network analysis
The interaction network of genes associated with ACE2 and TMPRSS2 was built using the SIGnaling Network Open Resources (SIGNOR 2.0) (http://signor.uniroma2.it). The SIGNOR 3.0 web-based tool can be used to predict activation or inactivation, connections, and interactions between biomolecules and signaling molecules. All interactions with a relaxed layout and score of “0.3–0.7″ were selected.
Mouse phenotype enrichment analysis
The mouse phenotype model is an impactful resource for prioritizing key candidate genes involved in disease processes. In this study, we used the Mammalian Phenotype (MP) enrichment analysis in ToppGene (https://toppgene.cchmc.org/) to determine the associated phenotype of genes. Mouse phenotype characteristics associated with query gene knockout were acquired from the Mouse Genome informatics database (MGI) (http://www.informatics.jax.org/).
Results
Data preprocessing and differentially expressed genes identification
We initially collected gene expression datasets from COVID-19, comorbidities (CVD, atherosclerosis, diabetes, and obesity), and lung infection (RSV, influenza, and MERS) patients (Supplementary Table S1). Each dataset was preprocessed separately using appropriate R packages and algorithm implemented in the R program and the differences in the gene expression profiles between patients and healthy participants were evaluated. Figure 1 illustrates an overview of our experimental workflow. DEGs were identified and ranked for each dataset based on their log2FC and volcano plots across all processed datasets were generated (Figure 2). For COVID-19 datasets, only DEGs of severe, critical and critical and deceased patients against the healthy individuals were selected. Subsequently, upregulated and downregulated genes were then grouped and added to their respective category, as shown in Figure 3.
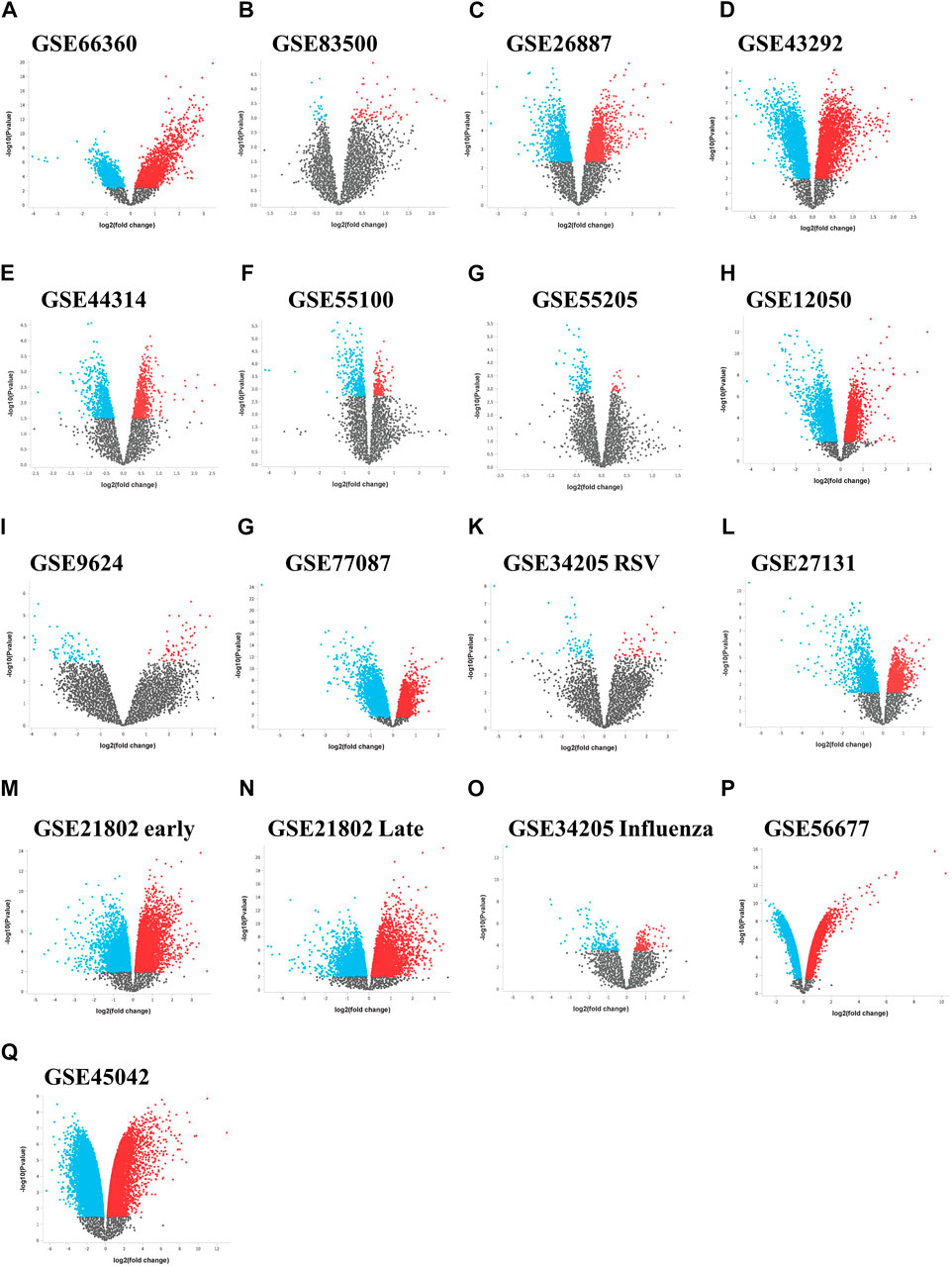
FIGURE 2. Differential expression volcano plots across data sets. Volcano plots of the gene expression data from (A–I) the comorbidities category, (G–M) lung infection category including influenza and (P,Q) MERS infection category. The horizontal axis represents the log2 (fold change) and the vertical axis represents the-log10 (p-value). The colored dots represent the significant DEGs with adjusted p-values < 0.05. DEGs, differentially expressed genes.
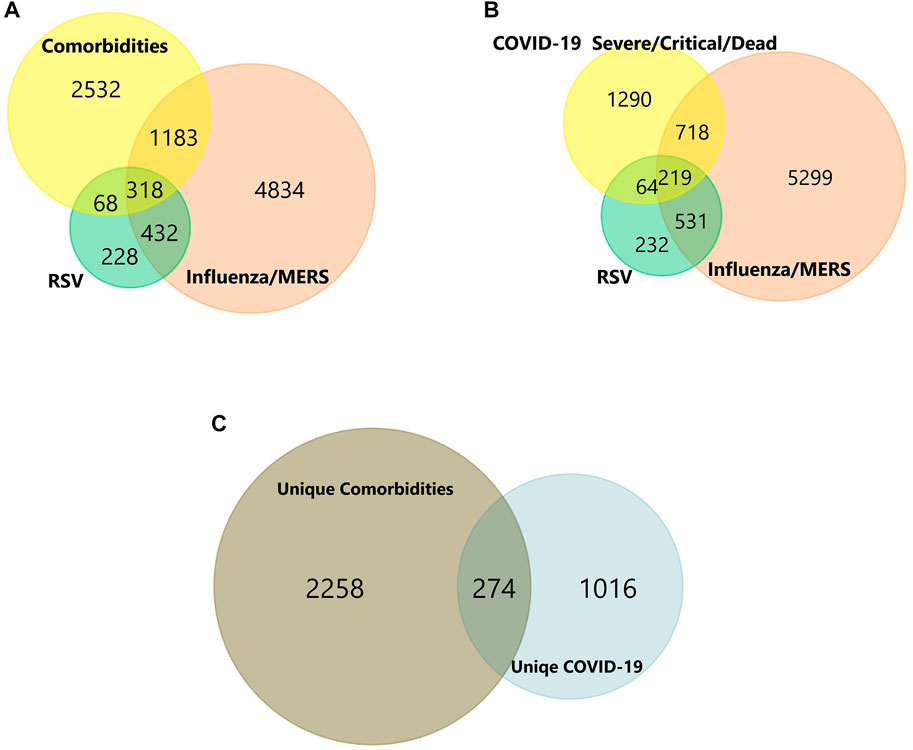
FIGURE 3. (A-C) Venn diagram of shared and unique genes of Comorbidities and COVID-19 infection. Colors represent different datasets. The overlapping areas are the shared DEGs. Statistically significant DEGs were defined with p < 0.05 and [log2FC] > 1.5 as the cut-off criterion.
Top shared differentially expressed genes across COVID-19 and comorbidities datasets
In total, the comorbidities category independently contains 4,092 significantly altered genes; 10,005 in the lung infection categories, of which 4,641 and 2,291 genes were mapped from MERS and COVID-19 datasets, respectively. To identify the genes that are unique to COVID-19 pathogenesis, we mapped the common genes between COVID-19 vs. comorbidities category and eliminated them. We initially compared other lung infection datasets to comorbidities category as well. The shared DEGs were excluded and unique to comorbidities DEGs were retained for further analysis. Subsequently, the COVID-19 dataset was also compared to the lung infection category and COVID-19 unique DEGs were selected for further analysis. Our analysis showed that 2,532 genes were unique to comorbidities and 1,290 genes were unique to COVID-19 (Figures 3A,B). These unique DEGs were intersected to identify 274 genes shared between comorbidities and severe COVID-19 datasets, suggesting their potential role in the clinical severity of COVID-19 (Figure 3C) with comorbidities. Protein-Protein Interaction (PPI) network of these genes was generated with Cytoscape 3.9.1 (Figure 4). Based on the computation of a comprehensive set of topological parameters and centrality measures with the Cytoscape plugin NetworkAnalyzer, we identified 13 top-ranked genes: ITGAX, ICAM1, CCL3, TYROB, PFCGR3B, PTGS2, CXCL10, GZMB, PLEK, ITGAL, NCAM1, KLRK1, and SERPINE1 (Figure 4).
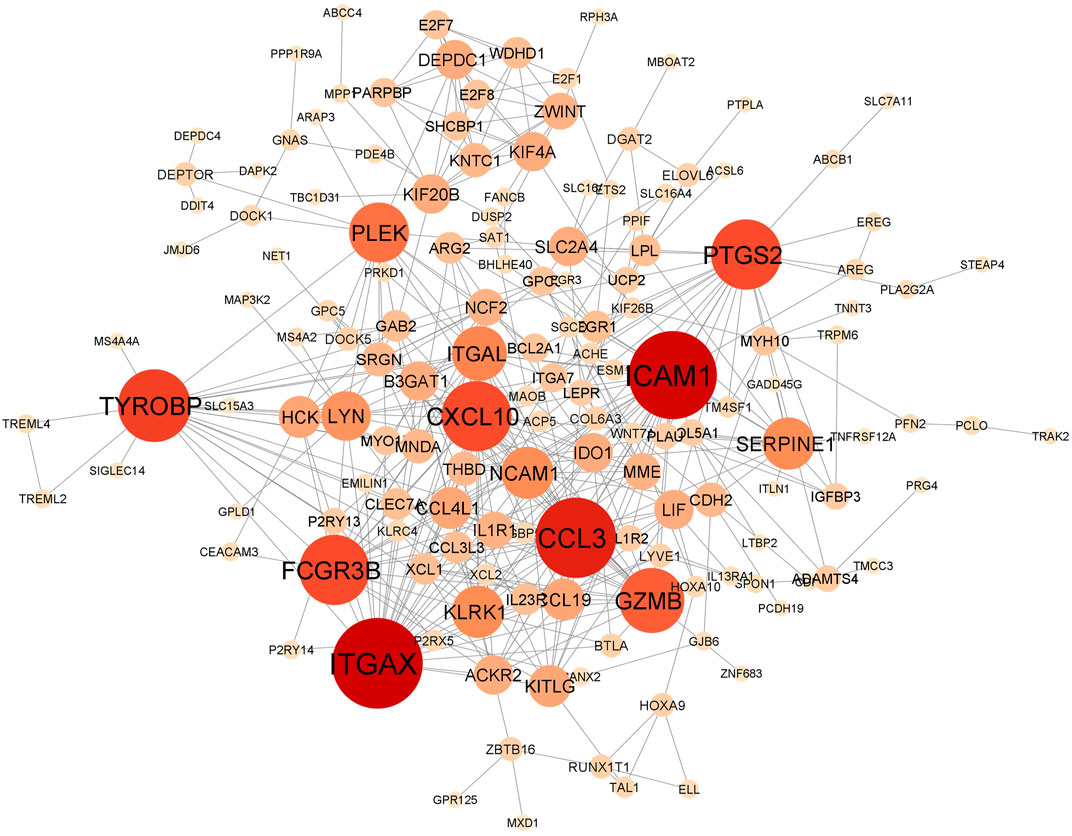
FIGURE 4. Protein-protein interaction network of 274 genes shared by the comorbidities and COVID-19 infections and classified by their degree of centrality. Node size is proportional to the degree of connection. Top gene, with at least 15 connections, includes ITGAX, ICAM1, CCL3, TYROB, PFCGR3B, PTGS2, CXCL10, GZMB, PLEK, ITGAL, NCAM1, KLRK1 and SERPINE1.
Cell and tissue-specific distribution of the identified genes
We investigated the tissue and organ specific expression of the 274 unique genes. Several genes have enriched expressions in multiple organs, tissues, or cell types where they play a role in transcriptional regulation, epigenetic modification, differentiation and pathogenesis. Three online Bioinformatics tools, EnrichR web-tool (http://amp.pharm.mssm.edu/Enrichr), TSEA web-tool (http://genetics.wustl.edu/jdlab/tsea) and PaGenBase database in Metascape (http://metascape.org) were used to determine whether the identified genes are lung-specific or also expressed by other tissues. Through ARCHS4 resource in ErichR, cells of origin of identified genes were identified. Our analysis found that 150 of the identified genes (54.75%) are enriched in lung, spleen, colon bulk tissue and omentum as well as neutrophil and macrophages (Figure 5A). In TSEA web-tool, we found an over-representation of the 274 DEGs in the lung, blood, and adipose tissue (Figure 5B). The Pattern Gene Database (PaGenBase), which combines gene patterns from literature and data mining, was employed to identify tissue/cell-specific genes. We observe the same enriched pattern of expression in blood, lung, spleen and the heart (Figure 5C). This may suggest that dysregulated genes detected in the injured lung might recapitulate expression changes observed in spleen, heart, or colon of COVID-19 patients with pre-existing comorbidities.
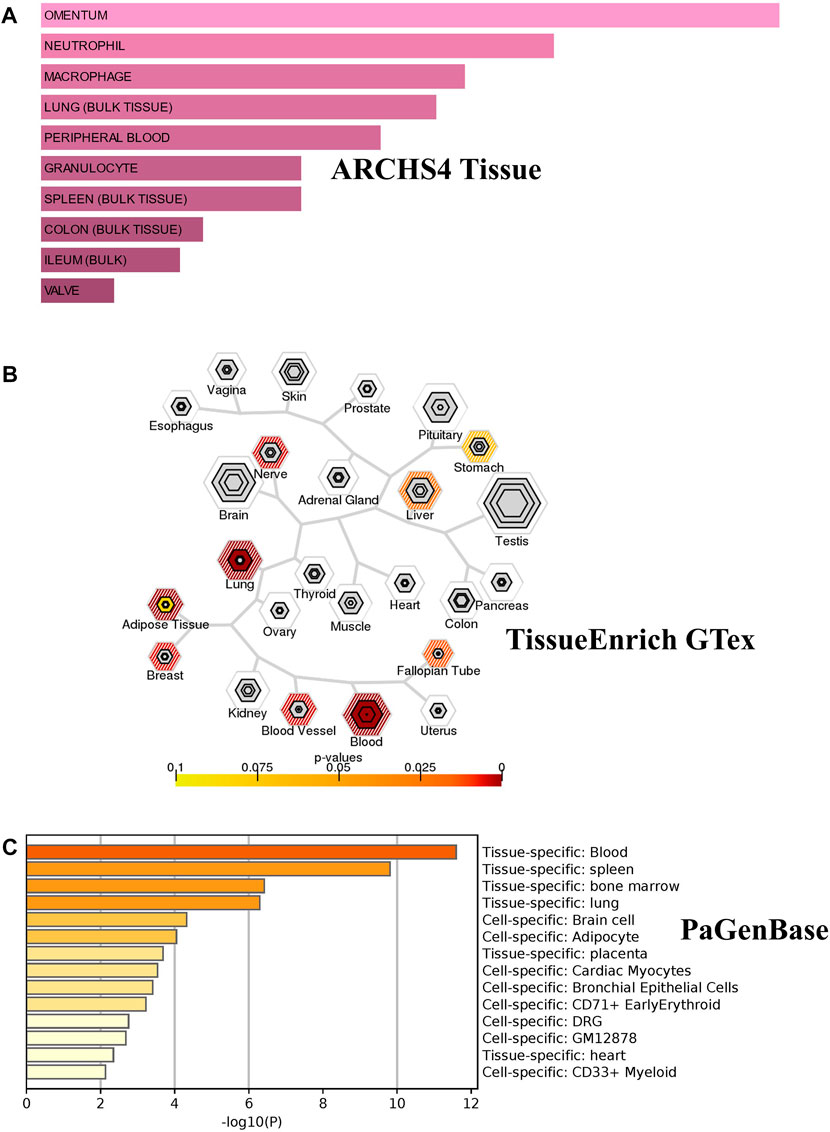
FIGURE 5. Tissue/cells-specific enrichment of Shared comorbidities-COVID-19 genes. (A) Enrichr Bar Graph data were collected from Enricher web (https://amp.pharm.mssm.edu/Enrichr). The length of the bar represents the significance of that specific gene-set or term. The brighter the color, the more significant that term is. (B) Tissue specific expression analysis (TSEA) network for common genes showing enrichment for genes expressed in related tissues. (C) Tissue/cell enrichment of the PaGenBase category of Metascape. The darker the color, the more significant that term is. Colored bars correspond to tissues with an adjusted p-value ≤ 0.01.
Functional enrichment analysis of unique and shared differentially expressed genes
We used Metascape to generate a graphical representation of the specific enrichment of biological processes and pathways shared among COVID-19 infected patients with comorbidities. First, we interrogated the biological processes separately using unique to comorbidities (Supplementary Figure S1A) and to COVID-19 DEGs (Supplementary Figure S1B) to capture the pathways for each category. Secondly, we looked exclusively for pathways enrichment in KEGG and Reactome for unique comorbidities and COVID-19 gene sets, respectively (Supplementary Figures S2A,B). Shared biological processes and pathways were identified as well (Figures 6A,C). Major non-redundant biological process networks were also generated using WebGestalt (http://www.webgestalt.org/) (Figure 6B).
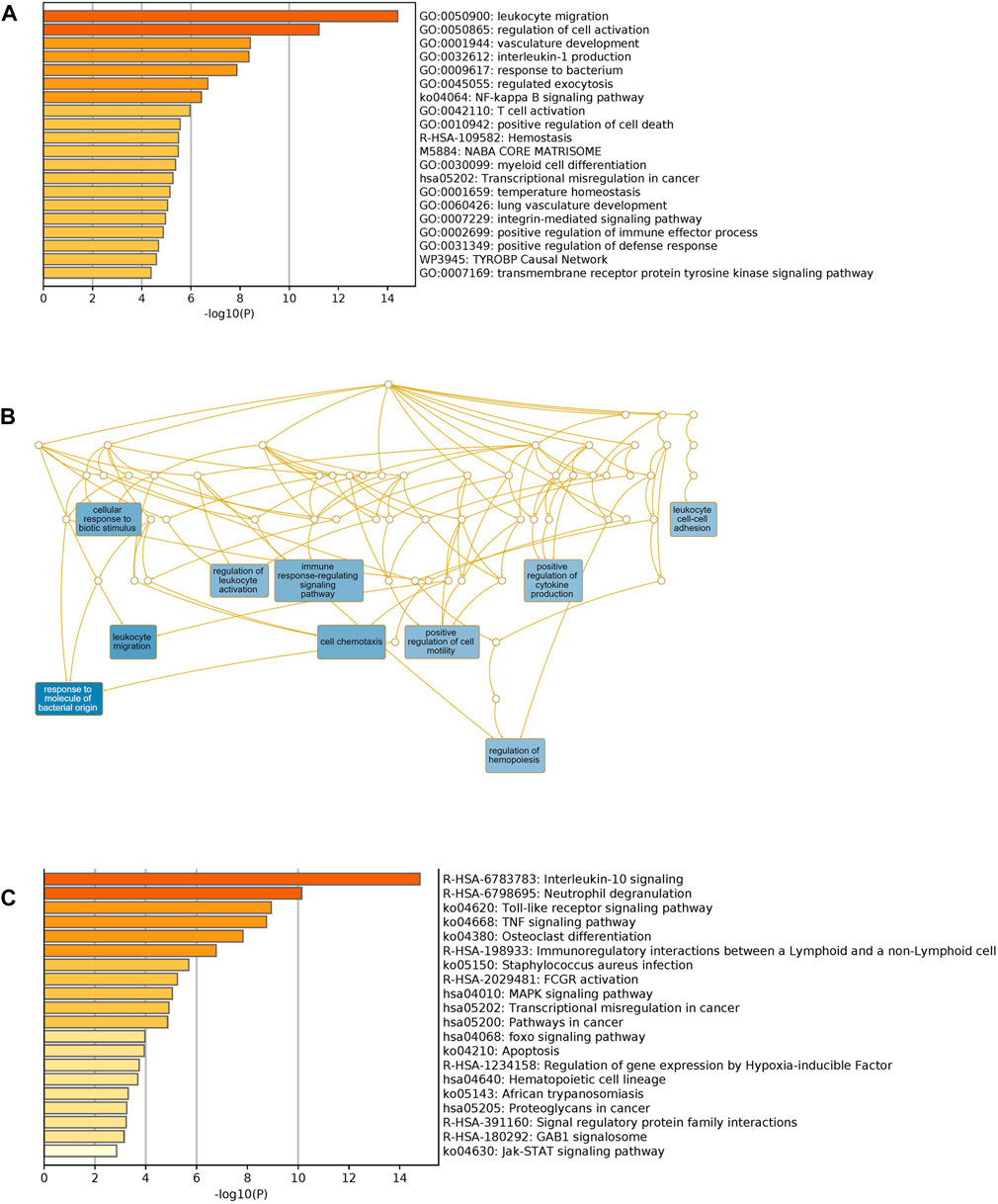
FIGURE 6. Functional enrichment of shared comorbidities-COVID-19 DEGs. (A) Bar graphs showing most significant biological processes. (B) Network illustrates the major non-redundant biological process. DEGs are enriched in biological processes related to leukocyte migration, T cell activation, regulation of hemopoiesis and integrin pathway. (C) Top Shared pathways; IL-10, TLRs and TNF signaling. The bar graphs were generated using Metascape (http://metascape.org) to illustrate the most enriched pathways and the Network was generated using WebGestalt (http://www.webgestalt.org/).
Unique genes for comorbidities alone were significantly enriched in the adaptive immune system, phagosome, neutrophil degranulation and signaling by interleukins. Unique genes for COVID-19 were also enriched in the adaptive immune system: signaling by Rho GTPases, heme biosynthesis and nervous system development. We observed, as expected, that shared pathways of identified genes were related to immune response, interleukin signaling and FCGR activation. Interestingly, among the top significantly enriched pathways, many are related to immunity processes such as response to bacteria, cytokines regulation and secretion of chemokines and regulation of leukocyte migration. Collectively these analyses support the role of the identified candidate genes in increasing the severity of clinical symptoms of COVID-19 infection among patients with pre-existing comorbidities.
Molecular drug targets identification and similarity analysis with ACE2 and TMPRSS2
In order to identify drug target, we searched the DisGeNet database to identify significant disease genes associated with comorbidities in Metascape. Myocardial ischemia, fatty liver disease, inflammation, vascular disease, and pneumonia were among the top disease classes represented in DisGeNet for all shared genes (Figure 7A). We identified 150 genes associated with top comorbidities (Figure 7B). This subset of genes was first submitted to the Open Targets Platform for druggability analysis and identification of potential novel drug targets, for existing drug repurposing against COVID-19 infection. Using the Open Targets Platform, 29 out of 150 genes were found to have known inhibitors/antagonists, modulators or agonists (Supplementary Table S2) and we prioritized these genes based on their association to top comorbidities (Figure 7C).
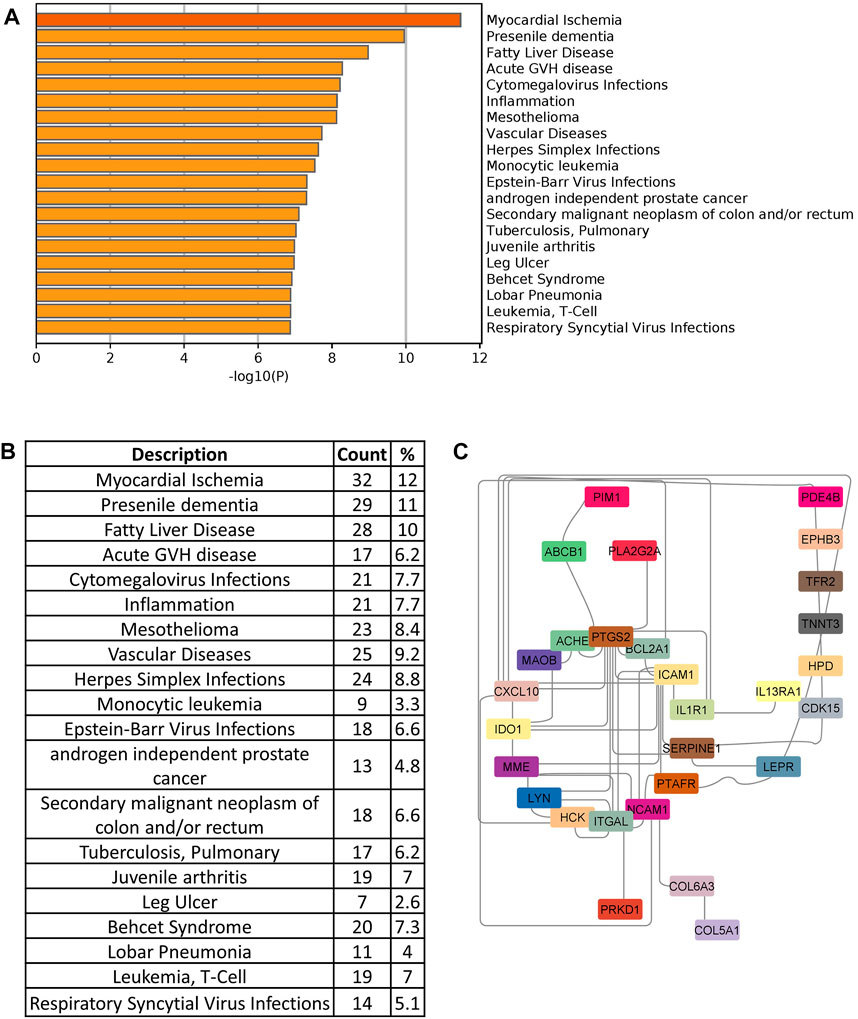
FIGURE 7. (A) Disease enrichment of shared genes of comorbidities and COVID-19 infection. Bar chart shows significant disease enrichment. (B) List of diseases related to comorbidities and COVID-19 with the percentage of specific common genes. (C) Network showing the specific drug targets.
To further refine our drug target list, we performed a functional similarity analysis of the identified 29 target genes against Angiotensin-converting enzyme 2 (ACE2) and Transmembrane protease serine 2 (TMPRSS2), which play an important role in COVID-19 infection using the XGR web-tool for enhanced interpretation (http://galahad.well.ox.ac.uk:3020/). We found that 13 genes have a high similarity score (≥0.5) with ACE2 and TMPRSS2 (Figure 8A). Remaining 16 genes with a similarity score (≤0.5) were also shown (Figure 8B). Our analysis showed that some genes have similarities to both ACE2 and TMPRSS2, but some are present only with ACE2, especially, IDO1, MME, LEPR, ABCB1, and PTARF. We found that only the PRKD1 gene is similar to TMPRSS2 and not to ACE2 (Figure 8A). These results suggest that genes with the highest similarity to ACE2 and TMPRSS2 could influence the COVID-19 virus infection directly, leading to the possibility of molecular interaction and functional changes in severe COVID-19 patients. Furthermore, it may result in overexpression of ACE2 and TMPRSS2, which explains the higher infection rate and disease severity in individuals with comorbidities. Drug target with high similarity scores to ACE2 and TMPRSS2 includes CXCL10, ICAM1, SERPINE1, PTGS2, ABCB1, IDO1, IL1R1, LEPR, MME, PTAFR, ITGAL, NCAM1 and PRKD1, (all up-regulated except LEPR) (Table 1) of which six targets overlap with PPI network top genes including ICAM1, PTGS2, CXCL10, ITGAL, NCAM1, SERPINE1 (Figure 4). Based on the association to top comorbidities and ACE2 and TMPRSS2 similarity scores, 17 drug target genes were identified, Marketed drugs for identified genes are indicated for the treatment of hypertension, heart failure, kidney failure, lung cancer, diabetes mellitus, emphysema, pulmonary obstruction, acute coronary syndrome, allergic rhinitis, inflammation, diabetic foot fever, diabetes mellitus, rheumatic disease and generalized lipodystrophy (Table 1). Identified drugs could be tested further for repurposing to reduce the severity risk among COVID-19-infected patients. Drug repurposing of marketed or approved drugs is an effective alternative method to uncover new indications rapidly.
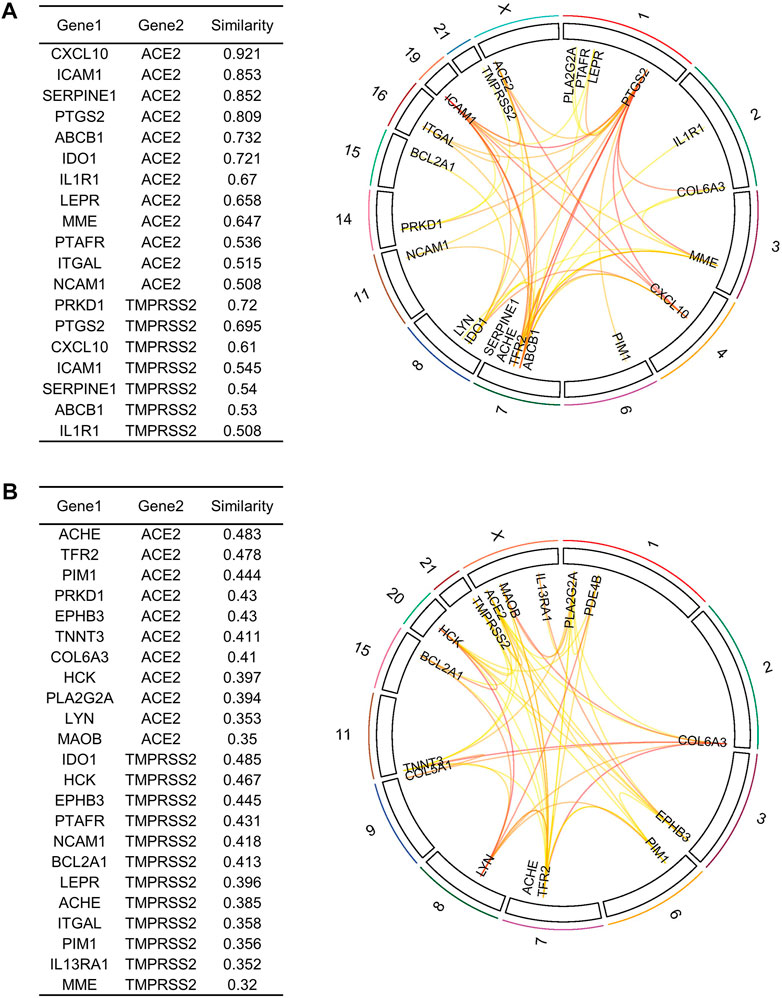
FIGURE 8. Similarity analysis and degree of relatedness of drug target genes to ACE2 and TMPRSS2 as a group to each other using eXploring Genomic Relations for enhanced interpretation. (A) ACE2 and TMPRSS2 showed a high degree of similarity with 13 out of the 29 identified genes. (B) Similarity analysis of the remaining 16 drug target genes. Drug targets were filtered based on cutoff score ≥ 0.5 as highly similar and ≤ 0.5 as slightly similar to ACE2 and TMPRSS2.
Signaling network analysis
The signaling causal interaction network (in SIGNOR 3.0) of the candidate genes showed that 15 out of 17 genes were central regulators of multiple signaling pathways (Supplementary Figure S3). Dysregulated pathways includes inflammation, immune response, chemotaxis, fibrinolysis, cell adhesion and acute respiratory distress syndrome (ARDS). Most genes like PTGS2, PTAFR, MOAB, PRKD1, ABCB1, IL1R1 and LEPR showed high interaction (with >8 connectivity). Of note, IDO1 and PLA2G2A were not present in the SIGNOR 3.0 database.
Phenotypic implications of the identified genes
Finally, we investigated the overall phenotypic association of shared genes. We performed our analysis using ToppGene (https://toppgene.cchmc.org/) for mouse phenotype analysis. We found a consistent phenotype pattern with the gene ontology and pathway enrichment, including abnormal innate and adaptive immunity, abnormal macrophage production, pulmonary interstitial fibrosis, and decreased inflammatory response (Supplementary Figure S4A). We also performed the phenotypic association of the 17 candidate genes from Open Target Platform analysis, to increase the specificity of observed phenotypes. In addition to the above-mentioned phenotypes, candidate genes were highly associated with abnormal type IV hypersensitivity reaction, abnormal body temperature, abnormal interleukin level and altered susceptibility to infection (Supplementary Figure S4B).
Discussion
The discovery of new biomarkers that can differentiate and categorize distinct COVID-19 phenotypes is urgently needed. Elucidating specific biomarkers to severe COVID-19 disease phenotype allows early intervention that may improve the outcome. Patients with pre-existing comorbidities, including cardiovascular disease, obesity, diabetes, and respiratory system diseases, are at a greater risk of developing severe symptoms or fatal with COVID-19 infection. Chronic diseases share risk factors or disease pathways with infectious disorders, like the pro-inflammatory state and the attenuation of the innate immune response (Yang et al., 2020a).
Differential gene expression analysis between patients and healthy control groups is the common logical method utilized to identify disease-associated changes. Here, we used transcriptomic data integration, differential expression analysis, and co-expression network analysis to identify underlying genetic factors and elucidate potential target drug or biomarkers that might explain the contribution of the comorbidities to increase the severity of COVID-19 infection symptoms by molecular interactions. Data for other respiratory illnesses such as influenza virus, RSV and MERS were intersected with COVID-19 infection DEGs to remove the common viral pathway genes, which otherwise will be tagged as shared biomarkers for comorbidities-associated severe COVID-19 symptoms.
Comorbidities-COVID-19 pathogenicity
We identified 274 significant DEGs (|log2FC| >1.5; p < 0.05) shared between comorbidities and COVID-19. Functional analysis of shared genes among both comorbidities and COVID-19 infection revealed a critical involvement and significant enrichment in immune response processes including leukocyte migration, interleukin production, T cell activation, lung vasculature development, cell death and temperature homeostasis (Figure 5). Shared pathways are mostly related to interleukin-10, TNF and FOXO signaling which are involved in cellular processes like apoptosis, cell cycle, control of cell cycle glucose metabolism and oxidative stress resistance (Figure 5C).
Based on PPI, biological process and molecular pathway enrichments, we found that both comorbidities and COVID-19 have a strong association with symptomatically related diseases such as early-onset dementia, fatty liver disease, mesothelioma, vascular diseases, cytomegalovirus, herpes simplex and Epstein–Barr Virus infections (Figure 6). COVID-19 infection causes a severe multisystem inflammatory disease in critically ill patients consisting of respiratory distress, cardiovascular dysfunction, thrombosis, neurological manifestations, dysregulated inflammatory process and fibrosis. The strong link of shared genes with several diseases can be attributed to the critical role of the identified genes.
ACE2 and TMPRSS2 correlation and target genes drug repurposing
ACE2 and TMPRSS2 are the two major host receptors which contribute to the virulence and the severe pathogenesis of COVID-19. Our computational analysis of the PPI network identified several highly interacting genes such as ICAM1, PTGS2, CXCL10, ITGAL, NCAM1, SERPINE1, IDO1, LEPR, PTAFR, and PLA2G2A (Figure 4), which also showed a higher degree of functional similarity with ACE2 and TMPRSS2 (Figure 7) suggesting a potential direct or indirect interaction with the COVID-19 spike protein (Cuperlovic-Culf et al., 2021).
Systemic inflammation is a hallmark of many infectious diseases including COVID-19. An appropriate inflammatory response is essential for eradicating pathogens. Prolonged or excessive inflammatory response is clearly detrimental and fatal to the host if not carefully managed. Identified biomarkers could be categorized as acute phase reacting immunomodulators as they significantly alter the inflammatory cells’ responses, which trigger the pro-inflammatory signaling pathway (Hata and Breyer, 2004; Vazirinejad et al., 2014; Guo et al., 2019; Kiernan and MacIver, 2020).
Identified biomarkers were found to be involved in innate immunity, mostly in monocyte and macrophage function and are essential for immune cell homeostasis. Their early activation/inactivation results in uncontrolled production, perturbation, and inadequate recruitment of active immune cells, which in turn promotes the cellular stress, resident cell’s damage and, eventually, organ failure. The interplay of these main biomarkers during infection induced early immune response are discussed elsewhere (Fang et al., 2020; Goyal et al., 2020; Grasselli et al., 2020; Holman et al., 2020; Madjid et al., 2020; Shi et al., 2020; Wu and McGoogan, 2020; Zhou et al., 2020).
Dysregulation of these COVID-19 acute phase pro-inflammatory genes can greatly induce a detrimental effect by prolonging the inflammatory response, delayed viral clearance and thus increasing the risk of severe and prolonged COVID-19 symptoms (Coperchini et al., 2021) For example, only augmented CXCL10 plasma levels in COVID-19 infected patients were found to be significantly correlated with the viral load and disease severity (Guo et al., 2022a; Guo et al., 2022b) and higher mortality, especially in males (Draxler et al., 2017). It is worth noting that CXCL10 is not expressed in healthy individuals and was significantly lower in asymptomatic cases than in symptomatic patients. Furthermore, COVID-19 patients have delayed or slow immune dysfunction response, making these patients more susceptible to suffer from exacerbation and impaired lung function (Danladi and Sabir, 2021). Increased expression of PDE4B results in a pro-inflammatory phenotype in neutrophils and macrophages (Ulm et al., 2013). Selective inhibitors in the knockout mouse model for severe chronic obstructive pulmonary disorder (COPD) have established the beneficial anti-inflammatory effect of PDE4B to improve lung function and decrease exacerbation rates. Specific inhibition of these factors significantly alters the functions of specialized inflammatory cells suggesting that these factors may help in early diagnosis and could be predictors of clinical symptoms (Guo et al., 2022a). IDO1 is also an important immunomodulatory gene in T lymphocyte activation and in modulating immune tolerance (Veazey et al., 2020; Makaremi et al., 2022). In preclinical models, pharmacological inhibitors of IDO1 have therapeutic utility in various diseases, including cancer, HIV and influenza (Yang et al., 2020b; Chang et al., 2020; Chi et al., 2020). Interestingly, IDO1 inhibitors suppress the COVID-19-induced pro-inflammatory cytokine release, including TNF-α, IL-6, IL-1α and IL-1β in isolated PBMCs derived from COVID-19-infected rhesus macaques and lower mortality among critically ill patients with COVID-19 (Song et al., 2020; Agrawal et al., 2021). This confirms the critical role of the IDO1 gene network in COVID-19-exaggerated cytokine release. Moreover, MAOB, ICAM1, SERPINE1, MME, IL1R1, PLA2G2A, LEPR and PTGS2 have emerged as attractive pharmaceutical targets for COVID-19 infection treatment recently (Puccetti and Grohmann, 2007; Iyer et al., 2018; Blair et al., 2019; Tibbo and Baillie, 2020).
Our analysis also showed that LEPR is downregulated in COVID-19. The expression of LEPR is inversely related to leptin concentrations (Potula et al., 2005). LEPR has been shown to influence both innate and adaptive immune responses (Singh and Salunke, 2021). Several studies on mouse models of viral infection addressed the role of LEPR and leptin in the pathogenesis of infectious diseases (Potula et al., 2005). Interestingly, global reduction of the LEPRs in obese mice demonstrated impaired influenza virus viral clearance and reduced survival (Group et al., 2021). Additionally, LEPR deficient mouse exhibits increased susceptibility to respiratory infections suggesting a leptin requirement in the pulmonary innate and adaptive immune response to infection (Tomazini et al., 2020).
Arterial thrombosis in the lung and other organs has been reported in critically ill COVID-19 patients (Bellis et al., 2020). Platelet Activating Factor Receptor (PTAFR or PAFR) is exploited by respiratory viruses and bacteria to interact with human cells and initiate infection (Cauchois et al., 2020). It was implicated in the entry of H1N1 and H3N2, Dengue and respiratory syncytial viruses (Mohammed El Tabaa and Mohammed El Tabaa, 2020). PTAFR deficient mice, as well as wild-type mice treated with a PTAFR antagonist, had less pulmonary inflammation and injury and reduced lethality rates when infected with Influenza A virus (Mohammed El Tabaa and Mohammed El Tabaa, 2020). These findings show that PTAFR is a major driver of exacerbated inflammation during viral infection. Inhibition of PTAFR offers significant protection against fatal outcomes and lung injury associated with the flu. PLA2G2A is a potent mediator of the inflammatory process and also proatherogenic, implicated in various clinical conditions, including sepsis (Ostadkarampour and Putnins, 2021). Increased PLA2G2A levels is a significant risk factor for coronary artery disease and are found to be highly associated with disease severity in COVID-19 patients (Lahlou et al., 2002). It will be interesting to explore the potential of these genes as early systemic diagnostic biomarkers for COVID-19.
Although our study aims to map key genes and pathway alterations in patients with COVID-19 and comorbidities, our results at present are observational and there are limitations to our study. First, there was a lack of data from COVID-19 patients with comorbidities and present COVID-19 datasets were mostly published on 2020 before the emergence of Omicron variant. Second, there was a lack of data on COVID-19 variants effect in the present study, which may become a focus of future studies as more datasets are put in the public domain. That will allow the scientists to explore the effect of different COVID-19 variants into the specific molecular mechanism and to the clinical severity of COVID-19. Despite these disadvantages, the present study was powered by integrated bioinformatics analysis identified DEGs that exhibited large-fold changes with high statistical significance. Moreover, there was consistency between our findings and the previous studies. Hence, the results of our analysis could be reliable to a large extent and explain the specific role of these genes in COVID-19 and indirectly give insights into how such genes contribute to the severity of COVID-19 infection. Lastly, there was a lack of experimental validation for these findings in the present study, which may become a focus of future studies.
Conclusion
In summary, we used publicly available gene expression profiles from severe/deceased COVID-19 infected individuals and patients with comorbidities alone — CVD, atherosclerosis, diabetes, and obesity, to identify overlapping key genes associated with the severity of COVID-19 infection. We identified 29 genes shared between severe COVID-19 and comorbidities. Our results show that some of the available drugs against the identified genes are already in use as COVID-19 therapy, suggesting that identified genes as clinical indicators for COVID-19 disease severity, central drivers of immune deficit and multiorgan failure. We recommend that existing drugs for identified genes can be investigated further for their therapeutic efficacy and repurposed to treat COVID-19 patients with severe symptoms.
Data availability statement
The datasets presented in this study can be found in online repositories. The names of the repository/repositories and accession number(s) can be found in the article/Supplementary Material.
Author contributions
Conceptualization: AM and RE; Data curation: AM, RE, BB, and NS; Formal analysis: AM and KA; Funding acquisition: NS; Methodology: AM, BB, and RE; Software: AM and BB; Supervision: AM and RE; Validation: AM and KN; Visualization: AM; Writing original draft and editing: AM, NA-R, BB, NS, and RE. All authors contributed to the article and approved the submitted version.
Funding
Deputyship for Research and Innovation, Ministry of Education in Saudi Arabia for funding this research work through the project number “IFPHI-132-140-2020.”
Acknowledgments
The authors extend their appreciation to the Deputyship for Research and Innovation, Ministry and King Abdulaziz University, DSR, Jeddah, Saudi Arabia for their technical support.
Conflict of interest
The authors declare that the research was conducted in the absence of any commercial or financial relationships that could be construed as a potential conflict of interest.
Publisher’s note
All claims expressed in this article are solely those of the authors and do not necessarily represent those of their affiliated organizations, or those of the publisher, the editors and the reviewers. Any product that may be evaluated in this article, or claim that may be made by its manufacturer, is not guaranteed or endorsed by the publisher.
Supplementary material
The Supplementary Material for this article can be found online at: https://www.frontiersin.org/articles/10.3389/fphys.2022.1045469/full#supplementary-material
References
Agrawal S., Salazar J., Tran T. M., Agrawal A. (2021). Sex-related differences in innate and adaptive immune responses to SARS-CoV-2. Front. Immunol. 12, 739757. doi:10.3389/fimmu.2021.739757
Alti D., Sambamurthy C., Kalangi S. K. (2018). Emergence of leptin in infection and immunity: Scope and challenges in vaccines formulation. Front. Cell. Infect. Microbiol. 8, 147. doi:10.3389/fcimb.2018.00147
Banaganapalli B., Al-Rayes N., Awan Z. A., Alsulaimany F. A., Alamri A. S., Elango R., et al. (2021). Multilevel systems biology analysis of lung transcriptomics data identifies key miRNAs and potential miRNA target genes for SARS-CoV-2 infection. Comput. Biol. Med. 135, 104570. doi:10.1016/j.compbiomed.2021.104570
Banaganapalli B., Mansour H., Mohammed A., Alharthi A. M., Aljuaid N. M., Nasser K. K., et al. (2020). Exploring celiac disease candidate pathways by global gene expression profiling and gene network cluster analysis. Sci. Rep. 10, 16290. doi:10.1038/s41598-020-73288-6
Bellis A., Mauro C., Barbato E., Trimarco B., Morisco C. (2020). The rationale for Angiotensin receptor neprilysin inhibitors in a multi-targeted therapeutic approach to COVID-19. Int. J. Mol. Sci. 21, E8612. doi:10.3390/ijms21228612
Blair A. B., Kleponis J., Thomas D. L., Muth S. T., Murphy A. G., Kim V., et al. (2019). Ido1 inhibition potentiates vaccine-induced immunity against pancreatic adenocarcinoma. J. Clin. Invest. 129, 1742–1755. doi:10.1172/JCI124077
Cauchois R., Koubi M., Delarbre D., Manet C., Carvelli J., Blasco V. B., et al. (2020). Early IL-1 receptor blockade in severe inflammatory respiratory failure complicating COVID-19. Proc. Natl. Acad. Sci. U. S. A. 117, 18951–18953. doi:10.1073/pnas.2009017117
Chang Mo G., Yuan X., Tao Y., Peng X., Wang F. S., Xie L., et al. (2020). Time kinetics of viral clearance and resolution of symptoms in novel coronavirus infection. Am. J. Respir. Crit. Care Med. 201, 1150–1152. doi:10.1164/rccm.202003-0524LE
Chi Y., Ge Y., Wu B., Zhang W., Wu T., Wen T., et al. (2020). Serum cytokine and chemokine profile in relation to the severity of coronavirus disease 2019 in China. J. Infect. Dis. 222, 746–754. doi:10.1093/infdis/jiaa363
Coperchini F., Chiovato L., Rotondi M. (2021). Interleukin-6, CXCL10 and infiltrating macrophages in COVID-19-related cytokine storm: Not one for all but all for one. Front. Immunol. 12, 668507. doi:10.3389/fimmu.2021.668507
Cuperlovic-Culf M., Cunningham E. L., Teimoorinia H., Surendra A., Pan X., Bennett S. A. L., et al. (2021). Cerebrospinal fluid spermidine, glutamine and putrescine predict postoperative delirium following elective orthopaedic surgery. Sci. Rep. 11, 4191. doi:10.1038/s41598-019-40544-3
Danladi J., Sabir H. (2021). Innate immunity, inflammation activation and heat-shock protein in COVID-19 pathogenesis. J. Neuroimmunol. 358, 577632. doi:10.1016/j.jneuroim.2021.577632
Dolan M. E., Hill D. P., Mukherjee G., McAndrews M. S., Chesler E. J., Blake J. A. (2020). Investigation of COVID-19 comorbidities reveals genes and pathways coincident with the SARS-CoV-2 viral disease. Sci. Rep. 10, 20848. doi:10.1038/s41598-020-77632-8
Dore E., Boilard E. (2019). Roles of secreted phospholipase A2 group IIA in inflammation and host defense. Biochim. Biophys. Acta. Mol. Cell Biol. Lipids 1864, 789–802. doi:10.1016/j.bbalip.2018.08.017
Draxler D. F., Sashindranath M., Medcalf R. L. (2017). Plasmin: A modulator of immune function. Semin. Thromb. Hemost. 43, 143–153. doi:10.1055/s-0036-1586227
Facchiano A., Facchiano F., Facchiano A. (2020). An investigation into the molecular basis of cancer comorbidities in coronavirus infection. FEBS Open Bio 10, 2363–2374. doi:10.1002/2211-5463.12984
Fang L., Karakiulakis G., Roth M. (2020). Are patients with hypertension and diabetes mellitus at increased risk for COVID-19 infection? Lancet. Respir. Med. 8, e21. doi:10.1016/S2213-2600(20)30116-8
Fox T., Ruddiman K., Lo K. B., Peterson E., DeJoy R., Salacup G., et al. (2021). The relationship between diabetes and clinical outcomes in COVID-19: A single-center retrospective analysis. Acta Diabetol. 58, 33–38. doi:10.1007/s00592-020-01592-8
Garcia C. C., Russo R. C., Guabiraba R., Fagundes C. T., Polidoro R. B., Tavares L. P., et al. (2010). Platelet-activating factor receptor plays a role in lung injury and death caused by Influenza A in mice. PLoS Pathog. 6, e1001171. doi:10.1371/journal.ppat.1001171
Goyal P., Choi J. J., Pinheiro L. C., Schenck E. J., Chen R., Jabri A., et al. (2020). Clinical characteristics of covid-19 in New York city. N. Engl. J. Med. 382, 2372–2374. doi:10.1056/NEJMc2010419
Grasselli G., Zangrillo A., Zanella A., Antonelli M., Cabrini L., Castelli A., et al. (2020). Baseline characteristics and outcomes of 1591 patients infected with SARS-CoV-2 admitted to ICUs of the lombardy region, Italy. JAMA 323, 1574–1581. doi:10.1001/jama.2020.5394
Group R. C., Horby P., Lim W. S., Emberson J. R., Mafham M., Bell J. L., et al. (2021). Dexamethasone in hospitalized patients with covid-19. N. Engl. J. Med. Overseas. Ed. 384, 693–704. doi:10.1056/nejmoa2021436
Guo G., Sun L., Yang L., Xu H. (2019). Ido1 depletion induces an anti-inflammatory response in macrophages in mice with chronic viral myocarditis. Cell Cycle 18, 2598–2613. doi:10.1080/15384101.2019.1652471
Guo L., Schurink B., Roos E., Nossent E. J., Duitman J. W., Vlaar A. P., et al. (2022). Indoleamine 2, 3-dioxygenase (Ido)-1 and Ido-2 activity and severe course of COVID-19. J. Pathol. 256, 256–261. doi:10.1002/path.5842
Guo Z., Yang H., Zhang J-R., Zeng W., Hu X. (2022). Leptin receptor signaling sustains metabolic fitness of alveolar macrophages to attenuate pulmonary inflammation. Sci. Adv. 8, eabo3064. doi:10.1126/sciadv.abo3064
Hata A. N., Breyer R. M. (2004). Pharmacology and signaling of prostaglandin receptors: Multiple roles in inflammation and immune modulation. Pharmacol. Ther. 103, 147–166. doi:10.1016/j.pharmthera.2004.06.003
Hernandez-Garduno E. (2020). Obesity is the comorbidity more strongly associated for Covid-19 in Mexico. A case-control study. Obes. Res. Clin. Pract. 14, 375–379. doi:10.1016/j.orcp.2020.06.001
Holman N., Knighton P., Kar P., O'Keefe J., Curley M., Weaver A., et al. (2020). Risk factors for COVID-19-related mortality in people with type 1 and type 2 diabetes in england: A population-based cohort study. Lancet. Diabetes Endocrinol. 8, 823–833. doi:10.1016/S2213-8587(20)30271-0
Hottz E. D., Azevedo-Quintanilha I. G., Palhinha L., Teixeira L., Barreto E. A., Pao C. R. R., et al. (2020). Platelet activation and platelet-monocyte aggregate formation trigger tissue factor expression in patients with severe COVID-19. Blood 136, 1330–1341. doi:10.1182/blood.2020007252
Iyer S. S., Gensollen T., Gandhi A., Oh S. F., Neves J. F., Collin F., et al. (2018). Dietary and microbial oxazoles induce intestinal inflammation by modulating aryl hydrocarbon receptor responses. Cell 173, 1123–1134. doi:10.1016/j.cell.2018.04.037
Kiernan K., MacIver N. J. (2020). The role of the adipokine leptin in immune cell function in health and disease. Front. Immunol. 11, 622468. doi:10.3389/fimmu.2020.622468
Lahlou N., Issad T., Lebouc Y., Carel J. C., Camoin L., Roger M., et al. (2002). Mutations in the human leptin and leptin receptor genes as models of serum leptin receptor regulation. Diabetes 51, 1980–1985. doi:10.2337/diabetes.51.6.1980
Madjid M., Safavi-Naeini P., Solomon S. D., Vardeny O. (2020). Potential effects of coronaviruses on the cardiovascular system: A review. JAMA Cardiol. 5, 831–840. doi:10.1001/jamacardio.2020.1286
Makaremi S., Asgarzadeh A., Kianfar H., Mohammadnia A., Asghariazar V., Safarzadeh E. (2022). The role of IL-1 family of cytokines and receptors in pathogenesis of COVID-19. Inflamm. Res. 71, 923–947. doi:10.1007/s00011-022-01596-w
Malik P., Patel U., Patel K., Martin M., Shah C., Mehta D., et al. (2021). Obesity a predictor of outcomes of COVID-19 hospitalized patients-A systematic review and meta-analysis. J. Med. Virol. 93, 1188–1193. doi:10.1002/jmv.26555
Martin-Romero C., Santos-Alvarez J., Goberna R., Sanchez-Margalet V. (2000). Human leptin enhances activation and proliferation of human circulating T lymphocytes. Cell. Immunol. 199, 15–24. doi:10.1006/cimm.1999.1594
Mohammed El Tabaa M., Mohammed El Tabaa M. (2020). Targeting neprilysin (nep) pathways: A potential new hope to defeat COVID-19 ghost. Biochem. Pharmacol. 178, 114057. doi:10.1016/j.bcp.2020.114057
Mujalli A., Banaganapalli B., Alrayes N. M., Shaik N. A., Elango R., Al-Aama J. Y. (2020). Myocardial infarction biomarker discovery with integrated gene expression, pathways and biological networks analysis. Genomics 112, 5072–5085. doi:10.1016/j.ygeno.2020.09.004
Ostadkarampour M., Putnins E. E. (2021). Monoamine oxidase inhibitors: A review of their anti-inflammatory therapeutic potential and mechanisms of action. Front. Pharmacol. 12, 676239. doi:10.3389/fphar.2021.676239
Potula R., Poluektova L., Knipe B., Chrastil J., Heilman D., Dou H., et al. (2005). Inhibition of indoleamine 2, 3-dioxygenase (Ido) enhances elimination of virus-infected macrophages in an animal model of HIV-1 encephalitis. Blood 106, 2382–2390. doi:10.1182/blood-2005-04-1403
Puccetti P., Grohmann U. (2007). Ido and regulatory T cells: A role for reverse signalling and non-canonical NF-kappaB activation. Nat. Rev. Immunol. 7, 817–823. doi:10.1038/nri2163
Radigan K. A., Morales-Nebreda L., Soberanes S., Nicholson T., Nigdelioglu R., Cho T., et al. (2014). Impaired clearance of influenza A virus in obese, leptin receptor deficient mice is independent of leptin signaling in the lung epithelium and macrophages. PLoS One 9, e108138. doi:10.1371/journal.pone.0108138
Rijneveld A. W., Weijer S., Florquin S., Speelman P., Shimizu T., Ishii S., et al. (2004). Improved host defense against pneumococcal pneumonia in platelet-activating factor receptor-deficient mice. J. Infect. Dis. 189, 711–716. doi:10.1086/381392
Shi S., Qin M., Shen B., Cai Y., Liu T., Yang F., et al. (2020). Association of cardiac injury with mortality in hospitalized patients with COVID-19 in wuhan, China. JAMA Cardiol. 5, 802–810. doi:10.1001/jamacardio.2020.0950
Singh M. K., Mobeen A., Chandra A., Joshi S., Ramachandran S. (2021). A meta-analysis of comorbidities in COVID-19: Which diseases increase the susceptibility of SARS-CoV-2 infection? Comput. Biol. Med. 130, 104219. doi:10.1016/j.compbiomed.2021.104219
Singh R., Salunke D. B. (2021). Diverse chemical space of indoleamine-2, 3-dioxygenase 1 (Ido1) inhibitors. Eur. J. Med. Chem. 211, 113071. doi:10.1016/j.ejmech.2020.113071
Snider J. M., You J. K., Wang X., Snider A. J., Hallmark B., Seeds M. C., et al. (2021). Group iia secreted phospholipase A 2 plays a central role in the pathobiology of COVID-19. medRxiv. doi:10.1101/2021.02.22.21252237
Song C. Y., Xu J., He J. Q., Lu Y. Q. (2020). Immune dysfunction following COVID-19, especially in severe patients. Sci. Rep. 10, 15838. doi:10.1038/s41598-020-72718-9
Tibbo A. J., Baillie G. S. (2020). Phosphodiesterase 4B: Master regulator of brain signaling. Cells 9, E1254. doi:10.3390/cells9051254
Tomazini B. M., Maia I. S., Cavalcanti A. B., Berwanger O., Rosa R. G., Veiga V. C., et al. (2020). Effect of dexamethasone on days alive and ventilator-free in patients with moderate or severe acute respiratory distress syndrome and COVID-19: The CoDEX randomized clinical trial. JAMA 324, 1307–1316. doi:10.1001/jama.2020.17021
Ulm C., Saffarzadeh M., Mahavadi P., Müller S., Prem G., Saboor F., et al. (2013). Soluble polysialylated NCAM: A novel player of the innate immune system in the lung. Cell. Mol. Life Sci. 70, 3695–3708. doi:10.1007/s00018-013-1342-0
Vazirinejad R., Ahmadi Z., Kazemi Arababadi M., Hassanshahi G., Kennedy D. (2014). The biological functions, structure and sources of CXCL10 and its outstanding part in the pathophysiology of multiple sclerosis. Neuroimmunomodulation 21, 322–330. doi:10.1159/000357780
Veazey J. M., Eliseeva S. I., Hillman S. E., Stiles K., Smyth T. R., Morrissey C. E., et al. (2020). Inhibiting protein kinase D promotes airway epithelial barrier integrity in mouse models of influenza A virus infection. Front. Immunol. 11, 580401. doi:10.3389/fimmu.2020.580401
Wu Z., McGoogan J. M. (2020). Characteristics of and important lessons from the coronavirus disease 2019 (COVID-19) outbreak in China: Summary of a report of 72314 cases from the Chinese center for disease control and prevention. JAMA 323, 1239–1242. doi:10.1001/jama.2020.2648
Yang J., Zheng Y., Gou X., Pu K., Chen Z., Guo Q., et al. (2020). Prevalence of comorbidities and its effects in patients infected with SARS-CoV-2: A systematic review and meta-analysis. Int. J. Infect. Dis. 94, 91–95. doi:10.1016/j.ijid.2020.03.017
Yang Y., Shen C., Li J., Yuan J., Wei J., Huang F., et al. (2020). Plasma IP-10 and MCP-3 levels are highly associated with disease severity and predict the progression of COVID-19. J. Allergy Clin. Immunol. 146, 119–127. doi:10.1016/j.jaci.2020.04.027
Keywords: COVID-19, SARS-CoV-2, viral infections, comorbidities, cardiovascular diseases, diabetes, obesity, MERS
Citation: Mujalli A, Alghamdi KS, Nasser KK, Al-Rayes N, Banaganapalli B, Shaik NA and Elango R (2022) Bioinformatics insights into the genes and pathways on severe COVID-19 pathology in patients with comorbidities. Front. Physiol. 13:1045469. doi: 10.3389/fphys.2022.1045469
Received: 15 September 2022; Accepted: 15 November 2022;
Published: 14 December 2022.
Edited by:
Georges E. Haddad, Howard University, United StatesReviewed by:
Tlili Barhoumi, King Abdullah International Medical Research Center (KAIMRC), Saudi ArabiaRan You, Nanjing Children’s Hospital, China
Tariq Masoodi, Sidra Medicine, Qatar
Copyright © 2022 Mujalli, Alghamdi, Nasser, Al-Rayes, Banaganapalli, Shaik and Elango. This is an open-access article distributed under the terms of the Creative Commons Attribution License (CC BY). The use, distribution or reproduction in other forums is permitted, provided the original author(s) and the copyright owner(s) are credited and that the original publication in this journal is cited, in accordance with accepted academic practice. No use, distribution or reproduction is permitted which does not comply with these terms.
*Correspondence: Abdulrahman Mujalli, ammujalli@uqu.edu.sa; Ramu Elango, relango@kau.edu.sa