- 1Department of Biological Chemistry, University of California, Irvine, Irvine, CA, United States
- 2TSE Systems Inc., Chesterfield, MO, United States
Metabolic rewiring is a hallmark feature prevalent in cancer cells as well as insulin resistance (IR) associated with diet-induced obesity (DIO). For instance, tumor metabolism shifts towards an enhanced glycolytic state even under aerobic conditions. In contrast, DIO triggers lipid-induced IR by impairing insulin signaling and reducing insulin-stimulated glucose uptake. Based on physiological differences in systemic metabolism, we used a breath analysis approach to discriminate between different pathological states using glucose oxidation as a readout. We assessed glucose utilization in lung cancer-induced cachexia and DIO mouse models using a U-13C glucose tracer and stable isotope sensors integrated into an indirect calorimetry system. Our data showed increased 13CO2 expired by tumor-bearing (TB) mice and a reduction in exhaled 13CO2 in the DIO model. Taken together, our findings illustrate high glucose uptake and consumption in TB animals and decreased glucose uptake and oxidation in obese mice with an IR phenotype. Our work has important translational implications for the utility of stable isotopes in breath-based detection of glucose homeostasis in models of lung cancer progression and DIO.
Introduction
The circadian clock system coordinates organismal behavior and physiology within a 24-hour time period. This biological pacemaker governs rhythms in sleep/wake activity, feeding/fasting cycles, endocrine regulation, and metabolism. The circadian clock is entrained by environmental cues such as light, feeding, exercise, and temperature (Takahashi, 2017; Guan and Lazar, 2021). Deregulation of the circadian clock has been linked with several pathologies, including endocrine disruption, IR, and cancer (Shi et al., 2013; Masri and Sassone-Corsi, 2018; Stenvers et al., 2019; Verlande and Masri, 2019). Meal timing and dietary composition have been shown to rewire the circadian clock and systemic metabolism (Kohsaka et al., 2007; Hatori et al., 2012; Eckel-Mahan et al., 2013). Additionally, genetic clock disruption in mice impacts insulin secretion (Perelis et al., 2015), hepatic glucose production and export (Rudic et al., 2004; Lamia et al., 2008), postprandial glucose disposal in skeletal muscle (Dyar et al., 2014), and results in obesity and type 2 diabetes (Turek et al., 2005; Marcheva et al., 2010; Paschos et al., 2012; Perelis et al., 2015). In the context of cancer, clock-controlled uptake, transport, and metabolism of glucose is disrupted (Altman et al., 2015; Papagiannakopoulos et al., 2016). Importantly, tumors also distally rewire metabolism to alter systemic insulin and glucose signaling (Masri et al., 2016; Hojo et al., 2017; Verlande et al., 2021).
Metabolic rewiring is one of the hallmarks of cancer that promotes tumor proliferation in low nutrient and oxygen conditions (Hanahan, 2022). Metabolic reprogramming includes the Warburg effect or aerobic glycolysis, and the recycling of lactate, amino acids and ammonia to support cancer cell growth and progression (Vander Heiden et al., 2009; Hensley et al., 2016; Spinelli et al., 2017). Interestingly, lactate has been found to be the primary fuel source of the mitochondrial tricarboxylic acid (TCA) cycle in human lung tumors (Hensley et al., 2016; Faubert et al., 2017; Hui et al., 2017) and in many other tissues (Hui et al., 2017). The Warburg effect has been demonstrated in a vast majority of tumors, and clinical applications have been leveraged for imaging, such as 2-deoxy-2-[fluorine-18]fluoro-D-glucose Positron Emission Tomography (18F-FDG-PET) to detect glucose uptake in tumors (Vaquero and Kinahan, 2015). Also, increased glucose oxidation through glycolysis and the TCA cycle have been demonstrated in tumor tissues compared to surrounding non-cancerous tissues using 13C-glucose carbon tracing (Fan et al., 2009; Hensley et al., 2016). Taken together, studies from humans and mice show that increased glucose and lactate oxidation in the mitochondria is characteristic of altered metabolism of lung tumors and other tumor types.
Glucose homeostasis is also disrupted in metabolic syndrome, obesity and type 2 diabetes (Rosen and Spiegelman, 2006; Yang et al., 2018). Consumption of high fat and high sugar diets is one of the major causes of DIO (Malik et al., 2013), and is attributed to IR that is characterized by elevated blood glucose and triglyceride levels (Saltiel and Kahn, 2001; Eckel et al., 2005). Insulin facilitates glucose uptake and inhibits hepatic gluconeogenesis and glycogenolysis. In addition, insulin activates de novo lipogenesis and reduces lipolysis in adipocytes, thereby lowering circulating lipid content (Saltiel and Kahn, 2001; Czech, 2017). IR attenuates these processes and results in hyperglycemia and hyperlipidemia that is associated with heightened inflammation (Arkan et al., 2005), endoplasmic reticulum stress (Ozcan et al., 2004), and ectopic lipid deposition in tissues (Krssak et al., 1999; Fabbrini et al., 2009). Also, IR impacts mitochondrial dynamics and plasticity and the ability to switch from fatty acid to glucose oxidation in skeletal muscle (Ukropcova et al., 2007; Koves et al., 2008). Collectively, DIO-induced IR disrupts systemic glucose homeostasis.
In this study, we illustrate that metabolic alterations of glucose utilization can be leveraged for real-time detection of lung tumor progression and DIO. We utilize a 13C-glucose tracer, that upon injection, can be detected in real-time in exhaled breath to quantify glucose uptake and consumption using stable isotope sensors in combination with indirect calorimetry (Figure 1). We confirmed the increased glucose uptake and consumption in a lung cancer cachexia model using an exogenous breath-based approach with a 13C-glucose tracer and stable isotope sensors. Using this same technology, we also illustrate that glucose uptake and oxidation is reduced in a model of DIO, which accurately reflects an IR phenotype. Overall, the utility of stable isotopes is becoming prevalent for metabolic fate mapping (Buescher et al., 2015; Jang et al., 2018; Lopes et al., 2021; Diehl et al., 2022; Pachnis et al., 2022) and clinical diagnostics (Modak, 2007; Braden, 2009), and we demonstrate that use of 13C-glucose faithfully recapitulates metabolic deregulation in models of lung tumor progression and DIO.
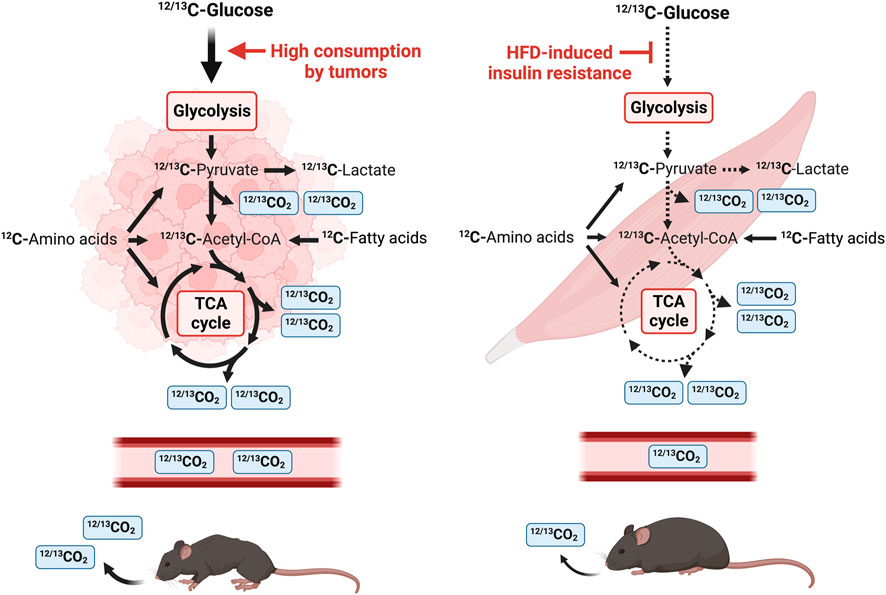
FIGURE 1. Schematic diagram of a stable isotope breath test study in mice using U-13C glucose. U-13C glucose tracer is introduced by intraperitoneal injection and the amount of 13CO2 and total CO2 is measured by a nondispersive infrared spectrometer. Tumor tissue consumes a mix of 12C and 13C glucose that is metabolized through glycolysis. 13C-pyruvate, the end-product of glycolysis, is either converted to 13C-lactate or enters the TCA cycle in the mitochondria. One CO2 is released from pyruvate oxidation to acetyl-CoA and two CO2 molecules are released per round of the TCA cycle. Tumors heavily consume glucose and tumor-bearing mice release a larger amount of 12CO2 and 13CO2 in the air. In contrast, HFD-induced IR leads to a reduction in systemic glucose uptake and utilization, and this is reflected in a reduction of expired 12CO2 and 13CO2.
Results
Rationale for the 13C breath assay
The breakdown of carbohydrates, lipids, and proteins into monosaccharides, fatty acids and amino acids generates ATP and provides cellular energy. These monomers are processed to acetyl-CoA and other metabolites of the TCA cycle (Figure 1). Monosaccharides such as glucose are further processed during glycolysis to produce two molecules of pyruvate, ATP and CO2 per molecule of glucose (Figure 1). Pyruvate is subsequently metabolized to lactate or shuttled into the mitochondrial matrix where it is converted to acetyl-CoA or oxaloacetate in the TCA cycle. Decarboxylation occurs twice in the TCA cycle, and four molecules of CO2 are produced per molecule of glucose (Figure 1). Therefore, six molecules of CO2 can be produced per molecule of glucose which is transported by red blood cells to be exhaled by the lungs. Use of a stable isotope tracer such as 13C-glucose allows for accurate quantification of 13CO2, relative to total CO2, which is detected by stable isotope sensors (Figure 1) (Fernández-Calleja et al., 2019; Seichter et al., 2021). Tumors are well-known to be avid metabolizers of glucose, and many metabolites are found at higher levels in lung cancer tissues versus surrounding non-cancerous tissues (Fan et al., 2009), indicating activated glycolysis and enhanced TCA cycle in tumors. As CO2 is released from the TCA cycle, these data suggest that TB mice release more CO2 derived from glucose than healthy animals. In contrast, DIO induces IR and results in a reduction of glucose uptake in skeletal muscle and a decrease of released CO2 (Figure 1).
Metabolic alterations in a mouse model of lung cancer-associated cachexia
The KrasLSL-G12D/+;p53fl/fl genetically engineered mouse model (GEMM) of lung cancer mirrors human non–small cell lung cancer and exhibits cancer-associated cachexia (CAC) (Jackson et al., 2001; Jackson et al., 2005; Papagiannakopoulos et al., 2016). Administration of the viral Cre recombinase via the trachea induces the genetic rearrangement of the Lox-stop-Lox cassette to activate oncogenic Kras and delete the tumor suppressor p53. Lung tumors developed uniformly with 100% penetrance and tumors can distinctively be observed two to 3 months post-infection (Masri et al., 2016; Verlande et al., 2021). WT mice had an average weight of 32.54 g versus 27.18 g for TB mice at 4 months post-infection (Figure 2A), indicating that lung TB mice exhibited a significant weight loss compared to WT mice. Consistent with the development of cachexia, TB mice displayed a loss of adipose tissue and skeletal muscle as determined by fat mass and lean mass measurement using EchoMRI (magnetic resonance imaging) (Figure 2B), which is consistent with our previous findings (Masri et al., 2016; Verlande et al., 2021). Metabolic phenotyping analysis using indirect calorimetry was performed to better characterize this GEMM. The respiratory exchange ratio (RER) is the ratio between the amount of CO2 that is produced to the amount of O2 that is consumed. RER oscillates with a daily pattern, reaching the lowest point during the light/resting phase and the highest point during the dark/active phase (Figure 2C). The amount of CO2 that is produced in the TCA cycle and the amount of O2 that is consumed during oxidative phosphorylation varies depending on the nutrients (monosaccharides, fatty acids, amino acids) that are used for substrate utilization. If glucose is used as a substrate, RER will be approximately 1, as six molecules of O2 are required to produce six molecules of CO2 for glucose oxidation. Most glucose molecules are derived from diet and oxidation occurs during the dark/active phase in mice. Compared to carbohydrates, fatty acids require more O2 molecules for oxidation and therefore the RER value will be less than 1. Fatty acid oxidation occurs during the light/resting phase when carbohydrates are not available from the diet. Interestingly, we observed a significant difference in the RER during the light phase, where TB mice exhibited a lower RER (Figure 2C). These data indicate that TB mice utilize fatty acids as a fuel source during the resting phase, more than the WT mice.
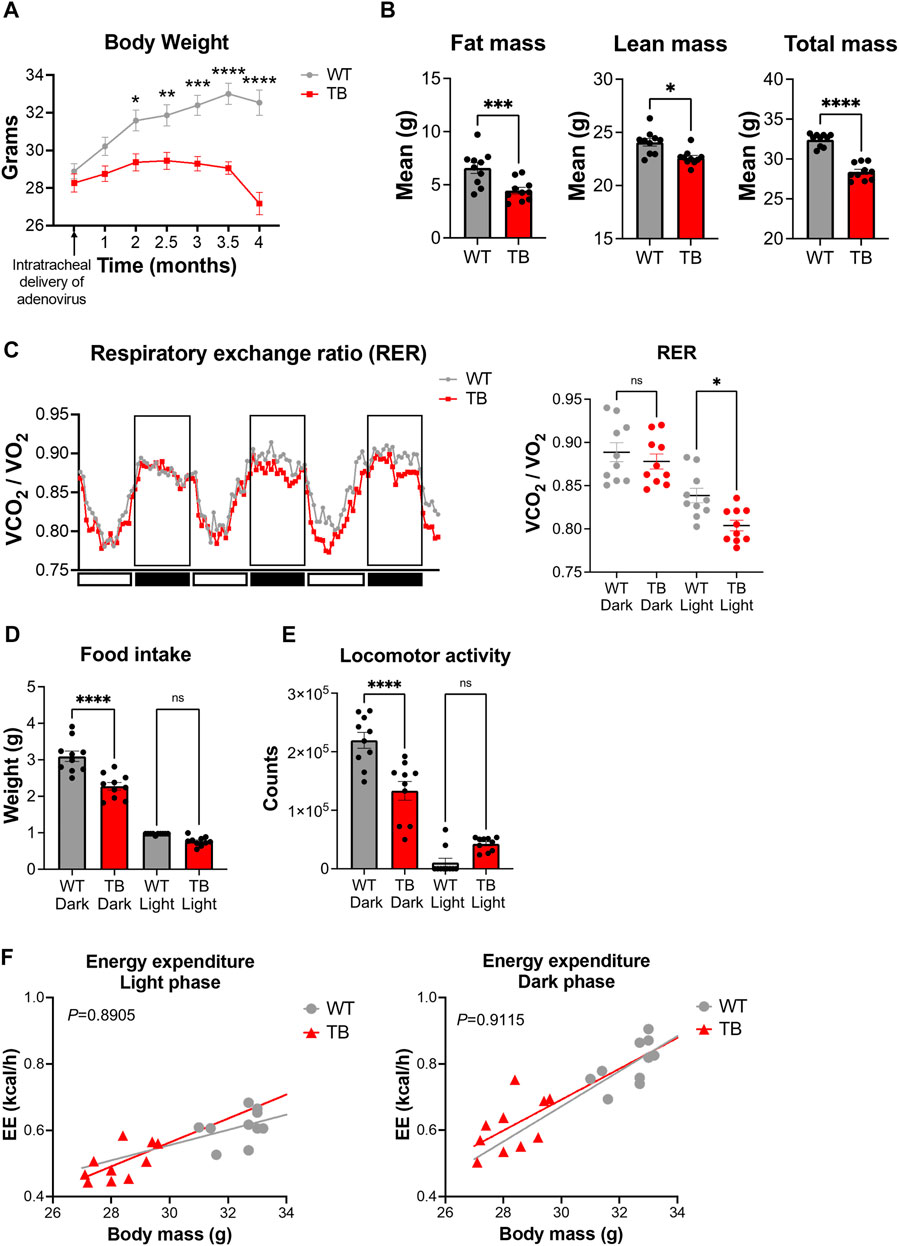
FIGURE 2. Metabolic phenotyping of a mouse model of lung cancer-associated cachexia. (A) Body weight over the course of 4 months post-intratracheal delivery of the adenovirus FlpO or Cre recombinase. Data represent the mean ± SEM with p value cutoff indicated as *p < 0.05, **p < 0.01 ***p < 0.001, and ****p < 0.0001 as determined by two-way ANOVA. (B) Fat, lean, and total mass measurements at 4 months post-delivery of adenovirus (n = 10 mice per genotype). Data represent the mean ± SEM with p value cutoff indicated as *p < 0.05, ***p < 0.001, and ****p < 0.0001 as determined by Student’s t-test. (C) (Left) RER (VCO2/VO2) in WT and TB mice over 3 days. (Right) Quantification of the mean RER in WT and TB mice over 4 days (n = 10 mice). Data represent the mean ± SEM with p value cutoff indicated as *p < 0.05 as determined by one-way ANOVA. (D) Food intake of WT and TB mice over 4 days (n = 10 mice). Data represent the mean ± SEM with p value cutoff indicated as ****p < 0.0001 as determined by one-way ANOVA. (E) Locomotor activity in WT and TB mice over 4 days (n = 10 mice). Data represent the mean ± SEM with p value cutoff indicated as ****p < 0.0001 as determined by one-way ANOVA. (F) Linear regression plots of TEE are the average heat production (H3 in kcal/h) plotted to body mass during the dark and light phases. Formula of the regression plot from the light phase for WT mice is y = 0.023 × −0.1346 and TB mice is y = 0.03616–0.5214. For the dark phase, formula of the regression plot for WT mice is y = 0.05309 × −0.9206 and TB mice is y = 0.04664–0.7068. p values for group differences in slopes are 0.6193 for light phase and 0.8599 for dark phase. ns, not significant.
Next, we looked at the food consumption and locomotor activity, two parameters that contribute to energy homeostasis. We observed a significant decrease in food intake (Figure 2D) and locomotor activity (Figure 2E) in TB mice during the dark/active phase, even though we did not observe differences in RER values between WT and TB mice (Figure 2C). We then looked at the total energy expenditure (TEE) relative to body weight using a regression-based approach (Kaiyala and Schwartz, 2011; Tschöp et al., 2011). TEE can be divided into activity energy expenditure (AEE) and resting energy expenditure (REE) (Hills et al., 2014). TB mice displayed a similar AEE during the dark/active phase and comparable REE during the light/resting cycle compared to WT despite the presence of lung tumors (Figure 2F). However, skeletal muscle is the main tissue contributing to TEE (Tschöp et al., 2011; Goncalves et al., 2018; Queiroz et al., 2022) and TB mice exhibited decreased lean mass and locomotor activity (Figures 2D,E). Taken together, these data suggest that the decreased supply of dietary energy is compensated by a reduction in energy consumed for movement as AEE and REE were similar between both genotypes.
Metabolic perturbation in a mouse model of diet-induced obesity
To characterize metabolic deregulation in DIO, male C57BL/6J mice were fed with control low-fat (CTL, 10% kcal from fat) or high-fat diet (HFD, 45% kcal from fat) for 4 weeks. Body weight changes were measured weekly and showed that HFD fed mice gained significantly more body weight than CTL diet fed mice, starting from the third week of feeding (Figure 3A). After 4 weeks of feeding, CTL diet fed mice had an average body weight of 27.45 g versus 30.85 g for the HFD fed group (Figure 3A). To further validate the DIO phenotype, we performed whole-body composition analysis of these mice by EchoMRI. Average fat mass of HFD fed mice was 7.19 g versus 3.90 g for the CTL fed group (Figure 3B). Conversely, average lean mass in HFD fed mice was not significantly different from CTL diet fed mice (Figure 3B), suggesting that body weight gain in HFD fed mice is a result of fat accumulation. These results show that 4 weeks of HFD feeding promotes fat accumulation and weight gain, without a significant impact on lean mass, which is consistent with previous studies (Fisher-Wellman et al., 2016).
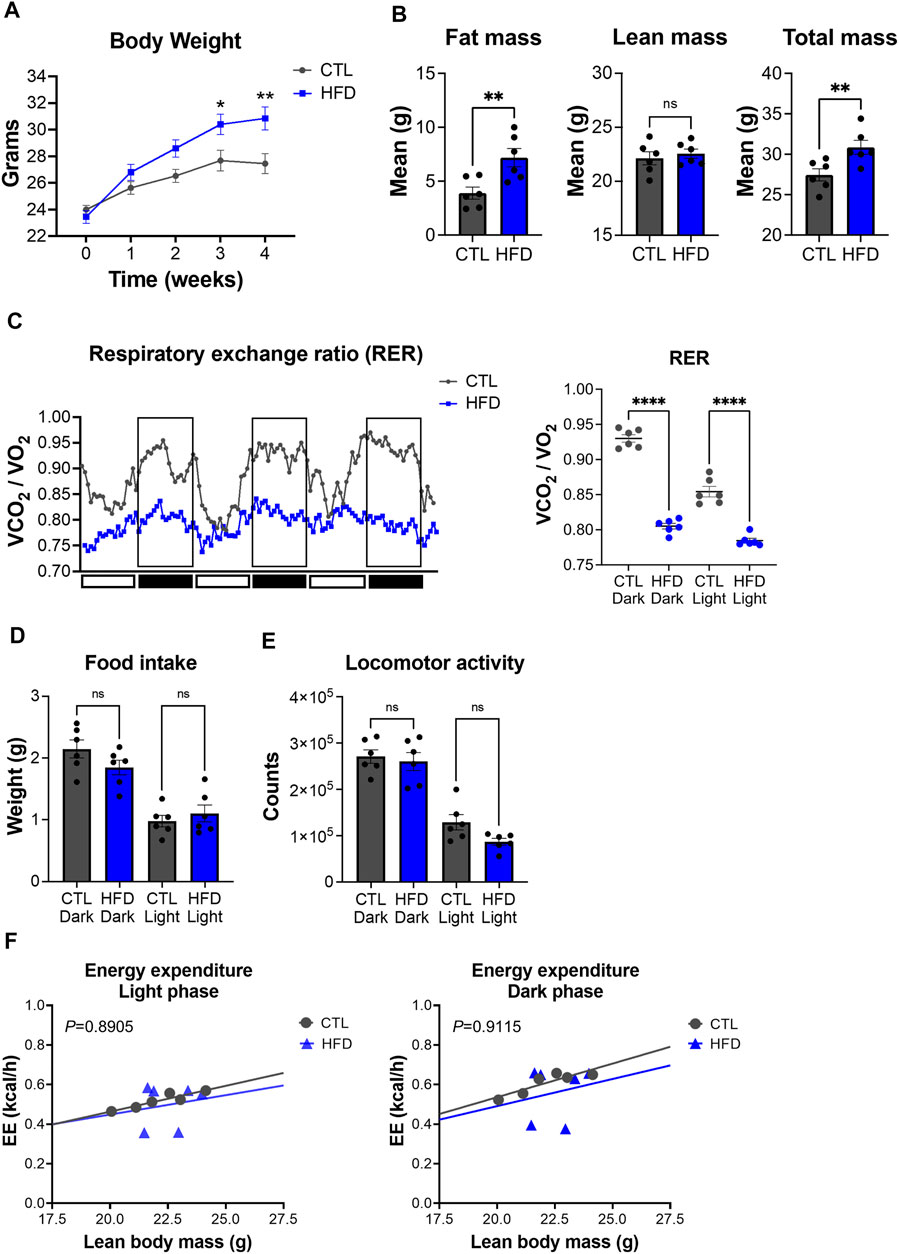
FIGURE 3. Metabolic phenotyping of a mouse model of DIO. (A) Body weight over the course of 4 weeks with feeding of low-fat control diet (CTL) or HFD. Data represent the mean ± SEM with p value cutoff indicated as *p < 0.05 and **p < 0.01 as determined by two-way ANOVA. (B) Fat, lean, and total mass measurements at 4 weeks after feeding of CTL or HFD (n = 6 mice per group). Data represent the mean ± SEM with p value cutoff indicated as **p < 0.01 determined by Student’s t-test. (C) (Left) RER (VCO2/VO2) in CTL and HFD fed mice over 3 days. (Right) Quantification of the mean RER in CTL and HFD fed mice over 4 days (n = 6 mice). Data represent the mean ± SEM with p value cutoff indicated as ****p < 0.0001 as determined by one-way ANOVA. (D) Food intake in CTL and HFD fed mice over 4 days (n = 6). Data represent the mean ± SEM and statistical significance was determined by one-way ANOVA. (E) Locomotor activity in CTL and HFD fed mice over 4 days (n = 6). Data represent the mean ± SEM and statistical significance was determined by one-way ANOVA. (F) Linear regression plots of TEE are the average heat production (H3 in kcal/h) plotted to lean body mass during the dark and light phases. Formula of the regression plot from the light phase for CTL mice is y = 0.02609 × −0.05851 and HFD mice is y = 0.0196 + 0.05654. For dark phase, formula of the regression plot for CTL mice is y = 0.03397 × −0.1431 and HFD mice is y = 0.02740 × −0.05685. p values for group differences in slopes 0.8905 for light phase and 0.9115 for dark phase. ns, not significant.
To further dissect metabolic dysfunction, we performed indirect calorimetry with our DIO mouse model. While CTL diet fed mice showed rhythmicity of RER over the day/night cycle, HFD fed mice display a significant reduction of RER, which was accompanied with a loss of daily rhythmicity (Figure 3C). These data suggest that HFD fed mice have a higher contribution of fat oxidation as an energy source rather than carbohydrates, which is a hallmark feature of DIO (Eckel et al., 2005). Unlike RER, HFD feeding induced no significant changes in locomotor activity and food intake in both light and dark phases (Figures 3D,E). TEE changes relative to lean body mass did not differ between CTL and HFD fed mice (Figure 3F). These results suggest that metabolic alterations found in our DIO mouse model are not derived from changes in food intake or physical activity, but from fat used as a substrate for oxidation under HFD feeding. Overall, our data demonstrate that HFD fed mice harbor metabolic deficits that model DIO and IR.
Real-time U-13C glucose oxidation in two pathophysiological mouse models
Considering the phenotypic changes that were observed in both mouse models, systemic glucose metabolism was assessed by measuring exhaled 13CO2 and total CO2 using a 13C-glucose tracer and stable isotope sensors. Environmental levels of 12C and 13C isotopes in the sealed cages were determined prior to the start of the experiment and were 98.77% ± 1.4% and 1.12% ± 0.08% for 12CO2 and 13CO2, respectively (Figure 4A). This is in accordance with the natural abundance of 13CO2 of 1.109%. The experimental design is depicted in Figure 4B. Each mouse received a mixture of 0.2 g/kg of 12C-glucose and 0.1 g/kg of 13C-glucose, and real-time detection of glucose uptake and consumption was determined. We observed a significant increase in oxidation of 13C-glucose in TB mice compared to WT mice (Figure 4C). The level of 13CO2 in the air reached 2.75% in TB mice compared to 2% in WT after 26 min. Glucose oxidation was significantly elevated in TB mice up to 182 min. These data demonstrate that lung TB mice uptake and oxidize more glucose than WT littermates.
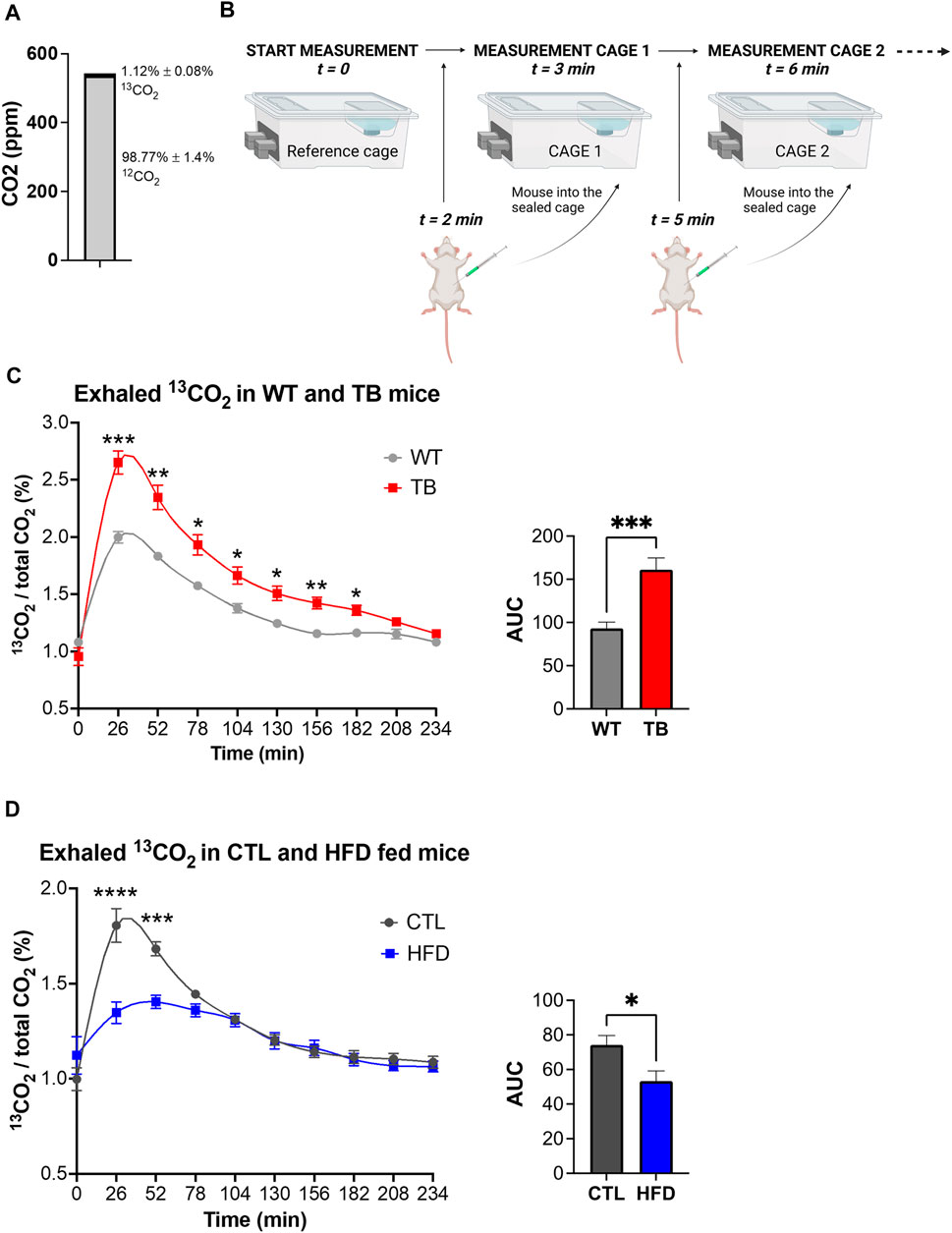
FIGURE 4. Glucose oxidation in two pathophysiological mouse models. (A) Environmental levels of 13CO2 and 12CO2 in the metabolic cages. (B) Experimental design depicting the reference cage and two of the experimental cages. (C) Measurements of exhaled 13CO2 normalized to total CO2 and area under the curve (AUC) in WT and TB mice (n = 10 mice per genotype). Data represent the mean ± SEM with p value cutoff indicated as *p < 0.05, **p < 0.01, and ***p < 0.001 as determined by two-way ANOVA and Student’s t-test. (D) Measurements of exhaled 13CO2 normalized to total CO2 and area under the curve (AUC) in CTL and HFD fed mice (n = 6 mice per diet type). Data represent the mean ± SEM with p value cutoff indicated as *p < 0.05 and ***p < 0.001, and ****p < 0.0001 as determined by two-way ANOVA and Student’s t-test.
Using a similar approach, we measured 13C-glucose oxidation in the DIO mouse model. Each mouse received a mixture of 0.2 g/kg of 12C-glucose and 0.1 g/kg of 13C-glucose based on their lean body mass. The ratio of expired 13CO2 to 12CO2 was measured and a significant reduction was found in HFD fed mice compared to CTL (Figure 4D), suggesting a decrease in glucose oxidation. The maximum level of 13CO2 in the air reached 1.5% in HFD fed mice versus 2% in WT after 26 min. Taken together, TB mice oxidize more glucose than WT littermates, while HFD fed mice uptake and oxidize less glucose than CTL fed mice. These results demonstrate that the uptake and oxidation of glucose vary in healthy and disease states and that stable isotope sensors accurately quantify glucose oxidation in lung cancer and DIO mouse models.
Discussion
In this study, we performed a metabolic analysis of the phenotypic changes and glucose utilization in a model of lung cancer-associated cachexia and DIO. We demonstrated that energy metabolism is altered in both pathophysiological mouse models. The lung cancer-associated cachexia GEMM exhibited a reduction in food intake, lean and fat mass, suggesting increased global catabolism (Baracos et al., 2018). Active protein and lipid turnover are observed in patients with cachexia and whole-body proteolysis and lipolysis rates increase by 40% and 50%, respectively (Hall and Baracos, 2008). Also, the rate of fatty acid release is greater in cachexic versus non-cachexic animals (Beck and Tisdale, 2004) and we observed an increased fat oxidation during the resting phase in TB mice. Insulin and glucagon regulate lipolysis and we previously showed that serum glucagon is elevated (Verlande et al., 2021) and insulin signaling is dampened in late-stage lung TB mice (Masri et al., 2016), preventing insulin from exerting its anti-lipolytic effect. In addition, proinflammatory cytokines stimulate lipolysis and fat oxidation in humans (van Hall et al., 2003), and increased tumor-secreted IL-6 is characteristic in animal models of cancer cachexia (Strassmann et al., 1992; Das et al., 2011; Petruzzelli et al., 2014; Masri et al., 2016), as well as in cachectic cancer patients (Staal-van den Brekel et al., 1995; Weidle et al., 2010). We did not observe differences in activity or resting energy expenditure despite the presence of lung tumors. However, skeletal muscle is a primary determinant of TEE (Speakman, 2013; Goncalves et al., 2018; Queiroz et al., 2022) and TB mice exhibited decreased lean mass and locomotor activity. In contrast, early-onset obese mice did not display changes in lean mass or locomotor activity but exhibited increased body weight and fat mass. Body mass is strongly correlated with diabetes and IR and elevated plasma levels of non-esterified fatty acids have been shown to account for up to 50% of IR, which impairs glucose utilization in peripheral tissues (Boden, 2002).
Glucose is considered one the most important circulating energy precursors and we show that its use is oppositely regulated in these pathophysiological models. Our findings highlight that lung TB animals exhibited enhanced glucose uptake, oxidation and mitochondrial function, and this is in accordance with previous studies (Fan et al., 2009; Hensley et al., 2016). It has been reported that increased mitochondrial function is mainly due to elevated pyruvate carboxylation in lung tumors (Fan et al., 2009; Sellers et al., 2015). In addition, oncogenic signaling enhances expression of glucose transporters and glycolytic enzymes in cancer cells, which results in increased glucose uptake and subsequent biomass production (Vander Heiden and DeBerardinis, 2017). Additionally, lung tumors impinge on hepatic metabolism at a distance by rewiring insulin signaling and promoting de novo glucose production (Masri et al., 2016; Verlande et al., 2021), thereby disrupting the circadian rhythmicity of plasma glucose.
In the context of DIO, our results showed that IR reduced glucose uptake, oxidation, and mitochondrial function, and resulted in increased fat oxidation. Skeletal muscle plays a critical role in glucose homeostasis and is responsible for uptake of 70%–90% of the glucose from the blood in the postprandial state (DeFronzo et al., 1981; Baron et al., 1988). Previous studies demonstrated that IR in skeletal muscle can be attributed to impaired insulin signaling, reduced insulin-stimulated glucose uptake and transport, and reduced glucose transporter type 4 (GLUT4) translocation (Cline et al., 1994; Ciaraldi et al., 1995; Roden et al., 1996; Dresner et al., 1999; Griffin et al., 1999; Leto and Saltiel, 2012). It has been shown that muscle clock disruption reduces protein levels of GLUT4 and impairs insulin-stimulated glucose uptake (Dyar et al., 2014) and that high-fat diet alters clock-controlled genes governing fuel utilization (Kohsaka et al., 2007; Eckel-Mahan et al., 2013). Additionally, it has been reported that obesity-related IR in skeletal muscle is defined by hyperactivated mitochondrial β-oxidation, impairing the transition from fatty acid to carbohydrate oxidation during the fasted to fed state (An et al., 2004; Koves et al., 2005; Koves et al., 2008). Indeed, glucose and glucose-6-phosphate are reduced in skeletal muscle of diabetic patients (Cline et al., 1994; Cline et al., 1999) and during a lipid infusion, implying that glucose uptake is the rate-limiting step in DIO.
13C-glucose breath tests have been reported as an alternative method to measure insulin sensitivity to screen pre-diabetic and diabetic individuals, in contrast to the invasive hyperinsulinemic-euglycemic clamp (Mizrahi et al., 2010; Hussain et al., 2014). Exhaled breath test analysis may also be a promising approach for cancer detection (Dharmawardana et al., 2020; Meng et al., 2021; Kwon et al., 2022). To date, several breath tests have been successfully applied in the clinic for the assessment of gastroenterological symptoms and diseases. 13C-urea breath tests have been used to detect Helicobacter pylori infection in the stomach, where labeled urea is metabolized to produce 13CO2, which is transported to the lung to be exhaled (Alzoubi et al., 2020). Additionally, 13C-gastric breath tests have been used to document gastric emptying time (Keller et al., 2018). 13C-liver function breath tests can measure the elimination of a substance which is exclusively cleared by the liver (Dietrich et al., 2016). These tests have been used in patients with liver fibrosis and cirrhosis (Giannini and Savarino, 2013; Afolabi et al., 2018), and hepatocellular carcinoma (Palmieri et al., 2009). Finally, 13C-pancreatic function breath tests have been used to evaluate the exocrine function of the pancreas which is often disrupted in chronic pancreatitis and pancreatic cancer (Keller and Layer, 2005). While the 13C-urea breath is highly standardized and well-established, the 13C-gastric, liver and pancreatic breath tests are not widely used due to a lack of standardization. In this study, we provide evidence that altered systemic metabolism can be leveraged using 13C-glucose as a method to track lung cancer progression and hallmarks of DIO.
Materials and methods
Animal housing and experimental procedures
Male 7-month-old C57BL/6J KrasLSL-G12D/+;p53fl/fl mice have been previously described (Jackson et al., 2001; Johnson et al., 2001; Jackson et al., 2005; DuPage et al., 2009). Littermates were housed in a standard 12-hour light/dark paradigm and fed ad libitum with standard vivarium chow (TEKLAD Envigo 2020X Global Soy Protein-free Extruded Rodent Diet). For viral infection, ad5-CMV: FlpO or ad5-CMV: Cre (University of Iowa, Viral Vector Core) were used at a titer of 3 × 107 plaque-forming units and administered by intratracheal delivery. The intratracheal instillation method has been described previously (Jackson et al., 2001; Jackson et al., 2005). Equivalent titer of viral FlpO recombinase (ad5-CMV: FlpO) was delivered to p53fl/fl littermates of the same C57BL/6J background as a control that does not induce recombination to maintain wild-type expression of Kras and p53 (Masri et al., 2016; Verlande et al., 2021). At 3.75 month after viral infection, metabolic phenotyping of the mice was performed by indirect calorimetry. Male 8-week-old C57BL/6J mice were purchased from The Jackson Laboratory (#00064, Bar Harbor, ME). Mice were divided randomly into two groups and fed ad libitum with high-fat (45% calories from fat, D12451, Research Diet) or low-fat control (CTL) diet (10% calories from fat, D12450H, Research Diet) for 4 weeks. Body weight changes were measured every week. Metabolic phenotyping of the mice was performed by indirect calorimetry at 4 weeks after dietary challenge. All experiments were performed in accordance with the Institutional Animal Care and Use Committee (IACUC) guidelines at the University of California, Irvine.
Indirect calorimetry and echo magnetic resonance imaging
Oxygen consumption (ml/h), carbon dioxide release (ml/h), respiratory exchange ratio (RER), locomotor activity (counts), and food intake (grams) were monitored for individually housed mice using the Phenomaster metabolic cages (TSE Systems Inc., Chesterfield, MO). The climate chamber was set to 21°C, 50% humidity with a 12:12 light-dark cycle as the home-cage environment. Animals were entrained for 2 days in the metabolic cages before the start of each experiment to allow for environmental acclimation. Data were collected at 40-minute intervals and each cage was recorded for 3.25 min before time point collection. Body composition was measured using EchoMRI™ Whole Body Composition Analyzer (Houston, TX, United States) which provides whole body fat and lean mass measurements.
In vivo sensing of 13CO2 and total CO2
Mice were acclimated for 2 days before the experiment. Mice were morning fasted for 6 h before and during the stable isotope experiment. Environmental levels of 13CO2 and total CO2 in the sealed cages were calibrated in order to have a percent of ± 1.1% 13CO2, as the natural abundance of 13C. Measurements were started at t = 0 min, the first mouse was intraperitoneally injected at t = 2 min with a solution of 0.2 g/kg of 12C glucose mixed with 0.1 g/kg of U-13C glucose, and placed into the first sealed cage. The second mouse was IP injected at t = 5 min when the first cage was being measured. Each cage was measured for 3 min. Exhaled 13CO2 for each cage was normalized to total CO2 abundance to quantify changes in systemic glucose utilization, without accounting for differences in total respiration. Overall, changes of exhaled 13CO2 levels over time were analyzed by area under the curve (AUC) analysis after subtraction of the area below 1% which is considered baseline.
Data availability statement
The raw data supporting the conclusions of this article will be made available by the authors, without undue reservation.
Ethics statement
The animal study was reviewed and approved by IACUC, University of California, Irvine.
Author contributions
AV, SKC, and SM conceived the research and designed the experiments. AV, SKC, and WAS performed experiments. AV and SKC performed data analysis. AV, SKC, DO, HJK, and SM discussed data and wrote the manuscript with input from all co-authors.
Funding
The authors wish to thank members of the Masri lab for helpful discussion and feedback on the manuscript. The authors wish to acknowledge the support of the Chao Family Comprehensive Cancer Center (CFCCC) at the University of California, Irvine, which is supported by the National Institutes of Health (NIH)/National Cancer Institute (NCI) (Grant # P30 CA062203). AV was supported by the Hitachi-Nomura Postdoctoral Fellowship through the Department of Biological Chemistry, University of California, Irvine. Financial support for the Masri laboratory is provided through the NIH/NCI (Grants: R01CA244519, R01CA259370, K22CA212045), the Concern Foundation, the V Foundation for Cancer Research, the Cancer Research Coordinating Committee, Johnson and Johnson, and support from the Anti-Cancer Challenge through the CFCCC.
Acknowledgments
The authors wish to thank members of the Masri lab for helpful discussion and feedback on the manuscript. The authors wish to acknowledge the support of the Chao Family Comprehensive Cancer Center (CFCCC) at the University of California, Irvine, which is supported by the National Institutes of Health (NIH)/National Cancer Institute (NCI) (Grant # P30 CA062203).
Conflict of interest
Authors DO and HJK were employed by TSE Systems Inc.
The remaining authors declare that the research was conducted in the absence of any commercial or financial relationships that could be construed as a potential conflict of interest.
Publisher’s note
All claims expressed in this article are solely those of the authors and do not necessarily represent those of their affiliated organizations, or those of the publisher, the editors and the reviewers. Any product that may be evaluated in this article, or claim that may be made by its manufacturer, is not guaranteed or endorsed by the publisher.
References
Afolabi P. R., Scorletti E., Smith D. E., Almehmadi A. A., Calder P. C., Byrne C. D. (2018). The characterisation of hepatic mitochondrial function in patients with non-alcoholic fatty liver disease (NAFLD) using the 13 C-ketoisocaproate breath test. J. Breath. Res. 12, 046002. doi:10.1088/1752-7163/aacf12
Altman B. J., Hsieh A. L., Sengupta A., Krishnanaiah S. Y., Stine Z. E., Walton Z. E., et al. (2015). MYC disrupts the circadian clock and metabolism in cancer cells. Cell Metab. 22, 1009–1019. doi:10.1016/j.cmet.2015.09.003
Alzoubi H., Al-Mnayyis A., Al rfoa I., Aqel A., Abu-Lubad M., Hamdan O., et al. (2020). The use of 13C-urea breath test for non-invasive diagnosis of Helicobacter pylori infection in comparison to endoscopy and stool antigen test. Diagnostics 10, 448. doi:10.3390/diagnostics10070448
An J., Muoio D. M., Shiota M., Fujimoto Y., Cline G. W., Shulman G. I., et al. (2004). Hepatic expression of malonyl-CoA decarboxylase reverses muscle, liver and whole-animal insulin resistance. Nat. Med. 10, 268–274. doi:10.1038/nm995
Arkan M. C., Hevener A. L., Greten F. R., Maeda S., Li Z.-W., Long J. M., et al. (2005). IKK-beta links inflammation to obesity-induced insulin resistance. Nat. Med. 11, 191–198. doi:10.1038/nm1185
Baracos V. E., Martin L., Korc M., Guttridge D. C., Fearon K. C. H. (2018). Cancer-associated cachexia. Nat. Rev. Dis. Prim. 4, 17105. doi:10.1038/nrdp.2017.105
Baron A. D., Brechtel G., Wallace P., Edelman S. V. (1988). Rates and tissue sites of non-insulin- and insulin-mediated glucose uptake in humans. Am. J. Physiol. 255, E769–E774. doi:10.1152/ajpendo.1988.255.6.E769
Beck S. A., Tisdale M. J. (2004). Effect of cancer cachexia on triacylglycerol/fatty acid substrate cycling in white adipose tissue. Lipids 39, 1187–1189. doi:10.1007/s11745-004-1346-8
Boden G. (2002). Interaction between free fatty acids and glucose metabolism. Curr. Opin. Clin. Nutr. Metab. Care 5, 545–549. doi:10.1097/00075197-200209000-00014
Braden B. (2009). Methods and functions: Breath tests. Best. Pract. Res. Clin. Gastroenterol. 23, 337–352. doi:10.1016/j.bpg.2009.02.014
Buescher J. M., Antoniewicz M. R., Boros L. G., Burgess S. C., Brunengraber H., Clish C. B., et al. (2015). A roadmap for interpreting 13 C metabolite labeling patterns from cells. Curr. Opin. Biotechnol. 34, 189–201. doi:10.1016/j.copbio.2015.02.003
Ciaraldi T. P., Abrams L., Nikoulina S., Mudaliar S., Henry R. R. (1995). Glucose transport in cultured human skeletal muscle cells. Regulation by insulin and glucose in nondiabetic and non-insulin-dependent diabetes mellitus subjects. J. Clin. Invest. 96, 2820–2827. doi:10.1172/JCI118352
Cline G. W., Petersen K. F., Krssak M., Shen J., Hundal R. S., Trajanoski Z., et al. (1999). Impaired glucose transport as a cause of decreased insulin-stimulated muscle glycogen synthesis in type 2 diabetes. N. Engl. J. Med. 341, 240–246. doi:10.1056/NEJM199907223410404
Cline G. W., Rothman D. L., Magnusson I., Katz L. D., Shulman G. I. (1994). 13C-nuclear magnetic resonance spectroscopy studies of hepatic glucose metabolism in normal subjects and subjects with insulin-dependent diabetes mellitus. J. Clin. Invest. 94, 2369–2376. doi:10.1172/JCI117602
Czech M. P. (2017). Insulin action and resistance in obesity and type 2 diabetes. Nat. Med. 23, 804–814. doi:10.1038/nm.4350
Das S. K., Eder S., Schauer S., Diwoky C., Temmel H., Guertl B., et al. (2011). Adipose triglyceride lipase contributes to cancer-associated cachexia. Science 333, 233–238. doi:10.1126/science.1198973
DeFronzo R. A., Jacot E., Jequier E., Maeder E., Wahren J., Felber J. P. (1981). The effect of insulin on the disposal of intravenous glucose: Results from indirect calorimetry and hepatic and femoral venous catheterization. Diabetes 30, 1000–1007. doi:10.2337/diab.30.12.1000
Dharmawardana N., Goddard T., Woods C., Watson D. I., Ooi E. H., Yazbeck R. (2020). Development of a non-invasive exhaled breath test for the diagnosis of head and neck cancer. Br. J. Cancer 123, 1775–1781. doi:10.1038/s41416-020-01051-9
Diehl F. F., Miettinen T. P., Elbashir R., Nabel C. S., Darnell A. M., Do B. T., et al. (2022). Nucleotide imbalance decouples cell growth from cell proliferation. Nat. Cell Biol. 24, 1252–1264. doi:10.1038/s41556-022-00965-1
Dietrich C. G., Gotze O., Geier A. (2016). Molecular changes in hepatic metabolism and transport in cirrhosis and their functional importance. World J. Gastroenterol. 22, 72–88. doi:10.3748/wjg.v22.i1.72
Dresner A., Laurent D., Marcucci M., Griffin M. E., Dufour S., Cline G. W., et al. (1999). Effects of free fatty acids on glucose transport and IRS-1–associated phosphatidylinositol 3-kinase activity. J. Clin. Invest. 103, 253–259. doi:10.1172/JCI5001
DuPage M., Dooley A. L., Jacks T. (2009). Conditional mouse lung cancer models using adenoviral or lentiviral delivery of Cre recombinase. Nat. Protoc. 4, 1064–1072. doi:10.1038/nprot.2009.95
Dyar K. A., Ciciliot S., Wright L. E., Biensø R. S., Tagliazucchi G. M., Patel V. R., et al. (2014). Muscle insulin sensitivity and glucose metabolism are controlled by the intrinsic muscle clock. Mol. Metab. 3, 29–41. doi:10.1016/j.molmet.2013.10.005
Eckel R. H., Grundy S. M., Zimmet P. Z. (2005). The metabolic syndrome. Lancet 365, 1415–1428. doi:10.1016/S0140-6736(05)66378-7
Eckel-Mahan K. L., Patel V. R., de Mateo S., Orozco-Solis R., Ceglia N. J., Sahar S., et al. (2013). Reprogramming of the circadian clock by nutritional challenge. Cell 155, 1464–1478. doi:10.1016/j.cell.2013.11.034
Fabbrini E., Magkos F., Mohammed B. S., Pietka T., Abumrad N. A., Patterson B. W., et al. (2009). Intrahepatic fat, not visceral fat, is linked with metabolic complications of obesity. Proc. Natl. Acad. Sci. U. S. A. 106, 15430–15435. doi:10.1073/pnas.0904944106
Fan T. W. M., Lane A. N., Higashi R. M., Farag M. A., Gao H., Bousamra M., et al. (2009). Altered regulation of metabolic pathways in human lung cancer discerned by (13)C stable isotope-resolved metabolomics (SIRM). Mol. Cancer 8, 41. doi:10.1186/1476-4598-8-41
Faubert B., Li K. Y., Cai L., Hensley C. T., Kim J., Zacharias L. G., et al. (2017). Lactate metabolism in human lung tumors. Cell 171, 358–371. doi:10.1016/j.cell.2017.09.019
Fernández-Calleja J. M. S., Bouwman L. M. S., Swarts H. J. M., Oosting A., Keijer J., van Schothorst E. M. (2019). Extended indirect calorimetry with isotopic CO2 sensors for prolonged and continuous quantification of exogenous vs. total substrate oxidation in mice. Sci. Rep. 9, 11507. doi:10.1038/s41598-019-47977-w
Fisher-Wellman K. H., Ryan T. E., Smith C. D., Gilliam L. A. A., Lin C.-T., Reese L. R., et al. (2016). A direct comparison of metabolic responses to high-fat diet in C57bl/6J and C57bl/6NJ mice. Diabetes 65, 3249–3261. doi:10.2337/db16-0291
Giannini E. G., Savarino V. (2013). Relationship between 13C-aminopyrine breath test and the MELD score and its long-term prognostic use in patients with cirrhosis. Dig. Dis. Sci. 58, 3024–3028. doi:10.1007/s10620-013-2739-1
Goncalves M. D., Hwang S.-K., Pauli C., Murphy C. J., Cheng Z., Hopkins B. D., et al. (2018). Fenofibrate prevents skeletal muscle loss in mice with lung cancer. Proc. Natl. Acad. Sci. U. S. A. 115, E743. doi:10.1073/pnas.1714703115
Griffin M. E., Marcucci M. J., Cline G. W., Bell K., Barucci N., Lee D., et al. (1999). Free fatty acid-induced insulin resistance is associated with activation of protein kinase C theta and alterations in the insulin signaling cascade. Diabetes 48, 1270–1274. doi:10.2337/diabetes.48.6.1270
Guan D., Lazar M. A. (2021). Interconnections between circadian clocks and metabolism. J. Clin. Invest. 131, e148278. doi:10.1172/JCI148278
Hall K. D., Baracos V. E. (2008). Computational modeling of cancer cachexia. Curr. Opin. Clin. Nutr. Metab. Care 11, 214–221. doi:10.1097/MCO.0b013e3282f9ae4d
Hanahan D. (2022). Hallmarks of cancer: New dimensions. Cancer Discov. 12, 31–46. doi:10.1158/2159-8290.CD-21-1059
Hatori M., Vollmers C., Zarrinpar A., DiTacchio L., Bushong E. A., Gill S., et al. (2012). Time-restricted feeding without reducing caloric intake prevents metabolic diseases in mice fed a high-fat diet. Cell Metab. 15, 848–860. doi:10.1016/j.cmet.2012.04.019
Hensley C. T., Faubert B., Yuan Q., Lev-Cohain N., Jin E., Kim J., et al. (2016). Metabolic heterogeneity in human lung tumors. Cell 164, 681–694. doi:10.1016/j.cell.2015.12.034
Hills A. P., Mokhtar N., Byrne N. M. (2014). Assessment of physical activity and energy expenditure: An overview of objective measures. Front. Nutr. 1, 5. doi:10.3389/fnut.2014.00005
Hojo H., Enya S., Arai M., Suzuki Y., Nojiri T., Kangawa K., et al. (2017). Remote reprogramming of hepatic circadian transcriptome by breast cancer. Oncotarget 8, 34128–34140. doi:10.18632/oncotarget.16699
Hui S., Ghergurovich J. M., Morscher R. J., Jang C., Teng X., Lu W., et al. (2017). Glucose feeds the TCA cycle via circulating lactate. Nature 551, 115–118. doi:10.1038/nature24057
Hussain M., Jangorbhani M., Schuette S., Considine R. V., Chisholm R. L., Mather K. J. (2014). [ 13 C]glucose breath testing provides a noninvasive measure of insulin resistance: Calibration analyses against clamp studies. Diabetes Technol. Ther. 16, 102–112. doi:10.1089/dia.2013.0151
Jackson E. L., Olive K. P., Tuveson D. A., Bronson R., Crowley D., Brown M., et al. (2005). The differential effects of mutant p53 alleles on advanced murine lung cancer. Cancer Res. 65, 10280–10288. doi:10.1158/0008-5472.CAN-05-2193
Jackson E. L., Willis N., Mercer K., Bronson R. T., Crowley D., Montoya R., et al. (2001). Analysis of lung tumor initiation and progression using conditional expression of oncogenic K-ras. Genes Dev. 15, 3243–3248. doi:10.1101/gad.943001
Jang C., Chen L., Rabinowitz J. D. (2018). Metabolomics and isotope tracing. Cell 173, 822–837. doi:10.1016/j.cell.2018.03.055
Johnson L., Mercer K., Greenbaum D., Bronson R. T., Crowley D., Tuveson D. A., et al. (2001). Somatic activation of the K-ras oncogene causes early onset lung cancer in mice. Nature 410, 1111–1116. doi:10.1038/35074129
Kaiyala K. J., Schwartz M. W. (2011). Toward a more complete (and less controversial) understanding of energy expenditure and its role in obesity pathogenesis. Diabetes 60, 17–23. doi:10.2337/db10-0909
Keller J., Bassotti G., Clarke J., Dinning P., Fox M., Grover M., et al. (2018). Expert consensus document: Advances in the diagnosis and classification of gastric and intestinal motility disorders. Nat. Rev. Gastroenterol. Hepatol. 15, 291–308. doi:10.1038/nrgastro.2018.7
Keller J., Layer P. (2005). Human pancreatic exocrine response to nutrients in health and disease. Gut 54, 1–28. doi:10.1136/gut.2005.065946
Kohsaka A., Laposky A. D., Ramsey K. M., Estrada C., Joshu C., Kobayashi Y., et al. (2007). High-fat diet disrupts behavioral and molecular circadian rhythms in mice. Cell Metab. 6, 414–421. doi:10.1016/j.cmet.2007.09.006
Koves T. R., Li P., An J., Akimoto T., Slentz D., Ilkayeva O., et al. (2005). Peroxisome proliferator-activated receptor-gamma co-activator 1alpha-mediated metabolic remodeling of skeletal myocytes mimics exercise training and reverses lipid-induced mitochondrial inefficiency. J. Biol. Chem. 280, 33588–33598. doi:10.1074/jbc.M507621200
Koves T. R., Ussher J. R., Noland R. C., Slentz D., Mosedale M., Ilkayeva O., et al. (2008). Mitochondrial overload and incomplete fatty acid oxidation contribute to skeletal muscle insulin resistance. Cell Metab. 7, 45–56. doi:10.1016/j.cmet.2007.10.013
Krssak M., Falk Petersen K., Dresner A., DiPietro L., Vogel S. M., Rothman D. L., et al. (1999). Intramyocellular lipid concentrations are correlated with insulin sensitivity in humans: A 1H NMR spectroscopy study. Diabetologia 42, 113–116. doi:10.1007/s001250051123
Kwon I.-J., Jung T.-Y., Son Y., Kim B., Kim S.-M., Lee J.-H. (2022). Detection of volatile sulfur compounds (VSCs) in exhaled breath as a potential diagnostic method for oral squamous cell carcinoma. BMC Oral Health 22, 268. doi:10.1186/s12903-022-02301-3
Lamia K. A., Storch K.-F., Weitz C. J. (2008). Physiological significance of a peripheral tissue circadian clock. Proc. Natl. Acad. Sci. U. S. A. 105, 15172–15177. doi:10.1073/pnas.0806717105
Leto D., Saltiel A. R. (2012). Regulation of glucose transport by insulin: Traffic control of GLUT4. Nat. Rev. Mol. Cell Biol. 13, 383–396. doi:10.1038/nrm3351
Lopes M., Brejchova K., Riecan M., Novakova M., Rossmeisl M., Cajka T., et al. (2021). Metabolomics atlas of oral 13C-glucose tolerance test in mice. Cell Rep. 37, 109833. doi:10.1016/j.celrep.2021.109833
Malik V. S., Willett W. C., Hu F. B. (2013). Global obesity: Trends, risk factors and policy implications. Nat. Rev. Endocrinol. 9, 13–27. doi:10.1038/nrendo.2012.199
Marcheva B., Ramsey K. M., Buhr E. D., Kobayashi Y., Su H., Ko C. H., et al. (2010). Disruption of the clock components CLOCK and BMAL1 leads to hypoinsulinaemia and diabetes. Nature 466, 627–631. doi:10.1038/nature09253
Masri S., Papagiannakopoulos T., Kinouchi K., Liu Y., Cervantes M., Baldi P., et al. (2016). Lung adenocarcinoma distally rewires hepatic circadian homeostasis. Cell 165, 896–909. doi:10.1016/j.cell.2016.04.039
Masri S., Sassone-Corsi P. (2018). The emerging link between cancer, metabolism, and circadian rhythms. Nat. Med. 24, 1795–1803. doi:10.1038/s41591-018-0271-8
Meng S., Li Q., Zhou Z., Li H., Liu X., Pan S., et al. (2021). Assessment of an exhaled breath test using high-pressure photon ionization time-of-flight mass spectrometry to detect lung cancer. JAMA Netw. Open 4, e213486. doi:10.1001/jamanetworkopen.2021.3486
Mizrahi M., Lalazar G., Adar T., Raz I., Ilan Y. (2010). Assessment of insulin resistance by a 13C glucose breath test: A new tool for early diagnosis and follow-up of high-risk patients. Nutr. J. 9, 25. doi:10.1186/1475-2891-9-25
Modak A. S. (2007). Stable isotope breath tests in clinical medicine: A review. J. Breath. Res. 1, 014003. doi:10.1088/1752-7155/1/1/014003
Ozcan U., Cao Q., Yilmaz E., Lee A.-H., Iwakoshi N. N., Ozdelen E., et al. (2004). Endoplasmic reticulum stress links obesity, insulin action, and type 2 diabetes. Science 306, 457–461. doi:10.1126/science.1103160
Pachnis P., Wu Z., Faubert B., Tasdogan A., Gu W., Shelton S., et al. (2022). In vivo isotope tracing reveals a requirement for the electron transport chain in glucose and glutamine metabolism by tumors. Sci. Adv. 8, eabn9550. doi:10.1126/sciadv.abn9550
Palmieri V. O., Grattagliano I., Minerva F., Pollice S., Palasciano G., Portincasa P. (2009). Liver function as assessed by breath tests in patients with hepatocellular carcinoma. J. Surg. Res. 157, 199–207. doi:10.1016/j.jss.2008.09.029
Papagiannakopoulos T., Bauer M. R., Davidson S. M., Heimann M., Subbaraj L., Bhutkar A., et al. (2016). Circadian rhythm disruption promotes lung tumorigenesis. Cell Metab. 24, 324–331. doi:10.1016/j.cmet.2016.07.001
Paschos G. K., Ibrahim S., Song W.-L., Kunieda T., Grant G., Reyes T. M., et al. (2012). Obesity in mice with adipocyte-specific deletion of clock component Arntl. Nat. Med. 18, 1768–1777. doi:10.1038/nm.2979
Perelis M., Marcheva B., Ramsey K. M., Schipma M. J., Hutchison A. L., Taguchi A., et al. (2015). Pancreatic β cell enhancers regulate rhythmic transcription of genes controlling insulin secretion. Science 350, aac4250. doi:10.1126/science.aac4250
Petruzzelli M., Schweiger M., Schreiber R., Campos-Olivas R., Tsoli M., Allen J., et al. (2014). A switch from white to Brown fat increases energy expenditure in cancer-associated cachexia. Cell Metab. 20, 433–447. doi:10.1016/j.cmet.2014.06.011
Queiroz A. L., Dantas E., Ramsamooj S., Murthy A., Ahmed M., Zunica E. R. M., et al. (2022). Blocking ActRIIB and restoring appetite reverses cachexia and improves survival in mice with lung cancer. Nat. Commun. 13, 4633. doi:10.1038/s41467-022-32135-0
Roden M., Price T. B., Perseghin G., Petersen K. F., Rothman D. L., Cline G. W., et al. (1996). Mechanism of free fatty acid-induced insulin resistance in humans. J. Clin. Invest. 97, 2859–2865. doi:10.1172/JCI118742
Rosen E. D., Spiegelman B. M. (2006). Adipocytes as regulators of energy balance and glucose homeostasis. Nature 444, 847–853. doi:10.1038/nature05483
Rudic R. D., McNamara P., Curtis A.-M., Boston R. C., Panda S., Hogenesch J. B., et al. (2004). BMAL1 and CLOCK, two essential components of the circadian clock, are involved in glucose homeostasis. PLoS Biol. 2, e377. doi:10.1371/journal.pbio.0020377
Saltiel A. R., Kahn C. R. (2001). Insulin signalling and the regulation of glucose and lipid metabolism. Nature 414, 799–806. doi:10.1038/414799a
Seichter F., Vogt J., Tütüncü E., Hagemann L. T., Wachter U., Gröger M., et al. (2021). Metabolic monitoring via on-line analysis of 13 C-enriched carbon dioxide in exhaled mouse breath using substrate-integrated hollow waveguide infrared spectroscopy and luminescence sensing combined with Bayesian sampling. J. Breath. Res. 15, 026013. doi:10.1088/1752-7163/ab8dcd
Sellers K., Fox M. P., Bousamra M., Slone S. P., Higashi R. M., Miller D. M., et al. (2015). Pyruvate carboxylase is critical for non–small-cell lung cancer proliferation. J. Clin. Invest. 125, 687–698. doi:10.1172/JCI72873
Shi S., Ansari T. S., McGuinness O. P., Wasserman D. H., Johnson C. H. (2013). Circadian disruption leads to insulin resistance and obesity. Curr. Biol. 23, 372–381. doi:10.1016/j.cub.2013.01.048
Speakman J. (2013). Measuring energy metabolism in the mouse – theoretical, practical, and analytical considerations. Front. Physiol. 4, 34. doi:10.3389/fphys.2013.00034
Spinelli J. B., Yoon H., Ringel A. E., Jeanfavre S., Clish C. B., Haigis M. C. (2017). Metabolic recycling of ammonia via glutamate dehydrogenase supports breast cancer biomass. Science 358, 941–946. doi:10.1126/science.aam9305
Staal-van den Brekel A. J., Dentener M. A., Schols A. M., Buurman W. A., Wouters E. F. (1995). Increased resting energy expenditure and weight loss are related to a systemic inflammatory response in lung cancer patients. J. Clin. Oncol. 13, 2600–2605. doi:10.1200/JCO.1995.13.10.2600
Stenvers D. J., Scheer F. A. J. L., Schrauwen P., la Fleur S. E., Kalsbeek A. (2019). Circadian clocks and insulin resistance. Nat. Rev. Endocrinol. 15, 75–89. doi:10.1038/s41574-018-0122-1
Strassmann G., Fong M., Kenney J. S., Jacob C. O. (1992). Evidence for the involvement of interleukin 6 in experimental cancer cachexia. J. Clin. Invest. 89, 1681–1684. doi:10.1172/JCI115767
Takahashi J. S. (2017). Transcriptional architecture of the mammalian circadian clock. Nat. Rev. Genet. 18, 164–179. doi:10.1038/nrg.2016.150
Tschöp M. H., Speakman J. R., Arch J. R. S., Auwerx J., Brüning J. C., Chan L., et al. (2011). A guide to analysis of mouse energy metabolism. Nat. Methods 9, 57–63. doi:10.1038/nmeth.1806
Turek F. W., Joshu C., Kohsaka A., Lin E., Ivanova G., McDearmon E., et al. (2005). Obesity and metabolic syndrome in circadian Clock mutant mice. Science 308, 1043–1045. doi:10.1126/science.1108750
Ukropcova B., Sereda O., de Jonge L., Bogacka I., Nguyen T., Xie H., et al. (2007). Family history of diabetes links impaired substrate switching and reduced mitochondrial content in skeletal muscle. Diabetes 56, 720–727. doi:10.2337/db06-0521
van Hall G., Steensberg A., Sacchetti M., Fischer C., Keller C., Schjerling P., et al. (2003). Interleukin-6 stimulates lipolysis and fat oxidation in humans. J. Clin. Endocrinol. Metab. 88, 3005–3010. doi:10.1210/jc.2002-021687
Vander Heiden M. G., Cantley L. C., Thompson C. B. (2009). Understanding the Warburg effect: The metabolic requirements of cell proliferation. Science 324, 1029–1033. doi:10.1126/science.1160809
Vander Heiden M. G., DeBerardinis R. J. (2017). Understanding the intersections between metabolism and cancer biology. Cell 168, 657–669. doi:10.1016/j.cell.2016.12.039
Vaquero J. J., Kinahan P. (2015). Positron emission Tomography: Current challenges and opportunities for technological advances in clinical and preclinical imaging systems. Annu. Rev. Biomed. Eng. 17, 385–414. doi:10.1146/annurev-bioeng-071114-040723
Verlande A., Chun S. K., Goodson M. O., Fortin B. M., Bae H., Jang C., et al. (2021). Glucagon regulates the stability of REV-ERBα to modulate hepatic glucose production in a model of lung cancer-associated cachexia. Sci. Adv. 7, eabf3885. doi:10.1126/sciadv.abf3885
Verlande A., Masri S. (2019). Circadian clocks and cancer: Timekeeping governs cellular metabolism. Trends Endocrinol. Metab. 30, 445–458. doi:10.1016/j.tem.2019.05.001
Weidle U. H., Klostermann S., Eggle D., Krüger A. (2010). Interleukin 6/interleukin 6 receptor interaction and its role as a therapeutic target for treatment of cachexia and cancer. Cancer Genomics Proteomics 7, 287–302.
Keywords: tumor metabolism, diet-induced obesity (DIO), insulin resistance, glucose oxidation and detection, circadian clock
Citation: Verlande A, Chun SK, Song WA, Oettler D, Knot HJ and Masri S (2022) Exogenous detection of 13C-glucose metabolism in tumor and diet-induced obesity models. Front. Physiol. 13:1023614. doi: 10.3389/fphys.2022.1023614
Received: 20 August 2022; Accepted: 20 September 2022;
Published: 05 October 2022.
Edited by:
Joanna C. Chiu, University of California, Davis, United StatesReviewed by:
Carla V. Finkielstein, Virginia Tech, United StatesRobert Dallmann, University of Warwick, United Kingdom
Copyright © 2022 Verlande, Chun, Song, Oettler, Knot and Masri. This is an open-access article distributed under the terms of the Creative Commons Attribution License (CC BY). The use, distribution or reproduction in other forums is permitted, provided the original author(s) and the copyright owner(s) are credited and that the original publication in this journal is cited, in accordance with accepted academic practice. No use, distribution or reproduction is permitted which does not comply with these terms.
*Correspondence: Selma Masri, c21hc3JpQHVjaS5lZHU=
†These authors have contributed equally to this work