- 1Service de Médecine Interne, Diabète et Maladies Métaboliques de la Clinique Médicale B, Hôpitaux Universitaires de Strasbourg et Equipe EA 3072 “Mitochondrie, Stress Oxydant et Protection Musculaire,” Faculté de Médecine-Université de Strasbourg, Strasbourg, France
- 2Predimed Technology Society, Schiltigheim, France
- 3Faculté de Médecine-Université de Strasbourg, Service de Physiologie et d’Explorations Fonctionnelles, Hôpitaux Universitaires de Strasbourg et Equipe EA 3072 “Mitochondrie, Stress Oxydant et Protection Musculaire,” Strasbourg, France
- 4Centre d’Expertise des TIC pour l’Autonomie (CenTich) et Mutualité Française Anjou-Mayenne (MFAM)-Angers, Angers, France
- 5Laboratoire IRTES-SeT, Université de Technologie de Belfort-Montbéliard (UTBM), Belfort, France
Introduction: The coronavirus disease 2019 (COVID-19) pandemic has necessitated the use of new technologies and new processes to care for hospitalized patients, including diabetes patients. This was the basis for the “GER-e-TEC COVID study,” an experiment involving the use of the smart MyPrediTM e-platform to automatically detect the exacerbation of glycemic disorder risk in COVID-19 older diabetic patients.
Methods: The MyPrediTM platform is connected to a medical analysis system that receives physiological data from medical sensors in real time and analyzes this data to generate (when necessary) alerts. An experiment was conducted between December 14th, 2020 and February 25th, 2021 to test this alert system. During this time, the platform was used on COVID-19 patients being monitored in an internal medicine COVID-19 unit at the University Hospital of Strasbourg. The alerts were compiled and analyzed in terms of sensitivity, specificity, positive and negative predictive values with respect to clinical data.
Results: 10 older diabetic COVID-19 patients in total were monitored remotely, six of whom were male. The mean age of the patients was 84.1 years. The patients used the telemedicine solution for an average of 14.5 days. 142 alerts were emitted for the glycemic disorder risk indicating hyperglycemia, with an average of 20.3 alerts per patient and a standard deviation of 26.6. In our study, we did not note any hypoglycemia, so the system emitted any alerts. For the sensitivity of alerts emitted, the results were extremely satisfactory, and also in terms of positive and negative predictive values. In terms of survival analysis, the number of alerts and gender played no role in the length of the hospital stay, regardless of the reason for the hospitalization (COVID-19 management).
Conclusion: This work is a pilot study with preliminary results. To date, relatively few projects and trials in diabetic patients have been run within the “telemedicine 2.0” setting, particularly using AI, ICT and the Web 2.0 in the era of COVID-19 disease.
Introduction
Intensive glucose control has been shown to delay or prevent the development of micro- and macro-vascular complications related to diabetes (Andrès et al., 2018a).
In this context, telemedicine may be an effective approach to solve problems of education, compliance, and monitoring and provider access (Andrès et al., 2018a). Thus, telemedicine is likely to provide assistance and even optimize the management of these chronic conditions, in particular by avoiding certain emergencies and repeated hospitalizations, as demonstrated in the field of heart failure and diabetes through the use of remote monitoring or remote follow-up (Chaudhry et al., 2010; Anker et al., 2011; Andrès et al., 2018c; Seferovic et al., 2019; Zulfiqar et al., 2019).
Since the 2010–2015, more numerous mature telemedicine projects and studies have been developed in the setting of diabetes management, especially in the setting of telemonitoring like The Utah Remote Monitoring Project (Shane-McWhorter et al., 2014), or the DIABETe project which was developed to optimize home monitoring of diabetic patients via a telemonitoring 2.0 platform, situations with a risk of decompensation of diabetes and its cardiovascular complications (Andrès et al., 2018a). Over the last decade, the Internet has become increasingly popular and is now an important part of our daily life. The use of “Web 2.0” technologies in health/medicine care or in telemedicine is referred to as “Health 2.0” or “Medicine 2.0,” and “telemedicine 2.0.”
To date, relatively few projects and trials in diabetic patients have been run within the “telemedicine 2.0” setting, particularly using AI (Artificial Intelligence), ICT (Information and Communication Technologies) and the Web 2.0. The new generation telemedicine projects in diabetes (Telemonitoring and Health Counseling for Self-Management Support from Lindberg et al. (2017), TELESAGE (Charpentier et al., 2011), DIABETe) are often known as “telemedicine 2.0” projects, given that they all utilize new Information and Communication Technologies (ICT) and the Web (tools for the “e-Health 2.0”).
The COVID-19 epidemic, which has been raging in France since February 2020, is strongly impacting the Alsace region and patients with severe cases of COVID-19 need to be hospitalized.
These severe cases of COVID-19 are the reason for prolonged hospital stays and high morbidity and mortality rates. In addition, many complications can occur, directly related to the disease due to SARS-CoV-2 (secondary immunodepression and opportunistic infections, glycemic decompensation, chronic respiratory failure, chronic heart failure, stroke, chronic kidney failure, etc.).
The current understanding and the epidemiological evolution of the disease suggest that the number of chronic pathologies is increasing, and that therefore, it is essential to optimize the screening and management of the chronic complications of COVID-19.
The coronavirus disease 2019 (COVID-19) pandemic has necessitated the use of new technologies and new processes to care for hospitalized patients, including diabetes patients. Diabetes is strongly associated with a poor prognosis in COVID-19. Scrupulous blood sugar management is crucial, since the worse outcomes are closely associated with higher blood sugar levels in COVID-19 infection (Pranata et al., 2021).
Our team developed a remote monitoring platform designed to help prevent the deterioration of geriatric syndromes: GER-e-TEC project (Zulfiqar et al., 2020a, b).
The main goal of GER-e-TEC study is to evaluate the use of a remote monitoring platform as a means of structuring and standardizing the medical care of dependent elderly patients to prevent glycemic disorder risk. Below, we present an illustration of the use of the MyPrediTM platform for the glycemic remote monitoring in COVID-19 older diabetic patients.
Patients and Methods
Objective
The central objective of the present phase of the GER-e-TEC COVID pilot study is to evaluate the use of a remote monitoring platform, here the MyPrediTM platform (Zulfiqar et al., 2020a), and to experiment with this telemonitoring solution for diabetic elderly patients affected by the SARS-CoV-2 virus (COVID-19) to prevent glycemic disorder risk. Our project has been developed to optimize hospital monitoring of diabetic elderly patients affected by COVID-19, via a telemonitoring 2.0 platform.
The AI of the remote monitoring platform (MyPredi platform) automatically generates indicators of “health status” deterioration, i.e., “warning alerts” for any chronic disease worsening, particularly diabetes, its macrovascular complications and cardiovascular comorbidities (e.g., arterial hypertension, chronic heart failure).
Patients
This study took place during the 3rd wave of the epidemic in France, during the period extending between December 14th, 2020 and February 25th, 2021, conducted in the Department of Internal Medicine, Diabetes, and Metabolic Disorders at the University Hospital of Strasbourg (HUS, Strasbourg, France). Any diabetic patient over 65 years of age with COVID-19 infection admitted to the hospital or emergency room with one or more chronic diseases was eligible for the GER-e-TEC study. All patients with confirmed COVID-19 identified by positive results on reverse transcription polymerase chain reaction (RT-PCR) of nasopharyngeal swabs were included (SARS-CoV-2–Gene RdRp).
Patients with COVID-19-related infection who were monitored by the telemedicine solution for less than 48 h were excluded. Minors, pregnant women, patients who were unable to sign the eligibility and consent form, elderly patients in palliative care (i.e., patients in hospice with life-limiting diseases), and patients who refused to provide their consent were excluded from the study. If patients presented signs of severe dementia, the consent of their legal guardian was required.
Study Outline
During the experiment, the patients recorded their vital signs every day with the help of smart devices. This data was then sent directly to the intelligent platform to be processed and analyzed in the unit. The platform uses an algorithm to anticipate risk situations for diabetic patients.
During the experiment, the alerts were compiled in the order they were received. They were analyzed with regard to the clinical context at the time they were emitted, using the discharge letter and computer files (medical and nursing) of the patient in question. This analysis was performed retrospectively by two professionals involved in the present study in the unit but not in contact with the caregivers who cared for the patients on a daily basis. The alerts were classified as “pertinent” or “non-pertinent” i.e., whether or not they were associated with an action or intervention by the clinic.
For the glycemic disorder risk, the threshold for triggering an alert for hypoglycemia was set at 0.7 g/L; for hyperglycemia, the threshold was set at 2 g/L; this in accordance with current recommendations.
Experimental Protocol
The MyPrediTM solution (Zulfiqar et al., 2020a, b) (i.e., a tablet and connected sensors) was used to collect the patient’s physiological data three times per day (morning, noon, night) and whenever the nurses and doctors checked in on the patient (3 and 2 times per day, respectively). A number of physiological measurements were taken, including blood pressure, heart rate, weight, oxygen saturation, capillary blood glucose, temperature, physical activity, and sleep. Questionnaires were also used [daily stool frequency; Confusion Assessment Method (CAM) questionnaire; pain questionnaire; biological measurements and iatrogenesis questionnaire (Zulfiqar et al., 2020b)]. See Figure 1 the sensors.
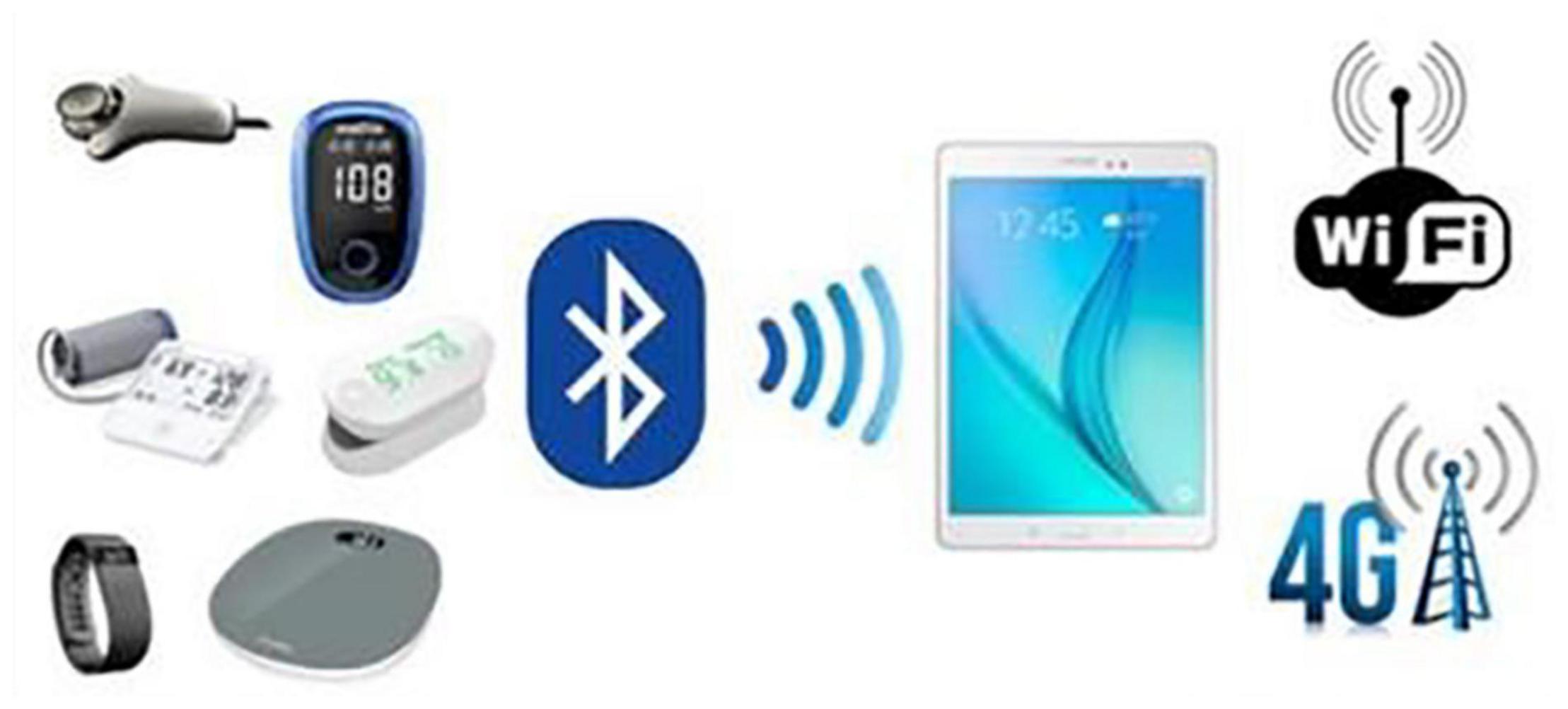
Figure 1. Ger-e-Tec’s connected non-intrusive medical sensors. Copyright: Predimed Technology, Schiltigheim, France.
The Remote Monitoring Platform
The MyPrediTM platform is a generic platform with an original architecture and proven capabilities to refer patients with chronic pathologies who require long-term management.
This remote monitoring platform is based on non-intrusive medical sensors, allowing the collection of capillary blood glucose, blood pressure, heart rate, arterial oxygen saturation (SaO2), temperature and body weight. These sensors communicate via Bluetooth, enabling real-time feedback of physiological information on the patient’s health status. The platform also includes a touch tablet, which communicates via Wi-Fi with a box, or via 3G/4G, enabling interaction with the patient and providing nutritional-hygienic and therapeutic education. The MyPrediTM system includes a server that hosts patient data and a secure Internet portal (website), allowing the patient and the various healthcare professionals to connect.
MyPrediTM is based on an “intelligent” system in the form of an inference engine and a medical ontology, enabling personalized data analysis, which is specific to each patient, in real time or delayed mode, with, ultimately, the generation of “alerts.” The MyPrediTM platform generates “indicators of deterioration in the patient’s health status,” known as “alerts,” in relation to a decompensation of chronic pathologies, in this case diabetes, with hyperglycemia and hypoglycemia alerts. This reasoning is based on an inference engine whose rules are created by medical experts (here, diabetologists, internists, geriatricians). The medical knowledge is derived from “evidence-based medicine.”
For the glycemic disorder risk, the threshold for triggering an alert for hypoglycemia was set at 0.7 g/L; for hyperglycemia, the threshold was set at 2 g/L; this in accordance with current recommendations.
For hypoglycemia, an alert was considered critical when capillary blood glucose was below 0.5 g/L; for hyperglycemia, an alert issued was considered mild when capillary blood glucose was between 2 and 2.5 g/l, moderate when capillary blood glucose was between 2.5 and 3 g/L and critical above 3 g/L.
Parameters Evaluated and Statistical Analyses
We calculated sensitivity, specificity, and positive and negative predictive value for alerts issued for the geriatric risk, in order to determine the clinical relevance of the alerts generated by the MyPrediTM remote monitoring platform for the glycemic disorder risk. Survival analyses were estimated using the Kaplan–Meier method. For the comparison of living and deceased elderly patients, we used the Student t-test and the Wilcoxon test.
We used RStudio software1 and R code (Boston, United States).
Administrative Requirements
All the COVID-19 older diabetic patients who participated in the GER-e-TEC project were required to sign a consent form. A clinical research protocol for the GER-e-TEC project was filed with the Ethics Committee of the Faculty of Medicine of Strasbourg under the number RNI 2020–HUS N°7792. We also obtained authorization to conduct the study from the Commission Nationale Informatique et Liberté (CNIL, “National Commission on Informatics and Liberty”).
Results
Characteristics of Patients
A total of 71 patients were hospitalized in the internal medicine unit between December 14th, 2020 and February 25th, 2021. Of these, 10 diabetic elderly patients affected by COVID-19 disease were monitored remotely, while there was no refusal.
The mean age of the patients was 84.1 years with a standard deviation of 6.9 years. The median age was 84.2 years. There were 6 (60%) male patients and 4 female patients: a male/female ratio of 1.5 to 1. The mean HbA1c was 7.52% (Anker et al., 2011; Charpentier et al., 2011; Shane-McWhorter et al., 2014; Lindberg et al., 2017; Zulfiqar et al., 2020a; Pranata et al., 2021).
The patients used the telemedicine solution for an average of 14.5 days with a standard deviation of 9.9 days.
Among the 10 diabetic patients, 7 (70%) had a history of hypertension, 4 (40%) had a history of dyslipidemia, and 4 (40%) had a history of cognitive disorders. See Table 1 for more information on the accompanying syndromes. The average number of drug treatments at the time of admission was 8.5 with a standard deviation of 4.7. See Table 1 for more information on the treatments. The mean Charlson score, that predicts the 1-year mortality, was 7.1 with a standard deviation of 1.
All of patients had positive result for SARS-CoV-2 confirmed by reverse transcriptase PCR (RT-PCR) tests on nasopharyngeal swabs (thereafter referred to as COVID-19 diabetic patients).
The average length of stay was 13.6 days (4–30).
Of the 10 diabetic COVID-19 patients, 7 (70%) lived at home and 3 (30%) lived in nursing homes. After hospitalization, 5 (50%) patients returned to their homes and 5 (50%) died during the experiment.
Data From the Sensors/Questionnaires
The MyPrediTM remote monitoring solution collected a total of 44,571 measurements while monitoring the geriatric syndromes of the entire patient group. On average, 4,457 measurements were recorded per patient for geriatric disorders and risks. On average, 312 measurements were recorded per patient per day.
Mean blood pressure was 100.75 mmHg with a standard deviation of 9.11. The mean heart rate was 81.2 bpm with a standard deviation of 12.8 bpm. Mean oxygen saturation was 93.8% with a standard deviation of 4.9. The mean blood glucose level was 176.5 mg/L, with a standard deviation of 87.4 mg/L.
Mean activity was 1,027.1 steps per day with a standard deviation of 1,508.6 steps per day. The mean daily activity index was 13.6%. Mean sleep per day was 493.2 min with a standard deviation of 139.5 min. Mean light sleep was 162.5 min with a standard deviation of 118.2 min. Mean deep sleep was 330.8 min with a standard deviation of 144.6 min. The mean VAS (visual analog scale) was 0.1. The mean EVS (simple verbal scale) was 0.0. The mean Algoplus was 1.5 with a standard deviation of 1.8. The mean weight was 76.7 kg with a standard deviation of 21.7 kg. The mean number of stools was 0.3 stools per day with a standard deviation of 0.6. The mean temperature was 36.5°C with a standard deviation of 0.9°C.
The mean albumin was 36.2 g/L, with a standard deviation of 4.6 g/L; the mean natremia was 140.9 mmol/L, with a standard deviation of 5.4 mmol/L; the mean kalemia was 4.2 mEq/L, with a standard deviation of 0.6 mEq/L, and the mean creatinine was 90.8 μmol/L, with a standard deviation of 28.6 μmol/L.
Number of Alerts for Diabetes Risk
During the monitoring, 142 alerts were emitted for the “glycemic disorder” risk indicating hyperglycemia, with an average of 20.3 alerts per patient and a standard deviation of 26.6; with 69 of these alerts classified as “mild,” 38 classified as “medium,” and 35 classified as “critical.”
In our pilot study, we did not note any hypoglycemia so the system emitted no alerts.
Clinical Relevance of Alerts
Table 2 illustrates the clinical relevance of the alerts in terms of Se, Spe, PPV, and NPV for the evaluated criteria. Note the sensitivity of 100% for the alerts and the high negative predictive value.
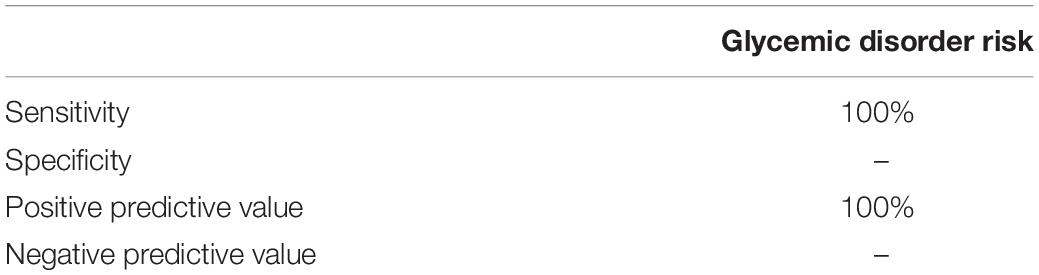
Table 2. Sensitivity (Se), specificity (Spe), positive and negative predictive values (PPV; NPV) for alerts from the MyPrediTM remote monitoring platform.
Survival Analyses
Survival analyses showed that gender played no role in the length of the hospital stay (a), regardless of the reason for the hospitalization (COVID-19 management). The analyses revealed that the length of the hospital stay was not affected also by the number of alerts in COVID-19 diabetic older patients (b). See results Figure 2.
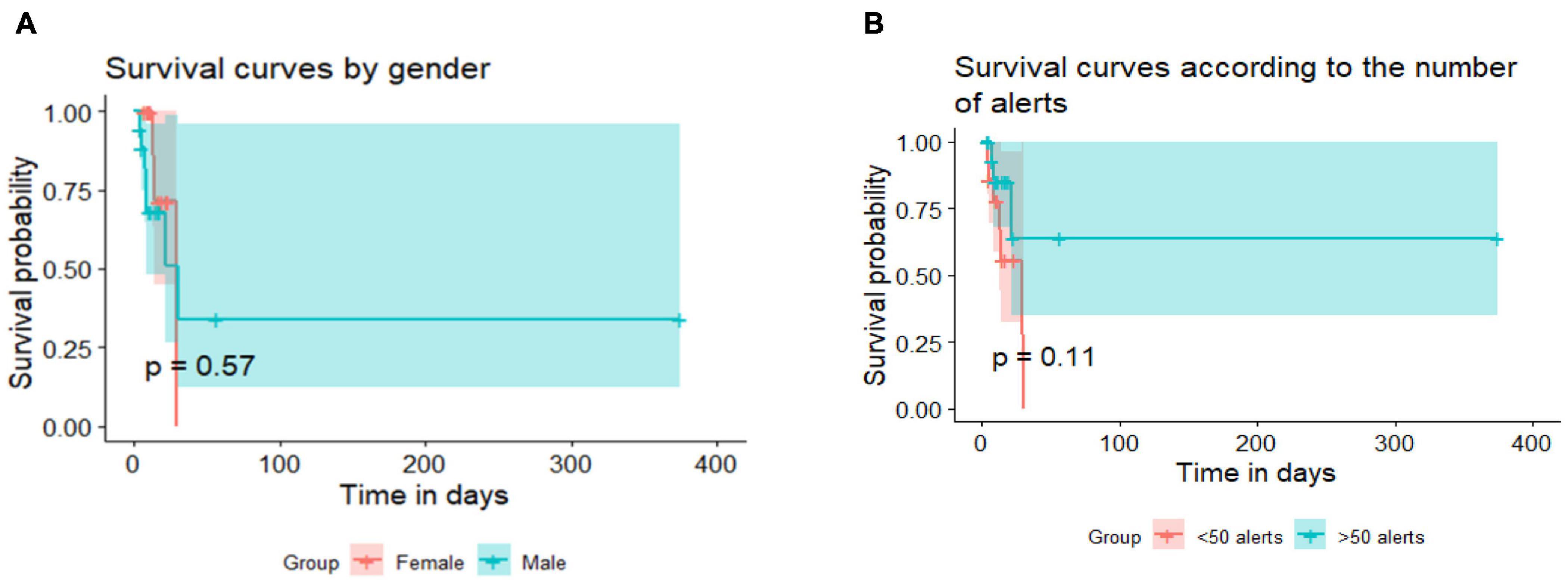
Figure 2. Survival analysis. (A) Survival curves by gender. (B) Survival curves by number of alerts. Copyright: Predimed Technology, Schiltigheim, France.
Clinical Relevance of Alerts for the Other Geriatric Risk
Table 3 indicates alerts and recommendations issued for the other geriatric risks studied in the 10 diabetic elderly COVID patients. Risks associated with and iatrogenesis (n = 175), decompensated heart failure (n = 126), and hypertension (n = 90) generated the most alerts.
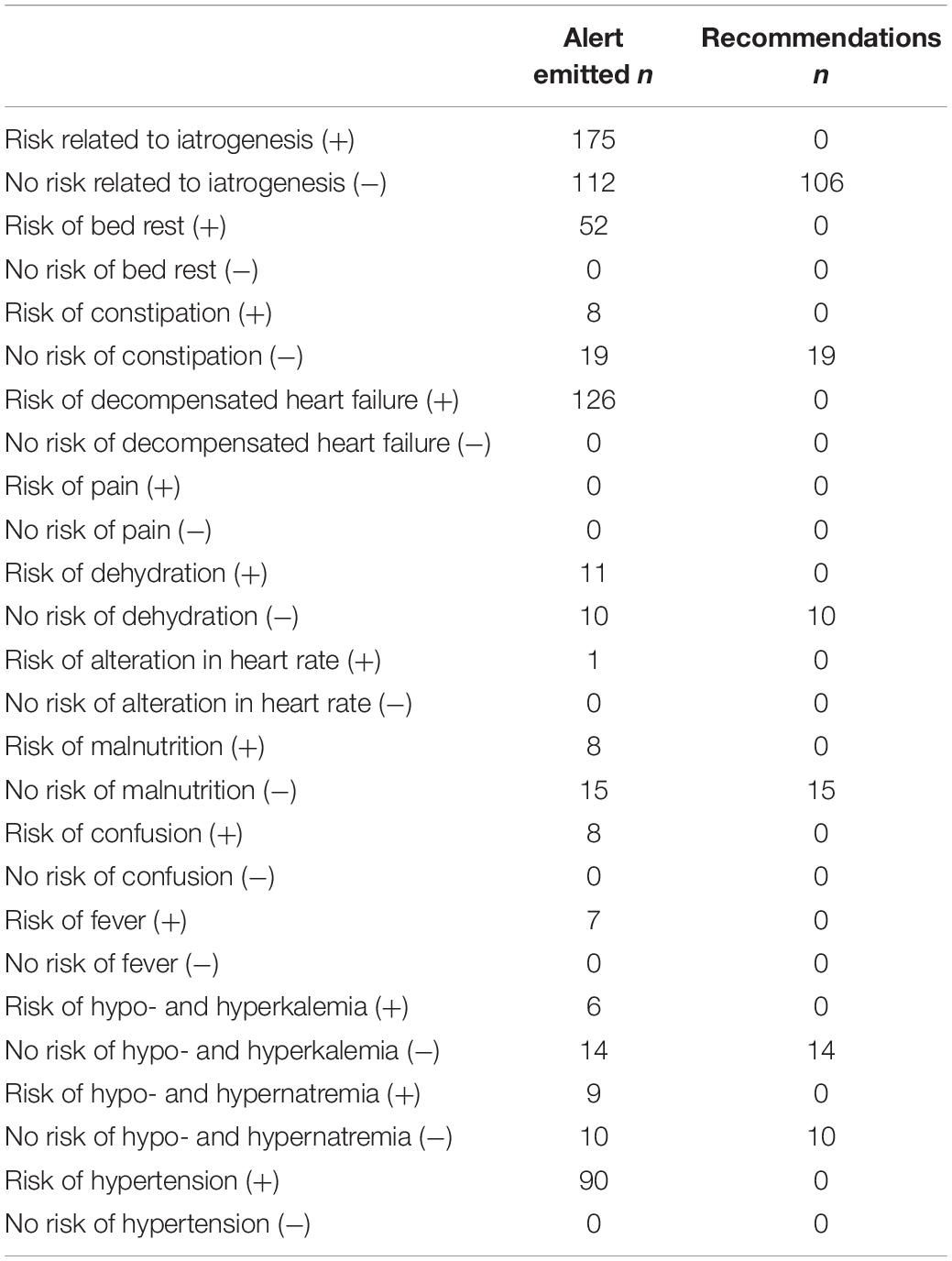
Table 3. Alerts and recommendations issued for the other geriatric risks studied in the 10 diabetic elderly COVID patients.
For all geriatric risks, there were an average of 17 alerts per patient; we had, on average, 4.6 alerts per day and per patient with a median of three alerts per day, per patient.
There were on average five recommendations per patient for all geriatric risks, with a standard deviation of 6.8. We had, on average, two recommendations per day, per patient and per geriatric risk.
Sensitivity, Specificity, Positive Predictive Values and Negative Predictive Values of Alerts for Other Geriatric Risks From the MyPredi Telemonitoring Platform for the 10 Elderly Diabetics Affected by COVID-19
Table 4 illustrates the clinical relevance of the alerts in terms of Se, Spe, PPV, and NPV for the other geriatric risk. Note the sensitivity of 100% for the alerts of all the other evaluated geriatric risks and the high negative predictive value.
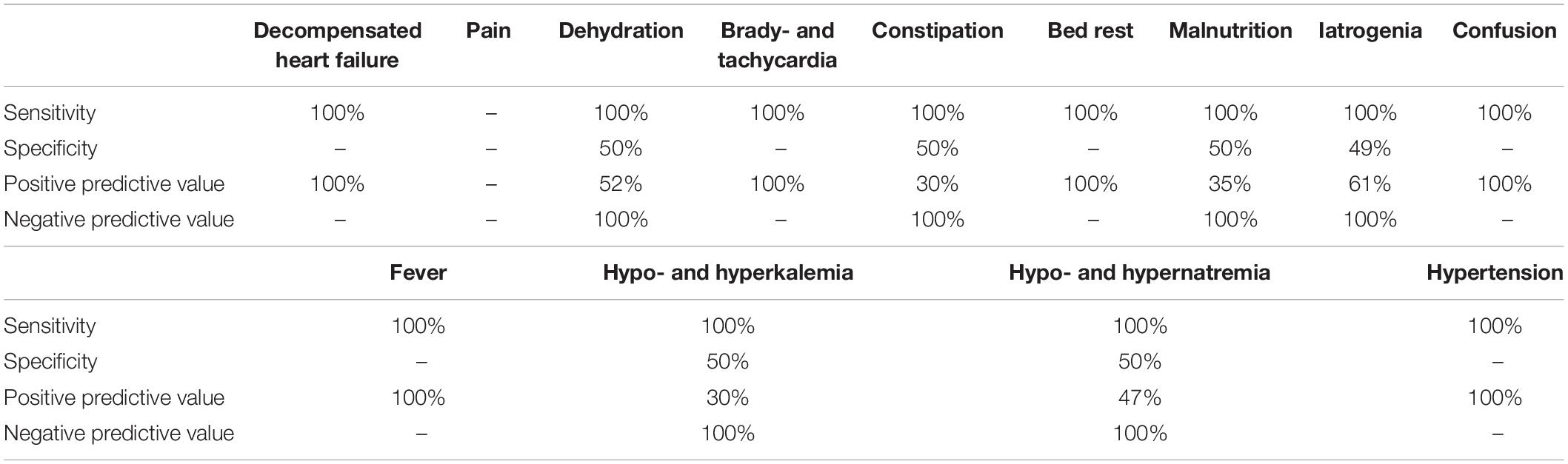
Table 4. Sensitivity, specificity, positive and negative predictive values for alerts from the MyPrediTM remote monitoring platform.
Elderly Diabetic Patients COVID-19 Alive vs Elderly Diabetic Patients COVID-19 Deceased
We present the results of the comparison of elderly patients who survived COVID-19 infection to the series of elderly patients who died from COVID-19 infection. We note heart rate, hyperglycemia, and the amount of deep sleep are significantly higher in elderly diabetics who died from COVID-19 infection. In addition, oxygen saturation, physical activity and the daily frequency of stools are significantly lower in elderly diabetics who died from infection linked to COVID-19.
See Table 5 for more results.
Discussion
Glucose monitoring plays a crucial role in glucose control, which has been shown to be significantly related to the mortality risk of the new coronavirus disease 2019 (COVID-19) (Zhu et al., 2020). Elders, especially those with diabetes, are at the highest risk of COVID-19 related adverse outcomes and mortality. The COVID-19 pandemic has necessitated the use of new technologies and new processes to care for hospitalized patients, including diabetes patients. In the literature, some projects developed the use of remote real-time continuous glucose monitoring (CGM) in hospital like Davis et al. (2021), in a COVID-19 intensive care unit with nine patients included.
In our pilot study, COVID-19 infection clearly impacted the experiment we conducted, five elderly diabetic people with COVID-19 died. In our pilot study beyond hemodynamic parameters, such as oxygen saturation being significantly lower in elderly people with COVID who died, and hyperglycemia being significantly higher in elderly people with COVID who died, we noted that physical activity measured by the pedometer and the frequency of stools were significantly lower in diabetic elderly people with COVID-19 infection who died.
Unlike our work, the vast majority of telemedicine projects concerning diabetes have concerned the management or care of diabetic subjects during the period of the pandemic. We did not find any work on the glycemic monitoring of diabetic subjects affected by COVID-19 on the main scientific search engines (PubMed, Google Scholar).
To our knowledge, this is the first experimentation carried out with elderly patients suffering from COVID and who have multiple pathologies, with a telemedicine solution enabling the upstream detection of situations likely to degenerate into hyperglycemia or hypoglycemia. To our knowledge, this is one of the first projects that uses AI in addition to ICT (Information and Communication Technologies), which also evaluates other geriatric risk like the physical activity, constipation, dehydration, confusion and iatrogenesis.
At this level, we are in the context of predictive medicine, which is also personalized in the context of the MyPrediTM platform, i.e., adapted to the phenotype of each older patient. On the other hand, it seems that this is the first time that such a communicative and “intelligent” system has been developed with the support of new technology tools, foreshadowing telemedicine 2.0 solutions.
All patients and health professionals used the MyPrediTM system without any problems during the entire experiment. Thus, in our experience, age does not appear to be a limiting factor in the adoption and use of new technologies. Several recent studies have pointed in the same direction, documenting the use of telemedicine solutions, including among octogenarians. The telemonitoring platform used in our pilot study was first validated in a monocentric study conducted in the same hospital, primarily focused on the problem of heart failure (Andrès et al., 2015, 2018b, 2019a, 2019b). One hundred and seventy-five patients (mean age of 72 years) were included, 30% of the patients suffered from type 2 diabetes. During this first study, 1,500 measurements were taken to generate 700 alerts in 68 patients. One hundred and seven subjects (61.1%) had no alerts upon follow-up. Analysis of the warning alerts in the 68 other patients showed that MyPrediTM detected any worsening of the “patient’s health,” with satisfactory results for sensitivity, and negative predictive values (Andrès et al., 2018b).
It should be emphasized that these telemedicine projects are perfectly in line with the “care pathway” theme currently being promoted by the French health authorities (Ministry of Health and Solidarity, Health Insurance, etc.) in the field of chronic diseases (heart failure, diabetes, COPD, and in the future, long-term COVID and its consequences). These projects undoubtedly contribute to the structuring of patient care pathways. In the field of chronic diseases, and in particular diabetes in the elderly, given the epidemiology of these diseases and the foreseeable shortage of available healthcare time, we need to improve monitoring and education, develop prevention and forecasting, and above all, improve how we select the patients who will need to use the healthcare system.
Nowadays, residents in nursing homes typically have multiple illnesses (cognitive and psycho-behavioral pathologies, undernutrition, heart failure, diabetes, COPD, kidney failure, etc.) and are taking multiple medications. On the medical level, this implies the need for regular monitoring and a high level of medical expertise, including multidisciplinary expertise, for healthcare teams. The GER-e-TECTM project aims to provide these complex patients with telemedicine tools that enable protocolized and personalized, non-intrusive monitoring. More specifically, the GER-e-TECTM project takes into account the prevalent issues of aging along with the main geriatric syndromes (falls, malnutrition, cognitive-behavioral disorders, iatropathogenesis, etc.) and does this in the highly specific context of COVID-19-related infection.
With the MyPrediTM telemedicine platform, we have demonstrated the value of remote monitoring in the management of elderly patients at the University Hospital of Strasbourg. Moreover, this intelligent platform has been tested in the context of other chronic pathologies through various institutional research projects such as the DIABETe project (Andrès et al., 2018b) (monitoring of patients with type II diabetes). Our work is all the more innovative because remote monitoring was performed on very elderly diabetic patients suffering from COVID-19 infection, which to our knowledge had never been done before, according to recent data in the literature.
As part of the GER-e-TEC project, MyPrediTM assists the healthcare staff by automating the processing of information gathered from sensors and questionnaires in order to provide early detection and warning in situations where there is a risk of acute decompensation from a medical point of view. MyPrediTM provides a set of recommendations, including operational assistance in emergency situations, and offers accessibility to all healthcare professionals, whether in the home or remotely.
Limitations
The main weakness of our work lies in the very limited number of elderly patients included for telemonitoring. Indeed, our sample is small (n = 10). This is explained by the realization of a study with a monocentric nature, this in an exceptional health environment linked to the COVID-19 epidemic wave.
Our work has other limitations, and we acknowledge that the remote monitoring system put in place can be improved. Indeed, the main limitation of our work lies in the number of alerts issued by our remote monitoring system for the 10 diabetic COVID-19 older patients included. It should be noted that the number of alerts issued for glycemic disorders remains extremely high. Since our remote monitoring solution should be easy to use on a daily basis, it will be necessary to make adjustments to ensure the system does not become overloaded. Smoothing is currently underway, in coordination with the scientific committee steering the study (geriatricians, diabetologists and the Predimed team managing the platform).
Conclusion
Further investigation of telemonitoring efficacy and cost- effectiveness over longer periods of time, and larger samples is needed.
This work is part of a larger work, consisting in the prevention of geriatric syndromes in COVID-19 older patients: GER-e-TEC COVID project.
GER-e-TEC COVID project is a unique and innovative project. In fact, to the best of our knowledge, it is the only remote monitoring platform designed to help prevent the deterioration of geriatric syndromes in the era of COVID-19. The main objective of the GER-e-TEC project is the study of the contribution of telemonitoring of dependent elderly patients with a structuring and a protocolization of their medical care, in order to avoid situations of acute decompensation and complications of geriatric risks in COVID-19 patients. The geriatric risks concerned are: falls, constipation, dehydration, confusion, iatrogenia, malnourishment, pain, sleep disorders, heart failure, diabetes, infections, and bedsores.
The results of this 2nd phase of the GER-e-TEC covid study will be communicated later.
Data Availability Statement
The original contributions presented in the study are included in the article/supplementary material, further inquiries can be directed to the corresponding author/s.
Ethics Statement
All the COVID-19 older diabetic patients who participated in the GER-e-TEC project were required to sign a consent form. A clinical research protocol for the GER-e-TEC project was filed with the Ethics Committee of the Faculty of Medicine of Strasbourg under the number RNI 2020–HUS N°7792. We also obtained authorization to conduct the study from the Commission Nationale Informatique et Liberté (CNIL, “National Commission on Informatics and Liberty”).
Author Contributions
A-AZ and EA: conceptualization. A-AZ, MH, and EA: methodology. DM, MH, and AH: software. A-AZ, EA, MH, JH, SE, and AH: validation, writing—review and editing, visualization, project administration, and funding acquisition. A-AZ, DM, MH, and EA: formal analysis. A-AZ: investigation. A-AZ, EA, MH, JH, SE, and AH: resources. A-AZ, DM, MH, and EA: data curation. A-AZ, EA, MH, and AH: writing—original draft preparation. A-AZ, EA, MH, BG, ST, JH, SE, and AH: supervision. All authors have read and agreed to the published version of the manuscript.
Conflict of Interest
MH is the CEO of predimed.
The remaining authors declare that the research was conducted in the absence of any commercial or financial relationships that could be construed as a potential conflict of interest.
Publisher’s Note
All claims expressed in this article are solely those of the authors and do not necessarily represent those of their affiliated organizations, or those of the publisher, the editors and the reviewers. Any product that may be evaluated in this article, or claim that may be made by its manufacturer, is not guaranteed or endorsed by the publisher.
Footnotes
References
Andrès, E., Hajjam, M., Talha, S., Meyer, L., Jeandidier, N., Hajjam, J., et al. (2018a). Télémédecine dans le domaine de l’insuffisance cardiaque. État des lieux et focus sur le projet de télémédecine 2.0 E-care. Perspectives dans le domaine de la diabétologie. Médecine des Maladies Métaboliques 12, 224–231.
Andrès, E., Talha, S., Zulfiqar, A. A., Hajjam, M., Ervé, S., Hajjam, J., et al. (2018c). Current researches and new perspectives of telemedicine in chronic heart failure: narrative review and points of interest for the clinician. J. Clin. Med. 7:544. doi: 10.3390/jcm7120544
Andrès, E., Talha, S., Hajjam, M., Hajjam, J., Ervé, S., and Hajjam, A. (2018b). Experimentation of 2.0 telemedicine in elderly patients with chronic heart failure: a study prospective in 175 patients. Eur. J. Intern. Med. 51, e11–e12.
Andrès, E., Meyer, L., Zulfiqar, A. A., Hajjam, M., Talha, S., Bahougne, T., et al. (2019a). Telemonitoring in diabetes: evolution of concepts and technologies, with a focus on results of the more recent studies. J. Med. Life 12, 203–214.
Andrès, E., Meyer, L., Zulfiqar, A. A., Hajjam, M., Talha, S., Ervé, S., et al. (2019b). Current research on telemonitoring in patients with diabetes mellitus: a short pragmatic narrative review. Trends Telemed. Ehealth 1, 1–11. doi: 10.31031/TTEH.2019.01.000513
Andrès, E., Talha, S., Benyahia, A. A., Keller, O., Hajjam, M., Moukadem, A., et al. (2015). e-Health: a promising solution for the optimized management of chronic diseases. Example of a national e-Health project E-care based on an e-platform in the context of chronic heart failure. Eur. Res. Telemed. 4, 87–94. doi: 10.1016/j.eurtel.2015.08.001
Anker, S. D., Koehler, F., and Abraham, W. T. (2011). Telemedicine and remote management of patients with heart failure. Lancet 378, 731–739. doi: 10.1016/s0140-6736(11)61229-4
Charpentier, G., Benhamou, P. Y., Dardari, D., Clergeot, A., Franc, S., Schaepelynck-Belicar, P., et al. (2011). The Diabeo software enabling individualized insulin dose adjustments combined with telemedicine support improves HbA1c in poorly controlled type 1 diabetic patients: a 6-month, randomized, open-label, parallel-group, multicenter trial (TeleDiab 1 Study). Diabetes Care 34, 533–539. doi: 10.2337/dc10-1259
Chaudhry, S. I., Mattera, J. A., Curtis, J. P., Spertus, J. A., Herrin, J., Lin, Z., et al. (2010). Telemonitoring in patients with heart failure. N. Engl. J. Med. 363, 2301–2309.
Davis, G. M., Faulds, E., Walker, T., Vigliotti, D., Rabinovich, M., Hester, J., et al. (2021). Remote continuous glucose monitoring with a computerized insulin infusion protocol for critically Ill patients in a COVID-19 medical ICU: proof of concept. Diabetes Care 44, 1055–1058. doi: 10.2337/dc20-2085
Lindberg, I., Torbjørnsen, A., Söderberg, S., and Ribu, L. (2017). Telemonitoring and health counseling for self-management support of patients with type 2 diabetes: a randomized controlled trial. JMIR Diabetes 2:e10. doi: 10.2196/diabetes.6884
Pranata, R., Henrina, J., Raffaello, W. M., Lawrensia, S., and Huang, I. (2021). Diabetes and COVID-19: the past, the present, and the future. Metabolism 121:154814. doi: 10.1016/j.metabol.2021.154814
Seferovic, P. M., Ponikowski, P., Anker, S. D., Bauersachs, J., Chioncel, O., Cleland, J. G. F., et al. (2019). Clinical practice update on heart failure 2019: pharmacotherapy, procedures, devices and patient management. An expert consensus meeting report of the Heart Failure Association of the European Society of Cardiology. Eur. J. Heart Fail. 1, 1169–1186. doi: 10.1002/ejhf.1531
Shane-McWhorter, L., Lenert, L., Petersen, M., Woolsey, S., McAdam-Marx, C., Coursey, J. M., et al. (2014). The Utah remote monitoring project: improving health care one patient at a time. Diabetes Technol Ther. 16, 653–660. doi: 10.1089/dia.2014.0045
Zhu, L., She, Z. G., Cheng, X., Qin, J. J., Zhang, X. J., Cai, J., et al. (2020). Association of blood glucose control and outcomes in patients with COVID-19 and pre-existing type 2 diabetes. Cell Metab. 31, 1068–1077.
Zulfiqar, A. A., El Hassani Hajjam, A., Talha, S., Hajjam, M., Hajjam, J., Ervé, S., et al. (2019). Les expérimentations de télémédecine en établissement d’hébergement pour personnes âgées dépendantes en France : revue de la littérature. Méd. Thér. 25, 107–113. doi: 10.1684/met.2019.0748
Zulfiqar, A. A., Hajjam, A., and Andrès, E. (2019). Focus on the different projects of telemedicine centered on the elderly in France. Curr. Aging Sci. 11, 202–215. doi: 10.2174/1874609812666190304115426
Zulfiqar, A. A., Lorenzo-Villalba, N., Zulfiqar, O.-A., Hajjam, M., Courbon, Q., Esteoulle, L., et al. (2020a). e-Health: a future solution for optimized management of elderly patients. GER-e-TECTM Project. Medicines 7:41. doi: 10.3390/medicines7080041
Keywords: diabetes risk, COVID-19, remote monitoring, MyPrediTM platform, GER-e-TEC study, prevention, elderly patients
Citation: Zulfiqar A-A, Massimbo DND, Hajjam M, Gény B, Talha S, Hajjam J, Ervé S, Hassani AHE and Andrès E (2021) Glycemic Disorder Risk Remote Monitoring Program in the COVID-19 Very Elderly Patients: Preliminary Results. Front. Physiol. 12:749731. doi: 10.3389/fphys.2021.749731
Received: 29 July 2021; Accepted: 05 October 2021;
Published: 27 October 2021.
Edited by:
Stefano Schena, The Johns Hopkins Hospital, Johns Hopkins Medicine, United StatesReviewed by:
Steve McKeever, Uppsala University, SwedenFernando Pasquini Santos, Federal University of Uberlândia, Brazil
Copyright © 2021 Zulfiqar, Massimbo, Hajjam, Gény, Talha, Hajjam, Ervé, Hassani and Andrès. This is an open-access article distributed under the terms of the Creative Commons Attribution License (CC BY). The use, distribution or reproduction in other forums is permitted, provided the original author(s) and the copyright owner(s) are credited and that the original publication in this journal is cited, in accordance with accepted academic practice. No use, distribution or reproduction is permitted which does not comply with these terms.
*Correspondence: Abrar-Ahmad Zulfiqar, abzulfiqar@gmail.com