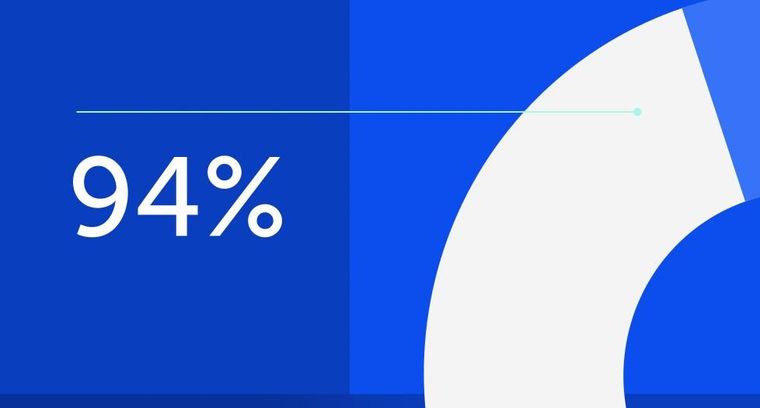
94% of researchers rate our articles as excellent or good
Learn more about the work of our research integrity team to safeguard the quality of each article we publish.
Find out more
REVIEW article
Front. Physiol., 18 November 2021
Sec. Computational Physiology and Medicine
Volume 12 - 2021 | https://doi.org/10.3389/fphys.2021.733868
This article is part of the Research TopicComputational Modeling for Liver Surgery and InterventionsView all 9 articles
Liver resection causes marked perfusion alterations in the liver remnant both on the organ scale (vascular anatomy) and on the microscale (sinusoidal blood flow on tissue level). These changes in perfusion affect hepatic functions via direct alterations in blood supply and drainage, followed by indirect changes of biomechanical tissue properties and cellular function. Changes in blood flow impose compression, tension and shear forces on the liver tissue. These forces are perceived by mechanosensors on parenchymal and non-parenchymal cells of the liver and regulate cell-cell and cell-matrix interactions as well as cellular signaling and metabolism. These interactions are key players in tissue growth and remodeling, a prerequisite to restore tissue function after PHx. Their dysregulation is associated with metabolic impairment of the liver eventually leading to liver failure, a serious post-hepatectomy complication with high morbidity and mortality. Though certain links are known, the overall functional change after liver surgery is not understood due to complex feedback loops, non-linearities, spatial heterogeneities and different time-scales of events. Computational modeling is a unique approach to gain a better understanding of complex biomedical systems. This approach allows (i) integration of heterogeneous data and knowledge on multiple scales into a consistent view of how perfusion is related to hepatic function; (ii) testing and generating hypotheses based on predictive models, which must be validated experimentally and clinically. In the long term, computational modeling will (iii) support surgical planning by predicting surgery-induced perfusion perturbations and their functional (metabolic) consequences; and thereby (iv) allow minimizing surgical risks for the individual patient. Here, we review the alterations of hepatic perfusion, biomechanical properties and function associated with hepatectomy. Specifically, we provide an overview over the clinical problem, preoperative diagnostics, functional imaging approaches, experimental approaches in animal models, mechanoperception in the liver and impact on cellular metabolism, omics approaches with a focus on transcriptomics, data integration and uncertainty analysis, and computational modeling on multiple scales. Finally, we provide a perspective on how multi-scale computational models, which couple perfusion changes to hepatic function, could become part of clinical workflows to predict and optimize patient outcome after complex liver surgery.
Liver resection, i.e., removal of part of the liver, is the most important procedure in liver surgery. In Germany, more than 20,000 liver resections are performed annually (Filmann et al., 2019). Due to demographic changes, the incidence of primary and secondary liver tumors increases as patients age. In parallel, the risk of liver surgery increases due to age-associated preexisting liver disease and other comorbidities that affect blood flow to the liver, such as cardiovascular disease.
Extended liver resection remains a high risk procedure, as potential postoperative hepatic dysfunction and eventual liver failure can lead to patient morbidity and even mortality. Removal of large parts of the liver not only poses a high regenerative challenge but also imposes a high metabolic load on the liver remnant (Ray et al., 2018). First, the loss of liver mass impairs the function of the remnant liver through portal hypertension (increase of pressure in the portal venous system) and hyperperfusion (increased perfusion). Both are unavoidable consequences of removing not only hepatic parenchyma but also the vascular bed. Second, extended liver resection compromises hepatic perfusion because of the mismatch between the two supplying portal veins and three draining hepatic veins. Transection of hepatic parenchyma inevitably leads to an impairment of either supply or drainage in the corresponding hepatic region. In addition, the surgical procedure itself carries functional risks (e.g., ischemia-reperfusion injury).
Current preoperative diagnostics allows a detailed anatomical and functional assessment of the liver. As part of the clinical routine, the location of the tumor to be resected is visualized in the context of the patient's vascular anatomy. In case of extended resection, hepatic hemodynamics, consisting of measurement of portal venous flow and pressure is assessed additionally. Furthermore, selected metabolic functions of the liver indicative of the overall function of the liver (e.g., LiMAx or indocyanine green ICG clearance) are usually quantified.
However, current preoperative diagnostics have distinct limitations. Despite high-quality imaging, precise determination of hepatic hemodynamics and sophisticated functional assays, the spatial resolution of specific hepatic functions is still rather low. Although it is known that liver perfusion and function are closely related (Takahashi et al., 2014), it is currently not possible to quantify this relationship, neither for the whole liver nor for a defined liver lobe. Changes in blood flow affect transport to and from regions of the liver (macroscale), in turn changing gradients of oxygen and nutrients in the lobulus and sinusoid (micro-scale), and thus directly impacting metabolic functions. Furthermore, changes in blood flow impose traction, tension and shear forces on liver tissue. Metabolic consequences of those mechanical forces cannot yet be determined, because the molecular links between perfusion and function are unknown. Although perfusion changes are likely sensed via mechanosensors that transmit mechanical forces into the cell, the link to hepatic metabolism is largely elusive.
The liver is the only parenchymal organ capable of near complete regeneration in response to tissue loss. Loss of liver mass by liver resection initiates liver regeneration and tissue remodeling, both necessary to restore tissue homeostasis and volume. Although the physiology and molecular mechanisms involved in liver regeneration have been studied for many years, prediction of the course and outcome of liver regeneration for individual patients is still not possible. The perfusion-associated mechanical forces are crucial for tissue regeneration and remodeling. Both, regeneration and remodeling, are ultimate prerequisites for restoring tissue homeostasis after partial hepatectomy. The molecular basis of functional changes after liver surgery is not well understood because of complex feedback loops, non-linearities, spatial heterogeneities, and different time-scales of events. This complexity requires novel approaches to relate surgically induced alterations in liver perfusion to hepatic metabolic functions. A better understanding of perfusion-function relationships hence is needed to improve preoperative diagnostic and risk assessment. This would allow us to identify patients who benefit most from surgery and those at increased risk for complications.
Systems medicine using multi-scale computational modeling is a unique approach to gain a better understanding of complex biomedical systems, such as the perfusion-function relationship after hepatectomy.
To improve patient-specific risk assessment in the context of liver surgery, computational modeling aims to (i) integrate heterogeneous data and knowledge at multiple scales about how perfusion connects to hepatic function, (ii) generate hypotheses based on integrated models (which need to be validated experimentally and related to clinical data), (iii) support surgical planning by predicting surgically induced perfusion perturbations and their functional (metabolic) consequences, and (iv) minimize surgical risk for the patient.
In this review, we will delineate the relationships between alterations in hepatic perfusion and their consequences for hepatic functions in the context of liver surgery, using hepatectomy as an example. First, we provide an overview of the current knowledge and available tools in clinical and experimental settings. Second, we will discuss how computational models and systems medicine approaches can contribute to a better understanding of the complex perfusion-function interactions. We end with a perspective on how such a systems medicine approach based on multiscale predictive models can be incorporated into the clinical decision-making process.
The term liver resection does not refer to a single surgical procedure, but comprises a wide spectrum of procedures that differ in their respective surgical strategy and technique. Two key surgical strategies are currently in use: Conventional (single-stage) hepatectomies and, for critical extended liver resections, multiple two-stage procedures.
Conventional liver resections involve the removal of one or more anatomically defined liver segments, defined as the hepatic territory supplied by the corresponding portal venous branch. Removal of liver segments requires transection of the hepatic parenchyma. Surgical techniques have been developed to minimize the tissue and vascular damage associated with transection in order to preserve the viability and perfusion of the adjacent liver tissue.
Two-stage hepatectomy is performed when the volume and expected function of the future liver remnant is considered too small to maintain vital metabolic functions for the patient. In the first step, the portal vein branches of the tumor-bearing liver lobe are occluded. Occlusion causes atrophy of the corresponding liver lobe. To compensate for this reduction in functional liver tissue, the volume of the non-ligated liver lobule increases substantially. Once compensatory hypertrophy of the future remnant liver is deemed sufficient to maintain the life-saving functions, the atrophied tumor bearing lobe is resected during the second step.
However, frequently the liver does not regenerate sufficiently because preexisting liver conditions such as steatosis, fibrosis or cholestasis impair the course of regeneration. Furthermore, simple portal vein occlusion without parenchymal transection often leads to a compensatory flow redistribution via existing porto-portal shunts, which reduces the efficacy of this strategy (Deal et al., 2018). To prevent collateral formation, a novel procedure called associating liver partition and portal vein ligation for staged hepatectomy (ALPPS) has been developed (Schnitzbauer et al., 2012). Here, portal vein occlusion is combined with transection of the hepatic parenchyma in the first step, followed by removal of the already mobilized and transected portally deprived liver lobe in the second step. However, in two-stage hepatectomy, the patient must undergo two major operations within a short time period of 7–10 days. Therefore, the indication for this complex procedure is taken with even greater caution.
Currently, there is no generally accepted standard for preoperative diagnostics prior to partial liver resection regarding liver anatomy, technical operability, liver volume and function.
The minimum requirements are defined in national guidelines. For Germany, the S3 guideline recommends ultrasound of the liver and multiphase contrast-enhanced computed tomography to assess technical operability and to evaluate the expected remnant liver volume and overall parenchymal quality. If there is doubt about the technical operability, more detailed imaging such as additional MRI with liver-specific contrast agent (Barth et al., 2016; Geisel et al., 2017; Wang et al., 2021b) is recommended. However, all contrast-enhanced techniques (CT, MRI and US) represent volume-based procedures and are limited in their predictive power of postoperative organ function.
In daily clinical routine, most centers rely on standard laboratory parameters covering different aspects of hepatic function to assess overall liver function. Liver enzyme release is taken as an indicator of hepatocellular injury, bilirubin as a marker of excretory function, and serum ChE, albumin and clotting factors as parameters of hepatic protein synthesis. However, this approach has some pitfalls. Although these parameters indicate the condition and main functions of the liver (injury, detoxification, protein synthesis), none of them is considered a reliable marker to quantify either functional hepatic reserve or liver dysfunction in critically ill patients (Nista et al., 2004; Bonfrate et al., 2015). Furthermore, these parameters provide only a static snapshot of liver function.
Currently, additional liver function tests are used in selected hepatobiliary centers prior to complex resections: global liver function assays such as ICG-clearance and the LiMAx-Assay as well as spatially resolved imaging technologies such as scintigraphy with radiolabeled tracers (e.g., mebrofenin-scintigraphy) and contrast-enhanced MRI. All four provide more detailed insight into liver function (metabolism and/or excretion) by reflecting the dynamic elimination of the test substance from the body.
ICG based liver function testing such as ICG-PDR and ICG-R15 is an established clinical tool for the assessment of liver function and perfusion. It is the most commonly used dynamic liver function test performed at bedside. After intravenous injection, ICG is selectively taken up by hepatocytes and excreted into bile. The test is performed using transcutaneous pulse-densitometry, a non-invasive fingertip method, and provides results within 6–8 min. ICG kinetics can be a reliable indicator in the context of liver surgery. ICG-clearance successfully predicted postoperative mortality in cirrhotic patients undergoing hepatic resection unlike other parameters (Hemming et al., 1992) and is a very good prognostic marker for liver failure after hepatectomy (Nonami et al., 1999). Preoperatively impaired ICG results are significantly associated with postoperative liver dysfunction and may predict poor outcome on postoperative day 1 (Haegele et al., 2016).
The LiMAx test is based on the indirect determination of CYP 1A2 activity in hepatocytes. After i.v. injection of 13C-methacetin, the CYP1A2 system metabolizes the substance into paracetamol and 13CO2. Using the spectral laser technique, the ratio of 13CO2/12CO2 can be determined via a breath test. The kinetics of 13CO2 appearance in the expired air thereby indicates the relative liver function (Rubin et al., 2017). The LiMAx test has been applied to predict postoperative outcome after hepatectomy (Stockmann et al., 2009). Post-hepatectomy liver failure and related mortality could be reduced after implementation of a preoperative LiMAx-based patient selection algorithm (Jara et al., 2015). Furthermore, LiMAx has been applied to follow restoration of functional capacity after partial liver resection (Lock et al., 2012; Bednarsch et al., 2016). The prediction of future liver remnant function via LiMAx highly correlated with future liver volume, and can thus be used to estimate postoperative morbidity (Blüthner et al., 2020).
Nuclear medicine scintigraphy imaging techniques exploit the specific properties of different tracers. HBS allows visualization of the specific HEF (Gupta et al., 2018). For this purpose, Technetium (99mTc) mebrofenin is administered intravenously before liver scintigraphy is performed. Mebrofenin is transported into hepatocytes via specific transporter proteins (OATP1B1 and OATP1B3) (Ghibellini et al., 2008) and excreted into the bile canaliculi by MDRP2 (Hendrikse et al., 2004). The areas in the liver where 99mTc mebrofenin accumulates (high uptake) is indicative of regional and global function. There is also potential to quantitatively assess liver function before and after surgical intervention (Rassam et al., 2019b; Uz et al., 2019) such as HEF, mebrofenin uptake rate or hepatic extraction rate (Gupta et al., 2018; Rassam et al., 2019a). The advantage of this method compared to ICG and LiMAx is the spatial resolution, albeit very coarse. Mebrofenin HBS has shown a strong correlation with 15 min ICG clearance (Erdogan et al., 2004). Mebrofenin HBS has been applied to evaluate liver function in hepatectomy (Bennink et al., 2004; Dinant et al., 2007; de Graaf et al., 2010) and showed a strong correlation between preoperative remnant liver function and the actual 1-day post-hepatectomy measurement (Bennink et al., 2004).
Other functional tracer-based imaging technologies used to assess liver function include SPECT and PET. SPECT is a nuclear imaging scanning methodology that integrates CT and a radioactive tracer such as sulfur colloid. Uptake of the tracer by the liver is an indicator of hepatic function. PET also estimates liver function based on the uptake and clearance of different radioactive positron-emitting tracers (e.g., 18F FDGal) (Bak-Fredslund et al., 2017; Keiding et al., 2018) and has been applied to predict postoperative liver function (Cho et al., 2017). Multi compartment models are employed to derive further tissue parameters, like hepatic arterial or portal blood flow, hepatic arterial or portal perfusion index or blood volume, from the dynamic time course of the contrast agent passing through the tissue (Wang et al., 2021a).
MRI is a non-ionizing imaging technique routinely used to detect hepatic tumors (Liu et al., 2017). More detailed analysis of the time course of the liver-specific contrast agent Gd-EOB-DTPA also allows to assess liver function by imaging its spatially resolved uptake and excretion into the bile by the hepatocytes (Wang et al., 2021b). Dynamic Gd-EOB-DTPA imaging has been applied to evaluate preoperative remnant liver function and post-hepatectomy outcome (Yoon et al., 2016; Itoh et al., 2017; Asenbaum et al., 2018; Chuang et al., 2018; Kim et al., 2018; Araki et al., 2020; Wang et al., 2021b).
Liver diseases not only affect hepatic function, but also lead to morphological changes, which in turn alter the mechanical properties of the tissue. Most diseases lead to increased stiffness of the tissue, e.g., liver fibrosis results in enhanced stiffness due to an increased ECM (Wells, 2005; Li et al., 2020b). Recently, hepatic elastography has gained attention, a medical imaging modality that relies on sound waves or forced tissue vibrations to measure tissue elastic properties and stiffness. It can be performed in combination with US or MRI. Correlations exist between liver elasticity and liver functional reserve, as demonstrated with ICG (Sugiura et al., 2019) or LiMAx (Heucke et al., 2019).
Clinically, a variety of US elastography methods have been developed. SWE and ARFI are the dominant methods in clinics today, offering integration with other advanced US imaging modalities (Ferraioli, 2019).
Alternatively, by using an external vibration generator, elastography can be performed with MRI. Current literature generally attributes higher diagnostic performance and fewer technical failures to MRI compared to US methods (Yin and Venkatesh, 2018). MRE also offers great potential for further development of new multiparametric methods to distinguish processes like inflammation, fibrosis, venous congestion and portal hypertension (Roldán-Alzate et al., 2015; Palaniyappan et al., 2016; Frydrychowicz et al., 2017; Yin et al., 2017; Leung et al., 2018), and has been successfully used to predict outcome after hepatectomy (Lee et al., 2017; Sato et al., 2018) and regeneration capacity (Jang et al., 2017). In the evaluation of NAFLD, MRI has the added advantage of providing an independent method for fat quantification (Zhang et al., 2018).
A clinically frequently observed pathological liver condition that affects liver perfusion, function, and recovery is hepatic steatosis. Hepatic steatosis assessed by routine preoperative MRI has been shown to be an independent risk factor of severe postoperative complications after major liver resection (d'Assignies et al., 2016).
While US, CT, and MRI can be used to assess hepatic steatosis in vivo, PDFF determination with MRI is currently the most accurate imaging method for quantification (Zhang et al., 2018; Troelstra et al., 2021).
Preoperative assessment of hemodynamics and perfusion relies mainly on noninvasive technologies, whereas intraoperative assessment is also performed with direct invasive techniques. The two main noninvasive technologies, US and MRI, can quantify blood flow in the major supplying and draining vessels of the liver (Yzet et al., 2010; Chouhan et al., 2017). Doppler US typically provides localized, dynamic flow measurements.
Similar to water-fat quantification, the evaluation and quantification of tissue perfusion with MRI has a long history (Rinck et al., 1984; Rosen et al., 1990). Perfusion is defined here as blood delivery at the capillary level. Over the years, two main approaches to perfusion MRI have been developed. The first uses an exogenous contrast agent (Jahng et al., 2014) and includes DSC-MRI and DCE-MRI (Leporq et al., 2018; Weiss et al., 2019). DCE-MRI techniques allow quantitative characterization of parenchymal and (lesion) microcirculatory changes (Thng et al., 2010) and the study of liver damage (Byk et al., 2016; Lu et al., 2017). The assessment and derivation of the tissue parameters, like the perfusion and uptake of contrast agent as correlate of liver function, is facilitated by multi compartment models fitted to the dynamic time course of the MRI data (Simeth et al., 2018).
The second approach refers to ASL (Williams et al., 1992), in which magnetically labeled blood itself is used as an endogenous tracer and its tissue accumulation is measured (Johnson et al., 2016). By using different, carefully placed labeling planes, arterial and portal perfusion can be assessed separately (Martirosian et al., 2019).
Invasive assessment of hepatic hemodynamics involves direct measurement of portal and hepatic arterial flow rates using US Doppler technology. Determination of portal pressure requires placement of a pressure sensor in the vessel of interest. Another valuable parameter is the HVPG, which is usually measured by inserting a balloon catheter into a branch of the hepatic vein via the jugular vein. HVPG has been applied in the context of hepatectomy showing an association of outcome with preoperative HVPG and a cutoff of HVPG < 10 mmHg was proposed (Boleslawski et al., 2012; Cucchetti et al., 2016). Although progress has been made in the noninvasive assessment of portal hypertension (Gouya et al., 2016) it remains a clinical challenge (Wan et al., 2021).
DWI is an MRI technique that is sensitive to the mobility of water molecules in tissue and therefore can provide insight into local tissue changes. Additionally to the actual self-diffusion of water, the perfusion of tissue causes IVIM in the capillary system of the tissue. These two diffusion effects can be separated with DWI measurement using multiple, differently weighted DWI scans (Mürtz et al., 2018; Fujimoto et al., 2021). Sheng et al. demonstrated that DWI can detect and distinguish microstructural tissue changes during ALPPS and PVL procedures (Sheng et al., 2018). DWI has been applied to assess hepatic ischemia and reperfusion injury (Lu et al., 2017; Yang et al., 2021) and to predict survival after partial hepatectomy (Muhi et al., 2013).
In summary, the selection of a particular procedure for an individual patient is based on the results of the extensive preoperative assessment. The diagnostic strategy in preoperative assessment is tailored to the needs of the patient and follows the standards of the particular center. Current approaches provide a detailed, spatially resolved, albeit indirectly measured assessment of liver function. However, the improved imaging modalities are standing alone and individual tissue parameters are difficult to interpret. The integration of different tissue parameters in descriptive models has been realized (Wang et al., 2016) but currently spatially resolved tissue parameters are not incorporated into complex multiscale predictive models of the liver.
A main limitation for clinical research is the availability of tissue-based data. Human liver tissues can be obtained during surgical procedures and by liver biopsy. For ethical reasons, patients cannot be subjected to repeated liver biopsies pre- and postoperatively. Therefore, animal experiments are important to better understand the pathophysiological mechanisms and processes governing liver surgery and liver regeneration (see Figure 1).
Figure 1. Surgical procedures. (A) Open situs with liver (encircled in orange). (B) 70% portal vein ligation. Note the slightly darker color of the ligated median and left lateral lobe (encircled in gray) compared to the fresh color of the right and upper caudate lobe (encircled in orange) (C) 70% partial hepatectomy. Note the stump (encircled in white) above the right lobes (encircled in orange). (D) Combined 20% partial hepatectomy with 70% portal vein ligation: Note the dark color of the portally ligated median and left lateral lobe (encircled in gray), the fresh red color of the upper caudate lobe (encircled in orange) and the stumps from the right lobes (encircled in white). LLL left lateral lobe, ML median lobe, RL right lobe, Cl upper caudate lobe, RK right kidney, LLL, ML, and RL stumps of the respective lobe.
Experimental liver resection in small animals was first performed by Higgins (1931). Originally, 70% of the liver mass was removed after mass ligation of the wide stump of the median and left lateral lobe of the rodent liver, resulting in impaired hepatic outflow and congestion of the remnant liver. With the refinement of surgical techniques (see Table 1), the parenchyma-preserving vessel-oriented technique was established (Madrahimov et al., 2006). Avoidance of congestion and necrosis of the stumps allowed rat survival even after extended 90% resection, which is lethal when using the mass ligation technique. In contrast, additional ligation of the portal vessel reduces the functional remnant liver mass and prevents survival after 90% PHx.
Table 1. Overview of different liver resection techniques, differing in how the vascular structures (portal triad and hepatic vein) are ligated respectively transected.
A bit earlier, in 1920, the first experimental PVL was performed in rabbits by Rous and Larimore (1920). Comparative studies revealed that the time course of liver regeneration after partial resection or PVL followed different kinetics. After simple hepatectomy, hepatocyte proliferation peaks on day 1 in rats and on day 2 in mice (see Table 1) and declines rapidly thereafter. Within a week, the original liver mass is restored.
In both models, the regenerating liver lobes are hyperperfused. However, in the case of PVL, the regenerative requirement is initially much lower. Resection results in immediate loss of function because a substantial amount of liver tissue is removed. In contrast, PVL only compromises function, as the portally deprived lobe is still perfused with arterial blood and thus can contribute to the overall liver function. Therefore, hepatocyte proliferation after PVL in rats peaks later, on postoperative day 2, but lasts for several days (Rozga et al., 1986; García-Pérez et al., 2015). Along with the development of hepatic atrophy, the regenerative need increases, resulting in a reduced but prolonged regenerative response (see Table 2).
Several combined procedures were introduced to better understand regulation of liver regeneration. Sequential procedures include repeated hepatectomy and 2-stage PVL to elucidate the proliferative capacity of the regenerating liver (Saito et al., 2006; Sugimoto et al., 2009). The impact of obstructive jaundice on liver regeneration was studied by first performing bile duct ligation 1 week prior to liver resection (Li 2014). Different models of 2-stage hepatectomy (e.g., 70% PVL with (ALPPS) or without transection of the median lobe followed by PHx of atrophied liver lobes) were developed to better assess the impact of preventing collateral formation (García-Pérez et al., 2015; Wei et al., 2020). Here actually, the development of animal model only happened after introducing the procedure into clinic (Schnitzbauer et al., 2012).
Liver resections and portal occlusions have also been combined with other interventions to better understand factors affecting hepatocyte proliferation and liver regeneration. These include interventions affecting hepatic perfusion such as right median hepatic vein ligation (Dirsch et al., 2008a; Huang et al., 2014). To better understand the impact of additional damage to the portally ligated lobe, bile duct ligation was performed resulting in increased regeneration of the FLR. A similar effect on regeneration of the FLR was observed when inducing congestion of the portally ligated lobe by performing an additional ligation of the left lateral hepatic vein along with PVL. Combination of an additional resection with PVL induced hepatocyte proliferation in the portally deprived liver lobe (Wei et al., 2020). In conclusion, the wide spectrum of surgical models, which emerged over the years, is very useful to investigate the different facets of liver regeneration and the underlying complex pathophysiological mechanism (see Table 2).
In the past, animal models of liver resection were mainly used to study the molecular mechanism underlying the course of liver regeneration (see Table 3). Many experimental studies have focused on investigating single molecular pathways governing central processes involved in regeneration, such as proliferation, inflammation, angiogenesis (vessel formation process), and recently also autophagy (cell survival process), through classical interventional studies. Specific blocking and reintroduction of selected molecules was performed to elucidate their relevance in liver regeneration.
Metabolic pathways regulating energy homeostasis are central to liver regeneration. Their role has been studied in liver resection, particularly in knockout models (see Table 4). For example, lack of sirtuin and PPARβ reduced energy metabolism and inhibited regeneration (Liu et al., 2013, 2019). In contrast, lack of PTEN and aldolase reductase increased energy metabolism and induced regeneration (Kachaylo et al., 2017; Li et al., 2020a).
Few studies addressed the investigation of hepatic hemodynamics and microcirculation in small animals subjected to various hepatobiliary procedures (see Table 5). They are mainly descriptive in nature. This may be partly due to the technical difficulties in assessing hepatic hemodynamics and microcirculation in such models. Hepatic hemodynamics can be assessed in rats using standard equipment (fluid filled catheters and ultrasound flow probes). The same procedure is also feasible in mice (Xie et al., 2016), but is more challenging due to their small size. Both portal pressure and portal venous blood flow per liver weight in small animals are comparable to that in humans. Resection or portal vein occlusion induce portal hypertension, hepatic hyperperfusion, and in humans, FOO. Some experimental studies focused on describing and modulating resection-induced impairment of hepatic hemodynamics. Fewer studies aimed for interfering by surgical or pharmacological interventions (e.g., splenectomy, drug treatment). Good examples are the reports by Huang et al. (2014) and Arlt et al. (2017), both of whom used a rat model of 70% PHx combined with right median hepatic vein ligation. This combined procedure mimics resection-associated FOO due to hepatic vein transection. Dahmen's group explored the impact of different interventions (e.g., application of vasoactive drugs like Molsidomine, L-NAME) on the formation of sinusoidal vascular canals during the spontaneous recovery process from FOO (Arlt et al., 2017).
Table 5. Rodent (liver resection) models impacting on hepatic perfusion (in the context of liver surgery).
Two different technologies are commonly used to assess hepatic microcirculation: intravital microscopy with fluorescent dyes and dark-field microscopy. Intravital microscopy is frequently performed in animal experiments. It allows the assessment of fluidic flow based on the injection of fluorescent albumin, but also intravascular labeled blood cells as well as the migration of blood derived cells into the hepatic parenchyma. However, because of the required injection of fluorescently labeled molecules or cells, it is not used clinically. Dark-field microscopy also allows quantification of blood flow velocity (Dahmen et al., 2007) and has occasionally been applied in clinical studies (Puhl et al., 2003).
As early as 1981, Lautt (1981) described a seminal phenomenon, the hepatic arterial buffer response. He was the first to understand that maintenance of hepatic flow is of paramount importance to maintain homeostasis (Lautt, 2007). Since the liver cannot directly control portal venous blood flow, there are a number of interrelated mechanisms to compensate for changes in portal blood flow that occur after PHx. PHx results in decreased portal blood flow, which in turn results in reduced (1) intrahepatic distending pressure. This causes the highly compliant hepatic vasculature to passively expel half of its blood volume, thereby increasing venous return, cardiac output, and HBF. The reduction in portal blood flow also causes (2) activation of the hepatic arterial buffer response and (3) an HBF-dependent hepatorenal reflex (Eipel et al., 2010). Adenosine is constantly released into the Mall space, a small fluid-filled space surrounding the terminal branches of the hepatic arterioles, portal venules and sensory nerves. Adenosine concentration is regulated by washout into the portal venules. In case of reduced portal flow, the washout is also reduced and adenosine may accumulate. This in turn leads to dilatation of the hepatic artery, thereby compensating for the reduced portal flow.
Elevated levels of adenosine activate the surrounding sensory nerves, which in turn triggers a reflex causing fluid retention in the kidney, also increasing the total blood volume, thereby maintaining cardiac output and PF. When hepatic blood flow cannot be maintained, other mechanisms, such as a shear stress/nitric oxide-dependent mechanism, are activated and trigger hepatocyte proliferation or apoptosis to achieve a better match between total liver mass and blood supply (Vollmar and Menger, 2009). These mechanisms are unique to the liver and its vascular bed and demonstrate how a major homeostatic organ is subject to multiple integrative regulatory mechanisms. However, to date, few efforts have been made to understand the molecular mechanism of blood flow-induced mechanical forces underlying the regulation of liver function and regeneration.
In summary, a variety of experimental approaches have been developed to investigate the regulatory processes involved in liver regeneration after partial hepatectomy. However, few studies have been designed for a comprehensive evaluation of the interplay between physical challenges, perfusion changes, their recognition by mechanoperception and the impact on regeneration and metabolism.
Liver regeneration to restore tissue loss after surgical removal or toxic insult occurs through proliferation of both parenchymal and non-parenchymal cells of the liver. In loss due to PHx, proliferation of hepatic cells is distributed throughout the parenchyma, and the factors that trigger and maintain liver cell proliferation during regeneration have been described (Michalopoulos, 2017). However, the primary trigger sensing the parenchymal loss remains largely elusive.
Hepatectomy markedly changes blood flow in the remnant liver featuring, e.g., portal hypertension and arterial hypoperfusion. Experimental evidence suggests that flow changes may regulate regeneration. For example, in pigs, increases in total hepatic flow after PHx preceded the increase in liver regeneration. Changes in hepatic flow correlated with the extent of liver mass loss, resulting in a 2-3-fold increase in hepatic perfusion and a 10–30% increase in portal pressure, suggesting a quantitative relationship (Kahn et al., 1984; Dahmen et al., 2007).
Surgically induced increase in portal blood flow appears to be critical for regeneration. In dogs, liver regeneration was hampered when a portocaval shunt, reducing portal blood flow, was added to hepatectomy (Mann et al., 1931). Patients with poor clinical outcome experienced a significant decrease in portal flow during extensive hepatectomy, suggesting that an adequate increase in portal blood flow is essential for hepatic regeneration (Kawasaki et al., 1991). Similarly, post-hepatectomy outcome improved in patients featuring higher portal flow postoperatively. Functional improvements like bilirubin levels and hepatic growth rate correlated with mean portal flow velocity (Kin et al., 1994; Hou et al., 2018). In humans, portal blood flow was inhomogeneously distributed in the remnant portal branches after PHx, as was the distribution of hepatocyte proliferation, suggesting a causal relationship between heterogeneous distribution of portal blood flow and regeneration (Iimuro et al., 2013).
Yet, there is some evidence against portal blood flow being the only regulator of liver regeneration. Minor (10–30%) removal of liver mass results in only a marginal regenerative response, suggesting that a threshold change in portal blood flow is required to initiate appropriate compensatory growth (Abshagen et al., 2012). Moreover, liver regeneration may occur even in the absence of portal blood flow. Despite ligation of the portal branch, a moderate proliferative response was observed in the corresponding ligated liver lobe, especially after an additional liver resection (Weinbren, 1955).
Although portal hyperperfusion might not be indispensable, the importance of flow-related mechanical forces has been repeatedly demonstrated. Mechanical inflictions induced by flow changes may play a major role in both the initiation and the termination of liver regeneration (Song et al., 2017). This is corroborated by the inverse quantitative correlations between the increase in portal blood flow and the remnant liver volume, which is accompanied by the increase in hepatic shear stress stimulating liver mass restoration. Likely, the increase of the blood flow-to-liver mass-ratio immediately after PHx and the resulting increased intrahepatic shear stress stimulate and regulate liver regeneration (Niiya et al., 1999; Sato et al., 1999; Schoen et al., 2001; Nobuoka et al., 2006). Conversely, reduction of shear stress in the liver by portocaval shunts resulted in liver atrophy (Sato et al., 1997; Abshagen et al., 2012).
Changes in blood flow exert forces on liver tissue. Because hepatic sinusoids are likely the first to sense changes in hepatic flow, the sinusoidal endothelium may play a major role in transducing these forces (Shu et al., 2021). This is supported by the hierarchical topology of the hepatic sinusoids involving cell-cell and cell-matrix interactions. Hepatocytes communicate directly with the sinusoids via the ECM. The ECM is connecting the extraluminal side of endothelial cells and the sinusoidal face of hepatocytes, thereby bridging the space of Disse. Indirect communication connects cells in the space of Disse, like HSC via the ECM to endothelial cells, and in turn hepatic stellate cells to hepatocytes. Direct cell-cell contacts between adjacent hepatocytes maintain epithelial hepatocyte-to- hepatocyte communication (Kang, 2020).
Cellular adhesion molecules, which transmit mechanical forces into cells, mediate cellular contacts to the ECM or to neighboring cells. In focal adhesion contacts, integrins connect cells and the ECM and serve as receptors for components of the ECM like fibronectin and collagens. Mechanical challenges of the ECM induce conformational changes in the integrin chains, followed by integrin clustering and intracellular activation of signaling pathways that include activation of, e.g., FAK, phospholipase C, and PI3K and others (Alexius, 1991). Besides transmission of mechanical forces acting on the ECM, integrins transmit intrinsic properties of the ECM to anchored cells. In the healthy liver, quiescent HSC and sinusoidal endothelial cells create a homeostatic ECM of relatively low stiffness in the space of Disse, which is necessary for hepatocyte function linked to normal hepatocyte polarity (Müsch, 2014). In the fibrotic liver, activated stellate cells produce ECM featuring augmented stiffness, which affects hepatocyte polarity and function. TGFβ, the major mediator of liver fibrosis, is activated by release from its latent integrin-associated form, thus responding to any conformational change of the ECM, either triggered by mechanical challenges or by changes of the ECM composition and stiffness (Hintermann and Christen, 2019).
The major cell adhesion molecules include E- and N-cadherin. In the rodent liver, E-cadherin is expressed in periportal areas, whereas N-cadherin is expressed throughout the parenchyma (see Figure 2). By homodimeric binding of the extracellular domains of cadherins on adjacent cells, they form adherens junctions that link the junction complex to the cytoskeleton by connecting the intracellular domains of the cadherins via p120, β-catenin and α-catenin to actin. Thus, physical forces, as likewise induced by sinusoidal flow changes, affect cellular behavior in terms of proliferation, differentiation and tissue homeostasis (Buckley et al., 2014); (KEGG pathway entry: hsa04520). Further, changes in mechanical forces outside the cell are transmitted into the cell by the tight junction complex comprising occludins, claudins and JAM. These can couple to the actin filament system via interactions with ZO. The tight junction complex can activate intracellular signaling pathways via PKC, PI3K and others that affect cell polarity, differentiation, and paracellular transport (Chiba et al., 2008); (KEGG pathway entry: hsa04530).
Figure 2. Zonal expression of E-cadherin (red, left panels)and of N-cadherin (green, right panels) in mouse liver sections. The exclusive periportal expression of E-cadherin demarcates the boundary between periportal and perivenous hepatocytes in the liver lobule (solid arrowheads). In contrast, N-cadherin is expressed all over the hepatic parenchyma (open arrowheads). Also, the tight junction protein ZO-1 (green, left panels; red, right panels) designating the bile canaliculi (white arrows) is expressed pan-parenchymally. Lower panels are digital magnifications of images shown in the upper panels. pp, periportal; pv, perivenous.
Similar to the zonal expression of E-cadherin, metabolic functions are expressed heterogeneously in the hepatic parenchyma. For example periportal hepatocytes surrounding the branches of the portal vein are specialized in producing glucose by gluconeogenesis, whereas perivenous hepatocytes surrounding the branches of the hepatic vein utilize glucose by glycolysis. The zonal pattern of metabolic functions is dynamic and regulated by hormone, substrate and oxygen gradients forming in the blood during passage through the sinusoids (Kietzmann, 2017). These may change by variations in the nutritional state of the organism, by physical activity and in liver diseases with functional consequences for the whole organism (Jungermann and Kietzmann, 2000; Steinman et al., 2021). On the molecular level, hepatic zonation is regulated, besides others, by morphogenic pathways including the Wnt, Hedgehog and YAP/TAZ pathways, which interestingly are involved in both mechanotransduction and metabolic imprinting of the liver (see below) (Hu et al., 2017b; Kolbe et al., 2019; Wild et al., 2020). PHx has a major impact on the metabolic zonation of the hepatic parenchyma. In the rat, the gluconeogenic capacity of the liver is increased by increasing gluconeogenesis, while glycolysis decreases, which abrogates the zonal gradients of these opposing metabolic pathways (Andersen et al., 1984; Chatzipanagiotou et al., 1985). A very obvious sign of changes in hepatic metabolism is the accumulation of triglycerides after PHx. Surgery-induced stress releases fatty acids from adipose tissue that enter the liver to provide energy substrates for liver regeneration (Lafontan et al., 1997; Walldorf et al., 2010). However, in steatohepatitis and, due to the small liver remnant after extended hepatectomies, excess lipid load occurs and impairs post-hepatectomy regeneration, likely as a consequence of mitochondrial impairment (Hamano et al., 2014; Tautenhahn et al., 2016). Though single cell RNA sequencing revealed a sophisticated atlas of liver zonation and its spatio-temporal regulation (Halpern et al., 2017; Droin et al., 2021), computational modeling has not yet addressed metabolic zonation in the liver or its dynamic regulation by surgical challenges like partial hepatectomy.
Shear stress as a consequence of hepatectomy-induced portal hypertension seems to be a major trigger of hepatic regeneration and size control, which has been postulated for some time (Sato et al., 1997; Niiya et al., 1999). It has now become clear that LSEC are mainly involved in sensing increased shear stress induced by partial hepatectomy. They respond with an increase in vasoactive compounds like NO (Bartels and Hildebrand, 1975; Poisson et al., 2017), mediated by KLF2-dependent induction of, besides others, NO synthase (Parmar et al., 2006). NO regulates the regenerative response after PHx by orchestrating a cytokine network that includes HGF and IL6 to prime hepatocytes for proliferation and TGFβ as the growth terminating signal (Yagi et al., 2020; de Rudder et al., 2021; Kiseleva et al., 2021). Regulation of liver regeneration after partial hepatectomy by LSEC-mediated mechanical forces was predicted using a computational 3D network of sinusoids substantiating the functional link between hepatic flow control and liver regeneration (Ishikawa et al., 2021).
Flow changes after liver surgery may thus be sensed and transmitted to the cell interior, triggering responses like proliferation or cell migration. Little information is available on whether flow-associated metabolic changes might also be associated with mechano-transduction mechanisms. Yet, there is an obvious and hence likely potential crosstalk between mechano-transduction and molecules involved in metabolic regulation.
β-catenin is part of the adherens junction complex and acts as an intracellular transducer of Wnt (wingless/Int) signaling. It can be mobilized from the adhesion junction complex by tyrosine phosphorylation in response to receptor activation by growth factors like HGF, EGF, and TGFβ. Mobilization can lead to nuclear translocation of β-catenin, activation of genes that promote mitogenesis, and dissociation of the adherens junctions complex required for tissue morphogenesis during regeneration and development (Monga, 2014). Signaling through β-catenin, however, also plays a major role in hepatocyte metabolic specification (Burke and Tosh, 2006; Torre et al., 2011). In tumor cells, high expression of aberrantly active forms of β-catenin coincided with high expression of GS, the key enzyme of ammonia fixation in perivenous hepatocytes (Gebhardt et al., 2007). Providing further evidence, conditional disruption of β-catenin expression in mice caused impairment of ammonia metabolism and perivenous expression of enzymes of the CYP family (Sekine et al., 2006). Activation of Wnt/β-catenin signaling by the GSK3B inhibitor SB-216763 or Wnt-conditioned media increased perivenous marker protein expression in isolated hepatocytes (Hailfinger et al., 2006). Thus, β-catenin is involved in both adhesion junctions and metabolic regulation, predominantly in pericentral hepatocytes.
Coordination of tissue homeostasis via adherens junctions and metabolic specification of hepatocytes may be linked via AMPK (Salvi and DeMali, 2018), a central regulator of hepatic lipid metabolism. AMPK is activated through phosphorylation by the upstream kinase complex called LKB1/STRAD-MO25 (liver kinase B1/STE20-related adapter protein-MO25). Activated AMPK in turn inhibits fatty acid synthesis, lipogenesis, and triglyceride synthesis, while anti-lipogenic pathways like fatty acid oxidation and ketogenesis are stimulated (Jansen et al., 2009; Mihaylova and Shaw, 2011). In contrast, dephosphorylation and inactivation of AMPK stimulates fatty acid synthesis, lipogenesis and triglyceride synthesis, which in turn causes steatosis in the chronic stage. Cellular polarity is also regulated by E-cadherin via the LKB1 complex, thus creating a functional link between cell contact maintenance and metabolic regulation of polarized cells such as hepatocytes. In line, the LKB1/STRAD complex and AMPK localize to and thereby stabilize adherens junctions (Sebbagh et al., 2009).
Enhancement of matrix stiffness activates the YAP/TAZ pathway (Dupont et al., 2011). Changes in matrix stiffness are sensed and transduced to the cell interior by the cell adhesion receptors as described above, and communicated to the actin cytoskeleton filaments. Actin reorganization releases cytoplasmic retention of YAP/TAZ. This, in turn, promotes nuclear translocation and transcriptional activation of target genes involved in tissue growth processes like proliferation after injury, embryonic development, and tumor growth in cancer (Pocaterra et al., 2020). Similarly, YAP/TAZ relocation is mediated by substrate stiffness. While soft materials (~1 kPa) foster cytoplasmic localization, stiff substrates (~40 kPa) force nuclear translocation (Dupont et al., 2011; Halder et al., 2012). In the liver, hedgehog-dependent activation of the YAP pathway was necessary to sustain proliferation of hepatocytes after PHx in mice (Swiderska-Syn et al., 2016). The same mechanism also regulated energy supply from glutamine during proliferation of activated HSC. This was associated with epithelial-mesenchymal transition after acute and chronic liver injury (Choi et al., 2009; Du et al., 2018; Chen et al., 2019), indicating a functional relationship between regulation of tissue homeostasis, ECM mechanical properties, and metabolic regulation.
Taken together, mechanical forces induced by changes in hepatic hemodynamics, such as after PHx, appear to play a prominent role in regulating tissue homeostasis and function during liver regeneration, from the organ to the cellular scale.
It should be noted that PHx not only affects the liver but also other organs. In particular, extended liver resections carry a high risk of mortality due to multiorgan failure, indicating an extensive organ-to-organ communication. Cerebral dysfunction is a frequent complication as a result of the increase in neurotoxic metabolites that accumulate due to loss of hepatic detoxification capacity (Søreide and Deshpande, 2021). Although the pathomechanisms of acute kidney injury after hepatectomy remain largely unknown, hemodynamic complications seem to be the prevailing systemic cause of renal complications (Peres et al., 2016). Accordingly, portal hypertension and hyperperfusion in combination with surgery-associated technical procedures like the Pringle's maneuver (occlusion of hepatic artery and portal vein to minimize blood loss) may cause splanchic vasodilation followed by a decrease of the mean arterial pressure, suggesting insufficient perfusion of inner organs including the kidneys (Choukèr et al., 2004; Lee et al., 2009; Tautenhahn et al., 2017). Also, the loss of liver mass after PHx poses per se risks to whole body homeostasis due to the decrease in synthetic capacity providing plasma proteins for, e.g., the blood coagulation cascade and the acute phase response, the liver's defense reaction against surgical trauma and inflammation (Ramadori and Christ, 1999), both of which represent potential risk factors after PHx.
The state of a cell is mainly determined by its protein composition, which is steadily changing due to protein turnover, i.e., protein degradation and synthesis (Schoenheimer, 1942). An important method to gain information about the cell state is gene expression analysis. However, absolute quantification of gene expression is difficult; therefore, gene expression analysis mostly aims for relative quantification when comparing differences in transcription under various conditions (e.g., age, stress, disease, or surgery).
In the context of liver surgery, gene expression has mainly been analyzed in tissue samples, but more and more single cell studies have recently become available. Although most gene expression studies focus on protein-encoding transcripts, it should be kept in mind that only a small fragment of the human genome is protein coding, and many non-protein coding RNA exist.
Gene expression studies can contribute to the understanding of liver functions in general and liver regeneration after hepatectomy in particular. Recently, gene expression studies have used microarray technology and RNA sequencing to gain new insights into liver regeneration. Multiple studies exist that focus on differential gene expression after surgical interventions like PHx, PVL or ALPPS, with the main model organisms being rat and mouse (see Table 6 for an overview).
In general, the transcriptome is analyzed through RNA-Seq experiments. Figure 3 shows a state-of-the-art workflow. The raw reads, which are produced by sequencing the RNA extracted from the sample of interest, can vary greatly in quality. Thus, the first step during RNA-Seq analysis is quality control (Li et al., 2018a) and trimming, to remove sequencing remainders, e.g., sequencing adaptors. Next, the reads are mapped against the reference genome and post-processed (sorting, conversion to binary format). After counting the reads mapped per gene, the data are normalized to make the results comparable among the different replicates (Love et al., 2014). For differential expression, the normalized gene expression is compared between the different groups (e.g., PHx vs. sham). Thus, a list of differentially expressed genes is returned at the end, which can be used for further downstream analyses, such as pathway analysis or modeling.
Gene expression studies are useful to identify signaling pathways that are most affected by a given procedure. A number of studies have been performed focusing on PHx and confirmed the relevance of cell cycle associated genes in the process of liver regeneration (Borger et al., 2019; Colak et al., 2020). Pathway analyses or gene set enrichment analyses compare differentially expressed genes against pathway databases like KEGG (Kanehisa and Goto, 2000) or GO (Ashburner et al., 2000), in which genes are assigned to different pathways. In this way, the enrichment of complete pathways can be inferred from a list of differentially expressed genes. Examples for liver-specific pathways in the KEGG database are for example hsa05225 [Hepatocellular carcinoma–Homo sapiens (human)], hsa04932 [non-alcoholic fatty liver disease–Homo sapiens (human)] or more general ko04979 (Cholesterol metabolism) and ko01100 (metabolic pathways).
Comparative studies contribute to the molecular understanding of the different regeneration mechanisms and kinetics observed in different resection models.
Colak et al. (2020) investigated the differential gene expression patterns in rats subjected to PHx, PVL and ALPPS. This study was based on RNA sequencing and provided a comprehensive overview about transcriptomic changes. A similar study by Li et al. (2018a) revealed that hypoxia pathways were activated in the ligated lobes undergoing atrophy. In contrast, cell proliferation and cell-cycle pathways were activated in the non-ligated lobes undergoing regeneration. Nagano et al. (2004) investigated gene expression changes after PVL followed (4 days later) by PHx, compared with rats subjected to PHx alone. The group with PVL had significantly higher survival compared with the control group, which was associated with greater upregulation of CCND1.
Borger et al. (2019) performed transcriptome profiling on two different mouse models to identify pathways associated with liver regeneration. One group underwent ALPPS, while the other group underwent PVL followed by transection. After ALPPS, the IGF1R signaling pathway, the ILK pathway, and the IL10 pathway were significantly enriched, while the interferon pathway was reduced. Furthermore, the PAK- and ILK-associated intracellular signaling pathways were activated at an earlier time point compared to gene expression after 68% PHx only. These findings suggest accelerated liver regeneration after ALPPS. Dupont et al. (2011) already found that YAP expression changes occur in response to changes in tissue rigidity in liver cells. These changes are known to occur with age, but also after hepatectomy. Pibiri et al. (2015) demonstrated strong changes in gene expression of YAP when comparing normal and regenerating liver in young and old mice. Interestingly, YAP expression was significantly upregulated when comparing young and old mouse livers, but not when comparing quiescent and regenerating livers. Pibiri (2018) discussed the possibility of improving hepatic regenerative capacity in the elderly by eliminating senescent cells via autophagy. This hypothesis remains to be confirmed.
Genome-wide expression analysis provides a good basis for identifying differentially expressed pathways. The impact of different surgical methods on gene expression and pathway activation have been well studied (see Table 4). However, most of these studies analyze regeneration after liver surgery in general, without considering the underlying causes separately.
Perfusion, mechanosensing and mechanotransduction are important processes that possibly affect liver regeneration and functional recovery (Song et al., 2017). Only few studies exist that analyze the interplay between these processes (Tchirikov et al., 2002; Nishii et al., 2018).
A comprehensive review of liver metabolism can be found in Rui (2014). More specifically, multiple genes related to metabolism are described to be induced in severe NAFLD. For genes being specifically differentially expressed in the regeneration process after PHx with and without partial portal ligation, we refer to Nobuoka et al. (2006). Notably, minimal CYP reduction was observed during liver regeneration, with the conclusion that portal blood flow plays an important role in liver regeneration and cell cycle gene expression. In another study, shear stress on murine liver progenitor cells could be shown and was reflected by significant upregulation of regeneration-associated genes such as CFOS, IP10, MKP1, ALB, WNT, VEGF, or EpCAM (Nishii et al., 2018).
In contrast to transcriptomics analysis, which is widely applied in the context of liver surgery, data on changes in proteomics (e.g., Strey et al., 2005; Guo et al., 2006; Sun et al., 2007; Kumar et al., 2013; Chen and Xu, 2014 and metabolomics (e.g., Jung et al., 2013; Samino et al., 2013; Saito et al., 2018; Carril et al., 2020; Zhao et al., 2020) are rather sparse. Almost all studies focus on a single omics approach, while multi-omics has rarely been applied. One exception is the work of Caldez et al. who used an integrated transcriptomic and metabolomic approach to study metabolic remodeling during liver regeneration following hepatectomy (Caldez et al., 2018).
The information from omics studies can be used in combination with computational models to better understand changes in signaling, metabolism and hepatic function. The general workflow for integration is as follows:
(i) Preprocessing and normalization of omics data. In this initial step, data from different experimental conditions and time points are made comparable (normalization of data), outliers are filtered out and a quality control of the data is performed (e.g., removal of batch effects).
(ii) Quantification of differential changes. Most omics approaches provide reliable data for relative changes between conditions, whereas absolute approaches are more complex (e.g., spike ins), often challenging, and rarely applied. The result of the analysis is a list of top molecular candidates based on significance and/or fold change (e.g., a list of differentially expressed transcripts during regeneration after hepatectomy.
(iii) Linking the experimental data with the model. This includes mapping of the experimentally determined fold changes to model parameters and variables in the model. Transcriptomics data is often used as a proxy for changes in protein levels and mapped to the corresponding proteins in the model, whereas proteomics and metabolomics data can be mapped more directly. A computational model for the control condition is often used as a baseline model that is parametrized with the data to obtain multiple model variants corresponding to the various experimental conditions.
(iv) Simulation under differential parametrization. The last step consists of performing simulations with the baseline model and the different model variants and evaluating changes in model predictions.
Since omics data provides information on cellular components (RNA, proteins, metabolites), the corresponding computational models reflect the cellular scale (see section 3.2.1). A classical approach is the parametrization of constraint-based metabolic models to generate tissue-specific or condition-specific models. Examples in the context of liver metabolism are the stratification of patients with HCC based on acetate utilization (Björnson et al., 2015), a metabolic and functional evaluation of NAFLD by integrating metabolic flux data and global transcriptomic data from human liver biopsies (Hyötyläinen et al., 2016), or the study of metabolic pathways in NAFLD (Mardinoglu et al., 2014). Metabolic network-based stratification has been used to reveal distinct tumor subtypes or heterogenous redox responses in HCC using transcriptomics data (Bidkhori et al., 2018; Benfeitas et al., 2019). Benefits of a carbohydrate-restricted diet on hepatic steatosis have been studied using a similar multi-omics approach (Mardinoglu et al., 2018). A similar workflow is applied for kinetic pathway models, for instance to study metabolic heterogeneity in HCC based on proteomics data (Berndt et al., 2021).
The same approach can be applied for spatially resolved data, e.g., using zonated omics. The data is hereby mapped on sub-models corresponding to the respective spatial location (e.g., periportal or perivenous). An example is the study of ammonia detoxification in rodent liver (Bartl et al., 2015).
While omics data have been measured in the context of liver surgery, the integration of these data into computational models is still in its infancy. Time courses of the transcriptome in regeneration exist (different conditions and different time courses), but have not yet been integrated into computational models. Similarly, there are no approaches yet to predict hepatic functions after surgery-induced perfusion changes by computational modeling.
One vision of systems medicine in liver surgery is the development of an integrated modeling framework. This framework should (i) encompass state-of-the-art knowledge of vascular anatomy, tissue architecture, liver perfusion and their impact on key physiological processes, (ii) integrate clinical and experimental data from all relevant measurement/assessment procedures at the different temporal and spatial scales, (iii) allow the study of the system in silico using model predictions, and (iv) enable individual risk assessment.
Computational models can generally provide such a framework. In the context of hepatic surgery, the specific goal is to support pre-operative personalized diagnostic and risk assessment. This requires reliable predictions of the residual hepatic function after resection, the hepatic regeneration capacity, and the course of functional recovery after surgery. All three are influenced by perfusion alterations on different scales and their impact on function. In this context, consistent estimation of uncertainties in model predictions is important to inform decisions about surgical strategy (Saltelli et al., 2020).
Achieving this ambitious goal requires a concerted and iterative effort by clinicians, modelers, and experimenters in a modeling-experiment cycle. Such a process typically begins with an initial set of defined experiments and a set of models. In the context of hepatic surgery, these are measurements obtained in cell cultures and animal experiments and in patients undergoing PHx. Multidimensional data include anatomical and morphological data such as hepatic volumetry and information regarding type and severity of pre-existing disease (e.g., steatosis), liver perfusion, quantification of liver function (e.g., by test compounds), and additional data such as omics, all obtained from multiple sources.
Based on existing modeling approaches, structure and granularity of the models must be adapted to the data and the particular question being addressed. For example, assessment of liver function at the organ level does not reflect the heterogeneity of pathways in the liver parenchyma or even in individual hepatocytes, hence appropriate models must be coarse-grained in this regard. Likewise, liver regeneration is observed only on a daily basis, assuming that the time course can be interpolated between measurements on different days. Omics data is gathered from selected ROI, assuming that these ROIs are representative of the organ. Integrating data into computational models in such an iterative cycle is generally a challenging task, as is quantifying uncertainty. In this section, we present specific challenges of modeling hepatic perfusion-function relationships for better risk assessment in the context of liver surgery (section 3.1), followed by an overview of relevant computational models on the multiple scales (section 3.2).
Currently, there are no standards for integrating various data types and linking different spatial and temporal scales. In the context of liver surgery, data include (i) imaging data at different spatial scales such as CT and MRI reflecting organ scale and histology reflecting lobular scale, (ii) numerical data on hemodynamic information such as pressure and flow data as well as microcirculation data, (iii) numerical data on metabolism and proliferation reflecting organ and cell scale (see section 2), and (iv) omics data representing molecular scale in a given region of interest. Collectively, these data describe and quantify interrelated processes at the molecular, cellular, lobular and organ scale.
The calibration of models to experimental or clinical data requires a direct comparison between data and model outputs that goes beyond a purely qualitative agreement. Quantification of the quality of a fit in terms of numerical values is necessary for comparison of different model fits and enables the application of optimization algorithms and methods for model comparison. This procedure requires appropriate data pre-processing steps such as normalization, background correction, transformations, elimination of outliers, or estimation of summary statistics. For large datasets, for example omics or imaging data, additional machine learning approaches are used for feature selection and dimension reduction. Often, model calibration is then formulated as an optimization problem in which the pre-processed data are incorporated as numerical values. Such problems belong to the class of (non-linear) inverse problems whose solutions require efficient algorithmic schemes.
For example, a standard modeling approach for hepatic metabolic pathways such as the test compound metabolism or signaling pathways like YAP/TAZ-induced pathways is ordinary differential equations based on chemical reaction kinetics. Time course data of key metabolites are used to estimate unknown reaction rate constants. These data must be normalized and often contain information about fold changes rather than absolute concentrations. This preprocessing step can be done in different ways (Degasperi et al., 2014) and affects sensitivity analysis and summary statistics (Kirch et al., 2016; Thomaseth and Radde, 2016). The objective function in the optimization problem can be the sum of squared differences (least squares estimate) or the likelihood function with an appropriate error model (Kreutz et al., 2007). Coupling metabolic models with models on larger scales, such as models of pressure and blood flow distributions in liver lobules, requires the definition of coupling parameters and, for model calibration, also model reduction techniques.
Standards for representing pathway-based models have been established, with the SBML being the de facto standard (Hucka et al., 2019; Keating et al., 2020). Extensions enable hierarchical model composition (comp package) (Smith et al., 2015), and uncertainty representation (distrib package) (Smith et al., 2020), an important requirement for multi-scale modeling approaches and tracking model and data uncertainty. While mechanisms exist for the annotation of models and data with meta-data (Neal et al., 2019), and how to share experimental and clinical data with the modeling community (König et al., 2021), standards for data-model integration and corresponding workflows are lacking, and coupling models in different mathematical frameworks remains a challenge.
Even if the structure of a model is defined, the data available for model calibration often do not contain sufficient information to unambiguously identify all model parameters. This already applies to models on individual scales, such as intracellular metabolic pathways or pharmacokinetic models at the whole-body scale, due to low time resolution of the measurements or because only a few model components can be quantified. The problem is exacerbated for larger and multi-scale models. Statistical methods generally provide a solution to this problem. They allow consistent tracking of variability in the input data via uncertainty in model parameters to confidence bounds in model predictions. However, many of those methods are computationally expensive and thus not applicable ad hoc to larger models, so they must be adjusted accordingly. In general, uncertainty in model predictions is often underestimated because model assumptions are not questioned, the effect of non-modeled factors is neglected, or local sensitivity methods are used even in cases where parameters and input variables are largely uncertain (Saltelli et al., 2020). Since decisions based on model predictions, such as the selection of patients for surgery, are highly dependent on uncertainties, it is important to develop methodology for the adaptation of statistical methods for consistent estimation of sensitivities and uncertainties for the specific problem at hand.
Coupled processes on different length and time scales, as well as the need for spatial resolution to describe spatial inhomogeneities in the liver, require multi-scale models. These models suffer from long forward simulation times. Hence, standard methods for sensitivity analysis, uncertainty quantification, parameter estimation, or identifiability analysis are not applicable ad hoc for these models. For example, a single forward simulation of a spatially-resolved liver lobule on a workstation with i7 processor of the 7th generation, 4 cores and 8 threats requires 15 min to simulate one-twelfth of a single lobule and more than 900 min for a group of seven adjacent lobules. For a global sensitivity analysis, the number of forward simulations to be performed grows exponentially with the number of parameters, resulting in a huge computational cost. Overall, long forward simulation times of multi-scale models combined with difficult inverse problems for model calibration and the need for uncertainty analysis due to sparse data, pose a major challenge toward an integrated framework to support decisions in liver surgery. On the modeling side, there is much room for development of methodology to make the analysis more efficient, such as model reduction techniques or the use of surrogate models to reduce simulation times or efficient numerical schemes to solve optimization problems and quantify uncertainties in model predictions.
Another challenge is the translation of models calibrated with animal data to models involving patient data. For example, computational models using hemodynamic measurements after liver resection or PVLs in rodents need to be adapted to the patient situation, taking into account different anatomical features as well as parameters like age, gender, and pre-existing diseases. It is known that animal studies are often poor direct predictors of human responses to medical treatments or exposures (Perel et al., 2007; Bracken, 2009). A modeling framework adapted to human parameters could address this problem, but is challenging. Not only does one have to deal with different types of data for humans and animals, human patients have much larger variability. This is because liver (dys)function, liver metabolic status, and function-perfusion relationships depend on individual lifestyle, environmental influences, and dietary habits. The effects of those factors can be controlled in designed animal studies. Thus, building predictive models for patients undergoing liver surgery based on models fitted to animal data is a multifaceted problem. However, it is also known that many physiological and mechanical parameters, such as lobulus architecture or liver perfusion, are similar in humans and can therefore be transferred (Kruepunga et al., 2019). For some parameters, such as the regeneration course after resection, transfer can be done by proper rescaling of the time scale, for which comparison studies are available in the literature (Periwal et al., 2014). If a transfer of parameters is not possible, models must be adapted to patients by calibrating selected parameters to human data. Furthermore, models can be enriched by analysis methods applied to human data to extract influential features on regeneration trajectories as well as risk analyses and their integration into models.
Multiscale computational models are a unique approach to gain a better understanding of liver surgery-induced alterations in hepatic perfusion, function and subsequent regeneration. The relevant scales range from whole body to single cell (Figure 4). At the whole-body scale, the systemic circulation connects the liver to the rest of the body. At the organ scale, blood is distributed within the liver through a network of hepatic vessels (macroscopic perfusion) that supply the liver with oxygen-rich blood from the hepatic artery and nutrient-rich blood from the portal vein. The hexagonal liver lobules form the functional units of the liver, in which the blood is guided via sinusoids along the liver cells from the outer periportal region to the central perivenous region (microcirculation). The perivenous blood is subsequently drained from the liver into the inferior vena cava via the hepatic veins. Mechanotransduction, signal transduction, and metabolism occur at the level of the hepatocytes located along the blood vessels (sinusoids) of the liver lobules.
Figure 4. Multiple scales relevant for computational modeling of perfusion-function relationships. Anatomograms under CC-BY Papatheodorou et al. (2020).
In this section, we review relevant computational models for understanding the effects of hepatectomy-induced alterations in perfusion on hepatic function at the cellular scale (section 3.2.1), lobular scale (section 3.2.2), organ and whole-body scale (section 3.2.3).
Computational models at the cellular scale can provide insights into the mechanism of how changes in tissue micro-perfusion and mechano-properties can alter hepatic function. Relevant processes include mechanotransduction, signaling, and metabolism (Figure 5); selected computational models are listed in Table 7.
Figure 5. Cellular adaptations to changes in tissue perfusion and mechanical properties (mechanotransduction, signaling, metabolism). The extracellular matrix (collagen, proteoglycans) plays an important role in how changes in perfusion and pressure are translated to changes in stiffness and force. Changes in stiffness and force are sensed by cadherins and integrins (mechanotransduction), which transfer the information via signaling cascades. A main signaling route is the FAK to YAP/TAZ pathway, with important crosstalk from the Hippo pathway. Important signaling pathways regulating metabolism can be modified due to mechanotransduction, among them AMPK, PI3K/AKT, MAPK, JNK and β-catenin which regulate central metabolic functions. See section 2.5 for details.
Mechanotransduction is the process of sensing and translating changes in stiffness/elasticity and force into a cellular signal (see also section 2.5). Substantial knowledge exists on the biochemical and mechanical properties of the ECM (collagen, proteoglycans) and its interaction with the cytoskeleton (actin, intermediate filaments). Detailed computational models have been developed on these interactions (see Elosegui-Artola et al., 2018 for a recent review). Less information and models exist on mechanotransduction via integrins and cadherins. Examples range from a model that couples mechanotransduction to YAP/TAZ signaling (Peng et al., 2017), to a model of how ECM mechanical properties convert to biochemical signals via adhesion and integrated intracellular signaling cascades associated with cytoskeleton dynamics (Sun et al., 2016), to a mathematical model of Hippo and TGFβ cross-talk to YAP/TAZ signaling (Labibi et al., 2020).
Signaling pathways translate the mechanical signals into cellular responses. Existing models representing mechanosensing and mechanotransduction mainly focus on the YAP/TAZ pathway, e.g., YAP/TAZ mechanosensing (Sun et al., 2016) or the control of TGFβ/Smad nuclear accumulation by YAP/TAZ (Labibi et al., 2020). An important part of the YAP/TAZ signaling is the shuttling of YAP/TAZ between nucleus and cytoplasm. Scott et al. modeled this nuclear translocation via a stiffness transfer function (Scott et al., 2020), and Wehling et al. used 2D reaction diffusion equations (Wehling et al., 2021). In contrast, Aprupe et al. applied an ODE approach to model YAP/TAZshuttling as a response to cell density, actin dynamics, and liver damaging drugs (Aprupe et al., 2020). Meyer et al. presented a mechanistic model predicting switch-like activation of YAP upon mechanical stimulation during regeneration (Meyer et al., 2020). Guberman et al. (2020) developed a Boolean regulatory network model in epithelial cells that synthesizes mechanosensitive signaling linking anchorage and matrix stiffness to proliferation and migration, including key signaling players such as YAP, PI3K, AKT, and MAPK.
Hence, YAP/TAZ has been the focus of numerous modeling approaches, but linkage and crosstalk to other signaling cascades is still in its infancy. One such example is a model of CDK with phenomenological effect of ECM via FAK and cell density (contact inhibition mediated via Hippo/YAP pathway) (Gérard and Goldbeter, 2014). Metabolism encompasses the set of chemical reactions, with the liver being the metabolic hub of the body. The activity of a key regulator of cell metabolism, AMPK, has been shown to be mechanoresponsive and thus can bridge adhesion mechanotransduction and energy homeostasis (Isogai et al., 2017).
Consequently, key hepatic pathways such as glucose metabolism, fatty acid metabolism, or oxidative phosphorylation can be affected by changes in perfusion. Similarly, many of the pathways relevant for evaluating liver function using test compounds, such as cytochrome P450 detoxification (e.g., LiMAx via CYP1A2) or transport and secretory activity (e.g., ICG) could be mechanosensitive and be affected by perfusion changes following hepatectomy. Metabolic models of many of these pathways have been established in recent years. Genome-scale models of hepatic metabolism using a constraint-based approach exist (Gille et al., 2010; Mardinoglu et al., 2014) as do detailed kinetic models of central carbon metabolism (Berndt et al., 2018) or glycolysis (König and Holzhütter, 2012; König et al., 2012). Small metabolic pathway models are often included in lobular and organ scale models, e.g., acetaminophen detoxification (Sluka et al., 2016; Fu et al., 2018; Means and Ho, 2019) or glucose metabolism (Ricken et al., 2015). However, linking metabolic pathway models to mechanotransduction and mechano-signaling has rarely been established. One such example is a mechanobiological model to study the effect of shear stress on urea and albumin synthesis (Nikmaneshi et al., 2020).
In summary, models have been established for key signaling pathways involved in mechanotransduction as well as metabolic models for pathways important for liver function, such as central carbon metabolism or detoxification. However, models integrating mechanosensing/mechanotransduction with these signaling pathways and subsequent changes in hepatic metabolism are lacking so far. Furthermore, most of the existing models on mechanotransduction and signaling are not liver-specific.
In recent years, a wide range of computational models for simulating hepatic perfusion at the liver lobule scale has been presented (Table 8). These models differ both in the selection of the method and in the consideration of perfusion processes. An overview of computational models for hepatic processes can be found in Christ et al. (2017) or Ricken and Lambers (2019). A common method for describing hepatic microcirculation at the lobular scale is CFD. Using this method, simulations of hemodynamics in a portion of a lobule (Rani et al., 2006) or one respective sinusoid (Ma et al., 2020) have been presented. Extension of the geometry to a hexagonal shaped liver lobule (Debbaut et al., 2014) using CFD has provided insights into the spatial distribution of lobular perfusion and microcirculation.
The porous structure of the liver and the resulting effects are captured by porous media approaches. Various models have been developed to simulate lobular perfusion (Antonov et al., 2017) after liver resection (Bonfiglio et al., 2010) or in fibrotic and cirrhotic liver (Hu et al., 2017a).
However, these models do not consider hepatic function and its coupling with perfusion. Several multiscale models combine processes on organ, lobular and cellular scale using PBPK equations (Diaz Ochoa et al., 2012; Sluka et al., 2016) or PDE-ODE coupling (Ricken et al., 2015).
To mimic the behavior of hepatic processes at the lobular scale, a detailed knowledge about the organ physiology as well as the sensitivity to changes in boundary conditions is required. A suitable simulation of function-perfusion-coupling depends on material parameters as well as boundary conditions used as initial values for the lobular model. Since the model quality is directly related to the quality of the available data, detailed observation of hepatic conditions and their measurements are necessary. Information on liver geometry, heterogeneity of liver lobules, damage such as the degree of fatty tissue, hyperperfusion, growth and remodeling (Ricken et al., 2007; Ateshian and Ricken, 2010; Ricken and Bluhm, 2010) and the degree of resection must be determined in experiments or clinical procedures and then integrated into the computational framework.
For example, computational modeling at the lobular scale requires information about tissue elasticity to capture the poroelastic behavior. Information on the mechanical behavior of liver tissue can be determined using, e.g., indentation methods or multiparametric MR elastography (Seyedpour et al., 2021). The elasticity modulus can be determined non-invasively via various methods described in Section 2.3.1. To capture the realistic geometry of liver lobules with the respective inflow and outflow conditions, the geometry can be determined by image segmentation from histological sections (Ahmadi-Badejani et al., 2020). Hepatic blood flow can be measured using Doppler-US or functional MRI for macroscopic blood flow as well as OPS for microcirculation (Ricken et al., 2010).
The development of a robust and efficient multiscale model must integrate the data provided by cellular models via appropriate coupling parameters. To reduce computational costs, MOR techniques (Armiti-Juber and Ricken, 2021) can be implemented. To get an initial intuition of the model properties, surrogate models of parts of the simulation are compiled for a quick analysis of the component behavior.
Computational models at the whole-body scale can provide important information on systemic circulation and hepatic blood flow, as well as whole-body function. PK models can provide an accurate description of whole liver clearance or metabolization/function (Willmann et al., 2003). An important class are PBPK models describing the whole body (Jones and Rowland-Yeo, 2013) (Figure 6). These models include the systemic circulation between organs and are therefore uniquely suited to evaluate the effect of blood flow changes on hepatic function. PBPK models integrate information from multiple sources, including drug-dependent, physiological, and biological parameters and their variation between species, subjects, or with age and disease state. The biological and mechanistic bases of PBPK models allow extrapolation of kinetic behavior between species (Espié et al., 2009).
Figure 6. Physiologically based pharmacokinetic (PBPK) model for liver function at the whole-body scale. (A) PBPK models include organs and the systemic circulation between these organs. The liver receives approximately 20% of the cardiac output as blood flow with one-quarter from the hepatic artery and the remainder from the portal vein. Liver function depends on hepatic perfusion and metabolism, as well as on mechanical tissue properties, which can influence hepatic function either directly via changes in blood flow or indirectly by changes in metabolism following mechanotransduction. In the example, all organs besides the liver and the lung have been pooled in the Rest compartment for simplicity. Qlung, blood flow lung; Qha, blood flow hepatic artery; Qgi, blood flow gastrointestinal tract; Qpv, blood flow portal vein; Qhv, blood flow hepatic vein; Qrest, blood flow rest of body. (B) The pharmacokinetics of liver test compounds, as measured in dynamic liver function tests such as ICG elimination or LiMAx, allows assessment of liver function at the whole body scale. Shown here in red caffeine (test compound) and in black paraxanthine (main product) in an example liver function test based on caffeine. PBPK models are excellent for modeling these tests. Anatomograms under CC-BY Papatheodorou et al. (2020).
Various hepatic clearance models have been developed to integrate the factors of blood flow, binding, transport and metabolism at the whole-body scale to predict clearance of substances (Ho and Zhang, 2020). The simplest model is the well-mixed model, which describes the liver as a single compartment in which the drug concentration in the liver is in equilibrium with that in the emergent blood and the concentration of compounds is identical throughout the liver (Rowland et al., 1973; Pang and Rowland, 1977). Approaches to describe heterogeneity within the liver include the parallel tube model (Winkler et al., 1973), the distributed-model (Bass et al., 1978), the series-compartment model (Gray and Tam, 1987), and the variable transit-time or dispersion model with mixing between sinusoidal blood and hepatocytes (Goresky et al., 1973).
While PBPK models are routinely applied to predict drug detoxification under healthy and disease conditions (e.g., liver cirrhosis Edginton and Willmann, 2008), their application in the context of liver surgery and hepatectomy is still in its infancy (Table 9). Existing models account for the loss of liver volume in hepatectomy by reducing the liver volume in silico (Lagneau et al., 2005; Lu et al., 2006; Köller et al., 2021a,b) and allow simulations of the effects of different resection rates on pharmacokinetics (e.g., on liver function measured by ICG Köller et al., 2021a,b). The model by Lagneau et al. considered changes in perfusion or hemodynamics due to liver surgery, i.e., intraoperative liver pedicular triad clamping, intraoperative blood loss, and the associated changes in cardiac output in addition to the volume loss (Lagneau et al., 2005).
Hemodynamics is included in PBPK models in the form of blood flows between compartments and tissues, but important information such as pressure, pressure gradients, or tissue resistance is missing. In contrast, approaches simulating CFD allow detailed capture of postoperative hepatic hemodynamics in reconstructed vascular geometries. CFD models have been applied to study the changes in hemodynamics following hepatectomy in humans (Ho et al., 2010, 2012; Ma et al., 2020; Lin et al., 2021). CFD approaches can be computationally very intensive (Lin et al., 2021). Moreover, the existing examples only cover the respective reconstructed vascular structures of the liver, without information about the systemic circulation. Closed loop 0D models (a lumped parameter model of the entire circulation without any spatial dimensions) allow detailed modeling of cardiac output and systemic circulation (Shi et al., 2011). Depending on their resolution, the liver can either be modeled as a single unit or the structure of the lobes can be resolved. Closed loop 0D models can be solved efficiently, reflect physiology, and be used to model surgical interventions. Such models have been applied to study the impact of PHx on the hepatic hemodynamics in rats (Debbaut et al., 2012), pigs (Audebert et al., 2017), and humans (Golse et al., 2021). 0D models can easily be coupled with 1D, 2D, or 3D models of vessels or tissues and contain information on pressures and flows, both important requirements for multi-scale approaches. Systemic circulation has been studied in detail with 0D models, but coupling with PBPK models to describe clearance of substances through the liver has not been done to our knowledge.
An important step would be to couple models of hemodynamics (0D closed loop) and function (PBPK) at the whole-body scale with spatially resolved 1D/2D/3D approaches on the sinusoid/lobule scale. Application of such multi-scale models to dynamic liver function tests (such asICG or LiMAx) could provide important insights in how alterations in hepatic hemodynamics after hepatectomy affect liver function.
The liver can only perform its various functions in the context of the entire body, and conditions affecting other organs can alter liver function. Heart disease is often associated with altered cardiac output, resulting in changes in hepatic perfusion, which affects the clearance of substances by the liver. The marked effects of changes in cardiac output on liver function as measured by ICG were studied with a recent PBPK model in Köller et al. (2021a). Simulations of blood flow using computational models of the heart could provide important insights into the heart-liver connection. Kidney disease resulting in renal impairment can have effects on liver function due to changes in clearance of substances by the kidneys, leading to an additional burden on the liver. Furthermore, renal impairment is often associated with alterations in blood pressure, resulting in changes in systemic circulation. Comorbidities of other organs can also affect the results of liver function tests, e.g., pulmonary disease can alter the results of respiration tests such as the LiMAx test. While some hepatic functions are affected by a few organs (e.g., ICG by the heart and changes in cardiac output), other functions such as carbohydrate and energy metabolism, which play a crucial role in regeneration after hepatectomy, can be affected by multiple organs (e.g., insulin secretion by the pancreas, glucose utilization by muscle, free fatty acid release by adipose tissue, glucose filtration by the kidneys, …).
PBPK models provide a unique platform to understand how organs besides the liver and comorbidities of these organs can affect hepatectomy and regeneration after hepatectomy. Important future work, not only in the context of liver surgery but for the systems medicine approach in general, will be the integration of computational models of various organs toward an in silico human.
In this review, we have delineated current knowledge on the relationships between alterations in hepatic perfusion and their consequences for hepatic function in the context of liver surgery, using hepatectomy as an example.
Computational models and systems medicine approaches can contribute to a better understanding of the complex perfusion-function interactions after hepatectomy at all relevant spatial and temporal scales, from the single cell to the whole liver and from immediate changes after surgery to long-term regeneration. Multiscale models provide a unique opportunity to integrate heterogeneous experimental and clinical data. But despite a wide array of available tools in clinical and experimental settings, data generated with these methods have not yet been systematically integrated into multi-scale computational models in the context of liver surgery. Major challenges remain, such as lack of data integration standards, sparse data settings and uncertainty quantification (Pivovarov et al., 2019), computational cost of multi-scale models, and transfer of models calibrated with animal data to patients. Key requirements for success are tight cooperations between animal, clinical, and modeling research in an iterative cycle. Especially important in this context is the generation of animal data orthogonal to the patient data for model calibration, complemented with data featuring sufficient overlap between animals and patients to enable transfer.
Current computational tools applied in the clinic are limited to visualization of tumor localization in relation to individual vascular anatomy and virtual planning of the resection plane. Virtual resection allows visualization and quantification of the volume of the territories at risk. However, current visualization tools cannot determine the impact of impaired hepatic venous drainage on hepatic function. They do not account for the impact of surgically induced perfusion perturbations on hepatic function after resection. A key challenge is to develop multi-scale computational models for liver function diagnostics applied in liver surgery, such as the dynamic liver function tests based on ICG or LiMAx. Currently, there is no integrated cross-scale computational model that integrates functional aspects at the molecular and cellular level as well as hepatic perfusion at the lobular level to predict global function at the organ level. Therefore, predictions that account for any spatial inhomogeneity are not possible. Multidimensional modeling is needed to bridge the gap between current possibilities to assess hepatic perfusion, metabolism and regeneration and the need for reliable predictions for individual patients.
A systems medicine approach based on multiscale predictive models and incorporated into the clinical decision-making process could provide actionable information. In the context of liver surgery, the use of these techniques will improve prediction of outcomes after extended liver resection. This may help avoid surgery in high-risk patients and enable a personalized approach to advanced hepatic surgery. Based on the models and resulting predictions, individual pre-rehabilitation interventions can be initiated to reduce perioperative morbidity and mortality after extensive liver resections.
To be successful, two major gaps need to be addressed: (i) the application of multi-scale models in the context of liver surgery and (ii) the consideration of the complex perfusion-mechano-function interactions at the micro- and macro-scale using such a systems medicine approach.
Future clinical translation is facing even higher complexity. Extrinsic factors and processes involving individualized patient data comprising underlying liver diseases and systemic comorbidities must be included. The high complexity of the data needs advanced modeling and data integration strategies that require concerted iterative actions between experimenters, clinicians, and modelers. In this sense, this review aims to promote mutual understanding of biological processes and their computation in systems approaches by natural scientists, physicians and modelers to pave the way for future systems medicine implementation in clinical practice. This also envisions collaborative efforts to couple systems medicine models with existing hospital information systems to enable interoperability and integration of patient data and predictive models. Our long-term vision is an application integrated in the hospital information system that enables accurate estimation of liver regeneration and postoperative morbidity and mortality already at the virtual planning stage of surgery, with the goal of establishing reliable individualized and patient-tailored liver surgery.
BC, UD, K-HH, MK, LL, MM, NR, JRR, TR, and H-MT were involved in design ing, writing and revising the manuscript. MC, SH, and DM wrote sections of the manuscript. All the authors contributed to manuscript revision, read, and approved the submitted version.
This work was supported by the German Research Foundation (DFG) within the Research Unit Programme FOR 5151 QuaLiPerF (Quantifying Liver Perfusion–Function Relationship in Complex Resection—A Systems Medicine Approach) by grant no. 436883643. NR, TR, and LL are supported by Deutsche Forschungsgemeinschaft (DFG, German Research Foundation) under Germany's Excellence Strategy–EXC 2075–390740016. UD, TR, and H-MT are supported by Deutsche Forschungsgemeinschaft (DFG, German Research Foundation) by grant no. 465194077 (Priority Programme SPP 2311, Subproject SimLivA). TR is supported by Deutsche Forschungsgemeinschaft (DFG, German Research Foundation) by grant no. 312860381 (Priority Programme SPP 1886, Subproject 12). MK is supported by the Federal Ministry of Education and Research (BMBF, Germany) within the research network Systems Medicine of the Liver (LiSyM, grant no. 031L0054). BC and H-MT are supported by the Deutsche Forschungsgemeinschaft (DFG, German Research Foundation) in a joint research project (CH 109/25 and TA 1583/1). UD is supported by the Deutsche Forschungsgemeinschaft (DFG, German Research Foundation) by grant no. DA 251/13-1 (SteaPKMod). MM is supported by the Carl-Zeiss-Stiftung by grant no. IKZ0563-2.8/738/2 and 5575/10-9.
The authors declare that the research was conducted in the absence of any commercial or financial relationships that could be construed as a potential conflict of interest.
All claims expressed in this article are solely those of the authors and do not necessarily represent those of their affiliated organizations, or those of the publisher, the editors and the reviewers. Any product that may be evaluated in this article, or claim that may be made by its manufacturer, is not guaranteed or endorsed by the publisher.
ALPPS, associating liver partition and portal vein ligation for staged hepatectomy; AMPK, AMP-activated protein kinase; ARFI, acoustic radiation force impulse; ASL, arterial spin-labeling; CDK, cyclin-dependent kinase; CFD, computational fluid dynamics; ChE, cholinesterase; CT, computed tomography; CYP, cytochrome P450; DCE-MRI, dynamic contrast-enhanced MRI; DSC-MRI, dynamic susceptibility contrast MRI; DWI, diffusion weighted imaging; ECM, extracellular matrix; EGF, epidermal growth factor; FAK, focal adhesion kinase; FLR, future liver remnant; FOO, focal outflow obstruction; Gd-EOB-DTPA, gadolinium ethoxybenzyl-diethylenetriaminepentaacetic acid; GO, gene ontology; GS, glutamine synthetase; GSK3B, glycogen synthase kinase 3 beta; HBF, hepatic blood flow; HBS, hepatobiliary mebrofenin scintigraphy; HCC, hepatocellular carcinoma; HEF, hepatic extraction fraction; HGF, hepatocyte growth factor; HSC, hepatic stellate cells; HVPG, hepatic vein pressure gradient; ICG, indocyanine green; ICG-PDR, indocyanine green plasma disappearance rate; IVIM, intravoxel incoherent motion; JAM, junctional adhesion molecules; KEGG, kyoto encyclopedia of genes and genomes; LiMAx, Liver MAximum capacity test; LSEC, liver sinusoidal endothelial cells; MAPK, mitogen-activated protein kinase; MDRP2, multidrug resistance protein 2; MOR, model order reduction; MRE, magnetic resonance elastography; MRI, magnetic resonance imaging; MR, magnetic resonance; NAFLD, non-alcoholic fatty liver disease; NO, nitric oxide; OPS, orthogonal polarization spectroscopy; PBPK, physiologically based pharmacokinetic; PDFF, proton-density fat fraction; PET, positron emission tomography; PHx, partial hepatectomy; PI3K, phosphoinositide 3-kinase; PKC, protein kinase C; PK, pharmacokinetic; PVL, portal vein ligation; ROI, regions of interest; SBML, systems biology markup language; SPECT, single-photon emission-computed tomography; SWE, shear wave elastography; TAZ, WW domain-containing transcription regulator protein 1; TGF-β, transforming growth factor β; US, ultrasound; YAP, Yes-associated protein; ZO, zonula occludens proteins.
Abshagen, K., Eipel, C., and Vollmar, B. (2012). A critical appraisal of the hemodynamic signal driving liver regeneration. Langenbecks Arch. Surg. 397, 579–590. doi: 10.1007/s00423-012-0913-0
Adas, G., Koc, B., Adas, M., Duruksu, G., Subasi, C., Kemik, O., et al. (2016). Effects of mesenchymal stem cells and vegf on liver regeneration following major resection. Langenbecks Arch. Surg. 401, 725–740. doi: 10.1007/s00423-016-1380-9
Ahmadi-Badejani, R., Mosharaf-Dehkordi, M., and Ahmadikia, H. (2020). An image-based geometric model for numerical simulation of blood perfusion within the liver lobules. Comput. Methods Biomech. Biomed. Eng. 23, 987–1004. doi: 10.1080/10255842.2020.1782389
Alexius, B. (1991). [CNS changes can be present in asymptomatic HIV infection]. Lakartidningen 88, 4004–4005.
Aller, M.-A., Mendez, M., Nava, M.-P., Lopez, L., Arias, J.-L., and Arias, J. (2009). The value of microsurgery in liver research. Liver Int. 29, 1132–1140. doi: 10.1111/j.1478-3231.2009.02078.x
Andersen, B., Zierz, S., and Jungermann, K. (1984). Alteration in zonation of succinate dehydrogenase, phosphoenolpyruvate carboxykinase and glucose-6-phosphatase in regenerating rat liver. Histochemistry 80, 97–101. doi: 10.1007/BF00492778
Antonov, M. Y., Grigorev, A. V., and Kolesov, A. E. (2017). “Numerical modeling of fluid flow in liver lobule using double porosity model,” in Numerical Analysis and Its Applications, Vol. 10187 of Lecture Notes in Computer Science, eds I. Dimov, I. Faragó and L. Vulkov (Cham: Springer International Publishing), 187–194.
Aprupe, L., Schmitt, J., Liu, K., Schirmacher, P., Kummer, U., and Breuhahn, K. (2020). Mathematical modeling of the yap/taz shuttling as a response to cell density, actin dynamics, and liver damaging drugs. Z. Gastroenterol. 58, 4–21. doi: 10.1055/s-0039-3402221
Araki, K., Harimoto, N., Kubo, N., Watanabe, A., Igarashi, T., Tsukagoshi, M., et al. (2020). Functional remnant liver volumetry using gd-eob-dtpa-enhanced magnetic resonance imaging (mri) predicts post-hepatectomy liver failure in resection of more than one segment. HPB 22, 318–327. doi: 10.1016/j.hpb.2019.08.002
Arlt, J., Wei, W., Xie, C., Homeyer, A., Settmacher, U., Dahmen, U., et al. (2017). Modulation of hepatic perfusion did not improve recovery from hepatic outflow obstruction. BMC Pharmacol. Toxicol. 18, 50. doi: 10.1186/s40360-017-0155-4
Armiti-Juber, A., and Ricken, T. (2021). Model order reduction for deformable porous materials in thin domains via asymptotic analysis. Arch. Appl. Mech. 477, 873. doi: 10.1007/s00419-021-01907-3
Asenbaum, U., Kaczirek, K., Ba-Ssalamah, A., Ringl, H., Schwarz, C., Waneck, F., et al. (2018). Post-hepatectomy liver failure after major hepatic surgery: not only size matters. Eur. Radiol. 28, 4748–4756. doi: 10.1007/s00330-018-5487-y
Ashburner, M., Ball, C. A., Blake, J. A., Botstein, D., Butler, H., Cherry, J. M., et al. (2000). Gene ontology: tool for the unification of biology. the gene ontology consortium. Nat. Genet. 25, 25–29. doi: 10.1038/75556
Ateshian, G. A., and Ricken, T. (2010). Multigenerational interstitial growth of biological tissues. Biomech. Model Mechanobiol. 9, 689–702. doi: 10.1007/s10237-010-0205-y
Audebert, C., Bekheit, M., Bucur, P., Vibert, E., and Vignon-Clementel, I. E. (2017). Partial hepatectomy hemodynamics changes: experimental data explained by closed-loop lumped modeling. J. Biomech. 50, 202–208. doi: 10.1016/j.jbiomech.2016.11.037
Bak-Fredslund, K. P., Lykke Eriksen, P., Munk, O. L., Villadsen, G. E., Keiding, S., and Sørensen, M. (2017). Metabolic liver function in humans measured by 2-18f-fluoro-2-deoxy-d-galactose pet/ct-reproducibility and clinical potential. EJNMMI Res. 7, 71. doi: 10.1186/s13550-017-0320-1
Bartels, H., and Hildebrand, F. D. (1975). Infusionsurethrographie beim mann. Helv Chir. Acta 42, 343–345.
Barth, B. K., Fischer, M. A., Kambakamba, P., Lesurtel, M., and Reiner, C. S. (2016). Liver-fat and liver-function indices derived from gd-eob-dtpa-enhanced liver mri for prediction of future liver remnant growth after portal vein occlusion. Eur. J. Radiol. 85, 843–849. doi: 10.1016/j.ejrad.2016.02.008
Bartl, M., Pfaff, M., Ghallab, A., Driesch, D., Henkel, S. G., Hengstler, J. G., et al. (2015). Optimality in the zonation of ammonia detoxification in rodent liver. Arch. Toxicol. 89, 2069–2078. doi: 10.1007/s00204-015-1596-4
Bass, L., Robinson, P., and Bracken, A. J. (1978). Hepatic elimination of flowing substrates: the distributed model. J. Theor. Biol. 72, 161–184. doi: 10.1016/0022-5193(78)90023-1
Bednarsch, J., Menk, M., Malinowski, M., Weber-Carstens, S., Pratschke, J., and Stockmann, M. (2016). (13) c breath tests are feasible in patients with extracorporeal membrane oxygenation devices. Artif. Organs. 40, 692–698. doi: 10.1111/aor.12634
Benfeitas, R., Bidkhori, G., Mukhopadhyay, B., Klevstig, M., Arif, M., Zhang, C., et al. (2019). Characterization of heterogeneous redox responses in hepatocellular carcinoma patients using network analysis. EBioMedicine 40, 471–487. doi: 10.1016/j.ebiom.2018.12.057
Bennink, R. J., Dinant, S., Erdogan, D., Heijnen, B. H., Straatsburg, I. H., van Vliet, A. K., et al. (2004). Preoperative assessment of postoperative remnant liver function using hepatobiliary scintigraphy. J. Nucl. Med. 45, 965–971. Available online at: https://jnm.snmjournals.org/content/jnumed/45/6/965.full.pdf
Berndt, N., Bulik, S., Wallach, I., Wünsch, T., König, M., Stockmann, M., et al. (2018). HEPATOKIN1 is a biochemistry-based model of liver metabolism for applications in medicine and pharmacology. Nat. Commun. 9, 2386. doi: 10.1038/s41467-018-04720-9
Berndt, N., Eckstein, J., Heucke, N., Wuensch, T., Gajowski, R., Stockmann, M., et al. (2021). Metabolic heterogeneity of human hepatocellular carcinoma: implications for personalized pharmacological treatment. FEBS J. 288, 2332–2346. doi: 10.1111/febs.15587
Bidkhori, G., Benfeitas, R., Klevstig, M., Zhang, C., Nielsen, J., Uhlen, M., et al. (2018). Metabolic network-based stratification of hepatocellular carcinoma reveals three distinct tumor subtypes. Proc. Natl. Acad. Sci. U.S.A. 115, E11874–E11883. doi: 10.1073/pnas.1807305115
Björnson, E., Mukhopadhyay, B., Asplund, A., Pristovsek, N., Cinar, R., Romeo, S., et al. (2015). Stratification of hepatocellular carcinoma patients based on acetate utilization. Cell. Rep. 13, 2014–2026. doi: 10.1016/j.celrep.2015.10.045
Blüthner, E., Bednarsch, J., Pape, U.-F., Karber, M., Maasberg, S., Gerlach, U. A., et al. (2020). Advanced liver function assessment in patients with intestinal failure on long-term parenteral nutrition. Clin. Nutr. 39, 540–547. doi: 10.1016/j.clnu.2019.02.039
Boissier, N., Drasdo, D., and Vignon-Clementel, I. E. (2020). Simulation of a detoxifying organ function: focus on hemodynamics modeling and convection-reaction numerical simulation in microcirculatory networks. Int. J. Numer. Methods Biomed. Eng. 37:e3422. doi: 10.1002/cnm.3422
Boleslawski, E., Petrovai, G., Truant, S., Dharancy, S., Duhamel, A., Salleron, J., et al. (2012). Hepatic venous pressure gradient in the assessment of portal hypertension before liver resection in patients with cirrhosis. Br. J. Surg. 99, 855–863. doi: 10.1002/bjs.8753
Bonfiglio, A., Leungchavaphongse, K., Repetto, R., and Siggers, J. H. (2010). Mathematical modeling of the circulation in the liver lobule. J. Biomech. Eng. 132, 111011. doi: 10.1115/1.4002563
Bonfrate, L., Grattagliano, I., Palasciano, G., and Portincasa, P. (2015). Dynamic carbon 13 breath tests for the study of liver function and gastric emptying. Gastroenterol. Rep. 3, 12–21. doi: 10.1093/gastro/gou068
Borger, P., Schneider, M., Frick, L., Langiewicz, M., Sorokin, M., Buzdin, A., et al. (2019). Exploration of the transcriptional landscape of ALPPS reveals the pathways of accelerated liver regeneration. Front. Oncol. 9:1206. doi: 10.3389/fonc.2019.01206
Bracken, M. B. (2009). Why animal studies are often poor predictors of human reactions to exposure. J. R. Soc. Med. 102, 120–122. doi: 10.1258/jrsm.2008.08k033
Buckley, C. D., Tan, J., Anderson, K. L., Hanein, D., Volkmann, N., Weis, W. I., et al. (2014). Cell adhesion. The minimal cadherin-catenin complex binds to actin filaments under force. Science 346, 1254211. doi: 10.1126/science.1254211
Burke, Z. D., and Tosh, D. (2006). The wnt/beta-catenin pathway: master regulator of liver zonation? Bioessays 28, 1072–1077. doi: 10.1002/bies.20485
Byk, K., Jasinski, K., Bartel, Z., Jasztal, A., Sitek, B., Tomanek, B., et al. (2016). Mri-based assessment of liver perfusion and hepatocyte injury in the murine model of acute hepatitis. Magma 29, 789–798. doi: 10.1007/s10334-016-0563-2
Caldez, M. J., van Hul, N., Koh, H. W. L., Teo, X. Q., Fan, J. J., Tan, P. Y., et al. (2018). Metabolic remodeling during liver regeneration. Dev. Cell. 47, 425.e5–438.e5. doi: 10.1016/j.devcel.2018.09.020
Carril, E., Valdecantos, M. P., Lanzón, B., Angulo, S., Valverde, Á. M., Godzien, J., et al. (2020). Metabolic impact of partial hepatectomy in the non-alcoholic steatohepatitis animal model of methionine-choline deficient diet. J. Pharm. Biomed. Anal. 178:112958. doi: 10.1016/j.jpba.2019.112958
Chatzipanagiotou, S., Nath, A., Vogt, B., and Jungermann, K. (1985). Alteration in the capacities as well as in the zonal and cellular distributions of pyruvate kinase l and m2 in regenerating rat liver. Biol. Chem. Hoppe Seyler. 366, 271–280. doi: 10.1515/bchm3.1985.366.1.271
Chen, G., Xia, B., Fu, Q., Huang, X., Wang, F., Chen, Z., et al. (2019). Matrix mechanics as regulatory factors and therapeutic targets in hepatic fibrosis. Int. J. Biol. Sci. 15, 2509–2521. doi: 10.7150/ijbs.37500
Chen, X.-G., and Xu, C.-S. (2014). Proteomic analysis of the regenerating liver following 2/3 partial hepatectomy in rats. Biol. Res. 47:59. doi: 10.1186/0717-6287-47-59
Cheng, Z., Liu, L., Zhang, X.-J., Lu, M., Wang, Y., Assfalg, V., et al. (2018). Peroxisome proliferator-activated receptor gamma negatively regulates liver regeneration after partial hepatectomy via the HGF/c-Met/ERK1/2 pathways. Sci. Rep. 8, 11894. doi: 10.1038/s41598-018-30426-5
Chiba, H., Osanai, M., Murata, M., Kojima, T., and Sawada, N. (2008). Transmembrane proteins of tight junctions. Biochim. Biophys. Acta 1778, 588–600. doi: 10.1016/j.bbamem.2007.08.017
Chiba, M., Yasue, H., and Ohkohchi, N. (2013). Gene expression profiling of sense and antisense transcripts in liver regeneration by microarray analysis. Biomed. Rep. 1, 383–388. doi: 10.3892/br.2013.80
Cho, A., Chung, Y. E., Choi, J. S., Kim, K. S., Choi, G. H., Park, Y. N., et al. (2017). Feasibility of preoperative fdg pet/ct total hepatic glycolysis in the remnant liver for the prediction of postoperative liver function. AJR Am. J. Roentgenol. 208, 624–631. doi: 10.2214/AJR.16.16450
Choi, S. S., Omenetti, A., Witek, R. P., Moylan, C. A., Syn, W.-K., Jung, Y., et al. (2009). Hedgehog pathway activation and epithelial-to-mesenchymal transitions during myofibroblastic transformation of rat hepatic cells in culture and cirrhosis. Am. J. Physiol. Gastrointest Liver Physiol. 297, G1093–G1106. doi: 10.1152/ajpgi.00292.2009
Chouhan, M. D., Mookerjee, R. P., Bainbridge, A., Punwani, S., Jones, H., Davies, N., et al. (2017). Caval subtraction 2d phase-contrast mri to measure total liver and hepatic arterial blood flow: Proof-of-principle, correlation with portal hypertension severity and validation in patients with chronic liver disease. Invest. Radiol. 52, 170–176. doi: 10.1097/RLI.0000000000000328
Choukèr, A., Schachtner, T., Schauer, R., Dugas, M., Löhe, F., Martignoni, A., et al. (2004). Effects of pringle manoeuvre and ischaemic preconditioning on haemodynamic stability in patients undergoing elective hepatectomy: a randomized trial. Br. J. Anaesth. 93, 204–211. doi: 10.1093/bja/aeh195
Christ, B., Dahmen, U., Herrmann, K.-H., König, M., Reichenbach, J. R., Ricken, T., et al. (2017). Computational modeling in liver surgery. Front. Physiol. 8:906. doi: 10.3389/fphys.2017.00906
Chuang, Y.-H., Ou, H.-Y., Lazo, M. Z., Chen, C.-L., Chen, M.-H., Weng, C.-C., et al. (2018). Predicting post-hepatectomy liver failure by combined volumetric, functional mr image and laboratory analysis. Liver Int. 38, 868–874. doi: 10.1111/liv.13608
Cimica, V., Batusic, D., Haralanova-Ilieva, B., Chen, Y., Hollemann, T., Pieler, T., et al. (2007). Serial analysis of gene expression (SAGE) in rat liver regeneration. Biochem. Biophys. Res. Commun. 360, 545–552. doi: 10.1016/j.bbrc.2007.06.039
Colak, D., Al-Harazi, O., Mustafa, O. M., Meng, F., Assiri, A. M., Dhar, D. K., et al. (2020). RNA-Seq transcriptome profiling in three liver regeneration models in rats: comparative analysis of partial hepatectomy, ALLPS, and PVL. Sci. Rep. 10, 5213. doi: 10.1038/s41598-020-61826-1
Cucchetti, A., Cescon, M., Golfieri, R., Piscaglia, F., Renzulli, M., Neri, F., et al. (2016). Hepatic venous pressure gradient in the preoperative assessment of patients with resectable hepatocellular carcinoma. J Hepatol. 64, 79–86. doi: 10.1016/j.jhep.2015.08.025
Dahmen, U., Hall, C. A., Madrahimov, N., Milekhin, V., and Dirsch, O. (2007). Regulation of hepatic microcirculation in stepwise liver resection. Acta Gastroenterol. Belg. 70, 345–351.
Damrauer, S. M., Studer, P., da Silva, C. G., Longo, C. R., Ramsey, H. E., Csizmadia, E., et al. (2011). A20 modulates lipid metabolism and energy production to promote liver regeneration. PLoS ONE 6:e17715. doi: 10.1371/journal.pone.0017715
d'Assignies, G., Fayard, C., Leitao, H., Alfaiate, T., Tubach, F., Dokmak, S., et al. (2016). Liver steatosis assessed by preoperative MRI: An independent risk factor for severe complications after major hepatic resection. Surgery 159, 1050–1057. doi: 10.1016/j.surg.2015.10.008
de Graaf, W., van Lienden, K. P., van Gulik, T. M., and Bennink, R. J. (2010). (99m)tc-mebrofenin hepatobiliary scintigraphy with spect for the assessment of hepatic function and liver functional volume before partial hepatectomy. J. Nucl Med. 51, 229–236. doi: 10.2967/jnumed.109.069724
de Rudder, M., Dili, A., Stärkel, P., and Leclercq, I. A. (2021). Critical role of lsec in post-hepatectomy liver regeneration and failure. Int. J. Mol. Sci. 22:8053. doi: 10.3390/ijms22158053
Deal, R., Frederiks, C., Williams, L., Olthof, P. B., Dirscherl, K., Keutgen, X., et al. (2018). Rapid liver hypertrophy after portal vein occlusion correlates with the degree of collateralization between lobes—a study in pigs. J. Gastrointest Surg. 22, 203–213. doi: 10.1007/s11605-017-3512-0
Debbaut, C., De Wilde, D., Casteleyn, C., Cornillie, P., Van Loo, D., Van Hoorebeke, L., et al. (2012). Modeling the impact of partial hepatectomy on the hepatic hemodynamics using a rat model. IEEE Trans. Biomed. Eng. 59, 3293–3303. doi: 10.1109/TBME.2012.2199108
Debbaut, C., Vierendeels, J., Siggers, J. H., Repetto, R., Monbaliu, D., and Segers, P. (2014). A 3d porous media liver lobule model: the importance of vascular septa and anisotropic permeability for homogeneous perfusion. Comput. Methods Biomech. Biomed. Eng. 17, 1295–1310. doi: 10.1080/10255842.2012.744399
Degasperi, A., Birtwistle, M. R., Volinsky, N., Rauch, J., Kolch, W., and Kholodenko, B. N. (2014). Evaluating strategies to normalise biological replicates of western blot data. PLoS ONE 9:e87293. doi: 10.1371/journal.pone.0087293
Diaz Ochoa, J. G., Bucher, J., Péry, A. R. R., Zaldivar Comenges, J. M., Niklas, J., and Mauch, K. (2012). A multi-scale modeling framework for individualized, spatiotemporal prediction of drug effects and toxicological risk. Front. Pharmacol. 3:204. doi: 10.3389/fphar.2012.00204
Dinant, S., de Graaf, W., Verwer, B. J., Bennink, R. J., van Lienden, K. P., Gouma, D. J., et al. (2007). Risk assessment of posthepatectomy liver failure using hepatobiliary scintigraphy and ct volumetry. J. Nucl Med. 48, 685–692. doi: 10.2967/jnumed.106.038430
Dirsch, O., Madrahimov, N., Chaudri, N., Deng, M., Madrahimova, F., Schenk, A., et al. (2008a). ERRATUM. Transplantation 85, 1866.
Dirsch, O., Madrahimov, N., Chaudri, N., Deng, M., Madrahimova, F., Schenk, A., et al. (2008b). Recovery of liver perfusion after focal outflow obstruction and liver resection. Transplantation 85, 748–756. doi: 10.1097/TP.0b013e31816631f9
Droin, C., Kholtei, J. E., Bahar Halpern, K., Hurni, C., Rozenberg, M., Muvkadi, S., et al. (2021). Space-time logic of liver gene expression at sub-lobular scale. Nat. Metab 3, 43–58. doi: 10.1038/s42255-020-00323-1
Du, K., Hyun, J., Premont, R. T., Choi, S. S., Michelotti, G. A., Swiderska-Syn, M., et al. (2018). Hedgehog-yap signaling pathway regulates glutaminolysis to control activation of hepatic stellate cells. Gastroenterology 154, 1465.e13–1479.e13. doi: 10.1053/j.gastro.2017.12.022
Dupont, S., Morsut, L., Aragona, M., Enzo, E., Giulitti, S., Cordenonsi, M., et al. (2011). Role of YAP/TAZ in mechanotransduction. Nature 474, 179–183. doi: 10.1038/nature10137
Edginton, A. N., and Willmann, S. (2008). Physiology-based simulations of a pathological condition: prediction of pharmacokinetics in patients with liver cirrhosis. Clin. Pharmacokinet 47, 743–752. doi: 10.2165/00003088-200847110-00005
Eipel, C., Abshagen, K., Ritter, J., Cantré, D., Menger, M. D., and Vollmar, B. (2010). Splenectomy improves survival by increasing arterial blood supply in a rat model of reduced-size liver. Transpl. Int. 23, 998–1007. doi: 10.1111/j.1432-2277.2010.01079.x
Elosegui-Artola, A., Trepat, X., and Roca-Cusachs, P. (2018). Control of mechanotransduction by molecular clutch dynamics. Trends Cell. Biol. 28, 356–367. doi: 10.1016/j.tcb.2018.01.008
Englert, F. A., Seidel, R. A., Galler, K., Gouveia, Z., Soares, M. P., Neugebauer, U., et al. (2019). Labile heme impairs hepatic microcirculation and promotes hepatic injury. Arch. Biochem. Biophys. 672:108075. doi: 10.1016/j.abb.2019.108075
Erdogan, D., Heijnen, B. H. M., Bennink, R. J., Kok, M., Dinant, S., Straatsburg, I. H., et al. (2004). Preoperative assessment of liver function: a comparison of 99mtc-mebrofenin scintigraphy with indocyanine green clearance test. Liver Int. 24, 117–123. doi: 10.1111/j.1478-3231.2004.00901.x
Espié, P., Tytgat, D., Sargentini-Maier, M.-L., Poggesi, I., and Watelet, J.-B. (2009). Physiologically based pharmacokinetics (pbpk). Drug Metab. Rev. 41, 391–407. doi: 10.1080/10837450902891360
Ferraioli, G. (2019). Review of liver elastography guidelines. J. Ultrasound Med. 38, 9–14. doi: 10.1002/jum.14856
Filmann, N., Walter, D., Schadde, E., Bruns, C., Keck, T., Lang, H., et al. (2019). Mortality after liver surgery in Germany. Br. J. Surg. 106, 1523–1529. doi: 10.1002/bjs.11236
Frydrychowicz, A., Roldan-Alzate, A., Winslow, E., Consigny, D., Campo, C. A., Motosugi, U., et al. (2017). Comparison of radial 4D Flow-MRI with perivascular ultrasound to quantify blood flow in the abdomen and introduction of a porcine model of pre-hepatic portal hypertension. Eur. Radiol. 27, 5316–5324. doi: 10.1007/s00330-017-4862-4
Fu, X., Sluka, J. P., Clendenon, S. G., Dunn, K. W., Wang, Z., Klaunig, J. E., et al. (2018). Modeling of xenobiotic transport and metabolism in virtual hepatic lobule models. PLoS ONE 13:e0198060. doi: 10.1371/journal.pone.0198060
Fujimoto, K., Noda, Y., Kawai, N., Kajita, K., Akamine, Y., Kawada, H., et al. (2021). Comparison of mono-exponential, bi-exponential, and stretched exponential diffusion-weighted mr imaging models in differentiating hepatic hemangiomas from liver metastases. Eur. J. Radiol. 141:109806. doi: 10.1016/j.ejrad.2021.109806
García-Pérez, R., Revilla-Nuin, B., Martínez, C. M., Bernabé-García, A., Baroja Mazo, A., and Parrilla Paricio, P. (2015). Associated liver partition and portal vein ligation (ALPPS) vs selective portal vein ligation (PVL) for staged hepatectomy in a rat model. Similar regenerative response? PLoS ONE 10:e0144096. doi: 10.1371/journal.pone.0144096
Gaub, J., and Iversen, J. (1984). Rat Liver Regeneration After 90% Partial Hepatectomy. Hepatology 4, 902–904. doi: 10.1002/hep.1840040519
Gebhardt, R., Baldysiak-Figiel, A., Krügel, V., Ueberham, E., and Gaunitz, F. (2007). Hepatocellular expression of glutamine synthetase: an indicator of morphogen actions as master regulators of zonation in adult liver. Prog. Histochem. Cytochem. 41, 201–266. doi: 10.1016/j.proghi.2006.12.001
Geisel, D., Raabe, P., Lüdemann, L., Malinowski, M., Stockmann, M., Seehofer, D., et al. (2017). Gd-eob-dtpa-enhanced mri for monitoring future liver remnant function after portal vein embolization and extended hemihepatectomy: a prospective trial. Eur. Radiol. 27, 3080–3087. doi: 10.1007/s00330-016-4674-y
Gérard, C., and Goldbeter, A. (2014). The balance between cell cycle arrest and cell proliferation: control by the extracellular matrix and by contact inhibition. Interface Focus. 4, 20130075. doi: 10.1098/rsfs.2013.0075
Ghibellini, G., Leslie, E. M., Pollack, G. M., and Brouwer, K. L. R. (2008). Use of tc-99m mebrofenin as a clinical probe to assess altered hepatobiliary transport: integration of in vitro, pharmacokinetic modeling, and simulation studies. Pharm. Res. 25, 1851–1860. doi: 10.1007/s11095-008-9597-0
Gille, C., Bölling, C., Hoppe, A., Bulik, S., Hoffmann, S., Hübner, K., et al. (2010). HepatoNet1: a comprehensive metabolic reconstruction of the human hepatocyte for the analysis of liver physiology. Mol. Syst. Biol. 6, 411. doi: 10.1038/msb.2010.62
Golse, N., Joly, F., Combari, P., Lewin, M., Nicolas, Q., Audebert, C., et al. (2021). Predicting the risk of post-hepatectomy portal hypertension using a digital twin: a clinical proof of concept. J. Hepatol. 74, 661–669. doi: 10.1016/j.jhep.2020.10.036
Goresky, C. A., Bach, G. G., and Nadeau, B. E. (1973). On the uptake of materials by the intact liver. the transport and net removal of galactose. J. Clin. Invest. 52, 991–1009. doi: 10.1172/JCI107300
Gouya, H., Grabar, S., Vignaux, O., Saade, A., Pol, S., Legmann, P., et al. (2016). Portal hypertension in patients with cirrhosis: indirect assessment of hepatic venous pressure gradient by measuring azygos flow with 2d-cine phase-contrast magnetic resonance imaging. Eur. Radiol. 26, 1981–1990. doi: 10.1007/s00330-015-3991-x
Gray, M. R., and Tam, Y. K. (1987). The series-compartment model for hepatic elimination. Drug Metab. Dispos. 15, 27–31.
Guberman, E., Sherief, H., and Regan, E. R. (2020). Boolean model of anchorage dependence and contact inhibition points to coordinated inhibition but semi-independent induction of proliferation and migration. Comput. Struct. Biotechnol. J. 18, 2145–2165. doi: 10.1016/j.csbj.2020.07.016
Guo, F., Nian, H., Zhang, H., Huang, L., Tang, Y., Xiao, X., et al. (2006). Proteomic analysis of the transition from quiescent to proliferating stages in rat liver hepatectomy model. Proteomics 6, 3075–3086. doi: 10.1002/pmic.200500322
Gupta, M., Choudhury, P. S., Singh, S., and Hazarika, D. (2018). Liver functional volumetry by Tc-99m mebrofenin hepatobiliary scintigraphy before major liver resection: a game changer. Indian J. Nucl. Med. 33, 277–283. doi: 10.4103/ijnm.IJNM_72_18
Gutiérrez-Salinas, J., Miranda-Gardu no, L., Trejo-Izquierdo, E., Díaz-Mu noz, M., Vidrio, S., Morales-González, J. A., et al. (1999). Redox state and energy metabolism during liver regeneration: alterations produced by acute ethanol administration. Biochem. Pharmacol. 58, 1831–1839. doi: 10.1016/S0006-2952(99)00261-0
Haegele, S., Reiter, S., Wanek, D., Offensperger, F., Pereyra, D., Stremitzer, S., et al. (2016). Perioperative non-invasive indocyanine green-clearance testing to predict postoperative outcome after liver resection. PLoS ONE 11:e0165481. doi: 10.1371/journal.pone.0165481
Hailfinger, S., Jaworski, M., Braeuning, A., Buchmann, A., and Schwarz, M. (2006). Zonal gene expression in murine liver: lessons from tumors. Hepatology 43, 407–414. doi: 10.1002/hep.21082
Halder, G., Dupont, S., and Piccolo, S. (2012). Transduction of mechanical and cytoskeletal cues by yap and taz. Nat. Rev. Mol. Cell. Biol. 13, 591–600. doi: 10.1038/nrm3416
Halpern, K. B., Shenhav, R., Matcovitch-Natan, O., Toth, B., Lemze, D., Golan, M., et al. (2017). Single-cell spatial reconstruction reveals global division of labour in the mammalian liver. Nature 542, 352–356. doi: 10.1038/nature21065
Hamano, M., Ezaki, H., Kiso, S., Furuta, K., Egawa, M., Kizu, T., et al. (2014). Lipid overloading during liver regeneration causes delayed hepatocyte dna replication by increasing er stress in mice with simple hepatic steatosis. J. Gastroenterol. 49, 305–316. doi: 10.1007/s00535-013-0780-7
Hemming, A. W., Scudamore, C. H., Shackleton, C. R., Pudek, M., and Erb, S. R. (1992). Indocyanine green clearance as a predictor of successful hepatic resection in cirrhotic patients. Am. J. Surg. 163, 515–518. doi: 10.1016/0002-9610(92)90400-L
Hendrikse, N. H., Kuipers, F., Meijer, C., Havinga, R., Bijleveld, C. M. A., van der Graaf, W. T. A., et al. (2004). In vivo imaging of hepatobiliary transport function mediated by multidrug resistance associated protein and p-glycoprotein. Cancer Chemother. Pharmacol. 54, 131–138. doi: 10.1007/s00280-004-0793-2
Heucke, N., Wuensch, T., Mohr, J., Kaffarnik, M., Arsenic, R., Sinn, B., et al. (2019). Non-invasive structure-function assessment of the liver by 2d time-harmonic elastography and the dynamic liver maximum capacity (limax) test. J. Gastroenterol. Hepatol. 34, 1611–1619. doi: 10.1111/jgh.14629
Higgins, G. M. (1931). Experimental pathology of the liver. i. restoration of the liver of the white rat following partial surgical removal. Arch. Pathol. 12, 186–202.
Hintermann, E., and Christen, U. (2019). The many roles of cell adhesion molecules in hepatic fibrosis. Cells 8, 1503. doi: 10.3390/cells8121503
Ho, C.-M., Lin, R.-K., Tsai, S.-F., Hu, R.-H., Liang, P.-C., Sheu, T. W.-H., et al. (2010). Simulation of portal hemodynamic changes in a donor after right hepatectomy. J. Biomech. Eng. 132, 041002. doi: 10.1115/1.4000957
Ho, H., Sorrell, K., Bartlett, A., and Hunter, P. (2012). Blood flow simulation for the liver after a virtual right lobe hepatectomy. Medical image computing and computer-assisted intervention : MICCAI … Int. Conf. Med. Image Comput. Comput. Assist. Interv. 15(Pt 3):525–532. doi: 10.1007/978-3-642-33454-2_65
Ho, H., and Zhang, E. (2020). Virtual lobule models are the key for multiscale biomechanical and pharmacological modeling for the liver. Front. Physiol. 11:1061. doi: 10.3389/fphys.2020.01061
Hoehme, S., Gebhardt, R., Hengstler, J. G., and Drasdo, D. (2020). Model predicts fundamental role of biomechanical control of cell cycle progression during liver regeneration after partial hepatectomy. arXiv:2005.09151. Available online at: https://arxiv.org/abs/2005.09151
Hossain, M. A., Izuishi, K., and Maeta, H. (2003). Effect of short-term administration of prostaglandin E1 on viability after ischemia/reperfusion injury with extended hepatectomy in cirrhotic rat liver. World J. Surg. 27, 1155–1160. doi: 10.1007/s00268-003-6914-y
Hou, C.-T., Chen, Y.-L., Lin, C.-C., Chou, C.-T., Lin, K.-H., Lin, P.-Y., et al. (2018). Portal venous velocity affects liver regeneration after right lobe living donor hepatectomy. PLoS ONE 13:e0204163. doi: 10.1371/journal.pone.0204163
Hu, J., Lü, S., Feng, S., and Long, M. (2017a). Flow dynamics analyses of pathophysiological liver lobules using porous media theory. Acta Mech. Sin. 33, 823–832. doi: 10.1007/s10409-017-0674-7
Hu, Y., Shin, D.-J., Pan, H., Lin, Z., Dreyfuss, J. M., Camargo, F. D., et al. (2017b). Yap suppresses gluconeogenic gene expression through pgc1α. Hepatology 66, 2029–2041. doi: 10.1002/hep.29373
Huang, H., Deng, M., Jin, H., Liu, A., Dahmen, U., and Dirsch, O. (2014). Reduced hepatic arterial perfusion impairs the recovery from focal hepatic venous outflow obstruction in liver-resected rats. Transplantation 97, 1009–1018. doi: 10.1097/TP.0000000000000089
Huang, H., Deng, M., Jin, H., Liu, A., Dirsch, O., and Dahmen, U. (2011). Hepatic arterial perfusion is essential for the spontaneous recovery from focal hepatic venous outflow obstruction in rats. Am. J. Transplant. 11, 2342–2352. doi: 10.1111/j.1600-6143.2011.03682.x
Hucka, M., Bergmann, F. T., Chaouiya, C., Dräger, A., Hoops, S., Keating, S. M., et al. (2019). The systems biology markup language (sbml): language specification for level 3 version 2 core release 2. J. Integr. Bioinform. 16:20190021. doi: 10.1515/jib-2019-0021
Hyötyläinen, T., Jerby, L., Petäjä, E. M., Mattila, I., Jäntti, S., Auvinen, P., et al. (2016). Genome-scale study reveals reduced metabolic adaptability in patients with non-alcoholic fatty liver disease. Nat. Commun. 7:8994. doi: 10.1038/ncomms9994
Iimuro, Y., Kondo, Y., Suzumura, K., Uyama, N., Asano, Y., Hirano, T., et al. (2013). Regional hepatic regeneration after liver resection correlates well with preceding changes in the regional portal circulation in humans. Dig. Dis. Sci. 58, 3001–3009. doi: 10.1007/s10620-013-2756-0
Ishikawa, J., Takeo, M., Iwadate, A., Koya, J., Kihira, M., Oshima, M., et al. (2021). Mechanical homeostasis of liver sinusoid is involved in the initiation and termination of liver regeneration. Commun. Biol. 4, 409. doi: 10.1038/s42003-021-01936-2
Isogai, T., Park, J. S., and Danuser, G. (2017). Cell forces meet cell metabolism. Nat. Cell Biol. 19, 591–593. doi: 10.1038/ncb3542
Itoh, S., Yoshizumi, T., Shirabe, K., Kimura, K., Okabe, H., Harimoto, N., et al. (2017). Functional remnant liver assessment predicts liver-related morbidity after hepatic resection in patients with hepatocellular carcinoma. Hepatol. Res. 47, 398–404. doi: 10.1111/hepr.12761
Iwasaki, J., Afify, M., Bleilevens, C., Klinge, U., Weiskirchen, R., Steitz, J., et al. (2019). The impact of a nitric oxide synthase inhibitor (L-NAME) on ischemia−reperfusion injury of cholestatic livers by pringle maneuver and liver resection after bile duct ligation in rats. Int. J. Mol. Sci. 20, E2114. doi: 10.3390/ijms20092114
Jahng, G.-H., Li, K.-L., Ostergaard, L., and Calamante, F. (2014). Perfusion magnetic resonance imaging: a comprehensive update on principles and techniques. Korean J. Radiol. 15, 554–577. doi: 10.3348/kjr.2014.15.5.554
Jang, S., Lee, J. M., Lee, D. H., Joo, I., Yoon, J. H., Chang, W., et al. (2017). Value of mr elastography for the preoperative estimation of liver regeneration capacity in patients with hepatocellular carcinoma. J. Magn. Reson. Imaging 45, 1627–1636. doi: 10.1002/jmri.25517
Jansen, M., ten Klooster, J. P., Offerhaus, G. J., and Clevers, H. (2009). Lkb1 and ampk family signaling: the intimate link between cell polarity and energy metabolism. Physiol. Rev. 89, 777–798. doi: 10.1152/physrev.00026.2008
Jara, M., Reese, T., Malinowski, M., Valle, E., Seehofer, D., Puhl, G., et al. (2015). Reductions in post-hepatectomy liver failure and related mortality after implementation of the limax algorithm in preoperative work-up: a single-centre analysis of 1170 hepatectomies of one or more segments. HPB 17, 651–658. doi: 10.1111/hpb.12424
Jepsen, B. N., Andersen, K. J., Knudsen, A. R., Nyengaard, J. R., Hamilton-Dutoit, S., Svendsen, P., et al. (2015). Anti-inflammatory liposomes have no impact on liver regeneration in rats. Ann. Med. Surg. 4, 452–461. doi: 10.1016/j.amsu.2015.10.018
Johnson, S. P., Ramasawmy, R., Campbell-Washburn, A. E., Wells, J. A., Robson, M., Rajkumar, V., et al. (2016). Acute changes in liver tumour perfusion measured non-invasively with arterial spin labelling. Br. J. Cancer 114, 897–904. doi: 10.1038/bjc.2016.51
Jones, H., and Rowland-Yeo, K. (2013). Basic concepts in physiologically based pharmacokinetic modeling in drug discovery and development. CPT Pharmacometrics Syst. Pharmacol. 2:e63. doi: 10.1038/psp.2013.41
Jung, Y. S., Kim, S. J., Kwon, D. Y., Jun, D. S., and Kim, Y. C. (2013). Significance of alterations in the metabolomics of sulfur-containing amino acids during liver regeneration. Biochimie 95, 1605–1610. doi: 10.1016/j.biochi.2013.04.015
Jungermann, K., and Kietzmann, T. (2000). Oxygen: modulator of metabolic zonation and disease of the liver. Hepatology 31, 255–260. doi: 10.1002/hep.510310201
Kachaylo, E., Tschuor, C., Calo, N., Borgeaud, N., Ungethüm, U., Limani, P., et al. (2017). PTEN down-regulation promotes β-oxidation to fuel hypertrophic liver growth after hepatectomy in mice. Hepatology 66, 908–921. doi: 10.1002/hep.29226
Kahn, D., van Hoorn-Hickman, R., and Terblanche, J. (1984). Liver blood flow after partial hepatectomy in the pig. J. Surg. Res. 37, 290–294. doi: 10.1016/0022-4804(84)90191-4
Kanehisa, M., and Goto, S. (2000). Kegg: kyoto encyclopedia of genes and genomes. Nucleic Acids Res. 28, 27–30. doi: 10.1093/nar/28.1.27
Kang, N. (2020). Mechanotransduction in liver diseases. Semin Liver Dis. 40, 84–90. doi: 10.1055/s-0039-3399502
Kawaguchi, D., Hiroshima, Y., Kumamoto, T., Mori, R., Matsuyama, R., Ichikawa, Y., et al. (2019). Effect of portal vein ligation plus venous congestion on liver regeneration in rats. Ann. Hepatol. 18, 89–100. doi: 10.5604/01.3001.0012.7866
Kawasaki, T., Moriyasu, F., Kimura, T., Someda, H., Fukuda, Y., and Ozawa, K. (1991). Changes in portal blood flow consequent to partial hepatectomy: doppler estimation. Radiology 180, 373–377. doi: 10.1148/radiology.180.2.2068298
Keating, S. M., Waltemath, D., König, M., Zhang, F., Dräger, A., Chaouiya, C., Bergmann, F. T., et al. (2020). SBML Level 3: an extensible format for the exchange and reuse of biological models. Mol. Syst. Biol. 16:e9110. doi: 10.15252/msb.20199110
Keiding, S., Sørensen, M., Frisch, K., Gormsen, L. C., and Munk, O. L. (2018). Quantitative PET of liver functions. Am. J. Nucl Med. Mol. Imaging 8, 73–85.
Kietzmann, T. (2017). Metabolic zonation of the liver: the oxygen gradient revisited. Redox. Biol. 11, 622–630. doi: 10.1016/j.redox.2017.01.012
Kim, D. K., Choi, J.-I., Choi, M. H., Park, M. Y., Lee, Y. J., Rha, S. E., et al. (2018). Prediction of posthepatectomy liver failure: Mri with hepatocyte-specific contrast agent versus indocyanine green clearance test. AJR Am. J. Roentgenol. 211, 580–587. doi: 10.2214/AJR.17.19206
Kin, Y., Nimura, Y., Hayakawa, N., Kamiya, J., Kondo, S., Nagino, M., et al. (1994). Doppler analysis of hepatic blood flow predicts liver dysfunction after major hepatectomy. World J. Surg. 18, 143–149. doi: 10.1007/BF00348207
Kirch, J., Thomaseth, C., Jensch, A., and Radde, N. E. (2016). The effect of model rescaling and normalization on sensitivity analysis on an example of a mapk pathway model. EPJ Nonlinear Biomed. Phys. 4, e107165. doi: 10.1140/epjnbp/s40366-016-0030-z
Kiseleva, Y. V., Antonyan, S. Z., Zharikova, T. S., Tupikin, K. A., Kalinin, D. V., and Zharikov, Y. O. (2021). Molecular pathways of liver regeneration: a comprehensive review. World J. Hepatol. 13, 270–290. doi: 10.4254/wjh.v13.i3.270
Kolbe, E., Aleithe, S., Rennert, C., Spormann, L., Ott, F., Meierhofer, D., et al. (2019). Mutual zonated interactions of wnt and hh signaling are orchestrating the metabolism of the adult liver in mice and human. Cell. Rep. 29, 4553.e7–4567.e7. doi: 10.1016/j.celrep.2019.11.104
Köller, A., Grzegorzewski, J., and König, M. (2021a). Physiologically based modeling of the effect of physiological and anthropometric variability on indocyanine green based liver function tests. bioRxiv. doi: 10.1101/2021.08.11.455999
Köller, A., Grzegorzewski, J., Tautenhahn, H.-M., and König, M. (2021b). Prediction of survival after partial hepatectomy using a physiologically based pharmacokinetic model of indocyanine green liver function tests. bioRxiv. doi: 10.1101/2021.06.15.448411
König, M., Bulik, S., and Holzhütter, H.-G. (2012). Quantifying the contribution of the liver to glucose homeostasis: a detailed kinetic model of human hepatic glucose metabolism. PLoS Comput. Biol. 8:e1002577. doi: 10.1371/journal.pcbi.1002577
König, M., Grzegorzewski, J., Golebiewski, M., Hermjakob, H., Hucka, M., Olivier, B., et al. (2021). Ten simple rules for sharing experimental and clinical data with the modeling community. Preprints. doi: 10.20944/preprints202108.0303.v1
König, M., and Holzhütter, H.-G. (2012). Kinetic modeling of human hepatic glucose metabolism in type 2 diabetes mellitus predicts higher risk of hypoglycemic events in rigorous insulin therapy. J. Biol. Chem. 287, 36978–36989. doi: 10.1074/jbc.M112.382069
Kreutz, C., Bartolome Rodriguez, M. M., Maiwald, T., Seidl, M., Blum, H. E., Mohr, L., et al. (2007). An error model for protein quantification. Bioinformatics 23, 2747–2753. doi: 10.1093/bioinformatics/btm397
Kruepunga, N., Hakvoort, T. B. M., Hikspoors, J. P. J. M., Köhler, S. E., and Lamers, W. H. (2019). Anatomy of rodent and human livers: what are the differences? Biochim. Biophys. Acta Mol. Basis Dis. 1865, 869–878. doi: 10.1016/j.bbadis.2018.05.019
Kubota, K. (1997). Measurement of liver volume and hepatic functional reserve as a guide to decision-making in resectional surgery for hepatic tumors. Hepatology 26, 1176–1181. doi: 10.1053/jhep.1997.v26.pm0009362359
Kumar, S., Zou, Y., Bao, Q., Wang, M., and Dai, G. (2013). Proteomic analysis of immediate-early response plasma proteins after 70% and 90% partial hepatectomy. Hepatol. Res. 43, 876–889. doi: 10.1111/hepr.12030
Labibi, B., Bashkurov, M., Wrana, J. L., and Attisano, L. (2020). Modeling the control of TGF-β/Smad nuclear accumulation by the hippo pathway effectors, Taz/Yap. iScience 23, 101416. doi: 10.1016/j.isci.2020.101416
Lafontan, M., Barbe, P., Galitzky, J., Tavernier, G., Langin, D., Carpéné, C., et al. (1997). Adrenergic regulation of adipocyte metabolism. Hum. Reprod. 12(Suppl. 1):6–20. doi: 10.1093/humrep/12.suppl_1.6
Lagneau, F., Marty, J., Beyne, P., and Tod, M. (2005). Physiological modeling for indirect evaluation of drug tissular pharmacokinetics under non-steady-state conditions: an example of antimicrobial prophylaxis during liver surgery. J. Pharmacokinet Pharmacodyn. 32, 1–32. doi: 10.1007/s10928-005-2101-0
Lai, H.-S., Chen, Y., Lin, W.-H., Chen, C.-N., Wu, H.-C., Chang, C.-J., et al. (2005). Quantitative gene expression analysis by cDNA microarray during liver regeneration after partial hepatectomy in rats. Surg. Today 35, 396–403. doi: 10.1007/s00595-004-2962-7
Lautt, W. W. (1981). Evaluation of surgical denervation of the liver in cats. Can. J. Physiol. Pharmacol. 59, 1013–1016. doi: 10.1139/y81-154
Lautt, W. W. (2007). Regulatory processes interacting to maintain hepatic blood flow constancy: vascular compliance, hepatic arterial buffer response, hepatorenal reflex, liver regeneration, escape from vasoconstriction. Hepatol. Res. 37, 891–903. doi: 10.1111/j.1872-034X.2007.00148.x
Lee, D. H., Lee, J. M., Yi, N.-J., Lee, K.-W., Suh, K.-S., Lee, J.-H., et al. (2017). Hepatic stiffness measurement by using mr elastography: prognostic values after hepatic resection for hepatocellular carcinoma. Eur. Radiol. 27, 1713–1721. doi: 10.1007/s00330-016-4499-8
Lee, H. T., Park, S. W., Kim, M., and D'Agati, V. D. (2009). Acute kidney injury after hepatic ischemia and reperfusion injury in mice. Lab Invest., 89, 196–208. doi: 10.1038/labinvest.2008.124
Leporq, B., Daire, J.-L., Pastor, C. M., Deltenre, P., Sempoux, C., Schmidt, S., et al. (2018). Quantification of hepatic perfusion and hepatocyte function with dynamic gadoxetic acid-enhanced mri in patients with chronic liver disease. Clin. Sci. 132, 813–824. doi: 10.1042/CS20171131
Leung, J. C.-F., Loong, T. C.-W., Pang, J., Wei, J. L., and Wong, V. W.-S. (2018). Invasive and non-invasive assessment of portal hypertension. Hepatol. Int. 12(Suppl. 1):44–55. doi: 10.1007/s12072-017-9795-0
Li, B., Zhu, Y., Xie, L., Hu, S., Liu, S., and Jiang, X. (2018a). Portal vein ligation alters coding and noncoding gene expression in rat livers. Biochem. Cell. Biol. 96, 1–10. doi: 10.1139/bcb-2017-0070
Li, C. X., Chen, L. L., Li, X. C., Ng, K. T.-P., Yang, X. X., Lo, C. M., et al. (2018b). ApoA-1 accelerates regeneration of small-for-size fatty liver graft after transplantation. Life Sci. 215, 128–135. doi: 10.1016/j.lfs.2018.10.053
Li, C. X., Wang, H. W., Jiang, W. J., Li, G. C., Zhang, Y. D., Luo, C. H., et al. (2020a). The Inhibition of aldose reductase accelerates liver regeneration through regulating energy metabolism. Oxid. Med. Cell. Longev. 2020:3076131. doi: 10.1155/2020/3076131
Li, J., Li, X., Xu, W., Wang, S., Hu, Z., Zhang, Q., et al. (2015). Antifibrotic effects of luteolin on hepatic stellate cells and liver fibrosis by targeting AKT/mTOR/p70S6K and TGFβ/Smad signalling pathways. Liver Int. 35, 1222–1233. doi: 10.1111/liv.12638
Li, W., Li, P., Li, N., Du, Y., Lü, S., Elad, D., et al. (2020b). Matrix stiffness and shear stresses modulate hepatocyte functions in a fibrotic liver sinusoidal model. Am. J. Physiol. Gastrointest. Liver Physiol. doi: 10.1152/ajpgi.00379.2019. [Epub ahead of print].
Lin, C.-W., Chen, Y.-S., Lin, C.-C., Chen, Y.-J., Lo, G.-H., Lee, P.-H., et al. (2015). Amiodarone as an autophagy promoter reduces liver injury and enhances liver regeneration and survival in mice after partial hepatectomy. Sci. Rep. 5:15807. doi: 10.1038/srep15807
Lin, Z., Chen, R., Gao, B., Qin, S., Wu, B., Liu, J., et al. (2021). A highly parallel simulation of patient-specific hepatic flows. Int. J. Numer. Method Biomed. Eng. 37:e3451. doi: 10.1002/cnm.3451
Liska, V., Slowik, P., Eggenhofer, E., Treska, V., Renner, P., Popp, F. C., et al. (2009). Intraportal injection of porcine multipotent mesenchymal stromal cells augments liver regeneration after portal vein embolization. In Vivo 23, 229–235. Available online at: https://iv.iiarjournals.org/content/23/2/229
Liu, H.-X., Fang, Y., Hu, Y., Gonzalez, F. J., Fang, J., and Wan, Y.-J. Y. (2013). PPARβ regulates liver regeneration by modulating Akt and E2f signaling. PLoS ONE 8:e65644. doi: 10.1371/journal.pone.0065644
Liu, Q., Pu, S., Chen, L., Shen, J., Cheng, S., Kuang, J., et al. (2019). Liver-specific Sirtuin6 ablation impairs liver regeneration after 2/3 partial hepatectomy. Wound. Repair. Regen. 27, 366–374. doi: 10.1111/wrr.12703
Liu, X., Jiang, H., Chen, J., Zhou, Y., Huang, Z., and Song, B. (2017). Gadoxetic acid disodium-enhanced magnetic resonance imaging outperformed multidetector computed tomography in diagnosing small hepatocellular carcinoma: a meta-analysis. Liver Transpl. 23, 1505–1518. doi: 10.1002/lt.24867
Lock, J. F., Malinowski, M., Seehofer, D., Hoppe, S., Röhl, R. I., Niehues, S. M., et al. (2012). Function and volume recovery after partial hepatectomy: influence of preoperative liver function, residual liver volume, and obesity. Langenbecks Arch. Surg. 397, 1297–1304. doi: 10.1007/s00423-012-0972-2
Love, M. I., Huber, W., and Anders, S. (2014). Moderated estimation of fold change and dispersion for rna-seq data with deseq2. Genome Biol. 15, 550. doi: 10.1186/s13059-014-0550-8
Lu, T., Hao, J., Shen, C., Gu, G., Zhang, J., and Xu, N. (2018). Partial hepatectomy-induced upregulation of miR-1907 accelerates liver regeneration by activation autophagy. Biomed. Res. Int. 2018:3817057. doi: 10.1155/2018/3817057
Lu, Y., Liu, P., Fu, P., Chen, Y., Nan, D., and Yang, X. (2017). Comparison of the dwi and gd-eob-dtpa-enhanced mri on assessing the hepatic ischemia and reperfusion injury after partial hepatectomy. Biomed. Pharmacother. 86, 118–126. doi: 10.1016/j.biopha.2016.11.123
Lu, Y., Lohitnavy, M., Reddy, M. B., Lohitnavy, O., Ashley, A., and Yang, R. S. H. (2006). An updated physiologically based pharmacokinetic model for hexachlorobenzene: incorporation of pathophysiological states following partial hepatectomy and hexachlorobenzene treatment. Toxicol. Sci. 91, 29–41. doi: 10.1093/toxsci/kfj133
Ma, R., Hunter, P., Cousins, W., Ho, H., Bartlett, A., and Safaei, S. (2020). Modeling the hepatic arterial flow in living liver donor after left hepatectomy and postoperative boundary condition exploration. Int. J. Numer. Method Biomed. Eng. 36:e3268. doi: 10.1002/cnm.3268
Madrahimov, N., Dirsch, O., Broelsch, C., and Dahmen, U. (2006). Marginal hepatectomy in the rat: from anatomy to surgery. Ann. Surg. 244, 89–98. doi: 10.1097/01.sla.0000218093.12408.0f
Mann, F. C., Fishback, F. C., Gay, J. S., and Green, G. F. (1931). Experimental pathology of the liver. Arch. Pathol. 12, 787–793.
Mardinoglu, A., Agren, R., Kampf, C., Asplund, A., Uhlen, M., and Nielsen, J. (2014). Genome-scale metabolic modelling of hepatocytes reveals serine deficiency in patients with non-alcoholic fatty liver disease. Nat. Commun. 5:3083. doi: 10.1038/ncomms4083
Mardinoglu, A., Wu, H., Bjornson, E., Zhang, C., Hakkarainen, A., Räsänen, S. M., et al. (2018). An integrated understanding of the rapid metabolic benefits of a carbohydrate-restricted diet on hepatic steatosis in humans. Cell. Metab. 27, 559.e5–571.e5. doi: 10.1016/j.cmet.2018.01.005
Martirosian, P., Pohmann, R., Schraml, C., Schwartz, M., Kuestner, T., Schwenzer, N. F., et al. (2019). Spatial-temporal perfusion patterns of the human liver assessed by pseudo-continuous arterial spin labeling mri. Z. Med. Phys. 29, 173–183. doi: 10.1016/j.zemedi.2018.08.004
Means, S. A., and Ho, H. (2019). A spatial-temporal model for zonal hepatotoxicity of acetaminophen. Drug Metab Pharmacokinet. 34, 71–77. doi: 10.1016/j.dmpk.2018.09.266
Meyer, K., Morales-Navarrete, H., Seifert, S., Wilsch-Braeuninger, M., Dahmen, U., Tanaka, E. M., et al. (2020). Bile canaliculi remodeling activates YAP via the actin cytoskeleton during liver regeneration. Mol. Syst. Biol. 16, e8985. doi: 10.15252/msb.20198985
Michalopoulos, G. K. (2017). Hepatostat: liver regeneration and normal liver tissue maintenance. Hepatology 65, 1384–1392. doi: 10.1002/hep.28988
Mihaylova, M. M., and Shaw, R. J. (2011). The ampk signalling pathway coordinates cell growth, autophagy and metabolism. Nat. Cell. Biol. 13, 1016–1023. doi: 10.1038/ncb2329
Monga, S. P. S. (2014). Role and regulation of β-catenin signaling during physiological liver growth. Gene Expr. 16, 51–62. doi: 10.3727/105221614X13919976902138
Moreno-Carranza, B., Goya-Arce, M., Vega, C., Adán, N., Triebel, J., López-Barrera, F., et al. (2013). Prolactin promotes normal liver growth, survival, and regeneration in rodents: effects on hepatic il-6, suppressor of cytokine signaling-3, and angiogenesis. Am. J. Physiol. Regul. Integr. Comp. Physiol. 305, R720–R726. doi: 10.1152/ajpregu.00282.2013
Muhi, A., Ichikawa, T., Motosugi, U., Sano, K., Fatima, Z., Matsuda, M., et al. (2013). Diffusion-weighted imaging of hepatocellular carcinoma for predicting early recurrence and survival after hepatectomy. Hepatol. Int. 7, 662–668. doi: 10.1007/s12072-012-9383-2
Mürtz, P., Sprinkart, A. M., Reick, M., Pieper, C. C., Schievelkamp, A.-H., König, R., et al. (2018). Accurate ivim model-based liver lesion characterisation can be achieved with only three b-value dwi. Eur. Radiol. 28, 4418–4428. doi: 10.1007/s00330-018-5401-7
Müsch, A. (2014). The unique polarity phenotype of hepatocytes. Exp. Cell. Res. 328, 276–283. doi: 10.1016/j.yexcr.2014.06.006
Nagano, Y., Nagahori, K., Yoshiro, F., Hamaguchi, Y., Ishikawa, T., Ichikawa, Y., et al. (2004). Gene expression profile analysis of regenerating liver after portal vein ligation in rats by a cDNA microarray system. Liver Int. 24, 253–258. doi: 10.1111/j.1478-3231.2004.0912.x
Neal, M. L., König, M., Nickerson, D., Mısırlı, G., Kalbasi, R., Dräger, A., et al. (2019). Harmonizing semantic annotations for computational models in biology. Brief. Bioinform. 20, 540–550. doi: 10.1093/bib/bby087
Niiya, T., Murakami, M., Aoki, T., Murai, N., Shimizu, Y., and Kusano, M. (1999). Immediate increase of portal pressure, reflecting sinusoidal shear stress, induced liver regeneration after partial hepatectomy. J. Hepatobiliary Pancreat. Surg. 6, 275–280. doi: 10.1007/s005340050118
Nikmaneshi, M. R., Firoozabadi, B., and Munn, L. L. (2020). A mechanobiological mathematical model of liver metabolism. Biotechnol. Bioeng. 117, 2861–2874. doi: 10.1002/bit.27451
Nishii, K., Brodin, E., Renshaw, T., Weesner, R., Moran, E., Soker, S., et al. (2018). Shear stress upregulates regeneration-related immediate early genes in liver progenitors in 3d ecm-like microenvironments. J. Cell. Physiol. 233, 4272–4281. doi: 10.1002/jcp.26246
Nista, E. C., Fini, L., Armuzzi, A., Candelli, M., Zocco, M. A., Cazzato, I. A., et al. (2004). 13C-breath tests in the study of microsomal liver function. Eur. Rev. Med. Pharmacol. Sci. 8, 33–46. Available online at: http://www.europeanreview.org/wp/wp-content/uploads/104.pdf
Nobuoka, T., Mizuguchi, T., Oshima, H., Shibata, T., Kimura, Y., Mitaka, T., et al. (2006). Portal blood flow regulates volume recovery of the rat liver after partial hepatectomy: molecular evaluation. Eur. Surg. Res. 38, 522–532. doi: 10.1159/000096292
Nonami, T., Nakao, A., Kurokawa, T., Inagaki, H., Matsushita, Y., Sakamoto, J., et al. (1999). Blood loss and icg clearance as best prognostic markers of post-hepatectomy liver failure. Hepatogastroenterology 46, 1669–1672.
Palaniyappan, N., Cox, E., Bradley, C., Scott, R., Austin, A., O'Neill, R., et al. (2016). Non-invasive assessment of portal hypertension using quantitative magnetic resonance imaging. J. Hepatol. 65, 1131–1139. doi: 10.1016/j.jhep.2016.07.021
Pang, K. S., and Rowland, M. (1977). Hepatic clearance of drugs. i. theoretical considerations of a “well-stirred” model and a “parallel tube” model. influence of hepatic blood flow, plasma and blood cell binding, and the hepatocellular enzymatic activity on hepatic drug clearance. J. Pharmacokinet. Biopharm. 5, 625–653. doi: 10.1007/BF01059688
Papatheodorou, I., Moreno, P., Manning, J., Fuentes, A. M.-P., George, N., Fexova, S., et al. (2020). Expression Atlas update: from tissues to single cells. Nucleic Acids Res. 48, D77–D83. doi: 10.1093/nar/gkz947
Parmar, K. M., Larman, H. B., Dai, G., Zhang, Y., Wang, E. T., Moorthy, S. N., et al. (2006). Integration of flow-dependent endothelial phenotypes by kruppel-like factor 2. J. Clin. Invest. 116, 49–58. doi: 10.1172/JCI24787
Peng, T., Liu, L., MacLean, A. L., Wong, C. W., Zhao, W., and Nie, Q. (2017). A mathematical model of mechanotransduction reveals how mechanical memory regulates mesenchymal stem cell fate decisions. BMC Syst. Biol. 11, 55. doi: 10.1186/s12918-017-0429-x
Perel, P., Roberts, I., Sena, E., Wheble, P., Briscoe, C., Sandercock, P., et al. (2007). Comparison of treatment effects between animal experiments and clinical trials: systematic review. BMJ 334, 197. doi: 10.1136/bmj.39048.407928.BE
Peres, L. A. B., Bredt, L. C., and Cipriani, R. F. F. (2016). Acute renal injury after partial hepatectomy. World J. Hepatol. 8, 891–901. doi: 10.4254/wjh.v8.i21.891
Periwal, V., Gaillard, J. R., Needleman, L., and Doria, C. (2014). Mathematical model of liver regeneration in human live donors. J. Cell. Physiol. 229, 599–606. doi: 10.1002/jcp.24482
Pibiri, M. (2018). Liver regeneration in aged mice: new insights. Aging 10, 1801–1824. doi: 10.18632/aging.101524
Pibiri, M., Sulas, P., Leoni, V. P., Perra, A., Kowalik, M. A., Cordella, A., et al. (2015). Global gene expression profile of normal and regenerating liver in young and old mice. Age (Dordr) 37, 9796. doi: 10.1007/s11357-015-9796-7
Picard, C., Starkel, P., Sempoux, C., Saliez, A., Lebrun, V., and Horsmans, Y. (2004). Molecular mechanisms of apoptosis in the liver of rats after portal branch ligation with and without retrorsine. Lab. Invest. 84, 618–628. doi: 10.1038/labinvest.3700085
Pivovarov, D., Willner, K., Steinmann, P., Brumme, S., Müller, M., Srisupattarawanit, T., et al. (2019). Challenges of order reduction techniques for problems involving polymorphic uncertainty. GAMM Mitteilungen 42:e201900011. doi: 10.1002/gamm.201900011
Pocaterra, A., Romani, P., and Dupont, S. (2020). Yap/taz functions and their regulation at a glance. J. Cell. Sci. 133:jcs230425. doi: 10.1242/jcs.230425
Poisson, J., Lemoinne, S., Boulanger, C., Durand, F., Moreau, R., Valla, D., et al. (2017). Liver sinusoidal endothelial cells: Physiology and role in liver diseases. J. Hepatol. 66, 212–227. doi: 10.1016/j.jhep.2016.07.009
Puhl, G., Schaser, K. D., Vollmar, B., Menger, M. D., and Settmacher, U. (2003). Noninvasive in vivo analysis of the human hepatic microcirculation using orthogonal polorization spectral imaging. Transplantation 75, 756–761. doi: 10.1097/01.TP.0000056634.18191.1A
Ramadori, G., and Christ, B. (1999). Cytokines and the hepatic acute-phase response. Semin. Liver Dis. 19, 141–155. doi: 10.1055/s-2007-1007106
Rani, H. P., Sheu, T. W. H., Chang, T. M., and Liang, P. C. (2006). Numerical investigation of non-newtonian microcirculatory blood flow in hepatic lobule. J. Biomech. 39, 551–563. doi: 10.1016/j.jbiomech.2004.11.029
Rassam, F., Olthof, P. B., Richardson, H., van Gulik, T. M., and Bennink, R. J. (2019a). Practical guidelines for the use of technetium-99m mebrofenin hepatobiliary scintigraphy in the quantitative assessment of liver function. Nucl Med. Commun. 40, 297–307. doi: 10.1097/MNM.0000000000000973
Rassam, F., Uz, Z., van Lienden, K. P., Ince, C., Bennink, R. J., and van Gulik, T. M. (2019b). Quantitative assessment of liver function using hepatobiliary scintigraphy: the effect of microcirculatory alterations after portal vein embolization. Nucl. Med. Commun. 40, 720–726. doi: 10.1097/MNM.0000000000001012
Ray, S., Mehta, N. N., Golhar, A., and Nundy, S. (2018). Post hepatectomy liver failure - A comprehensive review of current concepts and controversies. Ann. Med. Surg. (Lond) 34, 4–10. doi: 10.1016/j.amsu.2018.08.012
Ren, P., Kang, Z., Gu, G., Liu, Y., Xu, W., Tao, H., et al. (2008). Hyperbaric oxygen preconditioning promotes angiogenesis in rat liver after partial hepatectomy. Life Sci. 83, 236–241. doi: 10.1016/j.lfs.2008.06.011
Ren, W., Chen, G., Wang, X., Zhang, A., Li, C., Lv, W., et al. (2015). Simultaneous bile duct and portal vein ligation induces faster atrophy/hypertrophy complex than portal vein ligation: role of bile acids. Sci. Rep. 5:8455. doi: 10.1038/srep08455
Rib, L., Villeneuve, D., Minocha, S., Praz, V., Hernandez, N., Guex, N., et al. (2018). Cycles of gene expression and genome response during mammalian tissue regeneration. Epigenetics Chromatin 11, 52. doi: 10.1186/s13072-018-0222-0
Ricken, T., and Bluhm, J. (2010). Remodeling and growth of living tissue: a multiphase theory. Arch. Appl. Mech. 80, 453–465. doi: 10.1007/s00419-009-0383-1
Ricken, T., Dahmen, U., and Dirsch, O. (2010). A biphasic model for sinusoidal liver perfusion remodeling after outflow obstruction. Biomech. Model Mechanobiol. 9, 435–450. doi: 10.1007/s10237-009-0186-x
Ricken, T., and Lambers, L. (2019). On computational approaches of liver lobule function and perfusion simulation. GAMM Mitteilungen 42:e0198060. doi: 10.1002/gamm.201900016
Ricken, T., Schwarz, A., and Bluhm, J. (2007). A triphasic model of transversely isotropic biological tissue with applications to stress and biologically induced growth. Comput. Mater. Sci. 39, 124–136. doi: 10.1016/j.commatsci.2006.03.025
Ricken, T., Werner, D., Holzhütter, H. G., König, M., Dahmen, U., and Dirsch, O. (2015). Modeling function-perfusion behavior in liver lobules including tissue, blood, glucose, lactate and glycogen by use of a coupled two-scale pde-ode approach. Biomech. Model Mechanobiol. 14, 515–536. doi: 10.1007/s10237-014-0619-z
Rinck, P. A., Petersen, S. B., and Lauterbur, P. C. (1984). [NMR imaging of fluorine-containing substances. 19-Fluorine ventilation and perfusion studies]. Rofo 140, 239–243. doi: 10.1055/s-2008-1052964
Roldán-Alzate, A., Frydrychowicz, A., Said, A., Johnson, K. M., Francois, C. J., Wieben, O., et al. (2015). Impaired regulation of portal venous flow in response to a meal challenge as quantified by 4D flow MRI. J. Magn. Reson. Imaging 42, 1009–1017. doi: 10.1002/jmri.24886
Rosen, B. R., Belliveau, J. W., Vevea, J. M., and Brady, T. J. (1990). Perfusion imaging with nmr contrast agents. Magn. Reson. Med. 14, 249–265. doi: 10.1002/mrm.1910140211
Rous, P., and Larimore, L. D. (1920). RELATION OF THE PORTAL BLOOD TO LIVER MAINTENANCE : A DEMONSTRATION OF LIVER ATROPHY CONDITIONAL ON COMPENSATION. J. Exp. Med. 31, 609–632. doi: 10.1084/jem.31.5.609
Rowland, M., Benet, L. Z., and Graham, G. G. (1973). Clearance concepts in pharmacokinetics. J. Pharmacokinet. Biopharm. 1, 123–136. doi: 10.1007/BF01059626
Rozga, J., Jeppsson, B., and Bengmark, S. (1986). Portal branch ligation in the rat. Reevaluation of a model. Am. J. Pathol. 125, 300–308.
Rubin, T. M., Heyne, K., Luchterhand, A., Jan, B.ednarsch, N. W. R., Vondran, F., et al. (2017). Kinetic validation of the LiMAx test during 10 000 intravenous 13C-methacetin breath tests. J. Breath Res. 12, 016005. doi: 10.1088/1752-7163/aa820b
Rui, L. (2014). Energy metabolism in the liver. Compr. Physiol. 4, 177–197. doi: 10.1002/cphy.c130024
Saito, S., Togo, S., Morioka, D., Matsuo, K.-i., Yoshimoto, N., Nagano, Y., et al. (2006). A rat model of a repeat 70% major hepatectomy. J. Surg. Res. 134, 322–326. doi: 10.1016/j.jss.2006.01.008
Saito, Y., Morine, Y., Iwahashi, S., Ikemoto, T., Imura, S., Yamanaka-Okumura, H., et al. (2018). Changes of liver metabolites following hepatectomy with ischemia reperfusion towards liver regeneration. Ann. Gastroenterol. Surg. 2, 204–211. doi: 10.1002/ags3.12058
Saltelli, A., Bammer, G., Bruno, I., Charters, E., Di Fiore, M., Didier, E., et al. (2020). Five ways to ensure that models serve society: a manifesto. Nature 582, 482–484. doi: 10.1038/d41586-020-01812-9
Salvi, A. M., and DeMali, K. A. (2018). Mechanisms linking mechanotransduction and cell metabolism. Curr. Opin. Cell. Biol. 54, 114–120. doi: 10.1016/j.ceb.2018.05.004
Samino, S., Revuelta-Cervantes, J., Vinaixa, M., Rodríguez, M. Á, Valverde, A. M., et al. (2013). A (1)h nmr metabolic profiling to the assessment of protein tyrosine phosphatase 1b role in liver regeneration after partial hepatectomy. Biochimie 95, 808–816. doi: 10.1016/j.biochi.2012.11.015
Sato, N., Kenjo, A., Kimura, T., Okada, R., Ishigame, T., Kofunato, Y., et al. (2018). Prediction of major complications after hepatectomy using liver stiffness values determined by magnetic resonance elastography. Br. J. Surg. 105, 1192–1199. doi: 10.1002/bjs.10831
Sato, Y., Koyama, S., Tsukada, K., and Hatakeyama, K. (1997). Acute portal hypertension reflecting shear stress as a trigger of liver regeneration following partial hepatectomy. Surg. Today 27, 518–526. doi: 10.1007/BF02385805
Sato, Y., Tsukada, K., and Hatakeyama, K. (1999). Role of shear stress and immune responses in liver regeneration after a partial hepatectomy. Surg. Today 29, 1–9. doi: 10.1007/BF02482962
Schnitzbauer, A. A., Lang, S. A., Goessmann, H., Nadalin, S., Baumgart, J., Farkas, S. A., et al. (2012). Right portal vein ligation combined with in situ splitting induces rapid left lateral liver lobe hypertrophy enabling 2-staged extended right hepatic resection in small-for-size settings. Ann. Surg. 255, 405–414. doi: 10.1097/SLA.0b013e31824856f5
Schoen, J. M., Wang, H. H., Minuk, G. Y., and Lautt, W. W. (2001). Shear stress-induced nitric oxide release triggers the liver regeneration cascade. Nitric Oxide 5, 453–464. doi: 10.1006/niox.2001.0373
Schoenheimer, R. (1942). The Dynamic State of Body Constituents. Cambridge, MA: Harvard University Press.
Scott, K. E., Fraley, S. I., and Rangamani, P. (2020). Transfer function for YAP/TAZ nuclear translocation revealed through spatial systems modeling. bioRxiv. 18. doi: 10.1101/2020.10.14.340349
Sebbagh, M., Santoni, M.-J., Hall, B., Borg, J.-P., and Schwartz, M. A. (2009). Regulation of lkb1/strad localization and function by e-cadherin. Curr. Biol. 19, 37–42. doi: 10.1016/j.cub.2008.11.033
Sekine, S., Lan, B. Y.-A., Bedolli, M., Feng, S., and Hebrok, M. (2006). Liver-specific loss of beta-catenin blocks glutamine synthesis pathway activity and cytochrome p450 expression in mice. Hepatology 43, 817–825. doi: 10.1002/hep.21131
Seyedpour, S. M., Nabati, M., Lambers, L., Nafisi, S., Tautenhahn, H.-M., Sack, I., et al. (2021). Application of magnetic resonance imaging in liver biomechanics: a systematic review. Front. Physiol. 12:733393. doi: 10.3389/fphys.2021.733393
Sheng, R.-F., Yang, L., Jin, K.-P., Wang, H.-Q., Liu, H., Ji, Y., et al. (2018). Assessment of liver regeneration after associating liver partition and portal vein ligation for staged hepatectomy: a comparative study with portal vein ligation. HPB 20, 305–312. doi: 10.1016/j.hpb.2017.09.004
Shi, Y., Lawford, P., and Hose, R. (2011). Review of zero-d and 1-d models of blood flow in the cardiovascular system. Biomed. Eng. Online 10:33. doi: 10.1186/1475-925X-10-33
Shin, S.-Y., and Nguyen, L. K. (2016). Unveiling hidden dynamics of hippo signalling: a systems analysis. Genes (Basel) 7:44. doi: 10.3390/genes7080044
Shu, X., Li, N., Wu, Y., Li, W., Zhang, X., Li, P., et al. (2021). Mechanotransduction of liver sinusoidal endothelial cells under varied mechanical stimuli. Acta Mech. Sin. 37, 201–217. doi: 10.1007/s10409-021-01057-3
Simeth, J., Johansson, A., Owen, D., Cuneo, K., Mierzwa, M., Feng, M., et al. (2018). Quantification of liver function by linearization of a two-compartment model of gadoxetic acid uptake using dynamic contrast-enhanced magnetic resonance imaging. NMR Biomed. 31, e3913. doi: 10.1002/nbm.3913
Sluka, J. P., Fu, X., Swat, M., Belmonte, J. M., Cosmanescu, A., Clendenon, S. G., et al. (2016). A liver-centric multiscale modeling framework for xenobiotics. PLoS ONE 11:e0162428. doi: 10.1371/journal.pone.0162428
Smith, L. P., Hucka, M., Hoops, S., Finney, A., Ginkel, M., Myers, C. J., et al. (2015). Sbml level 3 package: hierarchical model composition, version 1 release 3. J. Integr. Bioinform. 12:268. doi: 10.1515/jib-2015-268
Smith, L. P., Moodie, S. L., Bergmann, F. T., Gillespie, C., Keating, S. M., König, M., et al. (2020). Systems biology markup language (sbml) level 3 package: distributions, version 1, release 1. J. Integr. Bioinform. 17:20200018. doi: 10.1515/jib-2020-0018
Song, Z., Gupta, K., Ng, I. C., Xing, J., Yang, Y. A., and Yu, H. (2017). Mechanosensing in liver regeneration. Semin. Cell. Dev. Biol. 71, 153–167. doi: 10.1016/j.semcdb.2017.07.041
Søreide, J. A., and Deshpande, R. (2021). Post hepatectomy liver failure (phlf)-recent advances in prevention and clinical management. Eur. J. Surg. Oncol. 47, 216–224. doi: 10.1016/j.ejso.2020.09.001
Steinman, J. B., Salomao, M. A., and Pajvani, U. B. (2021). Zonation in nash - a key paradigm for understanding pathophysiology and clinical outcomes. Liver Int. 41, 2534–2546. doi: 10.1111/liv.15025
Stockmann, M., Lock, J. F., Riecke, B., Heyne, K., Martus, P., Fricke, M., et al. (2009). Prediction of postoperative outcome after hepatectomy with a new bedside test for maximal liver function capacity. Ann. Surg. 250, 119–125. doi: 10.1097/SLA.0b013e3181ad85b5
Strey, C. W., Winters, M. S., Markiewski, M. M., and Lambris, J. D. (2005). Partial hepatectomy induced liver proteome changes in mice. Proteomics 5, 318–325. doi: 10.1002/pmic.200400913
Sugimoto, T., Yamada, T., Iwata, H., Sekino, T., Matsumoto, S., Ishida, N., et al. (2009). Two-stage portal vein ligation facilitates liver regeneration in rats. Eur. Surg. Res. 42, 181–188. doi: 10.1159/000203406
Sugiura, R., Kuwatani, M., Nishida, M., Hirata, K., Sano, I., Kato, S., et al. (2019). Correlation between liver elasticity by ultrasound elastography and liver functional reserve. Ultrasound Med. Biol. 45, 2704–2712. doi: 10.1016/j.ultrasmedbio.2019.06.407
Sun, M., Spill, F., and Zaman, M. H. (2016). A computational model of YAP/TAZ mechanosensing. Biophys. J. 110, 2540–2550. doi: 10.1016/j.bpj.2016.04.040
Sun, Y., Deng, X., Li, W., Yan, Y., Wei, H., Jiang, Y., et al. (2007). Liver proteome analysis of adaptive response in rat immediately after partial hepatectomy. Proteomics 7, 4398–4407. doi: 10.1002/pmic.200600913
Swiderska-Syn, M., Xie, G., Michelotti, G. A., Jewell, M. L., Premont, R. T., Syn, W.-K., et al. (2016). Hedgehog regulates yes-associated protein 1 in regenerating mouse liver. Hepatology 64, 232–244. doi: 10.1002/hep.28542
Takahashi, H., Shigefuku, R., Yoshida, Y., Ikeda, H., Matsunaga, K., Matsumoto, N., et al. (2014). Correlation between hepatic blood flow and liver function in alcoholic liver cirrhosis. World J. Gastroenterol. 20, 17065–17074. doi: 10.3748/wjg.v20.i45.17065
Tautenhahn, H.-M., Brückner, S., Baumann, S., Winkler, S., Otto, W., von Bergen, M., et al. (2016). Attenuation of postoperative acute liver failure by mesenchymal stem cell treatment due to metabolic implications. Ann. Surg. 263, 546–556. doi: 10.1097/SLA.0000000000001155
Tautenhahn, H.-M., Brückner, S., Uder, C., Erler, S., Hempel, M., von Bergen, M., et al. (2017). Mesenchymal stem cells correct haemodynamic dysfunction associated with liver injury after extended resection in a pig model. Sci. Rep. 7, 2617. doi: 10.1038/s41598-017-02670-8
Tchirikov, M., Kertschanska, S., Stürenberg, H. J., and Schröder, H. J. (2002). Liver blood perfusion as a possible instrument for fetal growth regulation. Placenta 23(Suppl. A):S153–S158. doi: 10.1053/plac.2002.0810
Thng, C. H., Koh, T. S., Collins, D. J., and Koh, D. M. (2010). Perfusion magnetic resonance imaging of the liver. World J. Gastroenterol. 16, 1598–1609. doi: 10.3748/wjg.v16.i13.1598
Thomas, M. N., Weninger, E., Angele, M., Bösch, F., Pratschke, S., Andrassy, J., et al. (2015). Intraoperative simulation of remnant liver function during anatomic liver resection with indocyanine green clearance (LiMON) measurements. HPB 17, 471–476. doi: 10.1111/hpb.12380
Thomaseth, C., and Radde, N. (2016). Normalization of western blot data affects the statistics of estimators**this work was supported by the german federal ministry of education and research (bmbf) within the e:bio-innovationswettbewerb systembiologie project predict (grant number fkz0316186a) and the german research foundation (dfg) within the cluster of excellence in simulation technology (exc 310/2) at the university of stuttgart. IFAC Papers OnLine 49, 56–62. doi: 10.1016/j.ifacol.2016.12.103
Togo, S., Makino, H., Kobayashi, T., Morita, T., Shimizu, T., Kubota, T., et al. (2004). Mechanism of liver regeneration after partial hepatectomy using mouse cDNA microarray. J. Hepatol. 40, 464–471. doi: 10.1016/j.jhep.2003.11.005
Torre, C., Perret, C., and Colnot, S. (2011). Transcription dynamics in a physiological process: β-catenin signaling directs liver metabolic zonation. Int. J. Biochem. Cell Biol. 43, 271–278. doi: 10.1016/j.biocel.2009.11.004
Troelstra, M. A., Witjes, J. J., van Dijk, A.-M., Mak, A. L., Gurney-Champion, O., Runge, J. H., et al. (2021). Assessment of imaging modalities against liver biopsy in nonalcoholic fatty liver disease: the amsterdam nafld-nash cohort. J. Magn. Reson. Imaging doi: 10.1002/jmri.27703. [Epub ahead of print].
Uz, Z., Ince, C., Rassam, F., Ergin, B., van Lienden, K. P., and van Gulik, T. M. (2019). Assessment of hepatic microvascular flow and density in patients undergoing preoperative portal vein embolization. HPB 21, 187–194. doi: 10.1016/j.hpb.2018.07.002
Vollmar, B., and Menger, M. D. (2009). The hepatic microcirculation: mechanistic contributions and therapeutic targets in liver injury and repair. Physiol. Rev. 89, 1269–1339. doi: 10.1152/physrev.00027.2008
von Heesen, M., Dold, S., Müller, S., Scheuer, C., Kollmar, O., Schilling, M. K., et al. (2015). Cilostazol improves hepatic blood perfusion, microcirculation, and liver regeneration after major hepatectomy in rats. Liver Transpl. 21, 792–800. doi: 10.1002/lt.24114
Walldorf, J., Hillebrand, C., Aurich, H., Stock, P., Hempel, M., Ebensing, S., et al. (2010). Propranolol impairs liver regeneration after partial hepatectomy in c57bl/6-mice by transient attenuation of hepatic lipid accumulation and increased apoptosis. Scand. J. Gastroenterol. 45, 468–476. doi: 10.3109/00365520903583848
Wan, S., Liu, X., Jiang, H., Teng, Z., and Song, B. (2021). Noninvasive imaging assessment of portal hypertension: where are we now and where does the future lie? Expert. Rev. Mol. Diagn. 21, 343–345. doi: 10.1080/14737159.2021.1904897
Wang, H., Feng, M., Jackson, A., ten Haken, R. K., Lawrence, T. S., and Cao, Y. (2016). Local and global function model of the liver. Int. J. Radiat. Oncol. Biol. Phys. 94, 181–188. doi: 10.1016/j.ijrobp.2015.09.044
Wang, S., Li, B., Li, P., Xie, R., Wang, Q., Shi, H., et al. (2021a). Feasibility of perfusion and early-uptake 18f-fdg pet/ct in primary hepatocellular carcinoma: a dual-input dual-compartment uptake model. Jpn J. Radiol. doi: 10.1007/s11604-021-01140-6. [Epub ahead of print].
Wang, Y., Zhang, L., Ning, J., Zhang, X., Li, X., Zhang, L., et al. (2021b). Preoperative remnant liver function evaluation using a routine clinical dynamic Gd-EOB-DTPA-enhanced MRI protocol in patients with hepatocellular carcinoma. Ann Surg Oncol, 28, 3672–3682. doi: 10.1245/s10434-020-09361-1
Wehling, L., Keegan, L., Schmitt, J., Sahle, S., Schirmacher, P., Kummer, U., et al. (2021). Mathematical modeling of yap and taz nuclear/cytoplasmic shuttling in liver cancer cells. Z. Gastroenterol. 59, 4–15. doi: 10.1055/s-0040-1722055
Wei, W., Hua, C., Zhang, T., Dirsch, O., Gremse, F., Homeyer, A., et al. (2020). Size of portally deprived liver lobe after portal vein ligation and additional partial hepatectomy: Result of balancing proliferation and apoptosis. Sci. Rep. 10, 4893. doi: 10.1038/s41598-020-60310-0
Wei, W., Zhang, T., Zafarnia, S., Schenk, A., Xie, C., Kan, C., et al. (2016). Establishment of a rat model: associating liver partition with portal vein ligation for staged hepatectomy. Surgery 159, 1299–1307. doi: 10.1016/j.surg.2015.12.005
Weinbren, K. (1955). The portal blood supply and regeneration of the rat liver. Br. J. Exp. Pathol. 36, 583–591.
Weinbren, K., and Woodward, E. (1964). DELAYED INCORPORATION OF 32P FROM ORTHOPHOSPHATE INTO DEOXYRIBONUCLEIC ACID OF RAT LIVER AFTER SUBTOTAL HEPATECTOMY. Br. J. Exp. Pathol. 45, 442–449.
Weiss, J., Ruff, C., Grosse, U., Grözinger, G., Horger, M., Nikolaou, K., et al. (2019). Assessment of hepatic perfusion using grasp mri: bringing liver mri on a new level. Invest. Radiol. 54, 737–743. doi: 10.1097/RLI.0000000000000586
Wells, R. G. (2005). The role of matrix stiffness in hepatic stellate cell activation and liver fibrosis. J. Clin. Gastroenterol. 39(4 Suppl. 2):S158–S161. doi: 10.1097/01.mcg.0000155516.02468.0f
Wild, S. L., Elghajiji, A., Grimaldos Rodriguez, C., Weston, S. D., Burke, Z. D., and Tosh, D. (2020). The canonical wnt pathway as a key regulator in liver development, differentiation and homeostatic renewal. Genes 11:1163. doi: 10.3390/genes11101163
Williams, D. S., Detre, J. A., Leigh, J. S., and Koretsky, A. P. (1992). Magnetic resonance imaging of perfusion using spin inversion of arterial water. Proc. Natl. Acad. Sci. U.S.A. 89, 212–216. doi: 10.1073/pnas.89.1.212
Willmann, S., Schmitt, W., Keldenich, J., and Dressman, J. B. (2003). A physiologic model for simulating gastrointestinal flow and drug absorption in rats. Pharm. Res. 20, 1766–1771. doi: 10.1023/B:PHAM.0000003373.72652.c0
Winkler, K., Keiding, S., and Tygstrup, N. (1973). “Clearance as a quantitative measure of liver function”, in The Liver. Quantitative Aspects of Structure and Function. Proceedings. Karger, Basel, eds Paumgartner, G., Preisig, R. 144–155. doi: 10.1159/000394804
Xie, C., Wei, W., Schenk, A., Schwen, L. O., Zafarnia, S., Schwier, M., et al. (2016). Visualization of vascular and parenchymal regeneration after 70% partial hepatectomy in normal mice. J. Vis. Exp. 115:53935. doi: 10.3791/53935
Yagi, S., Hirata, M., Miyachi, Y., and Uemoto, S. (2020). Liver regeneration after hepatectomy and partial liver transplantation. Int. J. Mol. Sci. 21, 8414. doi: 10.3390/ijms21218414
Yang, J., Meng, M., Pan, C., Qian, L., Sun, Y., Shi, H., et al. (2021). Intravoxel incoherent motion and dynamic contrast-enhanced magnetic resonance imaging to early detect tissue injury and microcirculation alteration in hepatic injury induced by intestinal ischemia-reperfusion in a rat model. J. Magn. Reson. Imaging 54, 751–760. doi: 10.1002/jmri.27604
Yin, M., Glaser, K. J., Manduca, A., Mounajjed, T., Malhi, H., Simonetto, D. A., et al. (2017). Distinguishing between hepatic inflammation and fibrosis with MR elastography. Radiology 284, 694–705. doi: 10.1148/radiol.2017160622
Yin, M., and Venkatesh, S. K. (2018). Ultrasound or MR elastography of liver: which one shall I use? Abdom Radiol. (NY) 43, 1546–1551. doi: 10.1007/s00261-017-1340-z
Yoon, J. H., Choi, J.-I., Jeong, Y. Y., Schenk, A., Chen, L., Laue, H., et al. (2016). Pre-treatment estimation of future remnant liver function using gadoxetic acid mri in patients with hcc. J. Hepatol. 65, 1155–1162. doi: 10.1016/j.jhep.2016.07.024
Yoshida, D., Akahoshi, T., Kawanaka, H., Yamaguchi, S., Kinjo, N., Taketomi, A., et al. (2011). Roles of vascular endothelial growth factor and endothelial nitric oxide synthase during revascularization and regeneration after partial hepatectomy in a rat model. Surg. Today 41, 1622–1629. doi: 10.1007/s00595-010-4484-9
Yzet, T., Bouzerar, R., Baledent, O., Renard, C., Lumbala, D. M., Nguyen-Khac, E., et al. (2010). Dynamic measurements of total hepatic blood flow with Phase Contrast MRI. Eur. J. Radiol. 73, 119–124. doi: 10.1016/j.ejrad.2008.09.032
Zhang, Y. N., Fowler, K. J., Hamilton, G., Cui, J. Y., Sy, E. Z., Balanay, M., et al. (2018). Liver fat imaging-a clinical overview of ultrasound, CT, and MR imaging. Br. J. Radiol. 91, 20170959. doi: 10.1259/bjr.20170959
Zhao, Y., Chen, E., Huang, K., Xie, Z., Zhang, S., Wu, D., et al. (2020). Dynamic alterations of plasma metabolites in the progression of liver regeneration after partial hepatectomy. J. Proteome Res. 19, 174–185. doi: 10.1021/acs.jproteome.9b00493
Keywords: multi-scale modeling, liver regeneration, liver surgery, liver perfusion, perfusion/function relationship, mechanoperception, rodent models of liver surgery
Citation: Christ B, Collatz M, Dahmen U, Herrmann K-H, Höpfl S, König M, Lambers L, Marz M, Meyer D, Radde N, Reichenbach JR, Ricken T and Tautenhahn H-M (2021) Hepatectomy-Induced Alterations in Hepatic Perfusion and Function - Toward Multi-Scale Computational Modeling for a Better Prediction of Post-hepatectomy Liver Function. Front. Physiol. 12:733868. doi: 10.3389/fphys.2021.733868
Received: 30 June 2021; Accepted: 26 October 2021;
Published: 18 November 2021.
Edited by:
Matteo Barberis, University of Surrey, United KingdomReviewed by:
Aravind Rammohan, Corning Inc., United StatesCopyright © 2021 Christ, Collatz, Dahmen, Herrmann, Höpfl, König, Lambers, Marz, Meyer, Radde, Reichenbach, Ricken and Tautenhahn. This is an open-access article distributed under the terms of the Creative Commons Attribution License (CC BY). The use, distribution or reproduction in other forums is permitted, provided the original author(s) and the copyright owner(s) are credited and that the original publication in this journal is cited, in accordance with accepted academic practice. No use, distribution or reproduction is permitted which does not comply with these terms.
*Correspondence: Bruno Christ, QnJ1bm8uQ2hyaXN0QG1lZGl6aW4udW5pLWxlaXB6aWcuZGU=
†These authors have contributed equally to this work and share first authorship
Disclaimer: All claims expressed in this article are solely those of the authors and do not necessarily represent those of their affiliated organizations, or those of the publisher, the editors and the reviewers. Any product that may be evaluated in this article or claim that may be made by its manufacturer is not guaranteed or endorsed by the publisher.
Research integrity at Frontiers
Learn more about the work of our research integrity team to safeguard the quality of each article we publish.