- 1Department of Risk Assessment for Products in Development, The Netherlands Organization for Applied Scientific Research (TNO), Utrecht, Netherlands
- 2Department of Microbiology and Systems Biology, The Netherlands Organization for Applied Scientific Research (TNO), Zeist, Netherlands
- 3Department of Metabolic Health Research, The Netherlands Organization for Applied Scientific Research (TNO), Leiden, Netherlands
Metabolic disorders, such as obesity and type 2 diabetes have a large impact on global health, especially in industrialized countries. Tissue-specific chronic low-grade inflammation is a key contributor to complications in metabolic disorders. To support therapeutic approaches to these complications, it is crucial to gain a deeper understanding of the inflammatory dynamics and to monitor them on the individual level. To this end, blood-based biomarkers reflecting the tissue-specific inflammatory dynamics would be of great value. Here, we describe an in silico approach to select candidate biomarkers for tissue-specific inflammation by using a priori mechanistic knowledge from pathways and tissue-derived molecules. The workflow resulted in a list of candidate markers, in part consisting of literature confirmed biomarkers as well as a set of novel, more innovative biomarkers that reflect inflammation in the liver and adipose tissue. The first step of biomarker verification was on murine tissue gene-level by inducing hepatic inflammation and adipose tissue inflammation through a high-fat diet. Our data showed that in silico predicted hepatic markers had a strong correlation to hepatic inflammation in the absence of a relation to adipose tissue inflammation, while others had a strong correlation to adipose tissue inflammation in the absence of a relation to liver inflammation. Secondly, we evaluated the human translational value by performing a curation step in the literature using studies that describe the regulation of the markers in human, which identified 9 hepatic (such as Serum Amyloid A, Haptoglobin, and Interleukin 18 Binding Protein) and 2 adipose (Resistin and MMP-9) inflammatory biomarkers at the highest level of confirmation. Here, we identified and pre-clinically verified a set of in silico predicted biomarkers for liver and adipose tissue inflammation which can be of great value to study future development of therapeutic/lifestyle interventions to combat metabolic inflammatory complications.
Introduction
Inflammation is an important component of normal responses to infection and injury, whether locally confined or systemic. An healthy immune response follows a characteristic pathway, where the first response is strong but short, resulting in the exclusion of the pathogen/damage followed by a recovery to homeostasis. This characteristic pathway of inflammation is essential for recovery and remodeling of tissues and helps to regain a healthy homeostasis including its critical function (Hotamisligil and Erbay, 2008). The evolutionary benefits of an optimal effective immune system are evident in protecting against pathogenic intruders. Since immune responses are also linked to energy metabolism, it can therefore be argued that the integration of these systems and their cooperation in responding to fluctuations in the energy and nutritional environment would be beneficial. These responses, however, need to be temporally and locally regulated to maintain an healthy homeostasis.
Chronic disruption of metabolic homeostasis that occurs in, for example, overnutrition, could lead to disturbed immune responses. Especially when the chronic inflammatory activation occurs in metabolically important organs such as liver and adipose tissue, these tissues are stimulated to produce pro-inflammatory cytokines, acute phase proteins, pro-inflammatory lipids, and other biological inflammatory mediators into the circulation, leading to a systemic inflammatory condition (Gregor and Hotamisligil, 2011; Minihane et al., 2015; Hotamisligil, 2017). These processes have a crucial role in the chronic metabolic disease development, such as obesity, type 2 diabetes, fatty liver disease and cardiovascular disease (Hotamisligil, 2006), and forms the mechanistic basis for risk factors of viral infections, such as coronavirus disease 2019 (COVID-19) (Stefan et al., 2021). In order to support therapeutic approaches of metabolic diseases, it is crucial to gain a deeper understanding regarding the inflammatory dynamics in time to monitor or even predict the homeostatic inflammatory status of the individual. To this end, tissue-derived plasma biomarkers reflecting the tissue-specific and systemic inflammatory dynamics in time would be of great help.
Several systemic pro-inflammatory markers such as C-reactive protein (CRP), interleukin (IL) 6, IL 18, fibrinogen, and adhesion molecules [e.g., E-selectin, intercellular adhesion molecule 1 (ICAM-1), and vascular cell adhesion protein 1 (VCAM-1)] (Pradhan, 2001; Thorand et al., 2005; Kelesidis et al., 2006; Li et al., 2009; Shibata et al., 2009) have been identified to monitor “end-stage” of chronic-low-grade inflammatory diseases such as type 2 diabetes, cardiovascular disease (CVD), and cancer. In contrast, plasma levels of the anti-inflammatory adiponectin were inversely associated with CVD (Shibata et al., 2009), type 2 diabetes (Li et al., 2009), and obesity-related cancer (Kelesidis et al., 2006). To know whether these and other biomarkers represent early stages of disease progression at the tissue level, and not at the systemic level, careful assessment of inflammatory biomarkers is required at the pathway level to select those markers that enable assessment of tissue-derived sub-clinical low-grade inflammation.
Recent advances in high-throughput technologies have made it possible to generate, analyse and integrate large multi-omics datasets at molecular and cellular levels (genes, proteins, metabolites, cells) to identify molecular markers of disease processes. The increasing use of in silico approaches and bioinformatics has encouraged researchers to use these multi-level datasets together with existing knowledge and databases in order to generate a systems-level overview of disease development (Mcdermott et al., 2013; Vafaee et al., 2018). GeneSet Enrichment Analysis (GSEA) has become the golden standard in the analysis of omics data, thereby reducing the complexity of the analyses and providing a systems view of the biological processes involved in disease development (Khatri et al., 2012). A large number of methods have been proposed in the literature for this task. The majority of these methods use expression levels as input together with their associated biological pathways (Khatri et al., 2012; García-Campos et al., 2015). The approach described here inverts this strategy, taking known pathways and tissue-derived biomolecules a priori, thereby creating immediately interpretable candidate biomarkers that may help the monitoring of disease at an early phase and/or support treatment strategies. Focusing on the key events leading to chronic low-grade inflammation, enabled us to focus on generic features of a broad range of metabolic diseases. We developed an in silico-based approach which uses prior knowledge on dysregulated pathways in chronic low-grade inflammation to predict blood-based candidate biomarkers. We verified this approach in biomarker databases, experimental data, and scientific literature to identify blood-based biomarkers reflecting the dynamic inflammatory status during the sub-clinical process of chronic low-grade inflammation in liver and adipose tissue.
Materials and Methods
Selection of Genes From Gene Ontology and Human Protein Atlas
The workflow of the biomarker selection approach used in this study is visualized in Figure 1. Briefly, an initial selection of gene ontology (GO) terms was listed using the search terms ‘‘adipose’’ or ‘‘adipocyte’’ to identify adipose tissue related GO terms, and ‘‘liver,’’ ‘‘hepatic,’’ or ‘‘hepatocyte’’ to identify liver tissue related GO terms in the Gene Ontology knowledgebase (1 accessed September 2020). The list of GO terms was manually curated and only those that were associated with human, non-embryonic, endogenous and tissue-specific processes were selected, together with their “child” terms (i.e., a more specialized term than their broader “paren” term). Subsequently, all immune related genes from the GO knowledgebase were selected from the GO terms “immune system process,” “inflammatory response,” “cytokine production,” and their child terms. In order to select those tissue-specific pathways potentially associated with inflammation, only those adipose and liver GO terms were taken that contained genes also present in immune-related GO terms (Meijerink et al., 2019). The genes from these adipose and liver GO terms were then extracted from the GO knowledgebase under the conditions of species “homo sapiens” and type “protein.”
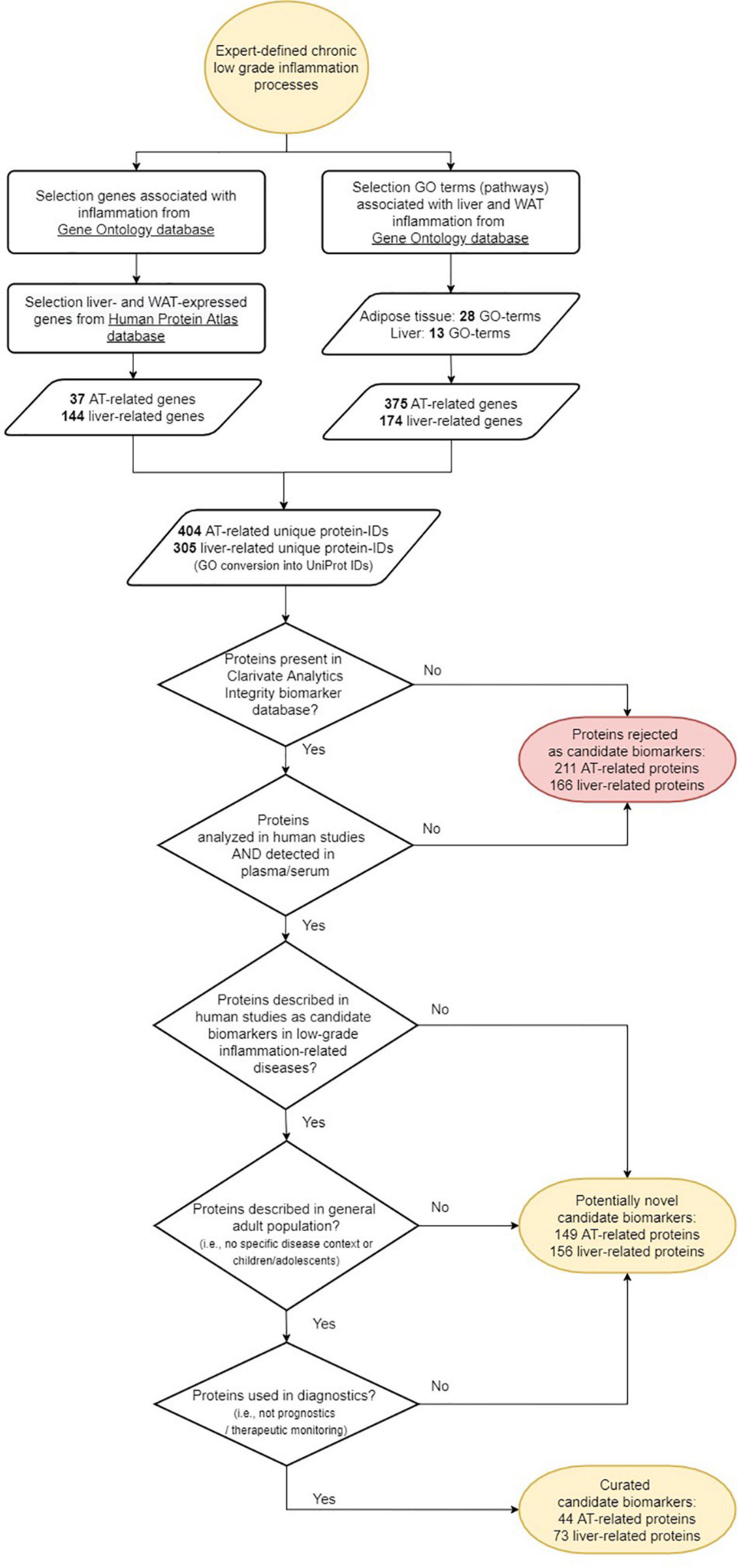
Figure 1. Workflow of the selection of candidate protein biomarkers for tissue inflammation of liver and/or adipose tissue.
Parallel to the extraction of genes from the GO knowledgebase, tissue-specific genes for adipose tissue and liver were selected from the Human Protein Atlas (HPA) (2 accessed September 2020). Details on the definition of tissue specificity can be found in the HPA documentation. In short, it is defined as at least four-fold higher mRNA level in a particular tissue or a group of 2–5 tissues compared to any other tissue or the average level in all other tissues. Only immune related genes as defined from the GO knowledgebase described above and those genes that were assigned a UniProt ID or a gene name were selected.
Selection and Curation of Candidate Protein Biomarkers
All gene names and the associated UniProt ID’s from the selected genes were compared to biomarkers from the Clarivate Analytics Integrity biomarker database3 labeled with type “proteome,” substrate “plasma” or “serum,” and evidence “early human studies,” “late human studies,” or “recommended/approved” to select only those candidate markers that are being identified as human protein biomarker in blood.
Thereafter, the candidate biomarker set was assessed for its novelty using 3 criteria: (i) candidate biomarker is described in one of the following low-grade inflammation related diseases or conditions (Clarivate Analytics Integrity term: “condition”): metabolic syndrome, type 2 diabetes, hyperglycemia, prediabetes, insulin resistance, glucose intolerance, dyslipidemia, hyperlipidemia, hypertriglyceridemia, or atherosclerosis; (ii) candidate biomarker is commonly analyzed in the human adult population; (iii) candidate biomarker is used as diagnostic biomarker in the diseases or conditions under the first criterium. If the answer was no to one of these 3 criteria, the candidate biomarker was assigned as potentially “novel” candidate biomarker. If the answer was yes to these 3 criteria, the candidate biomarker was assigned “curated” candidate biomarker.
Verification of Candidate Biomarkers
Obese Ldlr−/−.Leiden Mice
The in silico predicted candidate biomarkers were verified for their suitability as markers for liver- and/or adipose tissue inflammation in male 14–16 week-old obese and inflammation-prone Ldlr−/−.Leiden mice (van den Hoek et al., 2020). Animal care and use were performed in accordance with the general principles governing the use of animals in experiments of the European Communities (Directive 86/609/EEC) and Dutch Legislation (The Experiments on Animals Act, 1997). This included approval of the study by the ethical review committee (approval reference number TNO-312). The control and reference group of a larger study evaluating the organ-specific effects of lifestyle interventions (van den Hoek et al., 2021) were used for biomarker verification. Briefly, two experimental groups of Ldlr−/−.Leiden mice were included in the study: one group of mice (n = 10; healthy reference group) were fed a low-fat grain-based chow diet (R/M-H, Ssniff Spezialdieten GmbH, Soest, Germany) and the second group of mice (n = 17) were fed a high fat diet (HFD) containing 45 kcal% fat from lard, 35 kcal% from carbohydrates and 20 kcal% casein (D12451, Research Diets, new Brunswick, NJ, United States) for 50 weeks to induce an obese phenotype. Mice were group housed in a temperature-controlled room on a 12 h light-dark cycle and had free access to food and heat sterilized water. Body weight and food intake were determined regularly during the study. At t = 50 weeks mice were sacrificed unfasted using gradual-fill CO2 asphyxiation. Liver tissue and perigonadal white adipose tissue (WAT) were collected, weighed and fixed in formalin and paraffin-embedded for histological analysis or fresh-frozen in N2 and subsequently stored at -80°C for gene expression analysis. Hepatic inflammation was scored using 3 μm sections which were stained with hematoxylin and eosin (H&E) and by counting the number of aggregates of inflammatory cells per field using a 100x magnification (view size of 4.2 mm2). The averages of five random non-overlapping fields were taken and values were expressed per mm2. Perigonadal WAT inflammation was scored using 5 μm sections which were stained with hematoxylin-phloxine-saffron (HPS) using 3 randomly selected fields (1.56 mm2 for perigonadal WAT). Adipose tissue inflammation was measured by counting crown-like structures (CLS) per field using a 100× magnification and values were expressed as number of CLS per 1000 adipocytes. Plasma levels of Serum Amyloid A (SAA) were measured by ELISA specific for SAA (Invitrogen, # KMA0021).
Transcriptome Analysis of Liver and Adipose Tissue
To perform gene expression analysis RNA isolation was performed as described previously in detail (Verschuren et al., 2014). Total RNA was extracted from fresh frozen liver and perigonadal WAT samples using glass beads and RNA-Bee (Campro Scientific, Veenendaal, Netherlands). Briefly, mRNA was extracted from total RNA using oligo-dT magnetic beads. After fragmentation of the mRNA, cDNA was synthesized followed by ligation with the sequencing adapters and amplified by PCR. Quality and yield of the amplicon was determined (Fragment Analyzer, Agilent Technologies, Amstelveen, Netherlands) and fulfilled QC-criteria (broad peak between 300 and 500 bp). In total, a concentration of 1.1 nM of amplicon-library DNA was used for sequencing. Clustering and DNA sequencing, using the Illumina NovaSeq6000, was performed according to manufacturer’s protocols by service provider GenomeScan B.V (Leiden, Netherlands), yielding 15–40 million sequencing clusters per sample and 2 × 150 bp Paired-End reads (PE) per cluster. These counts serve as input for the statistical analysis using DEseq2 package (Love et al., 2014). Selected differentially expressed genes (DEGs), corrected for multiple testing (available in DEseq2 package), were used for expression analyses.
Verification in Human Studies
The candidate biomarkers for low grade inflammation in liver and/or WAT that were verified in the obese Ldlr−/−.Leiden mice, were further evaluated for their confirmed use as hepatic or adipose inflammation markers in human in the MEDLINE® database. The database was searched during February 2021 and March 2021 using the biomarker names and the tissue names. Publications were manually screened and evaluated to assess the use of the candidate biomarkers in a human inflamed setting. The level of confirmation was determined based on (i) a mechanistic rationale present for the marker to be related to liver or adipose tissue; (ii) the biomarker has been described to be associated with tissue specific diseases (e.g., steatosis for liver or obesity for adipose tissue); (iii) the biomarker has been described to be associated with one or more metabolic disorders (i.e., metabolic syndrome, type 2 diabetes, hyperglycemia, prediabetes, insulin resistance, glucose intolerance, dyslipidemia, hyperlipidemia, hypertriglyceridemia, or atherosclerosis).
Three levels of biomarker confirmation were determined: The biomarker was regarded confirmed (level 3) as tissue inflammation derived biomarker if all three conditions were met; putative (level 2) if two out of three conditions were met; possible (level 1) if only one condition was met, being either condition i or ii; not confirmed (level 0) if none of the conditions or only condition 3 was met.
Statistics
All values shown in graphs represent means ± Standard Error of the Mean (SEM). Statistical differences between groups were determined by using non-parametric Kruskal-Wallis followed by Mann-Whitney U test for independent samples using SPSS software. Two-tailed p-values were used and P-values < 0.05 was considered statistically significant. In the case of transcriptome analysis, differentially expressed genes were selected using p-values adjusted for multiple testing (available in DEseq2 package; False Discovery Rate, FDR) < 0.01. Candidate biomarkers were selected based the following criteria: (a) biomarker is detected on gene level in tissue from the mouse study; (b) biomarker is significantly different in liver or adipose tissue; (c) expression (count) level in target tissue is higher than expression in non-target tissue. Spearman’s Rank correlation analysis (MS office package, MS-Excel) was performed to calculate the relation between the absolute gene expression in the tissue and the quantified inflammatory aggregates per animal. A correlation cut-off value of ±0.6 was considered relevant for further evaluation.
Results
Selection of Genes From Gene Ontology and Human Protein Atlas
Table 1 presents an overview of human gene ontologies and genes that were selected as input for the biomarker workflow (Figure 1). For adipose tissue, the initial database search retrieved 47 gene ontologies of which 9 parent ontologies were manually selected (Supplementary Table 1). After extension with their child terms, 41 ontologies were selected for adipose tissue. After mapping with immune-related genes, 7 parent adipose tissue ontologies with 28 child ontologies were selected for further processing (Table 1). These ontologies contained 43% (20%–100%) genes that were also present in immune related ontologies. The search for liver ontologies resulted in 115 hits of which 15 ontologies were selected (Supplementary Table 2). With their child ontologies, a total of 37 terms were selected for liver. A number of 8 parent liver ontologies with their 13 child ontologies were selected for further processing based on their overlap with immune ontologies (Table 1). In these ontologies, 45% (25%–100%) of the genes were also related to immune GO terms. Overall, 375 adipose tissue related genes and 174 liver associated genes were selected from the GO knowledgebase.
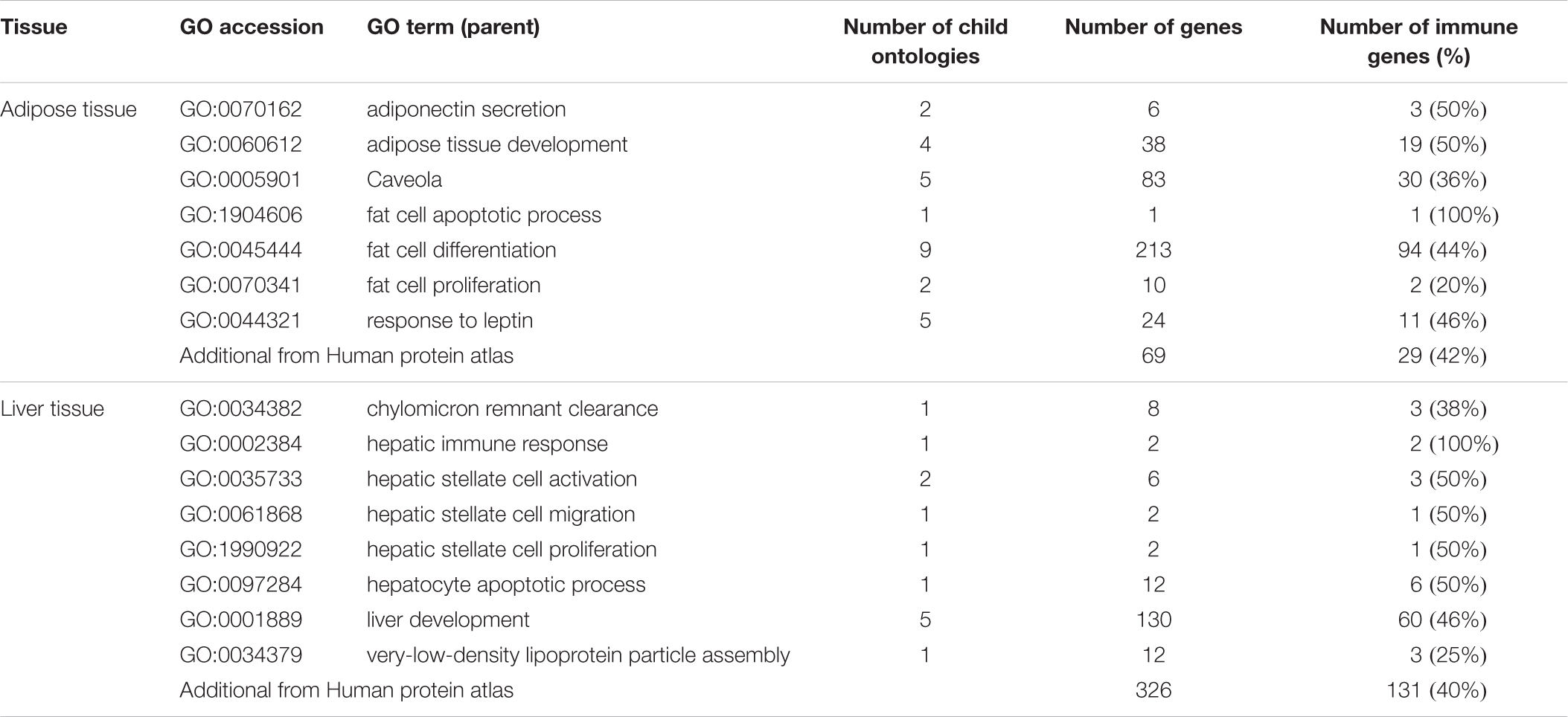
Table 1. Selected parent gene ontologies with the number of child ontologies and genes, as well as the number genes also present in immune related ontologies.
Additionally, a number of 79 adipose and 359 liver specific genes were retrieved from the Human Protein Atlas. Of these genes, 29 adipose and 131 liver specific immune-associated genes were a unique addition to the genes selected from GO.
Selection of Candidate Protein Biomarkers
Of the 404 adipose related genes selected from the Gene Ontology database and Human Protein Atlas database, 44 genes qualified as a curated candidate plasma protein biomarker of adipose tissue inflammation (Figure 1 and Table 2). Adipose processes that were highly represented included caveola (ADCY8, SELE, INSR, IRS1, TFPI, HMOX1, FASLG, NOS3), fat cell differentiation (SREBF1, METRNL, GPX1, RETN, TNF, FRZB, LPL, RARRES2, PPARG, ADIPOQ, SORT1, TGFB1, IL6), brown fat cell differentiation (ADIPOQ, FABP4, METRNL, FABP3, LEP, FTO, METRNL, FNDC5), white fat cell differentiation (FABP4, PPARG), and response to leptin (LEP, LEPR, FGF23, EDN1). Of the candidate biomarker list for adipose tissue LEP, ADIPOQ, LPL, FAB4, IL6, and PPARG are represented in the Human Protein Atlas as adipose tissue specific genes.
Of the 305 liver-related genes selected from the Gene Ontology and Human Protein Atlas databases, a number of 73 genes qualified as a curated candidate plasma protein biomarker for hepatic tissue inflammation (Figure 1 and Table 2). Highly manifest liver processes included chylomicron remnant clearance (LIPC, APOB, APOC3, APOC2, APOC1, APOE), liver regeneration (HAMP, CPB2, EGFR, REG1A, HMOX1, VTN, IL10), and very-low-density lipoprotein particle assembly (APOB, APOC3, APOC1). In the case of the candidate biomarkers, APOB, APOC3, APOC2, APOC1, APOE, GCLC, FGL1, HAMP, CPB2, VTN, PCSK9, APOB, APOC3, APOC1, were also present in Human Protein Atlas as tissue specific genes.
Verification in Obese Ldlr−/−.Leiden Mice
The first step of biomarker verification is based on their expression in tissue upon increased inflammatory conditions. To this end, curated candidate biomarkers (Table 2) for inflammation in liver and adipose tissue were determined in liver and adipose tissue after 50 weeks of HFD treatment and compared to the expression under healthy (chow-fed) conditions. As compared to their chow-fed counterparts, HFD feeding resulted in a strong induced hepatic inflammation, characterized by inflammatory cell aggregates of immune cells (Figure 2A). Quantification of the number of inflammatory aggregates showed that the HFD feeding resulted in a strong increase in the number of aggregates as compared to chow fed animal (31.6-fold increase, P < 0.01). High fat diet feeding also resulted in increased inflammation in perigonadal WAT depot as compared to the chow fed controls (Figure 2B). Quantification of the data shows that HFD feeding increased the number of crown-like structures (CLS)/mm2 perigonadal WAT (9.1-fold, p < 0.001). In all, these data show an increased inflammatory status in both liver and adipose tissue of mice fed a HFD as compared to chow fed controls.
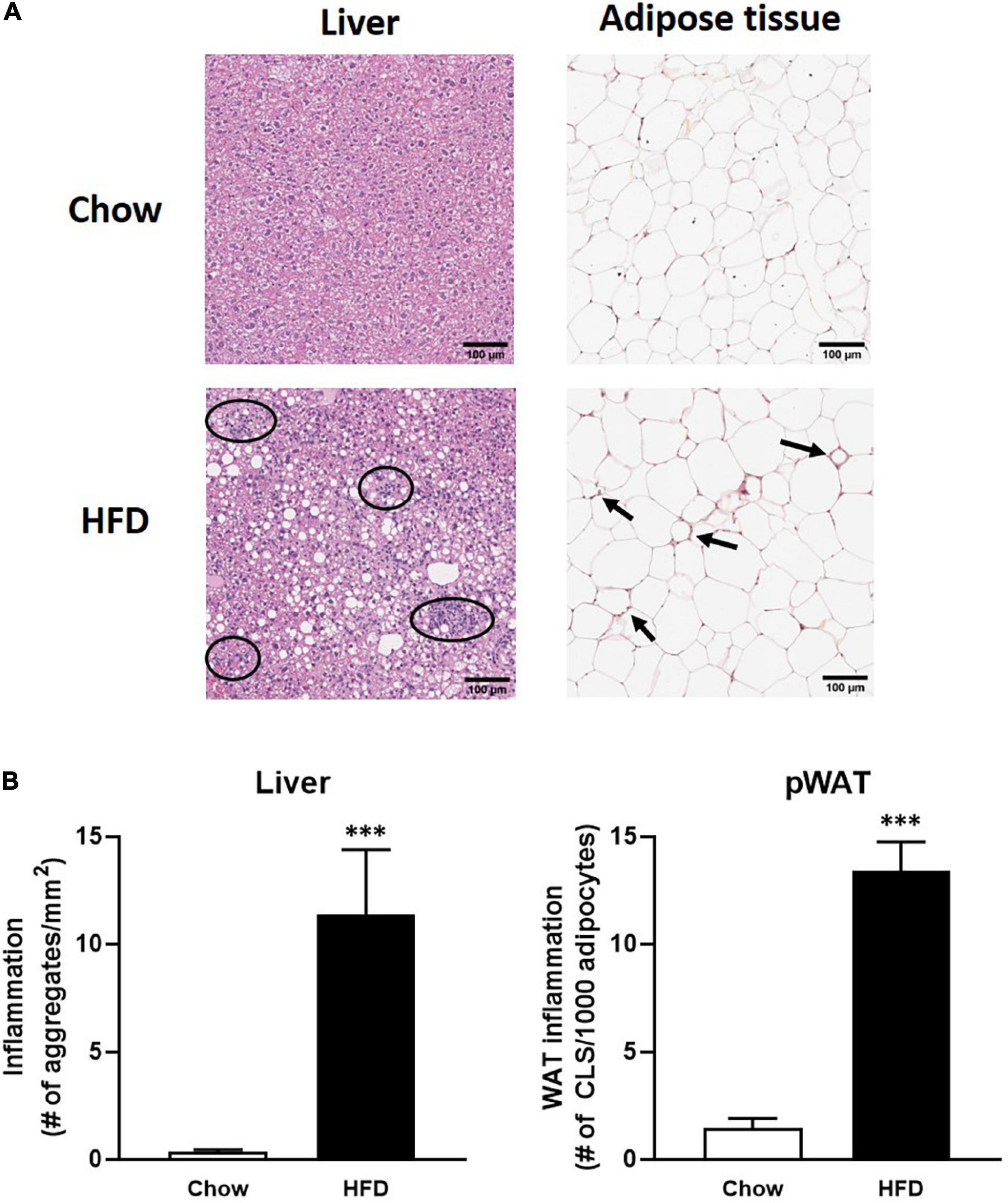
Figure 2. (A) Panels of immuno-histochemical staining of inflammatory aggregates in liver and adipose tissue; (B) quantification of inflammatory aggregates in HFD-fed mice (black bars) in liver (left) and adipose tissue (right) as compared to chow fed mice (open bars). ∗∗∗ indicates significance with P-values < 0.05.
Thereafter, the genes coding for the 44 adipose tissue-derived biomarker candidates and 73 liver tissue-derived biomarker candidates were analyzed in the inflamed tissues using RNAseq technology. A heatmap visualization of a selection of the candidate biomarker genes (see criteria in Materials and Methods section) is depicted in Figure 3. In inflamed livers from obese mice fed a HFD, the candidate biomarkers KRT18, SAA1, HP, GCLC, LEPR, LG1, IL27, GDF2, and IL18BP were significantly upregulated, whereas HRG, KLKB1, CPN2, PROC, SERPINA1, LDLR, KNG1, SERPINC1, SOD1, CAT, MBL2, APOC3, PCSK9, LIPC, SERPINA2, SDC1, A1BG, IGFBP2 and F7 are significantly downregulated in the inflamed livers. In the WAT from obese mice fed a HFD, FASLG expression was increased, while RETN, MMP9, PIK3CA, and FTO were significantly downregulated in the inflamed WAT.
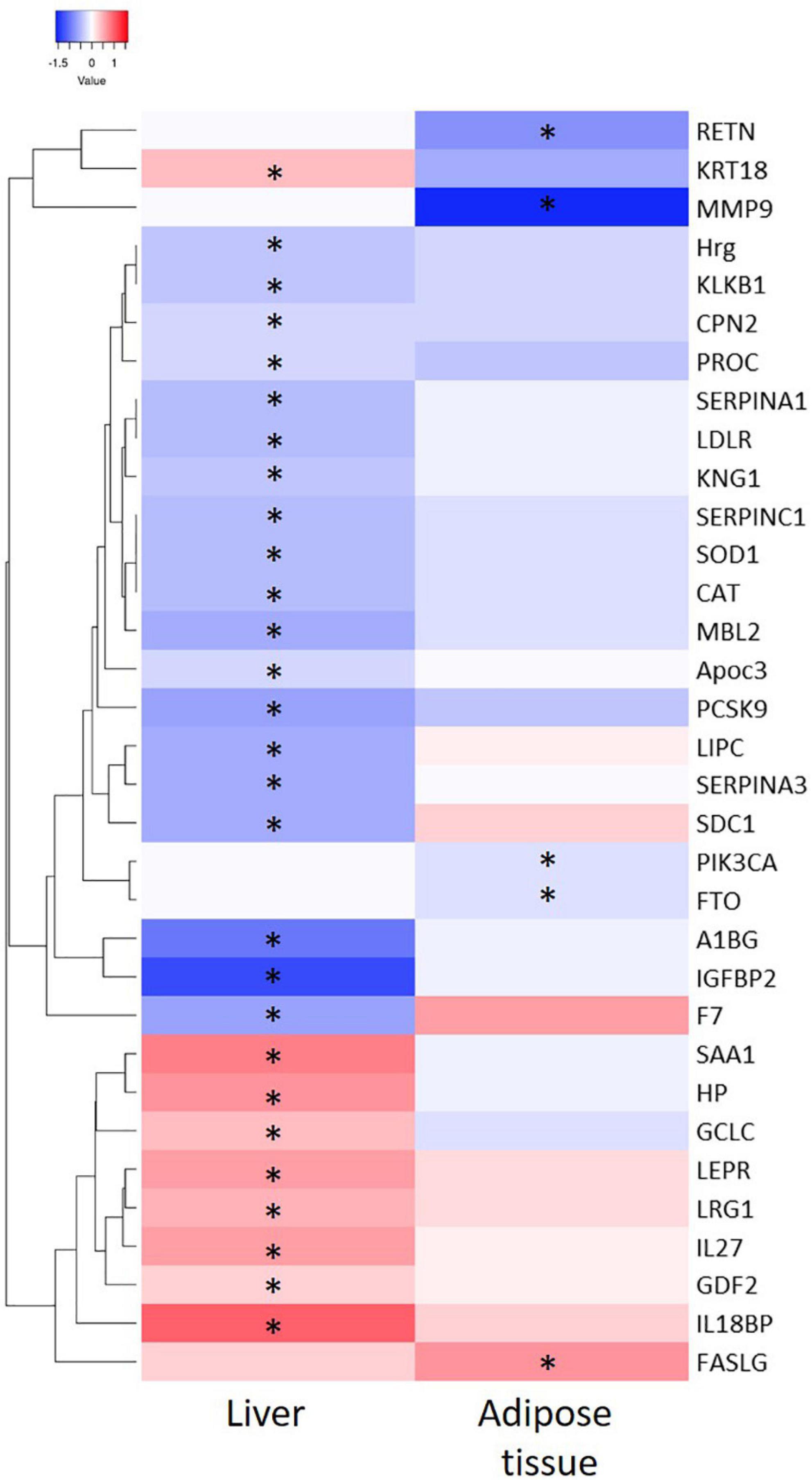
Figure 3. Heatmap representation of significant differential expressed genes from liver and adipose tissue and after 50 weeks of High Fat Diet feeding as compared to chow fed mice. ∗indicate significance of gene expression in specific tissue (P < 0.05).
Next step was to evaluate whether there is a relation between the differentially expressed biomarkers and the inflammatory state in liver and adipose tissue on the individual level. To this end we performed Spearman’s rank correlation to calculate the relation between the absolute gene expression in the tissue and the quantified inflammatory aggregates per animal (Table 3). This analysis shows a positive correlation of IL18BP, KRT18, SERPINA3, LRG1, LEPR, HP, and SAA1 with the level of inflammation in the liver and a negative correlation of IGFBP2, LIPC, MBL2, PCSK9, SERPINA1, SERPINC1, F7, LDLR, and CAT with the level of inflammation in the liver. In adipose tissue, a negative correlation of MMP9 and RETN expression was found with the level of inflammation.
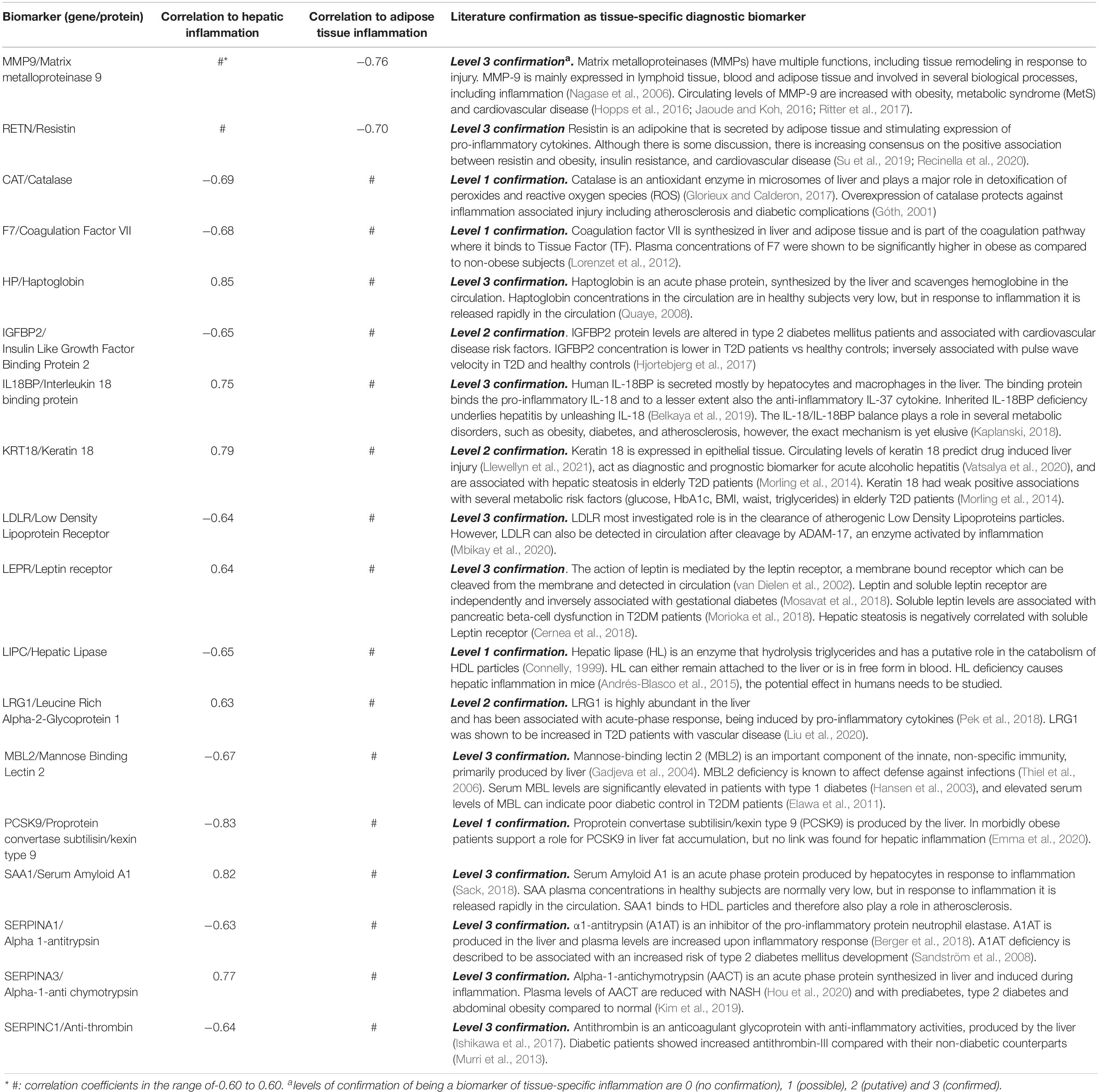
Table 3. Spearman’s Rank correlation between the amount of inflammation in the tissue (liver and adipose tissue) and the expression of the candidate marker based on gene expression.
To illustrate how a strong correlation between gene expression and inflammation of a specific gene can be translated in the concentration of a plasma marker SAA was analyzed. The experimental liver data shows a strong correlation between hepatic SAA1 expression and the number of hepatic inflammatory aggregates (Figure 4A) while a relation between adipose tissue gene expression and inflammation based on number of crown-like structures is clearly absent (Figure 4B). Biomarker analysis in plasma showed a good relation between hepatic inflammation and the concentration of plasma SAA (Figure 4C).
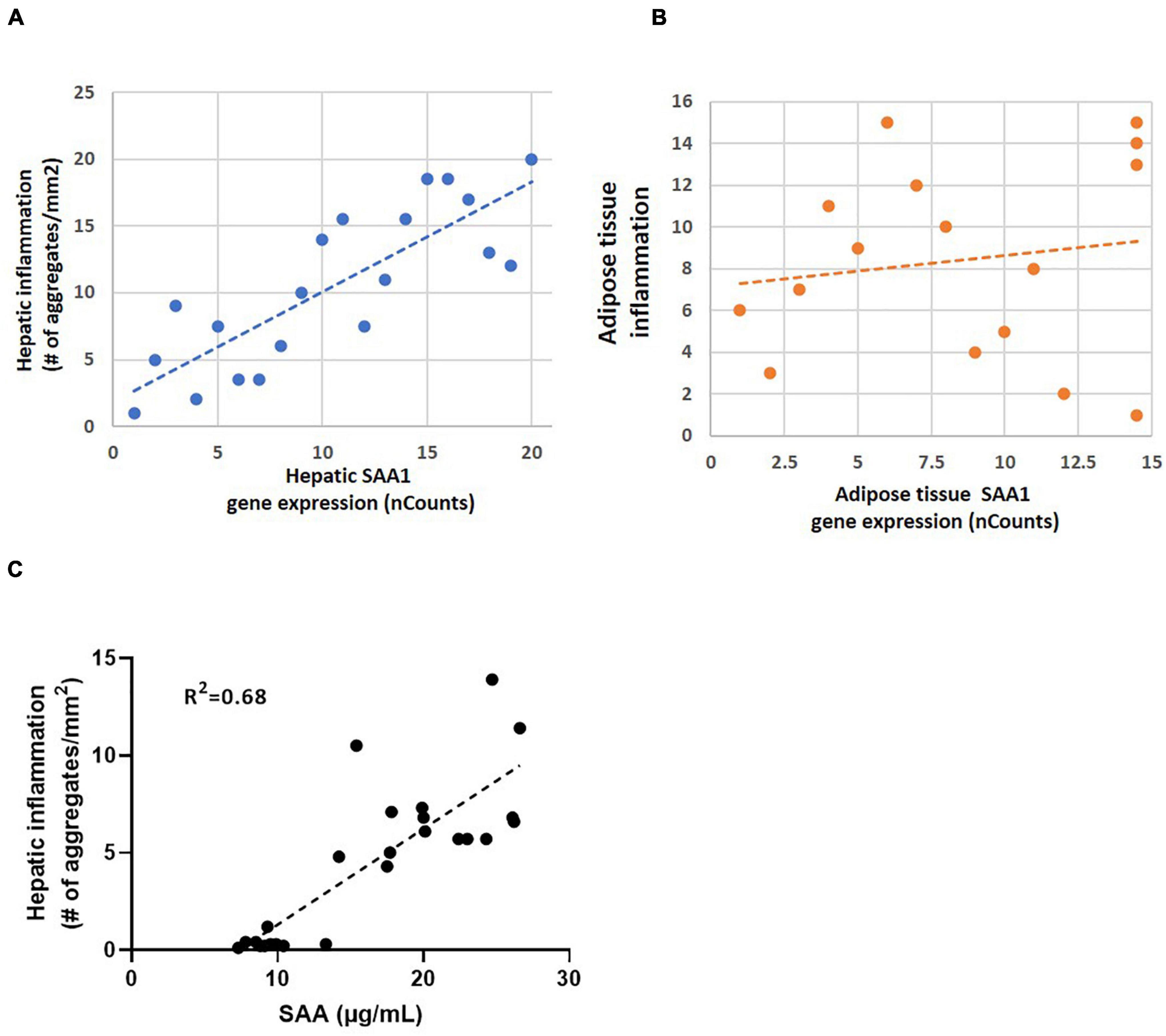
Figure 4. (A) Correlation analysis of hepatic inflammation based on immuno-histochemical staining of inflammatory aggregates in liver and hepatic SAA1 expression in mice (R2 = 0.82; P < 0.01); (B) Correlation analysis of adipose tissue inflammation based on immuno-histochemical staining of crown-like structures and adipose tissue SAA1 expression in mice (R2 = 0.15; P > 0.5); (C) Correlation analysis of hepatic inflammation in liver and plasma SAA1 levels (R2 = 0.68; P < 0.01).
Verification in Human Studies
The expression of 18 candidate biomarkers that correlated to inflammation in mice (Table 2, first three columns) could be further verified in literature as tissue-derived biomarker for adipose tissue and liver inflammation in humans (Table 3, last column). All 18 human candidate biomarkers could be more or less confirmed by literature: 4 out of 18 showed level 1 confirmation, 3 out of 18 showed level 2 confirmation, and 11 out of 18 showed level 3 confirmation.
As depicted in Table 3, the confirmed candidate biomarkers for inflammation in adipose tissue are linked to extracellular matrix processes (MMP-9) and to lipid metabolism (Resistin). The confirmed candidate liver inflammation biomarkers are linked to biological processes such as growth factors regulation (IGFBP2), serine proteases and inhibitors (coagulation factor VII, SERPINA1, SERPINA3, and SERPINC1), lipid metabolism (LEPR, LDLR, LIPC, and PCSK9), oxidative stress (Catalase), and immune response (SAA1, Haptoglobin, LRG1, MBL2, Keratin 18, and IL18BP). These data show that the in silico selection procedure, followed by in vivo verification in mice, results in a useful set of candidate biomarkers for human application.
Discussion
Chronic inflammatory diseases are recognized as the leading cause of death in industrialized countries today, with the majority of deaths accountable to inflammation-related diseases such as cardiovascular disease, diabetes mellitus, non-alcoholic fatty liver disease (NAFLD) and autoimmune and neurodegenerative conditions (Roth et al., 2018). Research has shown that obesity, similar to the most chronic diseases, is characterized by an inflammatory state reflected by elevated circulating levels of pro-inflammatory proteins (de Heredia et al., 2012). Both the local and systemic responses initiated by an inflammatory process can result in a dysbalance in metabolism in the tissues affected referred to as chronic low-grade inflammation. It is important to explore the general molecular mechanisms that integrate the immune response with systemic and local metabolic homeostasis and to identify biomarkers that reflect these pathways for early diagnosis and prevention/treatment of chronic metabolic diseases. Organ-specific candidate biomarkers of inflammation have been postulated previously by others in the context of many different diseases, however, whether these biomarkers are clinically validated or have diagnostic value remains to be clarified. Here, we describe an approach to predict and verify candidate biomarkers that can allow assessment of local inflammation in the context of metabolic imbalance as early indicators of chronic low-grade inflammation.
A workflow was created for screening of large databases (GO database and Human Protein Atlas) to determine the overlap between liver and adipose tissue-specific genes and inflammation-related genes. The overlapping genes/proteins were then selected based on their previous utilization as circulating biomarker in human clinical studies as indicated in the Clarivate Analytics biomarker database. Verification with murine gene expression data and human biomarker literature on tissue-derived low-grade inflammation indicated the feasibility of the workflow, represented by in a list of 18 biomarkers, of which 16 candidate plasma biomarkers were correlated with hepatic inflammation and 2 candidate biomarkers with adipose tissue inflammation. It must be noted that currently applied biomarkers for chronic low grade inflammation [e.g., Interleukin 6, adiponectin and soluble tumor necrosis factor-a receptor 2 (sTNFRII)] were not automatically selected in our verification procedure as they are not found to be tissue-specific and/or not expressed in mice as acute phase protein (C-reactive protein) and not fulfilling the selection criteria (e.g., differentially expressed as genes in inflamed tissue) as described in M&M.
Eleven out of 18 candidate biomarkers could be confirmed by human studies with the highest level of confirmation (Table 3). It should be noted that the number of verified candidate biomarkers was restricted to the genes that are also expressed in murine tissue (Figure 5) suggesting that the actual yield of the approach can be larger than described here.
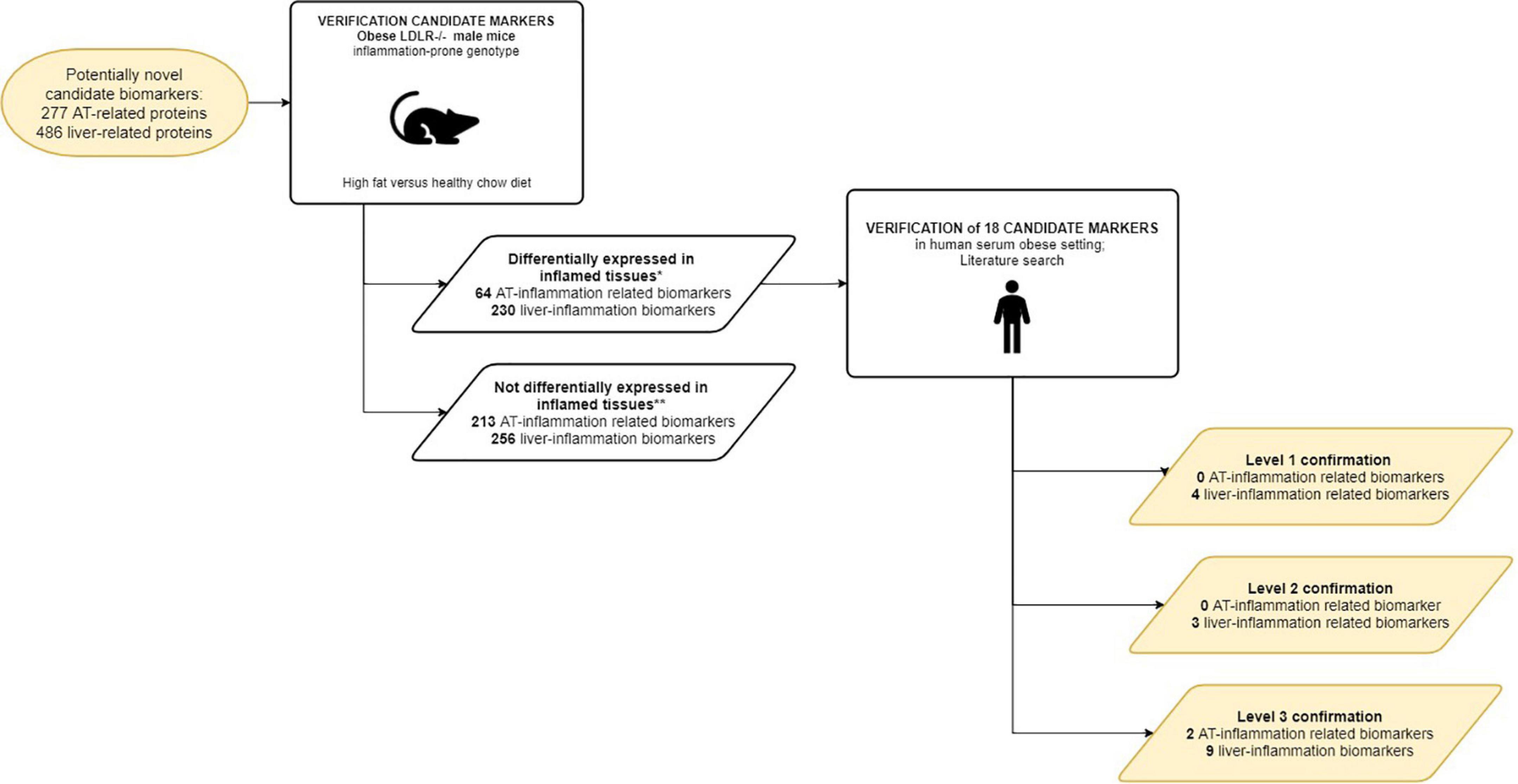
Figure 5. Workflow of the verification of the curated candidate biomarkers. Curated candidate biomarkers were checked for up/down regulated gene-expression in WAT and livers of obese mice. The curated candidate biomarkers were divided into 2 groups: markers differentially expressed in inflamed tissues; markers not differentially expressed in inflamed tissues. *Cut-off criteria described in M&M; ** Due to absence of murine analog of human candidate biomarker and/or lack of (differential) expression in murine tissues in RNAseq experiment.
The candidate biomarkers with the highest level of confirmation included extracellular matrix remodeling proteins, serine proteases (inhibitors), lipid metabolism related proteins, and mediators of immune activation (Table 4). Further study of the selected candidate biomarkers confirmed the selection procedure:

Table 4. Biological function of the 11 human candidate biomarkers with the highest level of confirmation (level 3).
MMP-9 is related to remodeling of extracellular matrix processes and inflammation. MMP-9 regulates inflammatory processes by its proteolytic activity (Manicone and Mcguire, 2008) and circulating levels of MMP-9 are increased in obesity, metabolic syndrome (MetS) and cardiovascular disease (Hopps et al., 2016; Jaoude and Koh, 2016; Ritter et al., 2017).
Serine protease inhibitors (SERPINs) are key regulators of numerous biological pathways that are principally involved in regulation of the inflammatory cascades by enzyme activity modification, fibrinolysis, complement activation and kinin release. Alpha1-antitrypsin (a1AT) is produced in the liver and protects tissues from damage caused by proteolytic enzymes of inflammatory cells, especially neutrophil elastase (Janciauskiene et al., 2018). Similarly, alpha-1-anti chymotrypsin protects tissues from damage by inhibiting the activity of cathepsin G that is found in neutrophils and chymases found in mast cells (Kalsheker, 1996). Antithrombin, for example modulates inflammatory responses not only by inhibiting thrombin and other clotting factors that induce cytokine activity and leukocyte endothelial cell interaction, but also by coagulation independent effects, including direct interaction with cellular mediators of inflammation (Levy et al., 2016). Together, these findings confirm the selection procedure by which these 3 members of the SERPIN family were selected as candidate biomarkers correlated with hepatic inflammation.
Three of the highest level candidate biomarkers that were negatively associated with inflammation, were involved in lipid metabolism, viz. LDLR, LEPR, and RETN coding, respectively, for low density lipoprotein receptor, leptin receptor and resistin. Resistin is a pro-inflammatory adipokine associated with insuline resistance and obesity (Bokarewa et al., 2005; Su et al., 2019). LDLR and LEPR are in part related to triglyceride and cholesterol metabolism in the liver. The hepatic LDL receptor plays an important role in the clearance of cholesterol-rich LDL lipoproteins from circulation to the liver (Brown and Goldstein, 1983). We have shown, in animal studies that increased clearance of these cholesterol-rich LDL particles contribute to increased hepatic inflammatory response (Kleemann et al., 2007). Leptin is a circulating adipokine derived from adipose tissue regulating food intake, insulin action and modulation of the immune system. These biological processes are initiated by the leptin receptor upon interaction with circulating leptin. In parallel, Leptin receptor is known to activate Janus kinase-STAT3 pathway (Ikejima et al., 2004) which contributes to an inflammatory response (Kubler, 2014).
Four of the selected candidate biomarkers are involved in direct activation of the immune pathways. Haptoglobin and SAA1 are well-known acute phase proteins produced by hepatocytes. Their plasma levels are increased rapidly in subjects following an inflammatory trigger. Our data shows their gene expression is positively correlated with inflammation in liver. Mannose-binding lectin 2 plays an important role in the innate, non-specific immunity, by activating complement resulting among others in cell lysis, phagocytosis, and inflammation (Beltrame et al., 2015). IL-18 Binding Protein was positively associated with hepatic inflammation, which could be confirmed by literature. IL18BP binds and neutralizes the pro-inflammatory cytokine IL-18, thereby inhibiting IL18-induced IFNgamma production (Dinarello et al., 2013).
The approach described here is promising, but the verification of this approach also has its limitations. One important limitation is that samples from human studies with well-characterized tissue inflammation and corresponding serum/plasma samples were not available to validate the biomarkers. Alternatively we used published human studies to validate the presence of predicted biomarkers in serum/plasma, and used animal data to link biomarkers to tissue inflammation. TAlso, it is known that literature is biased toward studies in which effects were observed and raw datasets are often not made available thereby limiting direct validation possibilities. As an alternative, we have used mouse tissue and plasma samples from preclinical studies, which were limited by the use of one particular mouse strain, the use of one particular diet instead of multiple different liver and adipose tissue evoking diets, and the use of one gender (male mice). Another important consideration may be that biomarkers could be synthesized before inflammation in liver or adipose tissue becomes manifest. Therefore it could be possible that biomarkers do not always correlate with cellular inflammatory features. Therefore, future studies for the identification of early markers of tissue inflammation would require dedicated studies (preferably time resolved).
One important issue is the validity to translate the experimental murine data to humans (Perlman, 2016), given the known difference in metabolism of mice and humans. It is known that the metabolic rate of mice version human is closely correlated with size, thus a 30 g mouse has a specific metabolic rate (metabolic rate per gram of tissue) roughly seven times that of a 70-kg human (Kleiber, 1961; Schmidt-Nielsen and Knut, 1984). Mice have relatively higher amounts of metabolically active tissues, such as liver and kidney and differ in the mitochondrial density and metabolic rate and also in the fatty acid composition of their membrane phospholipids [higher contents of polyunsaturated fatty acid docosahexaenoic acid (Hulbert, 2008)]. Despite these differences in metabolism between mice and humans, we have shown previously that the key molecular inflammatory responses in obese Ldlr−/−.Leiden mice and humans are similar (Morrison et al., 2018): the hepatic inflammatory response in NASH patients (non-alcohol steatohepatitis; due to an increased metabolic load) has large similarities to the obese Ldlr−/−.Leiden mice fed with a HFD demonstrated by the activation of the majority of identical inflammatory processes and master regulators (e.g., TNF, CSF2, TGFB1) (Morrison et al., 2018). Moreover, the candidate biomarkers that were verified in the obese Ldlr−/−.Leiden mice were further evaluated for their confirmed use as hepatic or adipose inflammation in human, albeit in a different disease context. Therefore we believe it is valid to translate the identified biomarkers to the human situation, although they still require validation in a human study.
Our study shows that by combining prior knowledge from multiple studies we were able to select and verify a set of in silico predicted biomarkers for liver and adipose tissue-derived inflammation. These biomarkers may be of great help to form a starting point to clarify tissue-specific inflammatory mechanisms. Tissue-specific chronic low-grade inflammation is an important underlying contributor to complications of metabolic disorders. To support therapeutic approaches to these complications, it is crucial to gain a deeper understanding of the inflammatory dynamics and to monitor them on the individual level. A hugh advantage of our approach is that our workflow is for a substantial part automated (database searches) which enables the screening of enormous amounts of data, thereby saving time and resources.
As one of the critical selection criteria of our approach was the selection based on the ability to detect these candidate markers as proteins in human plasma. This made it plausible that these candidate biomarkers can easily be implemented in future human studies to monitor or predict liver- and adipose tissue inflammation. This will become of great value to study future development of therapeutic/lifestyle interventions to combat metabolic inflammatory complications. Our next step will be to validate these selected candidate biomarkers in a human nutritional study as proof-of-concept of our approach.
Conclusion
We describe a promising Systems Biology approach that predicts tissue-derived, blood-based biomarkers reflecting liver- and adipose tissue inflammation that may be of great use to gain more mechanistic knowledge on tissue-specific inflammation and to monitor or predict the efficacy of interventions in metabolic inflammatory conditions.
Data Availability Statement
The original contributions presented in the study are included in the article/Supplementary Material, further inquiries can be directed to the corresponding author.
Ethics Statement
The animal study was reviewed and approved by Animal experimental commission TNO, approval reference number TNO-312.
Author Contributions
JB, WB, SW, and LV contributed to the conception and design of the study. RD and MC wrote scripts for literature database searches and biomarker selection. AH and RK contributed to the animal experiment. JB, WB, and LV wrote the manuscript. All authors contributed to manuscript revision, read, and approved the submitted version.
Funding
This work was conducted within the TNO early research program Personalized Health (ERP-PH) and the roadmap Biomedical Health 2020 (PMC#2).
Conflict of Interest
The authors declare that the research was conducted in the absence of any commercial or financial relationships that could be construed as a potential conflict of interest.
Publisher’s Note
All claims expressed in this article are solely those of the authors and do not necessarily represent those of their affiliated organizations, or those of the publisher, the editors and the reviewers. Any product that may be evaluated in this article, or claim that may be made by its manufacturer, is not guaranteed or endorsed by the publisher.
Supplementary Material
The Supplementary Material for this article can be found online at: https://www.frontiersin.org/articles/10.3389/fphys.2021.703370/full#supplementary-material
Footnotes
References
Andrés-Blasco, I., Herrero-Cervera, A., Vinué, Á., Martínez-Hervás, S., Piqueras, L., Sanz, M. J., et al. (2015). Hepatic lipase deficiency produces glucose intolerance, inflammation and hepatic steatosis. J. Endocrinol. 227, 179–191. doi: 10.1530/JOE-15-0219
Belkaya, S., Michailidis, E., Korol, C. B., Kabbani, M., Cobat, A., Bastard, P., et al. (2019). Inherited IL-18BP deficiency in human fulminant viral hepatitis. J. Exp. Med. 216, 1777–1790. doi: 10.1084/jem.20190669
Beltrame, M. H., Catarino, S. J., Goeldner, I., Boldt, A. B. W., and de Messias-Reason, I. J. (2015). The lectin pathway of complement and rheumatic heart disease. Front. Pediatr. 2:148. doi: 10.3389/fped.2014.00148
Berger, M., Liu, M., Uknis, M. E., and Koulmanda, M. (2018). Alpha-1-antitrypsin in cell and organ transplantation. Am. J. Transplant. 18, 1589–1595. doi: 10.1111/ajt.14756
Bokarewa, M., Nagaev, I., Dahlberg, L., Smith, U., and Tarkowski, A. (2005). Resistin, an adipokine with potent proinflammatory properties. J. Immunol. 174, 5789–5795. doi: 10.4049/jimmunol.174.9.5789
Brown, M. S., and Goldstein, J. L. (1983). Lipoprotein receptors in the liver. Control signals for plasma cholesterol traffic. J. Clin. Invest. 72, 743–747. doi: 10.1172/JCI111044
Cernea, S., Roiban, A. L., Both, E., and Huţanu, A. (2018). Serum leptin and leptin resistance correlations with NAFLD in patients with type 2 diabetes. Diabetes Metab. Res. Rev. 34:e3050. doi: 10.1002/dmrr.3050
Connelly, P. W. (1999). The role of hepatic lipase in lipoprotein metabolism. Clin. Chim. Acta 286, 243–255. doi: 10.1016/s0009-8981(99)00105-9
de Heredia, F. P., Gómez-Martínez, S., and Marcos, A. (2012). Obesity, inflammation and the immune system. Proc. Nutr. Soc. 71, 332–338. doi: 10.1017/S0029665112000092
Dinarello, C. A., Novick, D., Kim, S., and Kaplanski, G. (2013). Interleukin-18 and IL-18 binding protein. Front. Immunol. 4:289. doi: 10.3389/fimmu.2013.00289
Elawa, G., AoudAllah, A. M., Hasaneen, A. E., and El-Hammady, A. M. (2011). The predictive value of serum mannan-binding lectin levels for diabetic control and renal complications in type 2 diabetic patients. Saudi Med. J. 32, 784–790.
Emma, M. R., Giannitrapani, L., Cabibi, D., Porcasi, R., Pantuso, G., Augello, G., et al. (2020). Hepatic and circulating levels of PCSK9 in morbidly obese patients: relation with severity of liver steatosis. Biochim. Biophys. Acta 1865:158792. doi: 10.1016/j.bbalip.2020.158792
Gadjeva, M., Takahashi, K., and Thiel, S. (2004). Mannan-binding lectin–a soluble pattern recognition molecule. Mol. Immunol. 41, 113–121. doi: 10.1016/j.molimm.2004.03.015
García-Campos, M. A., Espinal-Enríquez, J., and Hernández-Lemus, E. (2015). Pathway analysis: state of the Art. Front. Physiol. 6:383. doi: 10.3389/fphys.2015.00383
Glorieux, C., and Calderon, P. B. (2017). Catalase, a remarkable enzyme: targeting the oldest antioxidant enzyme to find a new cancer treatment approach. Biol. Chem. 398, 1095–1108. doi: 10.1515/hsz-2017-0131
Góth, L. A. (2001). New type of inherited catalase deficiencies: its characterization and comparison to the Japanese and Swiss type of Acatalasemia. Blood Cells Mol. Dis. 27, 512–517. doi: 10.1006/bcmd.2001.0415
Gregor, M. F., and Hotamisligil, G. S. (2011). Inflammatory mechanisms in obesity. Annu. Rev. Immunol. 29, 415–445. doi: 10.1146/annurev-immunol-031210-101322
Hansen, T. K., Thiel, S., Knudsen, S. T., Gravholt, C. H., Christiansen, J. S., Mogensen, C. E., et al. (2003). Elevated levels of mannan-binding lectin in patients with type 1 diabetes. J. Clin. Endocrinol. Metab. 88, 4857–4861. doi: 10.1210/jc.2003-030742
Hjortebjerg, R., Laugesen, E., Hoyem, P., Oxvig, C., Stausbøl-Gron, B., Knudsen, S. T., et al. (2017). The IGF system in patients with type 2 diabetes: associations with markers of cardiovascular target organ damage. Eur. J. Endocrinol. 176, 521–531. doi: 10.1530/EJE-16-0940
Hopps, E., Canino, B., Montana, M., Calandrino, V., Urso, C., Lo Presti, R., et al. (2016). Gelatinases and their tissue inhibitors in a group of subjects with obstructive sleep apnea syndrome. Clin. Hemorheol. Microcirc. 62, 27–34. doi: 10.3233/CH-151928
Hotamisligil, G. S. (2006). Inflammation and metabolic disorders. Nature 444, 860–867. doi: 10.1038/nature05485
Hotamisligil, G. S. (2017). Inflammation, metaflammation and immunometabolic disorders. Nature 542, 177–185. doi: 10.1038/nature21363
Hotamisligil, G. S., and Erbay, E. (2008). Nutrient sensing and inflammation in metabolic diseases. Nat. Rev. Immunol. 8, 923–934. doi: 10.1038/nri2449
Hou, W., Janech, M. G., Sobolesky, P. M., Bland, A. M., Samsuddin, S., Alazawi, W., et al. (2020). Proteomic screening of plasma identifies potential noninvasive biomarkers associated with significant/advanced fibrosis in patients with nonalcoholic fatty liver disease. Biosci. Rep. 40, 1–8. doi: 10.1042/BSR20190395
Hulbert, A. J. (2008). The links between membrane composition, metabolic rate and lifespan. Comp. Biochem. Physiol. Part A Mol. Integr. Physiol. 150, 196–203. doi: 10.1016/j.cbpa.2006.05.014
Ikejima, K., Lang, T., Zhang, Y.-J., Yamashina, S., Honda, H., Yoshikawa, M., et al. (2004). Expression of leptin receptors in hepatic sinusoidal cells. Comp. Hepatol. 3, (Suppl. 1), S12. doi: 10.1186/1476-5926-2-S1-S12
Ishikawa, M., Yamashita, H., Oka, N., Ueda, T., Kohama, K., Nakao, A., et al. (2017). Antithrombin III improved neutrophil extracellular traps in lung after the onset of endotoxemia. J. Surg. Res. 208, 140–150. doi: 10.1016/j.jss.2016.09.041
Janciauskiene, S., Wrenger, S., Immenschuh, S., Olejnicka, B., Greulich, T., Welte, T., et al. (2018). The multifaceted effects of alpha1-antitrypsin on neutrophil functions. Front. Pharmacol. 9:341. doi: 10.3389/fphar.2018.00341
Jaoude, J., and Koh, Y. (2016). Matrix metalloproteinases in exercise and obesity. Vasc. Health Risk Manag. 12, 287–295. doi: 10.2147/VHRM.S103877
Kalsheker, N. A. (1996). α1-antichymotrypsin. Int. J. Biochem. Cell Biol. 28, 961–964. doi: 10.1016/1357-2725(96)00032-5
Kaplanski, G. (2018). Interleukin-18: biological properties and role in disease pathogenesis. Immunol. Rev. 281, 138–153. doi: 10.1111/imr.12616
Kelesidis, I., Kelesidis, T., and Mantzoros, C. S. (2006). Adiponectin and cancer: a systematic review. Br. J. Cancer 94, 1221–1225. doi: 10.1038/sj.bjc.6603051
Khatri, P., Sirota, M., and Butte, A. J. (2012). Ten years of pathway analysis: current approaches and outstanding challenges. PLoS Comput. Biol. 8:e1002375. doi: 10.1371/journal.pcbi.1002375
Kim, S. W., Choi, J.-W., Yun, J. W., Chung, I.-S., Cho, H. C., Song, S.-E., et al. (2019). Proteomics approach to identify serum biomarkers associated with the progression of diabetes in Korean patients with abdominal obesity. PLoS One 14:e0222032. doi: 10.1371/journal.pone.0222032
Kleemann, R., Verschuren, L., van Erk, M. J., Nikolsky, Y., Cnubben, N. H., Verheij, E. R., et al. (2007). Atherosclerosis and liver inflammation induced by increased dietary cholesterol intake: a combined transcriptomics and metabolomics analysis. Genome Biol. 8:R200. doi: 10.1186/gb-2007-8-9-r200
Kleiber, M. (1961). The Fire of Life. An Introduction to Animal Energetics. New York, NY: John Wiley & Sons, Inc.
Kubler, P. (2014). Experimental and Clinical Pharmacology:Janus kinase inhibitors: mechanisms of action. Aust. Prescr. 37, 154–157. doi: 10.18773/austprescr.2014.061
Levy, J. H., Sniecinski, R. M., Welsby, I. J., and Levi, M. (2016). Antithrombin: anti-inflammatory properties and clinical applications. Thromb. Haemost. 115, 712–728. doi: 10.1160/TH15-08-0687
Li, S., Shin, H. J., Ding, E. L., and van Dam, R. M. (2009). Adiponectin levels and risk of type 2 Diabetes. JAMA 302, 179–188. doi: 10.1001/jama.2009.976
Liu, C., Teo, M. H. Y., Pek, S. L. T., Wu, X., Leong, M. L., Tay, H. M., et al. (2020). A Multifunctional role of Leucine-Rich α-2-glycoprotein 1 in cutaneous wound healing under normal and diabetic conditions. Diabetes 69, 2467–2480. doi: 10.2337/db20-0585
Llewellyn, H. P., Vaidya, V. S., Wang, Z., Peng, Q., Hyde, C., Potter, D., et al. (2021). Evaluating the sensitivity and specificity of promising circulating biomarkers to diagnose liver injury in humans. Toxicol. Sci. 181, 23–34. doi: 10.1093/toxsci/kfab003
Lorenzet, R., Napoleone, E., Cutrone, A., and Donati, M. B. (2012). Thrombosis and obesity: cellular bases. Thromb. Res. 129, 285–289. doi: 10.1016/j.thromres.2011.10.021
Love, M. I., Huber, W., and Anders, S. (2014). Moderated estimation of fold change and dispersion for RNA-seq data with DESeq2. Genome Biol. 15:550. doi: 10.1186/s13059-014-0550-8
Manicone, A., and Mcguire, J. (2008). Matrix metalloproteinases as modulators of inflammation. Semin. Cell Dev. Biol. 19, 34–41. doi: 10.1016/j.semcdb.2007.07.003
Mbikay, M., Mayne, J., and Chrétien, M. (2020). The enigma of soluble LDLR: Could inflammation be the key? Lipids Health Dis. 19:17. doi: 10.1186/s12944-020-1199-9
Mcdermott, J. E., Wang, J., Mitchell, H., Hafen, R., Ramey, J., and Rodland, K. D. (2013). Challenges in biomarker discovery. Expert Opin. Med. Diagn. 7, 37–51. doi: 10.1517/17530059.2012.718329.Challenges
Meijerink, M., van den Broek, T., Dulos, R., Neergaard Jacobsen, L., Staudt Kvistgaard, A., Garthoff, J., et al. (2019). The impact of immune interventions: a systems biology strategy for predicting adverse and beneficial immune effects. Front. Immunol. 10:231. doi: 10.3389/fimmu.2019.00231
Minihane, A. M., Vinoy, S., Russell, W. R., Baka, A., Roche, H. M., Tuohy, K. M., et al. (2015). Low-grade inflammation, diet composition and health: current research evidence and its translation. Br. J. Nutr. 114, 999–1012. doi: 10.1017/S0007114515002093
Morioka, T., Emoto, M., Yamazaki, Y., Kurajoh, M., Motoyama, K., Mori, K., et al. (2018). Plasma soluble leptin receptor levels are associated with pancreatic β-cell dysfunction in patients with type 2 diabetes. J. Diabetes Investig. 9, 55–62. doi: 10.1111/jdi.12657
Morling, J. R., Fallowfield, J. A., Williamson, R. M., Nee, L. D., Jackson, A. P., Glancy, S., et al. (2014). Non-invasive hepatic biomarkers (ELF and CK18) in people with type 2 diabetes: the Edinburgh type 2 diabetes study. Liver Int. 34, 1267–1277. doi: 10.1111/liv.12385
Morrison, M. C., Kleemann, R., van Koppen, A., Hanemaaijer, R., and Verschuren, L. (2018). Key inflammatory processes in human NASH are reflected in Ldlr−/−.Leiden Mice: a translational gene profiling study. Front. Physiol. 9:132. doi: 10.3389/fphys.2018.00132
Mosavat, M., Omar, S. Z., Tan, P. C., Razif, M. F. M., and Sthaneshwar, P. (2018). Leptin and soluble leptin receptor in association with gestational diabetes: a prospective case–control study. Arch. Gynecol. Obstet. 297, 797–803. doi: 10.1007/s00404-017-4617-0
Murri, M., Insenser, M., Bernal-Lopez, M. R., Perez-Martinez, P., Escobar-Morreale, H. F., and Tinahones, F. J. (2013). Proteomic analysis of visceral adipose tissue in pre-obese patients with type 2 diabetes. Mol. Cell. Endocrinol. 376, 99–106. doi: 10.1016/j.mce.2013.06.010
Nagase, H., Visse, R., and Murphy, G. (2006). Structure and function of matrix metalloproteinases and TIMPs. Cardiovasc. Res. 69, 562–573. doi: 10.1016/j.cardiores.2005.12.002
Pek, S. L. T., Cheng, A. K. S., Lin, M. X., Wong, M. S., Chan, E. Z. L., Moh, A. M. C., et al. (2018). Association of circulating proinflammatory marker, leucine-rich-α2-glycoprotein (LRG1), following metabolic/bariatric surgery. Diabetes Metab. Res. Rev. 34:e3029. doi: 10.1002/dmrr.3029
Perlman, R. L. (2016). Mouse models of human disease: an evolutionary perspective. Evol. Med. Public Heal. 2016, 170–176. doi: 10.1093/emph/eow014
Pradhan, A. D. (2001). C-Reactive Protein, Interleukin 6, and risk of developing type 2 Diabetes Mellitus. JAMA 286, 327–334. doi: 10.1001/jama.286.3.327
Quaye, I. K. (2008). Haptoglobin, inflammation and disease. Trans. R. Soc. Trop. Med. Hyg. 102, 735–742. doi: 10.1016/j.trstmh.2008.04.010
Recinella, L., Orlando, G., Ferrante, C., Chiavaroli, A., Brunetti, L., and Leone, S. (2020). Adipokines: new potential therapeutic target for obesity and metabolic, rheumatic, and cardiovascular diseases. Front. Physiol. 11:578966. doi: 10.3389/fphys.2020.578966
Ritter, A. M. V., de Faria, A. P., Barbaro, N., Sabbatini, A. R., Corrêa, N. B., Brunelli, V., et al. (2017). Crosstalk between obesity and MMP-9 in cardiac remodelling –a cross-sectional study in apparent treatment-resistant hypertension. Blood Press 26, 122–129. doi: 10.1080/08037051.2016.1249336
Roth, G. A., Abate, D., Abate, K. H., Abay, S. M., Abbafati, C., Abbasi, N., et al. (2018). Global, regional, and national age-sex-specific mortality for 282 causes of death in 195 countries and territories, 1980–2017: a systematic analysis for the Global Burden of Disease Study 2017. Lancet 392, 1736–1788. doi: 10.1016/S0140-6736(18)32203-7
Sandström, C. S., Ohlsson, B., Melander, O., Westin, U., Mahadeva, R., and Janciauskiene, S. (2008). An association between Type 2 diabetes and alpha-antitrypsin deficiency. Diabet. Med. 25, 1370–1373. doi: 10.1111/j.1464-5491.2008.02584.x
Schmidt-Nielsen, K., and Knut, S.-N. (1984). Scaling: Why is Animal Size So Important? Cambridge: Cambridge University Press.
Shibata, R., Ouchi, N., and Murohara, T. (2009). Adiponectin and cardiovascular disease. Circ. J. 73, 608–614. doi: 10.1253/circj.CJ-09-0057
Stefan, N., Birkenfeld, A. L., and Schulze, M. B. (2021). Global pandemics interconnected — obesity, impaired metabolic health and COVID-19. Nat. Rev. Endocrinol. 17, 135–149. doi: 10.1038/s41574-020-00462-1
Su, K. Z., Li, Y. R., Zhang, D., Yuan, J. H., Zhang, C. S., Liu, Y., et al. (2019). Relation of circulating resistin to insulin resistance in type 2 diabetes and obesity: a systematic review and meta-analysis. Front. Physiol. 10: 1399. doi: 10.3389/fphys.2019.01399
Thiel, S., Frederiksen, P. D., and Jensenius, J. C. (2006). Clinical manifestations of mannan-binding lectin deficiency. Mol. Immunol. 43, 86–96. doi: 10.1016/j.molimm.2005.06.018
Thorand, B., Kolb, H., Baumert, J., Koenig, W., Chambless, L., Meisinger, C., et al. (2005). Elevated Levels of Interleukin-18 Predict the Development of Type 2 diabetes: results from the MONICA/KORA Augsburg study, 1984-2002. Diabetes 54, 2932–2938. doi: 10.2337/diabetes.54.10.2932
Vafaee, F., Diakos, C., Kirschner, M. B., Reid, G., Michael, M. Z., Horvath, L. G., et al. (2018). A data-driven, knowledge-based approach to biomarker discovery: application to circulating microRNA markers of colorectal cancer prognosis. NPJ Syst. Biol. Appl. 4:20. doi: 10.1038/s41540-018-0056-1
van den Hoek, A. M., de Jong, J. C. B. C., Worms, N., van Nieuwkoop, A., Voskuilen, M., Menke, A. L., et al. (2021). Diet and exercise reduce pre-existing NASH and fibrosis and have additional beneficial effects on the vasculature, adipose tissue and skeletal muscle via organ-crosstalk. Metabolism 124:154873. doi: 10.1016/j.metabol.2021.154873
van den Hoek, A. M., Verschuren, L., Worms, N., van Nieuwkoop, A., de Ruiter, C., Attema, J., et al. (2020). A translational mouse model for NASH with advanced fibrosis and atherosclerosis expressing key pathways of human pathology. Cells 9:2014. doi: 10.3390/cells9092014
van Dielen, F. M. H., van ‘t Veer, C., Buurman, W. A., and Greve, J. W. M. (2002). Leptin and soluble leptin receptor levels in obese and weight-losing individuals. J. Clin. Endocrinol. Metab. 87, 1708–1716. doi: 10.1210/jcem.87.4.8381
Vatsalya, V., Cave, M. C., Kong, M., Gobejishvili, L., Falkner, K. C., Craycroft, J., et al. (2020). Keratin 18 is a diagnostic and prognostic factor for acute alcoholic hepatitis. Clin. Gastroenterol. Hepatol. 18, 2046–2054. doi: 10.1016/j.cgh.2019.11.050
Keywords: mechanism, low-grade inflammation, blood-based biomarker, metabolic disease, lifestyle intervention
Citation: van Bilsen JHM, van den Brink W, van den Hoek AM, Dulos R, Caspers MPM, Kleemann R, Wopereis S and Verschuren L (2021) Mechanism-Based Biomarker Prediction for Low-Grade Inflammation in Liver and Adipose Tissue. Front. Physiol. 12:703370. doi: 10.3389/fphys.2021.703370
Received: 30 April 2021; Accepted: 18 October 2021;
Published: 10 November 2021.
Edited by:
Maria Elena Soto, Instituto Nacional de Cardiologia Ignacio Chavez, MexicoReviewed by:
Elisa Villalobos, University of Edinburgh, United KingdomRoel Tans, Maastricht University, Netherlands
Copyright © 2021 van Bilsen, van den Brink, van den Hoek, Dulos, Caspers, Kleemann, Wopereis and Verschuren. This is an open-access article distributed under the terms of the Creative Commons Attribution License (CC BY). The use, distribution or reproduction in other forums is permitted, provided the original author(s) and the copyright owner(s) are credited and that the original publication in this journal is cited, in accordance with accepted academic practice. No use, distribution or reproduction is permitted which does not comply with these terms.
*Correspondence: Lars Verschuren, lars.verschuren@tno.nl