- 1Institute of Biomedical Engineering, Department of Engineering Science, University of Oxford, Oxford, United Kingdom
- 2Institute for Systems and Robotics - Lisboa and Department of Bioengineering, Instituto Superior Técnico, Universidade de Lisboa, Lisbon, Portugal
- 3Physical Therapy and Human Movement Sciences, Feinberg School of Medicine, Northwestern University, Chicago, IL, United States
- 4Biomedical Engineering, McCormick School of Engineering, Northwestern University, Evanston, IL, United States
Cerebrovascular reactivity (CVR) is defined as the ability of vessels to alter their caliber in response to vasoactive factors, by means of dilating or constricting, in order to increase or decrease regional cerebral blood flow (CBF). Importantly, CVR may provide a sensitive biomarker for pathologies where vasculature is compromised. Furthermore, the spatiotemporal dynamics of CVR observed in healthy subjects, reflecting regional differences in cerebral vascular tone and response, may also be important in functional MRI studies based on neurovascular coupling mechanisms. Assessment of CVR is usually based on the use of a vasoactive stimulus combined with a CBF measurement technique. Although transcranial Doppler ultrasound has been frequently used to obtain global flow velocity measurements, MRI techniques are being increasingly employed for obtaining CBF maps. For the vasoactive stimulus, vasodilatory hypercapnia is usually induced through the manipulation of respiratory gases, including the inhalation of increased concentrations of carbon dioxide. However, most of these methods require an additional apparatus and complex setups, which not only may not be well-tolerated by some populations but are also not widely available. For these reasons, strategies based on voluntary breathing fluctuations without the need for external gas challenges have been proposed. These include the task-based methodologies of breath holding and paced deep breathing, as well as a new generation of methods based on spontaneous breathing fluctuations during resting-state. Despite the multitude of alternatives to gas challenges, existing literature lacks definitive conclusions regarding the best practices for the vasoactive modulation and associated analysis protocols. In this work, we perform an extensive review of CVR mapping techniques based on MRI and CO2 variations without gas challenges, focusing on the methodological aspects of the breathing protocols and corresponding data analysis. Finally, we outline a set of practical guidelines based on generally accepted practices and available data, extending previous reports and encouraging the wider application of CVR mapping methodologies in both clinical and academic MRI settings.
Introduction
Cerebrovascular reactivity (CVR) is an intrinsic regulatory mechanism whereby cerebral blood vessels adjust their caliber in response to a vasoactive stimulus, increasing or decreasing the regional cerebral blood flow (CBF). Importantly, CVR is thought to be a sensitive biomarker of the brain’s vascular health in a wide range of conditions and pathologies, including stroke (Krainik et al., 2005; Geranmayeh et al., 2015), glioma (Hsu et al., 2004; Pillai and Zacá, 2011; Zacà et al., 2014; Iranmahboob et al., 2016), small vessel disease (Hund-Georgiadis et al., 2003; Conijn et al., 2012), and obstructive sleep apnea (Prilipko et al., 2014; Buterbaugh et al., 2015; Ponsaing et al., 2018; Wu et al., 2019). Even in the healthy brain, CVR exhibits spatiotemporal patterns possibly reflecting regional variations in cerebral vasculature (Chang et al., 2008; Bright et al., 2009; Pinto et al., 2016), which may impact commonly used hemodynamic measures of brain activity, namely blood oxygen level dependent (BOLD) functional MRI (fMRI) (Ogawa et al., 1990). To take this into account, CVR mapping has been used as a normalizing factor in fMRI studies in order to reduce inter- and intrasubject variability (Handwerker et al., 2007; Thomason et al., 2007; Tsvetanov et al., 2015).
In order to evaluate CVR, a challenge to the vasculature is usually applied while the associated CBF changes are measured. While Transcranial Doppler Ultrasound (TCD) allows only global and indirect CVR evaluation, Positron Emission Tomography (PET) and Single-Photon Emission Computed Tomography (SPECT) enable whole brain CVR mapping; however, these techniques involve the administration of radiotracers, which can limit their ease of use and appropriateness in many studies. By overcoming both limitations, and further allowing improved spatial and temporal resolution, MRI techniques have become increasingly popular for CVR assessment. The BOLD contrast has been particularly useful in this context, as a surrogate of relative CBF changes. Although the BOLD signal results from a complex combination of several physiological parameters, including not only CBF but also cerebral blood volume (CBV) and blood oxygenation, it is nevertheless thought to reflect predominantly CBF changes (Mandell et al., 2008; Chen and Pike, 2010). For truly quantitative measurements of CBF, non-invasive Arterial Spin Labeling (ASL) perfusion imaging can be used (Detre et al., 1992; Williams et al., 1992; Alsop et al., 2015). However, the low signal-to-noise ratio (SNR) inherent to this type of measurement has limited its use. CVR values obtained with MRI methodologies have been shown to correlate with the ones obtained with gold standard techniques for CBF quantification (PET, SPECT) (Heijtel et al., 2014; Fierstra et al., 2018; Hauser et al., 2019).
Regarding the vascular challenge, the most common approach involves the induction of hypercapnia, whereby the arterial blood partial pressure of carbon dioxide (CO2) is increased leading to vasodilation and increased CBF. These vascular tone changes are thought to be mainly induced by CO2-mediated changes in pH levels (Kontos et al., 1977; Brian, 1998). A relatively common method of inducing hypercapnia is the inhalation of air with an increased CO2 partial pressure. For this purpose, a gas mixture with an altered concentration of CO2 (most commonly ∼5%) in relation to normal “room” air (consisting of approximately 21% O2 and 0.04% CO2 with balance nitrogen) is generally used. Different techniques have been developed and improved along the years in order to more precisely control gas concentrations. In particular, some respiratory gas manipulation techniques allow precise targeting of end-tidal carbon dioxide (PETCO2) and/or oxygen (PETO2) concentrations, which provide surrogates for the corresponding arterial gas concentrations (Mark et al., 2010; Spano et al., 2013). Nevertheless, the required experimental setups are quite complex, and for this reason may be associated with low tolerance by some clinical populations or be inappropriate in hospital scanning environments. Moreover, the required setups are quite expensive and may therefore simply not be available in many MRI facilities. Other less expensive gas delivery setups might also be used (Tancredi et al., 2014; Liu et al., 2017b), but these still require additional time for setting up the patient as well as expertise and equipment for manipulating gas levels.
Alternatively, strategies based on breathing tasks performed by the subjects without the need for external gas manipulations have been proposed: breath holding or paced deep breathing can readily induce changes in arterial pressure of blood gases and consequently drive vasodilation and vasoconstriction, respectively. Furthermore, a new generation of methods that are simply based on the spontaneous breathing fluctuations occurring during resting-state has also started to emerge. Both strategies have been shown to be well tolerated by populations generally considered less cooperative (Thomason et al., 2005; Handwerker et al., 2007; Kannurpatti et al., 2010; Raut et al., 2016; Dlamini et al., 2018) and to yield results comparable to those obtained with external gas manipulation techniques (Kastrup et al., 2001; Kannurpatti and Biswal, 2008; Golestani et al., 2016b; Liu et al., 2017a). Because of their simplicity and inexpensive implementation, these strategies might offer a new opportunity for the application of CVR mapping as a useful clinical and research tool.
The aim of this work is to review techniques for assessing CVR during MRI that do not require external gas manipulation. For this purpose, we will focus on the BOLD contrast for the MRI assessment of relative CBF changes, and we will consider the different methods that can be used to elicit a vascular response without the need for external gas manipulations. These include task-based methods, which rely on the voluntary execution of breathing tasks; and resting-state methods, which rely simply on the intrinsic breathing variations during an undirected or “resting” state. In each case, we first describe the experimental design and data acquisition for the respective vasoactive modulation approach, and we then discuss data analysis and modeling methodologies to derive CVR maps. A few reviews have addressed related aspects of CVR mapping: a summary of some of the stimuli commonly used to evaluate CVR, with the exception of resting-state methods (Fierstra et al., 2013; Moreton et al., 2016); technical reviews of CVR mapping, mainly focusing on CO2 inhalation methods (Moreton et al., 2016; Liu et al., 2019); and a systematic review of BH-based CVR mapping (Urback et al., 2017). Nevertheless, our review differs from these previous works by incorporating CVR methods based on spontaneous breathing fluctuations during resting-state and comparing them with task-based methodologies, and in this way giving a more comprehensive overview of the different methodologies for CVR assessment that do not require gas challenges. Additionally, we propose a set of practical guidelines for CVR mapping, addressing both acquisition and analysis methodologies, based on generally accepted practices and available data as described in this review.
Task-Based Methods
This category of methods encompasses the voluntary execution of breathing tasks, designed to induce changes in the arterial pressure of CO2. These include hypercapnia-inducing breath-holding (BH), as well as hypocapnia-inducing paced deep breathing (PDB) tasks (Table 1). In both tasks, several parameters and options need to be considered before implementation. We summarize these in Figure 1, depicting the design of each task. A more detailed description will be provided in the following subsections.

Table 1. Summary of the most common non-invasive methodologies to induce a vascular response, their impact on arterial CO2 levels, cerebral vessels caliber and BOLD signal.
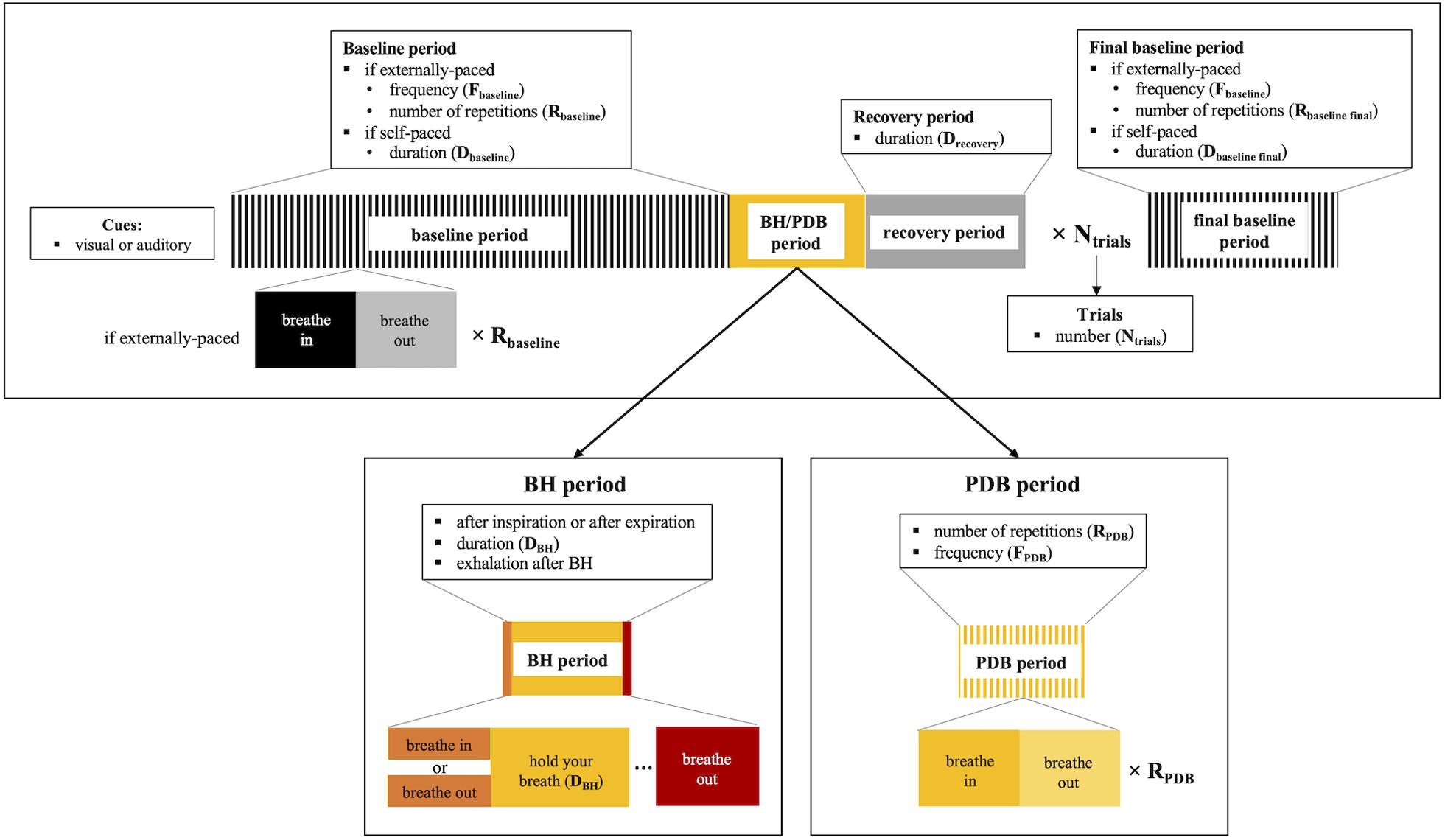
Figure 1. Schematic illustration of the breathing task paradigms (top), including details for breath-hold (BH, bottom left) and paced deep breathing (PDB, bottom right). The parameters and corresponding options that need to be considered for applying the breathing tasks are summarized.
The Breath-Hold Task
The use of the BH task for CVR measurement was first described by Ratnatunga and Adiseshiah (1990), and it has since been the most common voluntary challenge chosen to elicit a cerebrovascular response. This task increases arterial CO2 levels due to subjects holding their breath and ceasing ventilation, ultimately leading to dilation of vasculature and an increase in CBF. The BH task offers a readily accessible and simple method for voluntary breathing modulation being reproducible at 1.5 and 3T (Magon et al., 2009; Lipp et al., 2015; Pinto et al., 2016; Peng et al., 2020).
Despite being used mostly in studies of healthy subjects, their successful application in children and elderly healthy populations indicates that BH tasks can be used to measure CVR even in populations that are generally considered less cooperative (Riecker et al., 2003; Thomason et al., 2005; Handwerker et al., 2007; Kannurpatti et al., 2010; Thomas et al., 2013; Raut et al., 2016). In fact, BH tasks have also been successfully applied in patients with pathologies, such as stroke (Raut et al., 2016), obstructive sleep apnea (Prilipko et al., 2014; Buterbaugh et al., 2015; Ponsaing et al., 2018), schizophrenia (Friedman et al., 2008) and glioma (Hsu et al., 2004; Iranmahboob et al., 2016). Other works have investigated the relationship between BH-CVR measurements and factors such as vascular risk/hypertension (Haight et al., 2015; Tchistiakova et al., 2015), smoking (Friedman et al., 2008), altitude/diving (Yan et al., 2011; Vestergaard and Larsson, 2019) and physical activity (Gonzales et al., 2014; Svaldi et al., 2015, 2017). Furthermore, some studies have reported high correlations between CVR mapping obtained using BH compared with methods based on CO2 inhalation (Kastrup et al., 2001; Biswal et al., 2007; Kannurpatti and Biswal, 2008; Tancredi and Hoge, 2013).
A BH task typically consists of a protocol that follows a standard block design, with alternating periods of breath holding and normal breathing. Nevertheless, there are several parameters and options, shown schematically in Figure 1, that need to be considered when implementing such a task.
BH Period Duration
When selecting the BH period duration, two primary factors must be balanced: the BH must be short enough to be successfully performed by the participant but long enough to induce a robust hypercapnia and CVR response (Kastrup et al., 1999b; Andrade et al., 2006; Magon et al., 2009). The CBF response to CO2 increases with the BH duration, up to certain limit, leading to an increase in the measured imaging signal amplitude and yielding higher sensitivity and SNR as well as more reproducible results (Magon et al., 2009). Nevertheless, shorter BH durations are easier to tolerate, particularly in clinical settings (van Oers et al., 2018), and may also be less susceptible to head motion artifacts. In fact, longer BH periods may be more challenging for the participant, which can result in large “recovery” breaths upon completion of the BH introducing severe head motion confounds (Thomason et al., 2007; Bright et al., 2009). Whether a BH is performed before an inspiration or an expiration highly influences the level of tolerability of the BH duration (discussed below).
Significant hypercapnia states have been reported with BH periods as short as 6 s (Abbott et al., 2005). Most studies adopt a feasible BH duration between 10 and 30 s, although the spatial extent of the significant BOLD response reaches a plateau at a BH length of approximately 20 s (Liu et al., 2002). Several studies have successfully applied BH with a duration of ∼15 s, hence this value might be a good compromise for BH CVR assessment (Leoni et al., 2008; Chang et al., 2009; Pattinson et al., 2009; Bright et al., 2011; Hua et al., 2011; Lipp et al., 2014, 2015; Tchistiakova et al., 2014; Geranmayeh et al., 2015; Urback et al., 2019, 2018).
End-Expiration or End-Inspiration BH
End-inspiration BH yields more complex BOLD responses than end-expiration protocols, commonly exhibiting a triphasic shape, with an initial short positive phase, then a signal decrease followed by a major increase that reaches a maximum, and finally returning slowly back to baseline (Kastrup et al., 1998; Li et al., 1999a; Magon et al., 2009) (Figure 2). End-expiration BH displays a simpler response, without the first signal increase commonly observed in end-inspiration BH strategies. End-expiration BH protocols also tend to achieve faster responses than end-inspiration BH protocols. Nevertheless, if these timing differences are adequately taken into account, both BH protocols have been shown to yield comparable CVR amplitude results (Kastrup et al., 1999b) (Figure 2).
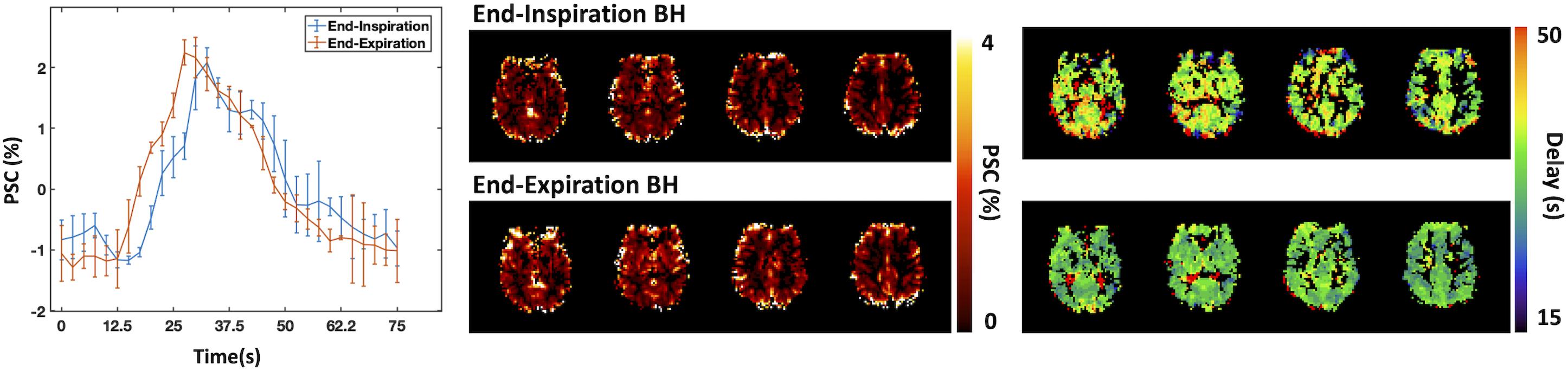
Figure 2. Comparison between end-inspiration (blue) and end-expiration (red) BH protocols: BOLD percent signal change (PSC) time-courses averaged across BH trials and across brain regions displaying significant signal changes. Error bars represent standard deviation across trials. Corresponding CVR maps representing the amplitude (top) and delay (bottom) of the BOLD response (due to the lack of PETCO2 information, the CVR amplitude values are presented in non-normalized units of absolute PSC). Maps were obtained using a Fourier basis modeling approach (Pinto et al., 2016).
End-inspiration BH tasks are naturally easier to perform, allowing for longer BH period durations, and may therefore be more suitable for potentially less cooperative patients (Roberts et al., 2009; Pillai and Mikulis, 2014). Nevertheless, when using an end-inspiration BH task, the amplitude of the BOLD response also depends on the depth of the preparatory inspiration, although visual feedback during task inspiration period has been proposed to tackle this issue (Thomason and Glover, 2008).
End-expiration tasks have been shown to be reproducible (Scouten and Schwarzbauer, 2008) but may induce additional head motion due to the urge to inspire toward the end of the task (Thomason et al., 2007). Nevertheless, chest position during the BH is more similar across trials when following exhalation rather than an arbitrary inhalation, with more consistent field changes due to chest position. Alternatively, instead of employing an explicit end-inspiration or end-expiration BH protocol, some studies instruct subjects to cease breathing wherever they are in their natural breathing cycle (Bright et al., 2009, 2011).
Baseline and Recovery Periods
Regarding the baseline periods between BH, these usually have a long duration in order to allow blood gas levels to return to baseline (Poulin et al., 1996). Another aspect that may be modulated is the breathing rhythm during these periods, which may be spontaneous (self-paced) or paced (externally, computer-paced). The latter may help minimize variability inherent to the spontaneous breathing rates within and between subjects, resulting in a more consistent baseline condition (Scouten and Schwarzbauer, 2008). On the other hand, computer-paced breathing methods require the choice of an appropriate breathing frequency for achieving a normocapnic baseline state, and this is highly dependent on physiological variations in lung function and breathing depth, within and across subjects. Breathing rates from 1/3 Hz to 1/6 Hz have been reported in literature (Handwerker et al., 2007; Chang et al., 2008; Scouten and Schwarzbauer, 2008; Bright and Murphy, 2013; Lipp et al., 2014, 2015; Wu et al., 2015; Cohen and Wang, 2019). However, Bright and Murphy observed mild hypocapnia when using 1/6 Hz compared to self-paced acquisition (Bright and Murphy, 2013). Using a higher breathing rate (1/3.75 Hz), Tancredi and Hoge also observed lower CO2 values in comparison to self-paced baseline values (Tancredi and Hoge, 2013). Residual effects after controlling for breathing rate may be related to the fact that breathing may automatically become deeper when paced, which could also lead to hypocapnia (Bright and Murphy, 2013). Targeting the breathing frequency to match the participant’s spontaneous breathing rate might minimize these hypocapnic effects of computer-paced breathing.
If a paced breathing approach is used for the baseline period, an additional recovery period, consisting of several seconds of spontaneous breathing, is commonly introduced after the BH and exhalation period, before resuming the baseline period (Bright and Murphy, 2013; Cohen and Wang, 2019). This recovery period primarily acts to accommodate faster and deeper breaths, as needed, following completion of the BH, which may minimize participant discomfort or anxiety and increase compliance when returning to the paced breathing pattern.
Number of BH Trials
In principle, the more BH trials are executed within a scan session the more averaging can be done and thus the higher measurement signal-to-noise ratio can be achieved. However, more trials also lead to longer protocol durations, which may increase motion artifacts and patient fatigue or non-compliance. Lipp and colleagues assessed the reproducibility of CVR measurements using different numbers of trials, with the recommendation of at least 3 to guarantee reproducible maps, when a breath-hold duration of 15 s is used in combination with 18 s of paced breathing (Lipp et al., 2015).
The Paced Deep Breathing Task
Despite the BH task being a valuable method to induce hypercapnia and vasodilation non-invasively, techniques to induce hypocapnia and vasoconstriction can also be useful, in particular when further vasodilation is compromised (Zhao et al., 2009; Bright et al., 2011). In that case, hyperventilation might provide a valuable alternative breathing task, as an increase in respiration rate/depth induces hypocapnia. Numerous studies have used this approach for CVR assessment in both healthy and patient populations (Posse et al., 1997; Weckesser et al., 1999; Cohen et al., 2002; Naganawa et al., 2002; Hund-Georgiadis et al., 2003; Krainik et al., 2005; Zhao et al., 2009; Hajjar et al., 2010; Tancredi and Hoge, 2013). However, prolonged hyperventilation periods may cause undesired effects such as light-headedness, dizziness, visual disturbance, numbness and paresthesia, palpitations, tachycardia, shakiness, tension or anxiety, panic attacks, and weakness or exhaustion (Posse et al., 1997). Moreover, hyperventilation tasks are usually associated with high levels of head motion synchronized with the inspiration/expiration cycle.
In order to overcome such limitations, paced breathing tasks have been designed to induce mild and transient hypocapnia. Bright et al. (2009) introduced a PDB paradigm consisting in a controlled and mild increase of respiration rate for brief periods of time. This method was compared with more standard approaches, such as BH or CO2 inhalation, yielding consistent results (Bright et al., 2009; Figure 3). In another study, Sousa et al. showed that a similar PDB protocol yielded CVR measurements with good within- and between-subject reproducibility (Sousa et al., 2014). Additionally, Bright et al. compared PDB and BH tasks during CO2 inhalation, mimicking cases where further basal vasodilation may be limited, and observed that PDB-derived CVR values (but not BH-derived CVR values) were significantly increased during this basal change in vascular tone (Bright et al., 2011). It is worth mentioning that a linear relationship between CBF and PETCO2 is assumed in the interpretation of these results, but this would not hold for extreme deviations of CO2 levels (more details in the section Other Considerations).
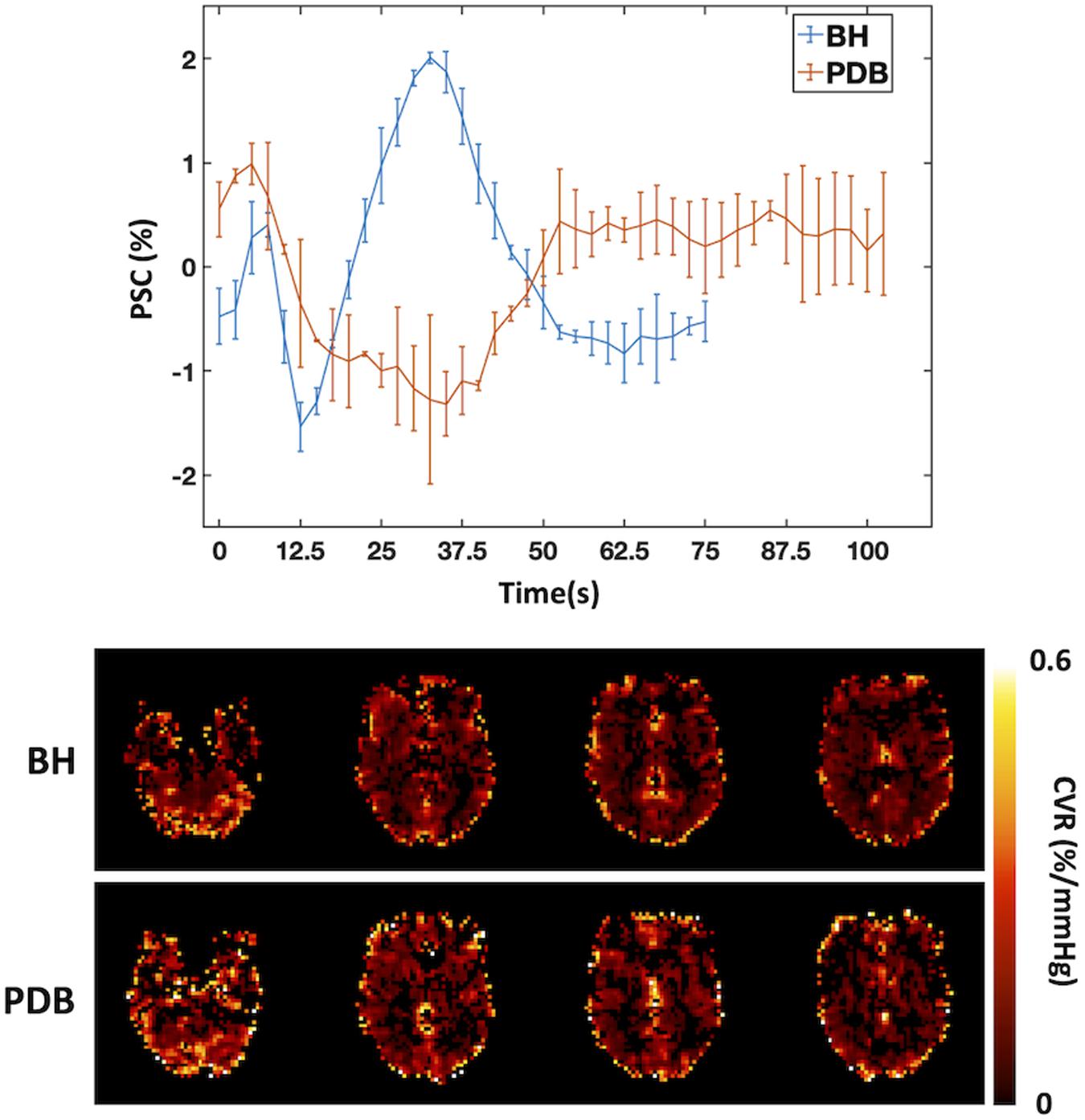
Figure 3. Comparison between BH and PDB protocols: Example of BOLD signal time courses averaged across trials and across regions that display significant signal responses (left, error bars represent standard deviation across trials), and corresponding CVR maps (in normalized units of BOLD percent signal change per mmHg (%/mmHg), right), for one illustrative subject. The BOLD signal changes in response to the different tasks can be seen clearly, with a signal increase/decrease for BH/PDB, respectively. A Fourier basis modeling approach was used to obtain the CVR maps (Pinto et al., 2016).
The PDB protocol typically follows a standard block design, with alternating periods of paced deep breathing and normal breathing. However, some parameters and options (shown schematically in Figure 1) need to be selected before implementing a PDB task.
Frequency, Depth and Duration of PDB
While some studies instruct subjects to hyperventilate without imposing a fixed rate or depth of respiration, others opt to specify this. In some studies, subjects practice the PDB task outside the scanner while PETCO2 levels are monitored: this can be done simply in order to verify their compliance (Posse et al., 1997; Zhao et al., 2009), or also to determine the subject-specific rate that best yields the required hypocapnic effect to be used in the final protocol (Cohen et al., 2002). Respiration rates of 1/4, 1/5 and 1/2.5 Hz have been previously used (Bright et al., 2009; Vogt et al., 2011; Sousa et al., 2014) in blocks of only a few breaths (2 or 3 breaths) (Bright et al., 2009, 2011) or for longer periods (8 breaths) (Sousa et al., 2014). Vogt and colleagues studied the impact of faster (1/2.5 Hz) and deeper (at a different frequency, 1/5 Hz) paced respiration for 20 s periods. As expected, increasing the breathing rate and depth decreased PETCO2 levels, but with faster breathing achieving lower values. Nevertheless, the combination of fast and deep breaths yields the lowest PETCO2 values compared to either fast or deep breathing separately (Vogt et al., 2011).
Baseline and Recovery Period
As in the case of BH tasks, PDB periods are alternated with “normal” breathing periods that act as a reference baseline. The duration of these baseline periods has varied between 60 and 90 s (Bright et al., 2009, 2011; Sousa et al., 2014). Although these studies employed self-paced breathing, Vogt et al. (2011) observed that PETCO2 decreases were greater when using external, computer-paced breathing compared to self-paced breathing (Vogt et al., 2011).
Deep recovery breaths are not typically required after performing PDB, but it may be more comfortable or natural for the participant to take slower or more shallow breaths following an hypocapnic task. It may therefore aid in improving participant comfort and compliance to include a short recovery period prior to the resumption of a paced baseline breathing pattern.
Number of Trials
The number of PDB trials has been highly dependent on the duration of each PDB period. While Sousa et al. used longer PDB periods and only two trials (Sousa et al., 2014), Bright et al. used shorter PDB periods but six trials (Bright et al., 2009). At present, there has not been a systematic assessment of the number of trials required for reliable CVR mapping using PDB.
Additional Practices
Monitoring
Monitoring of task performance through the measurement of complementary physiological variables is strongly recommended, particularly PETCO2 levels. This can be achieved by using a capnograph in combination with a nasal cannula to measure the CO2 levels expired air. CO2 sampling and measurement should ideally be performed as close to the subject’s expired air flow (nostrils) as possible, as reducing the sample tube length diminishes dispersion effects as well as the time delay between the true physiological effect and measured recording (Moreton et al., 2016). However, the logistics of the MRI environment typically require several meters of tubing between the nasal cannula, near the center of the scanner bore, and the capnograph, which must be located in the control room. The sampling delay caused by this physical distance is impacted by the power of the capnograph’s vacuum pump, and a high vacuum strength is recommended. One simple way to determine the effective sampling delay is to record the CO2 trace following a single BH and measure the time between the end of the BH and the first end-tidal CO2 measurement after the BH (Bulte and Wartolowska, 2017).
The PETCO2 levels are extracted by identifying the maximum peaks on the recorded CO2 trace, and the final PETCO2 time course is obtained by correcting for the sampling delay and interpolating to the time of each acquired fMRI volume (Bulte and Wartolowska, 2017). It is worth noting that, during a BH, no information is collected, and therefore the most important PETCO2 measurements in each trial are from the exhalations immediately before and after the BH period. It is important to emphasize that these exhalations are crucial for obtaining the most accurate estimate of the PETCO2 change evoked by the BH task (Bright and Murphy, 2013). It must also be acknowledged that beginning and ending the BH period with exhalations may feel unnatural and be challenging to perform, as typically the participant will feel the need for deep inhalation following the BH. Special instructions and training (see below) are likely needed to achieve these important measurements.
Respiratory information and/or task compliance can also be assessed using a respiratory belt. However, studies comparing respiratory belt measurements with PETCO2 have shown that, although somewhat correlated, PETCO2 better models BOLD signal changes due to breathing tasks (Chang and Glover, 2009; Vogt et al., 2011).
Training
Pre-scan training is highly recommended when using task-based methods in order to ensure that the participant understands the instructions and how to perform the task (Magon et al., 2009; Kannurpatti et al., 2010; Zacà et al., 2014). Additionally, training also helps make the participant more familiar and less anxious about the task itself, improving compliance.
As part of the training, it may be desirable to explain what the participant should do if they feel unable to complete a trial. For example, if the individual needs to stop a BH prematurely, this can be readily tolerated, but it would improve data quality and interpretation to still perform a short exhalation at that time. This has the added benefit of giving participants more autonomy during the experiment, which may also reduce anxiety.
Task Instructions
Task instructions are usually provided using visual cues, although auditory cues can also be used (Riecker et al., 2003; Chang et al., 2008; Kannurpatti and Biswal, 2008; Tancredi and Hoge, 2013; Wu et al., 2019). Visual cues have ranged from simple written instructions displayed on a screen (Bright and Murphy, 2013; Haight et al., 2015; Lipp et al., 2015; Pinto et al., 2016; Liu et al., 2020), to color-coded or symbolic cues (Friedman et al., 2008; Thomason and Glover, 2008; Kannurpatti et al., 2010; Gonzales et al., 2014; Prilipko et al., 2014). In order to control for the potentially strong neuronal activation induced by visual instructions, brightness levels should remain similar across task and baseline periods (Kastrup et al., 1999a,b). An additional fMRI scan displaying the same instructions but without the execution of the breathing task can be performed in order to detect any differences in neuronal activation between task and baseline periods.
Data Analysis
Several strategies have been proposed to analyze fMRI responses to a vasoactive task, with focus on model-driven approaches, including the general linear model (GLM) approach. A box-car function describing the block design of the breathing paradigms may be used to build a model of the BOLD-fMRI response (Kastrup et al., 1999b; Biswal et al., 2007). Some studies have instead used ramp functions, assuming a linear increase in the BOLD signal with time in response to a BH task (Bright and Murphy, 2013). Rather than using the ideal/theoretical task paradigm, more precise models of the BOLD response can, in principle, be built based on the recorded respiratory traces, namely PETCO2 or respiratory belt data (Birn et al., 2008; Murphy et al., 2011; Bright and Murphy, 2013; Lipp et al., 2015). Using recorded respiratory traces to build the model has the advantage of intrinsically accounting for variations in task performance between subjects as well as within subjects across time. In particular, it has been demonstrated that the use of PETCO2-based models can account for irregular task performance, which is particularly relevant in non-compliant participants and thus has potentially great impact on patient studies (Bright and Murphy, 2013). The use of PETCO2 also becomes crucial for the normalization of CVR units (more details in the Other Considerations section).
In all cases, the temporal dynamics of the vascular response to the breathing task needs to be taken into account. This can be achieved by assuming a linear time-invariant system and convolving the task boxcar function, or the measured respiratory trace, with an appropriate impulse response function. Some studies have used the canonic hemodynamic response function (HRF) commonly used to describe the BOLD response to an impulse of neuronal activity; in this case, adding the temporal derivative to the model is critical to allow for the longer delays observed in the case of the respiratory response (Murphy et al., 2011; Jahanian et al., 2017). Birn and colleagues performed BOLD-fMRI measurements of single deep breaths and derived a new impulse response function to model the BOLD response to variations in the respiration volume per time (RVT), extracted from respiratory belt measurements – the Respiration Response Function (RRF). Using the RRF has been shown to provide a significantly better model of the BOLD changes induced by BH and PDB tasks than using the canonic HRF (Birn et al., 2008). In a later study, Vogt et al. derived an impulse response function of the BOLD signal to PETCO2 variations during paced hyperventilation (Vogt et al., 2011).
One aspect that most of these modeling strategies have in common is the assumption that a single, fixed time course can explain the BOLD signal across the brain. However, it has been suggested that the response to vasoactive stimuli may exhibit different temporal dynamics in different brain regions, not only in patients within cerebrovascular disease but also in healthy subjects (Chang et al., 2008; Magon et al., 2009; Geranmayeh et al., 2015; Pinto et al., 2016). Some studies have attempted to overcome this limitation by incorporating delay information into the analysis (Chang et al., 2008; Magon et al., 2009; Geranmayeh et al., 2015). The most common approach to obtain this information is by determining the optimal time lag within a certain time interval using cross-correlation between the voxel’s BOLD signal and the model (Chang et al., 2008). This optimization step can be performed voxelwise, or on a regional basis by considering a number of regions of interest (ROI) across the brain; this latter option may be advantageous in terms of SNR. Using the global BOLD timeseries as the reference has also been used to obtain an approximate estimate of the time delay of the BOLD response – but this does not take regional variations into account (Geranmayeh et al., 2015; van Niftrik et al., 2016). Other approaches that aim to estimate the voxelwise response time delay, include methods such as Hilbert transform (Raut et al., 2016), recursive tracking approaches such as the Regressor Interpolation at Progressive Time Delays method (RIPTiDe, RapidTide) (more details in the Resting-State Methods subsection) (Tong et al., 2011; Donahue et al., 2016; Champagne et al., 2019), iterative GLM fitting using shifted regressors (Geranmayeh et al., 2015; Cohen and Wang, 2019; Moia et al., 2020a), or Fourier basis modeling (Murphy et al., 2011; Lipp et al., 2015; Pinto et al., 2016; van Niftrik et al., 2016). The latter exploits the essentially biphasic shape of the BOLD response to alternating periods of task and baseline (assuming similar durations of each period), resulting in approximately sinusoidal signal variations at the paradigm frequency. More precise modeling of the shape of the response can be achieved by considering a Fourier series with a number of harmonics. Moreover, if a linear combination of a sine and cosine is used, then a phase delay can be estimated as well as the response amplitude. It has been reported that a sine-cosine pair at the task frequency and its two first harmonics provides a suitable model for the BOLD response to an end-inspiration BH task, yielding reproducible estimates of CVR amplitude as well as its time delay (Pinto et al., 2016).
The Fourier series approach has been shown to yield similar results to PETCO2-based modeling (Murphy et al., 2011; Lipp et al., 2015). This approach was further investigated by van Niftrik and colleagues in patients with unilateral hemispheric impaired perfusion (van Niftrik et al., 2016). Two sine waves with different frequencies were used in order to accommodate the fact that the BH and baseline periods had considerably different durations. Using the Fourier series approach to account for voxelwise optimal delay yielded significantly higher CVR values and better differentiation between affected and unaffected brain tissues (van Niftrik et al., 2016). Using iterative modeling of shifted regressors to account for voxelwise delay showed similar improvements in healthy controls (Moia et al., 2020a). Characterizing voxelwise delay information is clearly important for improving the accuracy of CVR measurements and may also supply complementary insight into the health or pathology of the local CVR response.
Resting-State Methods
The last years have seen an increasing interest in the study of the brain’s intrinsic functional connectivity, based on synchronous fluctuations in the blood-oxygen level dependent (BOLD) signal across different brain regions (Biswal et al., 1995). The hemodynamically driven changes in tissue and blood oxygenation underlying the BOLD signal are, however, caused by a combination of neuronal activity as well as non-neuronal mechanisms, including respiration and cardiac sources. These fluctuations of non-neuronal origin are usually referred to as physiological noise in the context of conventional resting-state fMRI (rs-fMRI) studies of brain activity, and they are often modelled and eliminated as they are of no interest in those cases (Greicius et al., 2003; Birn, 2012; Pinto et al., 2017). However, this so-called physiological noise also contains information about cerebral hemodynamics that may be of interest, including CVR mechanisms.
In one approach to analyze rs-fMRI data, metrics are computed to quantify the amplitude of low-frequency oscillations, within the range of approximately 0.01 to 0.1 Hz (Biswal et al., 1995). Although spontaneous fluctuations in neuronal activity are thought to occur within this frequency range, non-neuronal physiological processes also contribute (Murphy et al., 2013; Bright and Murphy, 2015; Caballero-Gaudes and Reynolds, 2017; Tong et al., 2019a; Whittaker et al., 2019). In addition, it is well known that higher frequency fluctuations associated with the respiratory and cardiac cycles are aliased into the low-frequency range, due to the typically low temporal resolution of fMRI data, playing a significant role in spontaneous BOLD fluctuations. Furthermore, cardiac pulsations and the breathing cycle can lead to bulk motion of certain brain regions (Harvey et al., 2008) and spin history effects in the fMRI timeseries (Friston et al., 1996), while breathing-related chest movements can induce B0 field fluctuations that also influence the BOLD signal (Van de Moortele et al., 2002).
Some of the physiological fluctuations occurring within the low-frequency band are also thought to be governed by the autonomic nervous system. One source of such fluctuations, the variability of heart rate, is commonly used as a marker of autonomic nervous system activity (Chang et al., 2013). It has a higher frequency component thought to be related to parasympathetic modulation (0.15 - 0.4 Hz), and a lower frequency component (0.05 – 0.15 Hz) that appears to occur in synchrony with arterial blood pressure oscillations, also known as Mayer waves (∼ 0.1 Hz) (Julien, 2006; Draghici and Taylor, 2016). Another source of BOLD fluctuations in the low-frequency range is vasomotion caused by contraction of the smooth muscle of the arterioles, which is thought to be independent of cardiac and respiratory cycles (Murphy et al., 2013). Low- and very low-frequency BOLD fluctuations (0.023–0.73 Hz and 0.001–0.023 Hz, respectively) have also been associated with CSF pulsations (e.g., glymphatic system) (Mäkiranta et al., 2004; Kiviniemi et al., 2016). More recently, correlations between gastric oscillations (∼ 0.05 Hz) and low-frequency BOLD fluctuations have also been reported (Rebollo et al., 2018).
Pertinent to this review, natural fluctuations in PETCO2 levels also evoke low-frequency BOLD oscillations, providing more specific access to CVR. In 1997, Biswal et al. showed that the amplitude of low-frequency BOLD fluctuations was sensitive to hypercapnia (Biswal et al., 1997). In 2004, Wise et al. further demonstrated that low-frequency BOLD fluctuations across the human brain are temporally correlated with spontaneous PETCO2 fluctuations (Wise et al., 2004). Additional investigation confirmed that low-frequency BOLD fluctuations are also correlated with BH hypercapnic responses (Biswal et al., 2007; Kannurpatti and Biswal, 2008), in both younger and older populations (Kannurpatti et al., 2011).
Taking these physiological mechanisms into account, a new set of methods relying on the spontaneous variations of breathing and PETCO2 levels have been successfully used to assess CVR based on resting-state BOLD-fMRI. These methods have the advantage of not requiring any task to induce a vasoactive response and therefore being less demanding in terms of the experimental setup and more easily implemented, and also less dependent on the cooperation of the participant. This might be more suitable for CVR mapping in less cooperative populations, such as patients with language–comprehension problems or with visual/auditory impairment. Nevertheless, a limitation of this approach is that, if the subject’s spontaneous breathing pattern yields minimal fluctuations in their PETCO2 level, there might not be enough signal variation for reliable CVR assessment. In fact, de Vis and colleagues showed that a hypercapnic stimulus of at least 2 mmHg above baseline PETCO2 is necessary in order to evaluate any hemodynamic impairment (De Vis et al., 2018). Another challenge is isolating low-frequency BOLD fluctuations arising from CVR mechanisms versus other physiologic noise sources or neural processes described above.
Several metrics have been proposed to derive CVR information from rs-fMRI data, and they can be subdivided in two main categories: metrics based on signal variation or signal regression.
Signal Variation
The resting-state fluctuation amplitude (RSFA) is a relatively simple metric commonly used in rs-fMRI studies, being computed as the temporal standard deviation of the BOLD time series (Kannurpatti and Biswal, 2008). Several studies comparing RSFA with CO2 inhalation and BH tasks for CVR estimation have reported similar results (Kannurpatti and Biswal, 2008; Kannurpatti et al., 2014; Wang et al., 2016), while other studies observed a low agreement between measures (Lipp et al., 2015). Nevertheless, RSFA has been shown to have high spatial repeatability (Lipp et al., 2015) and to be an important scaling factor in the analysis of the effects of age on task-fMRI (Tsvetanov et al., 2015).
Several other metrics based on modifications of the RSFA have been proposed as potentially more specific alternatives for CVR measurement. Makedonov et al. developed a physiological fluctuations (PF) metric, in which the temporal variance in signal attributed to thermal noise is subtracted from the total temporal variance of the BOLD signal at a particular voxel (Makedonov et al., 2013, 2016). Jahanian et al. proposed a coefficient of variation (CV) metric, in which the signal temporal standard deviation is normalized by the mean signal intensity (Jahanian et al., 2014, 2017). Jahanian et al. demonstrated that CV can be used to differentiate young healthy volunteers from hypertensive elderly subjects with chronic kidney disease. These CV differences mainly arose from very low-frequency components of the BOLD signal (< 0.025 Hz), and they became more significant after removal of physiological motion effects using the retrospective image-based correction (RETROICOR) method (Jahanian et al., 2014).
When comparing CV and BH-CVR, a strong linear correlation was observed in older adults (Jahanian et al., 2017). In contrast, moderate correlations were observed between CV and strategies based on PETCO2 signal regression (more details in the following subsection), although CV was best at representing whole-brain CVR variations across subjects (Golestani et al., 2016b). Liu et al. compared different physiological modulators of fMRI signal, obtaining a marginal correlation between CV and CVR using CO2 inhalation (Liu et al., 2013). The authors attributed this weak connection to CV being affected by factors such as bulk motion, spontaneous neural activity and breathing fluctuations (Liu et al., 2013).
Considering that some physiological fluctuations can be described within a well-defined frequency range, some authors have restricted their analysis to a specific low-frequency band. Usually, a frequency band of the 0.01 – 0.1 Hz or 0.01 – 0.08 Hz is chosen in order to target the components of interest while also removing high-frequency components possibly mostly contaminated by noise. The amplitude of low-frequency fluctuations (ALFF) metric is computed as the square root of the power spectrum across a specific low-frequency band of the preprocessed rs-fMRI BOLD signal (Zang et al., 2007). This metric has been used to rescale the amplitude of task-related fMRI in order to account for vascular differences (Kazan et al., 2016). More recently, the ALFF metric has been applied in patients with leukoaraiosis, yielding both decreases and increases in specific brain regions (Cheng et al., 2017). Another work on leukoaraiosis has shown that altered ALFF is positively correlated with executive function (Wang et al., 2019). Still, the low-frequency band is quite broad and more specific bands within this range may reflect different physiologic contributions. Zuo et al. (2010) demonstrated differences in the spatial distributions of ALFF in bands slow-2 (0.25–0.20 Hz), slow-3 (0.20–0.07 Hz), slow-4 (0.07–0.03 Hz) and slow-5 (0.03– 0.01 Hz). Although it remains challenging to discern what components of the low-frequency signals are specific to CVR mechanisms, correlation analysis with PETCO2 levels has been shown to be highest in the frequency band of 0.02–0.04 Hz (Liu et al., 2017a) (more details in the following subsection).
A related metric is the fractional ALFF (fALFF), whereby the power within the low-frequency range is normalized by the power across the whole spectrum (Zou et al., 2008). Both ALFF and fALFF have been shown to yield moderate to high test-retest reliability in gray matter (GM) regions, although ALFF is more reliable than fALFF (Zuo et al., 2010). Nevertheless, it has been demonstrated that ALFF is more prone to non-CVR physiological noise sources in comparison to fALFF, in particular near the ventricles and large blood vessels (Zou et al., 2008; Zuo et al., 2010). In summary, there is a tradeoff between reliability/specificity between the two metrics, and therefore some authors recommend reporting both ALFF and fALFF (Zuo et al., 2010; Figure 4).
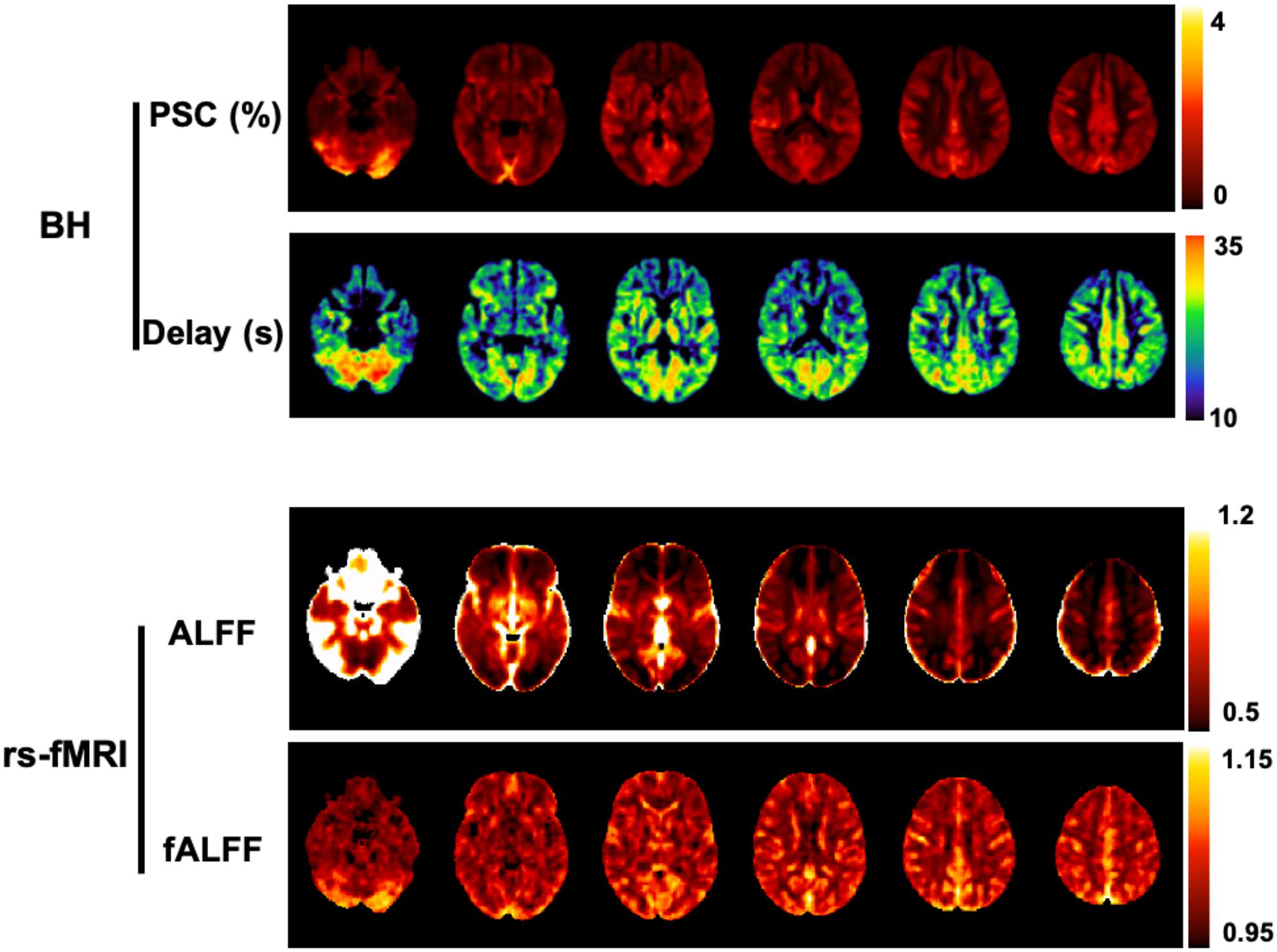
Figure 4. Comparison between BH and rs-fMRI protocols: (Top) CVR amplitude and delay maps obtained using BH BOLD-fMRI and the Fourier basis modeling approach (Pinto et al., 2016). (Bottom) Maps of ALFF and fALFF computed in the 0.01 - 0.023 Hz frequency band. All maps are group averages across 12 healthy individuals. For comparison purposes with the rs-fMRI metrics, the CVR amplitude maps are represented in non-normalized units of absolute BOLD percent signal change (PSC).
Recently, De Vis and colleagues compared ALFF and fALFF metrics of rs-fMRI with CVR fMRI measurements during hypercapnia and hyperoxia gas challenges. A moderate relationship was found between hypercapnia and resting-state CVR measures in healthy individuals, but this relationship was decreased in a patient group. While hypercapnia fMRI measures led to large effect sizes when detecting hemodynamic impairment, rs-fMRI ALFF/fALFF metrics only showed moderate effect sizes. Nevertheless, in both hypercapnia and resting-state CVR measures, resting CBV (using hyperoxia BOLD signal as a proxy) significantly explained a large portion of BOLD signal variance across the brain (hypercapnia: 53/37%; ALFF: 31/23%; fALFF: 29/14%, in healthy controls/patients, respectively) (De Vis et al., 2018). Similar to ALFF, fALFF maps have also been employed as a voxelwise scaling factor of task-based fMRI analysis, significantly reducing inter-subject variability (Kalcher et al., 2013).
It should be noted that all signal variation metrics presented in this section are merely qualitative since they are not normalized against a common scale, usually the amplitude of PETCO2 fluctuations. Nevertheless, they can be sensitive to spatial differences since they are computed for each voxel separately. A summary of the most common rs-fMRI signal variation CVR metrics and their computation is depicted in Table 2.
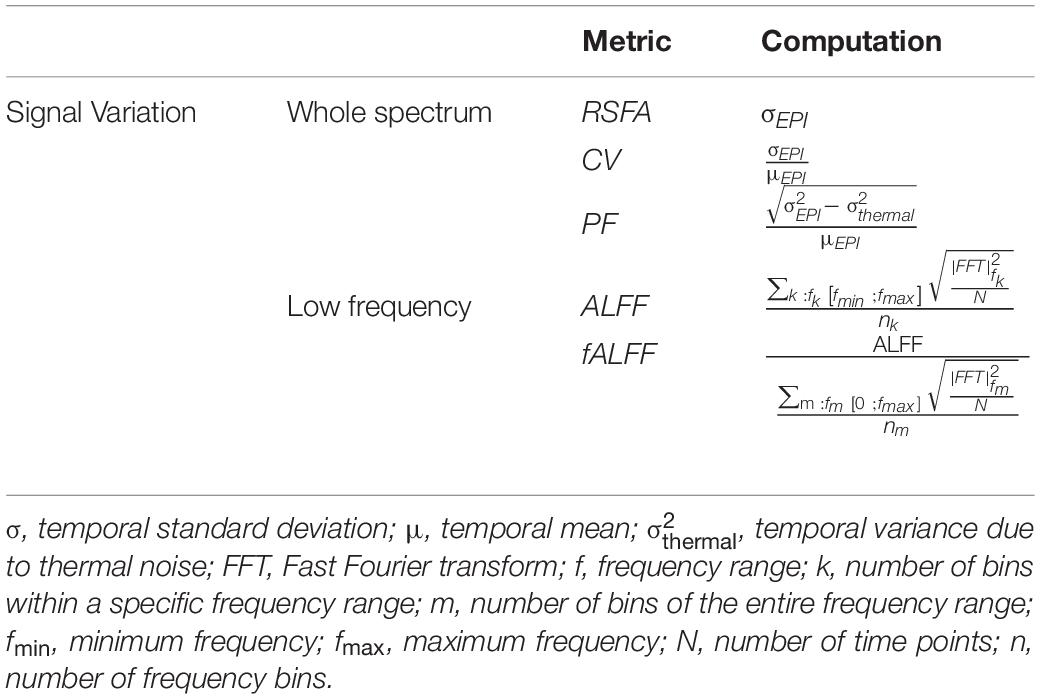
Table 2. Summary of the most common rs-fMRI CVR methodologies based on BOLD signal variation metrics.
Signal Regression
Given that PETCO2 is a well-established surrogate of arterial CO2, regression of BOLD signal by the spontaneous variations in this metric can also be used in order to estimate CVR in rs-fMRI (Wise et al., 2004). Another possible surrogate of arterial CO2 is the respiration volume per time unit, RVT (Birn et al., 2008; Chang and Glover, 2009). In 2009, Chang et al., 2009 verified that PETCO2 and RVT account for similar patterns of temporal and spatial variations of resting BOLD signal. However, the authors also observed differences between the two metrics, and indeed the BOLD signal variance explained was higher when using a combination of the two metrics compared to using only one of them (Chang and Glover, 2009). Several other studies have reported the use of PETCO2 as a regressor to map CVR based on rs-fMRI in healthy volunteers (Lipp et al., 2015; Golestani et al., 2016b) as well as in patients with cerebrovascular diseases (Liu et al., 2017a). Nevertheless, the rs-fMRI response to intrinsic PETCO2 fluctuations in healthy subjects may not be a reproducible metric, exhibiting lower intra-class correlation values than BH-CVR metrics (Lipp et al., 2015). In particular, the rs-fMRI response to PETCO2 fluctuations has been shown to vary with eyes-open and eyes-closed states (Peng et al., 2013). Golestani and colleagues investigated the impact of CVR mapping using PETCO2 in rs-fMRI after removing the cardiac and respiration contributions (Golestani et al., 2015, 2016b). They then estimated the relationship between rs-fMRI BOLD and PETCO2 fluctuations through parametric deconvolution, yielding a PETCO2-based response function (HRFCO2) (Golestani et al., 2015, 2016b).
In 2015, Liu et al. (2015) hypothesized that the average rs-fMRI BOLD signal across the whole brain reflects spontaneous arterial CO2 fluctuations, and employed global-signal regression (GSR) to derive CVR. This was validated in comparison with CO2-inhalation CVR maps. Furthermore, the authors also applied the proposed method to Moyamoya disease patients and found a reduced CVR in the diseased territories, with rs-fMRI CVR maps being comparable to those derived from CO2-inhalation (Liu et al., 2015). The same group also observed that rs-fMRI GSR methods provide significantly better CVR maps (in terms of Z-scores) compared to PETCO2 regression methods, and they speculate that this is due to the low sampling frequency of PETCO2 recordings (limited by breathing rate) compared to the BOLD signal (Liu et al., 2017a). More recently, this rs-fMRI GSR strategy was also applied in a group of stroke patients, confirming its feasibility (Taneja et al., 2019). Golestani and colleagues observed a good within-subject agreement between CVR values obtained using rs-fMRI GSR and conventional CO2 manipulation. However, rs-fMRI GSR was much less reproducible than methods based on rs-fMRI metrics, namely RSFA and ALFF. The authors state that one possible reason for this is the close relationship between GSR CVR estimates and frame-wise head motion (Golestani et al., 2016b). This confound may improve when isolating low-frequency components of the global signal. When different frequency bands (between 0 and 0.2 Hz) were taken into account, correlation between BOLD signal and PETCO2 was highest in the frequency band 0.02–0.04 Hz, yielding highly reproducible CVR maps that were spatially correlated with those obtained using the conventional CO2-CVR method (Liu et al., 2017a).
Regression using rs-fMRI averaged across a specific tissue mask has also been described by Jahanian et al. in a cohort of older adults (Jahanian et al., 2017), which may also facilitate the specificity of rs-fMRI signal regression to CVR mechanisms. In particular, when using the CSF signal, assuming it predominantly reflects fluctuations of purely non-neuronal origin, significant correlations with BH-derived CVR were observed, although these were lower than the CV method described earlier (Jahanian et al., 2017).
The temporal aspect of the low-frequency fluctuations of rs-fMRI has been also investigated with approaches primarily based on time-lag correlation, including simple seed-based lag mapping (Amemiya et al., 2014; Tong et al., 2017) as well as recursive tracking methods such as RIPTiDe (or its accelerated implementation, RapidTiDe) (Frederick et al., 2012; Erdoğan et al., 2016). The former generates a lag map based on the rs-fMRI time shift that yields the maximum correlation coefficients in each voxel relative to a predefined reference time course. In contrast, the latter approach takes into account the rs-fMRI data itself, with the reference time course being updated recursively, to better accommodate timing and shape differences. This is performed by shifting voxelwise the initial time course and applying principal component analysis or a weighted average to obtain an updated single time course that accounts for the highest shared variance. This approach might account for physiological differences in breathing responses and other possible factors that are difficult to know a priori. The use of optimized temporal delays at each voxel (using GSR) has been shown to increase the amount of BOLD signal variance explained relative to a GSR approach that does not perform this optimization (Erdoğan et al., 2016). Furthermore, the commonly seen negative correlations in GSR are minimized when using an optimized delay approach (Erdoğan et al., 2016). Although estimation of temporal delays may be less robust in rs-fMRI protocols compared to task-based CVR (Bright et al., 2017), rs-fMRI lag maps have revealed close correlation with SPECT-CVR maps, using acetazolamide as the vasoactive stimulus (Nishida et al., 2019). Some studies support the assumption that the delays of the low-frequency oscillations of rs-fMRI propagate in a way similar to cerebral blood circulation within the brain, reflecting vascular structure, both in healthy subjects (Tong et al., 2017, 2019b) as well as in a series of pathological conditions (Lv et al., 2013; Amemiya et al., 2014; Christen et al., 2015; Ni et al., 2017).
Other Considerations
PETCO2 as Surrogate of PaCO2
Estimation of PaCO2 during CVR assessment is a critical requirement for quantitative interpretation of results. However, direct measurement of PaCO2 requires blood sampling, making it highly invasive; for that reason, PETCO2 is generally used instead as a non-invasive surrogate. PETCO2 has been shown to provide a robust proxy of arterial CO2 partial pressure when using gas challenges that target PETCO2 levels in combination with respiratory rates greater than 12 breaths per minute (Ito et al., 2008). This assumption, however, might be compromised and not applicable in patients with diminished cardiopulmonary function, during exercise, or when using in combination with other breathing protocols (Peebles et al., 2007; Ito et al., 2008). Nevertheless, even if the absolute value of PETCO2 is not equivalent to the arterial CO2 content, its relative variation still accurately reflects the CO2 changes elicited by the stimulus (Mcswain et al., 2010). For these reasons, in practice, the use of PETCO2 as a surrogate of PaCO2 is a pragmatic choice for breathing modulation monitoring and analysis (Bright et al., 2019).
Non-linear PETCO2 - CVR Relationship
The impact of baseline PETCO2 has been shown to influence CVR assessment (Halani et al., 2015; Golestani et al., 2016a; van Niftrik et al., 2018). In stroke patients, the compensatory baseline condition where vessels are dilated may explain the enhanced vasoconstrictive reactivity to hypocapnia in contrast to no significant changes in hypercapnic vasodilatory reactivity (Bright et al., 2011). Recently, it was demonstrated that CVR is significantly different when measured relative to a predefined “normocapnic” baseline (40 mmHg) compared to the subjects’ specific resting PETCO2 level (van Niftrik et al., 2018). Additionally, Hou and colleagues showed that subjects with higher baseline PETCO2 had lower CVR (Hou et al., 2020). Given all these observations, it is recommended that baseline PETCO2 levels should be reported in combination with CVR changes (Tancredi and Hoge, 2013; van Niftrik et al., 2018; Hou et al., 2020).
One possible explanation for why baseline PETCO2 level influences CVR measurement is the non-linear relationship between CBF (and the BOLD signal) and PETCO2. Although a linear relationship is usually assumed for CVR mapping, by applying progressive levels of hypo-/hypercapnia, a sigmoidal relationship provides a better model of the BOLD response (Bhogal et al., 2014; Sobczyk et al., 2014). Bhogal et al. observed a distinct BOLD signal plateau for high PETCO2 levels (assessed through gas challenges) (Bhogal et al., 2014), whereas Tancredi and colleagues emphasized that the CBF non-linear portion is critical mainly in the lower range of PETCO2 values (obtained by hyperventilation and ASL imaging) (Tancredi and Hoge, 2013). Combined, these observations indicate that baseline PETCO2 influences where on this non-linear, sigmoidal dose-response curve an individual is at rest and will thus influence the vascular response to any modulation in PETCO2 levels. It may be critically important to understand the role of non-linear CVR responses, particularly when comparing patient cohorts with different baseline physiology.
There is also substantial spatial heterogeneity of the non-linear BOLD response to PETCO2 (assessed through gas challenges) (Bhogal et al., 2014, 2015, 2016; Duffin et al., 2015; Fisher et al., 2017), emphasizing the need of studying CVR in a region-based or voxelwise manner. This heterogeneity is partly explained by differences in tissue types, anatomical regions and vascular territories (Bhogal et al., 2015).
PETCO2 Normalization
Cerebrovascular reactivity can be expressed as percentage change in the fMRI signal normalized by the amplitude of concurrent PETCO2 variations. This normalization can minimize variability due to breathing task compliance differences across sessions and subjects (Bright and Murphy, 2013). When comparing BH-CVR with CO2-inhalation CVR, Kastrup and colleagues observed higher correlation when normalizing data with individual PETCO2 changes (Kastrup et al., 2001). However, Goode et al. found contradictory results when performing PETCO2 normalization on BOLD responses in a hypercapnic challenge, obtaining worse reproducibility and higher between-subject variability (Goode et al., 2009). Tancredi and colleagues also observed a reduction in CVR variability across different protocols (hypo- and hypercapnic, using ASL imaging) when CVR was expressed in terms of the percent change in CBF compared with normalized change. This was explained by the fact that normalized CVR values depend strongly on the range of PETCO2 levels considered, being greatly influenced by the non-linearity of the fMRI response to CO2 described earlier (Tancredi and Hoge, 2013).
In some cases, the PETCO2 normalization step is not mandatory and may not be useful; this is the case for example when using a representative ROI (e.g., lesion) time-series to compare with its contralateral location, in order to assess regional CVR alterations within the same individual. Additionally, CVR normalized in relation to whole brain or reference tissue values may be a more sensitive biomarker than absolute CVR in clinical applications, as it minimizes inter-subject variations (Yezhuvath et al., 2009). In general, methods based on resting-state signal variation are also not normalized against PETCO2 fluctuations. Still, when comparing CVR across individuals or cohorts, or tracking longitudinal changes in CVR, it is generally accepted that normalized CVR values are more appropriate (Liu et al., 2019).
Role of O2
Within normal physiological values, the role of arterial O2 pressure in CBF regulation seems to be negligible (Ainslie and Duffin, 2009; Moreton et al., 2016), with reactivity to CO2 being approximately 60 times larger than to O2 (Prisman et al., 2008). Mark and colleagues observed no change in global CBF, as assessed by ASL, during an isocapnic hyperoxia stimulus (Mark et al., 2011). Breathing tasks, as described in this review, might also change arterial and venous O2 saturation levels and impact the BOLD signal (Bulte et al., 2009). Tancredi and colleagues also verified that, when using BH, the level of PETO2 decreased causing mild hypoxia; this could contribute a slight vasodilatory input, which would tend to exaggerate CVR measures. While the latter effect could explain the observed tendency of BH-CVR values to be higher than those for CO2 inspiration, the difference was fairly small and not statistically significant (Tancredi and Hoge, 2013).
ASL
As previously described, the BOLD signal results from a complex combination of several physiological parameters, including CBF, CBV and blood oxygenation, although it is thought to reflect predominantly CBF changes. For direct, quantitative measures of CBF, the ASL technique can be used (Alsop et al., 2015; Pinto et al., 2020). Nevertheless, in CVR mapping, the main limitations of ASL are the intrinsically low signal-to-noise ratio (SNR) and poor temporal resolution, making this approach problematic when combined with short BH and PDB durations and associated non-steady-state vasoactive responses. Furthermore, the hypo- and hypercapnic states attained for CVR assessment might elicit changes in blood flow velocity, and hence labeling efficiency and bolus arrival time, as well as changes in arterial blood T1, and in this way compromise ASL perfusion quantification and CVR results (Aslan et al., 2010; Tancredi et al., 2012). To date, the number of studies employing ASL imaging in combination with breathing tasks for CVR evaluation is relatively low, particularly when compared to BOLD imaging (Kastrup et al., 1999b, 2001; Li et al., 1999a,b; Lu et al., 2003; MacIntosh et al., 2003; Leoni et al., 2012; Tancredi and Hoge, 2013; Prilipko et al., 2014; Haight et al., 2015; Cohen and Wang, 2019). Faster acquisition strategies with simultaneous ASL and BOLD imaging have shown promising results in CVR assessment based on breathing tasks (Cohen and Wang, 2019) and may increase the utility of ASL CVR mapping methods without gas inhalation in future research.
Practical Guidelines
Despite the multitude of strategies and options that have been proposed and reported, as described in this review, the CVR literature is still insufficient to derive definitive conclusions regarding the best practices. Nevertheless, here we provide some practical guidelines based on available data on how to get started with CVR mapping without the use of gas challenges. We highlight the need for guidance depending on the type of population under study and equipment available as well as the expertise of the researchers.
First, the literature strongly indicates that a voluntary breathing manipulation (task-based) is able to produce larger CBF changes and hence more robust CVR measures than spontaneous breathing fluctuations (resting-state). To date, the most commonly used and most well studied breathing task is breath holding. For this reason, the choice of a BH task is recommended at this point, unless this is not feasible or otherwise appropriate for the population under study (see below). Several protocols have been proposed and tested, and we recommend the following parameters (illustrated in Figure 5): end-expiration BH; BH duration of 15s; exhalation immediately after the BH period to allow measuring PETCO2 at the end of the BH; recovery period with self-paced breathing; baseline period of externally paced breathing with a frequency that matches the participant’s breathing rate and a duration that allows blood gas levels to return to baseline; and a protocol with at least 3 BH trials.
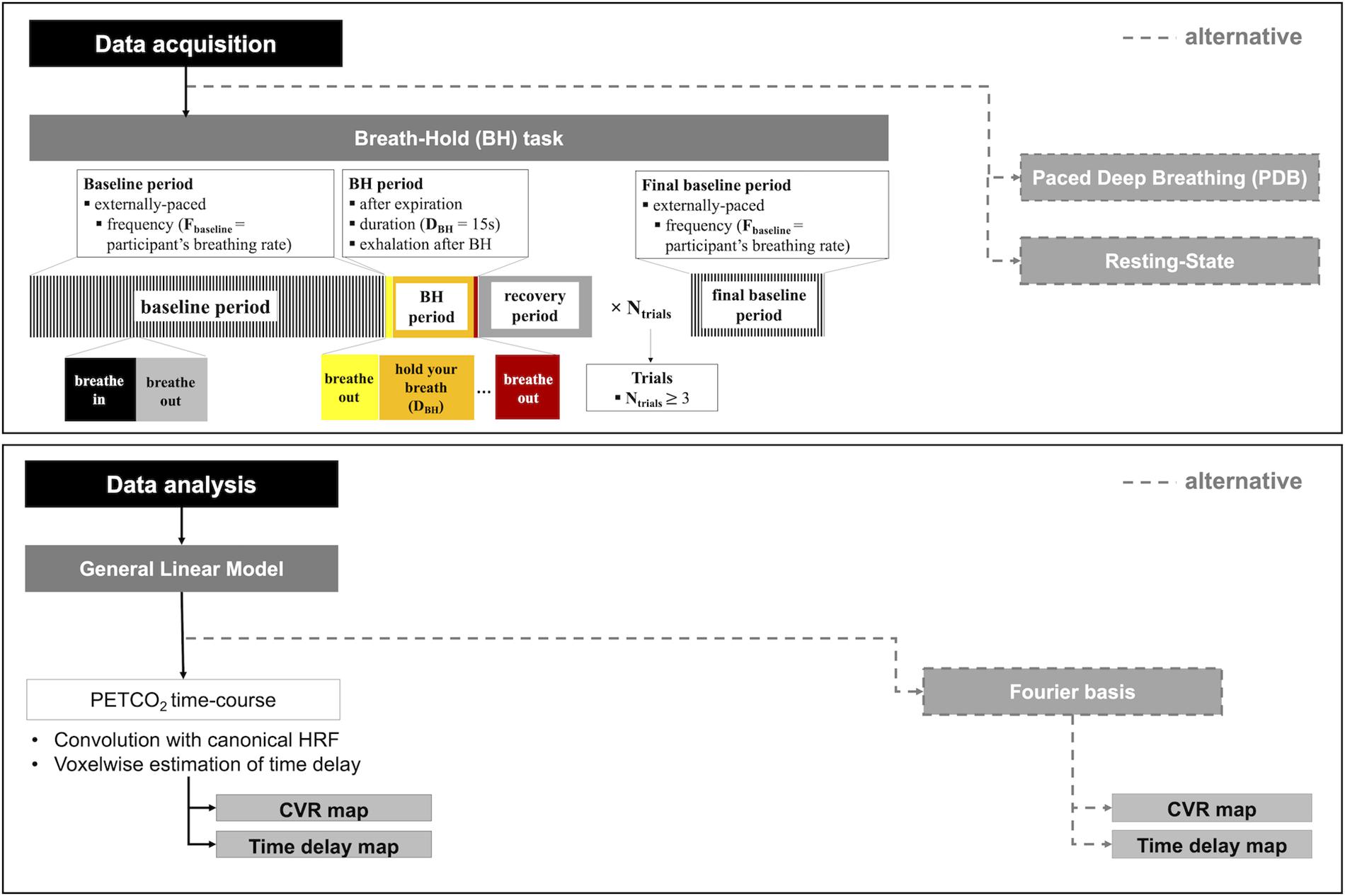
Figure 5. CVR mapping pipeline: recommended guidelines for data acquisition (top), including the BH protocol with corresponding parameters, and data analysis (bottom), including the GLM analysis, as well as recommended alternative approaches in each case.
Second, it is generally accepted that CVR metrics should be reported as a BOLD or CBF change normalized by the corresponding variation in PETCO2. We therefore recommend the recording of PETCO2 data throughout the MRI scan session: besides being crucial for this normalization, it also allows monitoring participant’s task compliance, assessment of baseline PETCO2 and estimation of both the PETCO2 sampling delay (if a brief BH followed by exhalation is performed) and regional hemodynamic delay. Furthermore, we also recommend the execution, prior to the MRI acquisition, of a practice run with PETCO2 recording. This will allow the assessment and optimization of the participant’s compliance as well as the retrieval of their natural breathing rate (to be incorporated in the BH protocol).
For data analysis, we recommend using the well-established GLM framework for fMRI. In fact, this is a relatively simple method and it can be easily implemented using widely available and finely tuned software tools (such as FSL1, SPM2, or AFNI3).
If PETCO2 data are collected, this should be pre-processed by end-tidal point extraction, interpolation, and sampling delay correction. For subsequent GLM analysis of the fMRI data, the resulting time course should then be convolved with a response function. Several studies have shown that the canonical HRF commonly used in fMRI studies is an appropriate choice and this can therefore be used for simplicity. Since variable temporal delays have been observed across the brain for the BOLD response to a BH, we recommend voxelwise estimation of this delay. This can be performed using the PETCO2 data, by applying the RapidTide method or the iterative shifted regressors GLM approach, for example.
If it is not possible to record the PETCO2, it should still be possible to obtain a non-normalized CVR map from a BH fMRI dataset. The GLM framework may again be used, but in this case we recommend using a Fourier-based description of the BH protocol, which depends only on the knowledge of the period of the task and not on a measured PETCO2 trace. In particular, we recommend using a GLM including a pair of sine and cosine regressors, together with their first two harmonics; this accounts for variable phases, or time delays, and has been shown to provide a good model of a BH BOLD response.
When studying pathologies where vessels are in a dilated state at baseline, hypercapnic/vasodilating challenges such as BH might not be appropriate. In these cases, PDB tasks eliciting a hypocapnic response may be more appropriate. Because the number of studies employing PDB tasks is still limited, it is not possible to make recommendations for the specific PDB protocol parameters based on the literature at this moment.
In the case of less cooperative participants, such as small children, and elderly or cognitively impaired patients, resting-state methods may be the best choice as they do not depend on task compliance. Nevertheless, again not enough data exist yet to allow the identification of one preferred acquisition and analysis protocol in this case.
In Figure 5 we summarize our recommended guidelines in a “CVR mapping pipeline”, that includes a BH protocol and GLM analysis, as well as the different alternatives.
Future Work
Further investigation is still required in order to understand the physiological mechanisms underlying the BOLD response to changes in CO2 levels, as well as its spatiotemporal dynamics. In particular, it may be insightful to combine BOLD imaging with methodologies that measure neuronal activation (e.g., EEG) and/or CBF (e.g., ASL) directly, in order to allow the separation of different potential contributions to the measured BOLD response. Moreover, the more detailed and systematic investigation of the spatiotemporal dynamics of the BOLD response to CO2 variations might provide additional information of interest. The use of accelerated MRI strategies (e.g., simultaneous multi-slice acquisition schemes) providing higher temporal resolution might be advantageous for this purpose. Moreover, in resting-state studies, this may allow the separation of different physiological contributions to the BOLD signal by avoiding aliasing of faster fluctuations. Multi-echo acquisitions may offer further benefits, allowing for automatic decomposition of the data to isolate physiologic BOLD signal fluctuations from concurrent motion or other artifacts (Moia et al., 2020b).
In this work, we propose the use of a canonical HRF as the impulse response function when modeling the BOLD response to respiratory tasks. However, this aspect still requires further investigation: in analyses that do not include voxelwise optimization of vascular delay in the BOLD response, the latency of the response function would directly impact the resulting CVR amplitude estimates. While we recommend using an analysis method that accounts for differences in vascular transit and local response dynamics, it may still be beneficial to systematically explore the impact of different response functions, and potentially spatially-varying response functions, when analyzing these data (Golestani et al., 2015; Pinto et al., 2017; Kassinopoulos and Mitsis, 2019).
In terms of vasoactive modulation, more studies of PDB tasks are required to deliver sufficient data and allow more general conclusions. Combined breathing protocols should also be explored. In fact, a newly proposed breathing protocol based on rs-fMRI combined with intermittent breath modulation was indicated as another option for less cooperative participants (Liu et al., 2020). This strategy combines the large dynamic range of changes induced by breathing tasks with the easiness of resting-state methods. Another very recently presented approach implements breathing tasks at the beginning of the rs-fMRI acquisition to achieve similar benefits while maintaining a truly “resting-state” portion of the acquisition (Stickland et al., 2020).
Overall, the convergence of standardized practices remains crucial for the broader application of CVR methodologies without gas challenges. Future studies should therefore consider following the practical guidelines or alternative strategies outlined in this review as much as possible, and more studies should be conducted to assess the impact of protocol details on CVR mapping.
Conclusion
In this work, we overviewed the methods used to map CVR using MRI based on task-induced and resting-state (spontaneous) breathing modulations. Validation studies are still required in order to derive definitive conclusions regarding the best practices. However, based on the methodological overview performed in this review, we outline a set of practical guidelines. In particular, we recommend the use of a BH task in combination with a GLM framework for data analysis, but other strategies can also be productively employed depending on the data available and population studied. Overall, we hope that this review will help motivate the wider and more consistent use of CVR mapping techniques without gas challenges.
Author Contributions
JP and PF contributed to the conception and initial design of the manuscript. JP performed the search and selection of the relevant studies. JP wrote the manuscript. MB, DB, and PF contributed to the manuscript revision and editing. All authors read and approved the submitted version.
Funding
This work was supported by the Portuguese Foundation for Science and Technology (FCT) grants UID/EEA/50009/2020 and PD/BD/135114/2017, by the Engineering and Physical Sciences Research Council (EPSRC) grant EP/S021507/1, and by the Eunice Kennedy Shriver National Institute of Child Health and Human Development of the National Institutes of Health under award number K12HD073945.
Conflict of Interest
The authors declare that the research was conducted in the absence of any commercial or financial relationships that could be construed as a potential conflict of interest.
Footnotes
- ^ https://fsl.fmrib.ox.ac.uk/fsl/fslwiki
- ^ https://www.fil.ion.ucl.ac.uk/spm/
- ^ https://afni.nimh.nih.gov/
References
Abbott, D. F., Opdam, H. I., Briellmann, R. S., and Jackson, G. D. (2005). Brief breath holding may confound functional magnetic resonance imaging studies. Hum. Brain Mapp. 24, 284–290. doi: 10.1002/hbm.20086
Ainslie, P. N., and Duffin, J. (2009). Integration of cerebrovascular CO2 reactivity and chemoreflex control of breathing: mechanisms of regulation, measurement, and interpretation. Am. J. Physiol. Regul. Integr. Comp. Physiol. 296, R1473–R1495. doi: 10.1152/ajpregu.91008.2008
Alsop, D. C., Detre, J. A., Golay, X., Günther, M., Hendrikse, J., Hernandez-Garcia, L., et al. (2015). Recommended implementation of arterial spin-labeled perfusion MRI for clinical applications: a consensus of the ISMRM perfusion study group and the European consortium for ASL in dementia. Magn. Reson. Med. 73:scone. doi: 10.1002/mrm.25607
Amemiya, S., Kunimatsu, A., Saito, N., and Ohtomo, K. (2014). Cerebral hemodynamic impairment: assessment with resting-state functional MR imaging. Radiology 270, 548–555. doi: 10.1148/radiol.13130982
Andrade, K. C., Pontes-Neto, O. M., Leite, J. P., Santos, A. C., Baffa, O., and de Araujo, D. B. (2006). Quantitative aspects of brain perfusion dynamic induced by BOLD fMRI. Arq. Neuropsiquiatr. 64, 895–898. doi: 10.1590/s0004-282x2006000600001
Aslan, S., Xu, F., Wang, P. L., Uh, J., Yezhuvath, U. S., Van Osch, M., et al. (2010). Estimation of labeling efficiency in pseudocontinuous arterial spin labeling. Magn. Reson. Med. 63, 765–771. doi: 10.1002/mrm.22245
Bhogal, A. A., De Vis, J. B., Siero, J. C. W., Petersen, E. T., Luijten, P. R., Hendrikse, J., et al. (2016). The BOLD cerebrovascular reactivity response to progressive hypercapnia in young and elderly. Neuroimage 139, 94–102. doi: 10.1016/J.NEUROIMAGE.2016.06.010
Bhogal, A. A., Philippens, M. E. P., Siero, J. C. W., Fisher, J. A., Petersen, E. T., Luijten, P. R., et al. (2015). Examining the regional and cerebral depth-dependent BOLD cerebrovascular reactivity response at 7T. Neuroimage 114, 239–248. doi: 10.1016/j.neuroimage.2015.04.014
Bhogal, A. A., Siero, J. C. W., Fisher, J. A., Froeling, M., Luijten, P., Philippens, M., et al. (2014). Investigating the non-linearity of the BOLD cerebrovascular reactivity response to targeted hypo/hypercapnia at 7T. Neuroimage 98, 296–305. doi: 10.1016/j.neuroimage.2014.05.006
Birn, R. M. (2012). The role of physiological noise in resting-state functional connectivity. Neuroimage 62, 864–870. doi: 10.1016/j.neuroimage.2012.01.016
Birn, R. M., Smith, M. A., Jones, T. B., and Bandettini, P. A. (2008). The respiration response function: the temporal dynamics of fMRI signal fluctuations related to changes in respiration. Neuroimage 40, 644–654. doi: 10.1016/j.neuroimage.2007.11.059
Biswal, B., Hudetz, A. G., Yetkin, F. Z., Haughton, V. M., and Hyde, J. S. (1997). Hypercapnia reversibly suppresses low-frequency fluctuations in the human motor cortex during rest using Echo–Planar MRI. J. Cereb. Blood Flow Metab. 17, 301–308. doi: 10.1097/00004647-199703000-00007
Biswal, B., Yetkin, F. Z., Haughton, V. M., and Hyde, J. S. (1995). Functional connectivity in the motor cortex of resting human brain using echo-planar MRI. Magn. Reson. Med. 34, 537–541. doi: 10.1002/mrm.1910340409
Biswal, B. B., Kannurpatti, S. S., and Rypma, B. (2007). Hemodynamic scaling of fMRI-BOLD signal: validation of low-frequency spectral amplitude as a scalability factor. Magn. Reson. Imaging 25, 1358–1369. doi: 10.1016/j.mri.2007.03.022
Brian, J. E. (1998). Carbon dioxide and the cerebral circulation. Anesthesiology 88, 1365–1386. doi: 10.1097/00000542-199805000-00029
Bright, M. G., Bulte, D. P., Jezzard, P., and Duyn, J. (2009). Characterization of regional heterogeneity in cerebrovascular reactivity dynamics using novel hypocapnia task and BOLD fMRI. Neuroimage 48, 166–175. doi: 10.1016/j.neuroimage.2009.05.026
Bright, M. G., Croal, P. L., Blockley, N. P., and Bulte, D. P. (2019). Multiparametric measurement of cerebral physiology using calibrated fMRI. Neuroimage 187, 128–144. doi: 10.1016/j.neuroimage.2017.12.049
Bright, M. G., Donahue, M., Duyn, J., Jezzard, P., and Bulte, D. P. (2011). The effect of basal vasodilation on hypercapnic and hypocapnic reactivity measured using magnetic resonance imaging. J. Cereb. Blood Flow Metab. 31, 426–438. doi: 10.1038/jcbfm.2010.187
Bright, M. G., and Murphy, K. (2013). Reliable quantification of BOLD fMRI cerebrovascular reactivity despite poor breath-hold performance. Neuroimage 83, 559–568. doi: 10.1016/j.neuroimage.2013.07.007
Bright, M. G., and Murphy, K. (2015). Is fMRI “noise” really noise? Resting state nuisance regressors remove variance with network structure. Neuroimage 114, 158–169. doi: 10.1016/j.neuroimage.2015.03.070
Bright, M. G., Tench, C. R., and Murphy, K. (2017). Potential pitfalls when denoising resting state fMRI data using nuisance regression. Neuroimage 154, 159–168. doi: 10.1016/j.neuroimage.2016.12.027
Bulte, D., and Wartolowska, K. (2017). Monitoring cardiac and respiratory physiology during FMRI. Neuroimage 154, 81–91. doi: 10.1016/j.neuroimage.2016.12.001
Bulte, D. P., Drescher, K., and Jezzard, P. (2009). Comparison of hypercapnia-based calibration techniques for measurement of cerebral oxygen metabolism with MRI. Magn. Reson. Med. 61, 391–398. doi: 10.1002/mrm.21862
Buterbaugh, J., Wynstra, C., Provencio, N., Combs, D., Gilbert, M., and Parthasarathy, S. (2015). Cerebrovascular reactivity in young subjects with sleep Apnea. Sleep 38, 241–250. doi: 10.5665/sleep.4406
Caballero-Gaudes, C., and Reynolds, R. C. (2017). Methods for cleaning the BOLD fMRI signal. Neuroimage 154, 128–149. doi: 10.1016/J.NEUROIMAGE.2016.12.018
Champagne, A. A., Bhogal, A. A., Coverdale, N. S., Mark, C. I., and Cook, D. J. (2019). A novel perspective to calibrate temporal delays in cerebrovascular reactivity using hypercapnic and hyperoxic respiratory challenges. Neuroimage 187, 154–165. doi: 10.1016/j.neuroimage.2017.11.044
Chang, C., and Glover, G. H. (2009). Relationship between respiration, end-tidal CO2, and BOLD signals in resting-state fMRI. Neuroimage 47, 1381–1393. doi: 10.1016/j.neuroimage.2009.04.048
Chang, C., Metzger, C. D., Glover, G. H., Duyn, J. H., Heinze, H.-J., and Walter, M. (2013). Association between heart rate variability and fluctuations in resting-state functional connectivity. Neuroimage 68, 93–104. doi: 10.1016/J.NEUROIMAGE.2012.11.038
Chang, C., Thomason, M. E., and Glover, G. H. (2008). Mapping and correction of vascular hemodynamic latency in the BOLD signal. Neuroimage 43, 90–102. doi: 10.1016/j.neuroimage.2008.06.030
Chang, T.-Y., Liu, H.-L., Lee, T.-H., Kuan, W.-C., Chang, C.-H., Wu, H.-C., et al. (2009). Change in cerebral perfusion after carotid angioplasty with stenting is related to cerebral vasoreactivity: a study using dynamic susceptibility-weighted contrast-enhanced MR imaging and functional MR imaging with a breath-holding paradigm. AJNR Am. J. Neuroradiol. 30, 1330–1336. doi: 10.3174/ajnr.A1589
Chen, J. J., and Pike, G. B. (2010). Global cerebral oxidative metabolism during hypercapnia and hypocapnia in humans: implications for BOLD fMRI. J. Cereb. Blood Flow Metab. 30, 1094–1099. doi: 10.1038/jcbfm.2010.42
Cheng, R., Qi, H., Liu, Y., Zhao, S., Li, C., Liu, C., et al. (2017). Abnormal amplitude of low-frequency fluctuations and functional connectivity of resting-state functional magnetic resonance imaging in patients with leukoaraiosis. Brain Behav. 7:e00714. doi: 10.1002/brb3.714
Christen, T., Jahanian, H., Ni, W. W., Qiu, D., Moseley, M. E., and Zaharchuk, G. (2015). Noncontrast mapping of arterial delay and functional connectivity using resting-state functional MRI: a study in Moyamoya patients. J. Magn. Reson. Imaging 41, 424–430. doi: 10.1002/jmri.24558
Cohen, A. D., and Wang, Y. (2019). Improving the assessment of breath-holding induced cerebral vascular reactivity using a multiband multi-echo AsL/BoLD sequence. Sci. Rep. 9:5079. doi: 10.1038/s41598-019-41199-w
Cohen, E. R., Ugurbil, K., and Kim, S.-G. (2002). Effect of basal conditions on the magnitude and dynamics of the blood oxygenation level-dependent fMRI response. J. Cereb. Blood Flow Metab. 22, 1042–1053. doi: 10.1097/00004647-200209000-00002
Conijn, M. M. A., Hoogduin, J. M., van der Graaf, Y., Hendrikse, J., Luijten, P. R., and Geerlings, M. I. (2012). Microbleeds, lacunar infarcts, white matter lesions and cerebrovascular reactivity — A 7 T study. Neuroimage 59, 950–956. doi: 10.1016/J.NEUROIMAGE.2011.08.059
De Vis, J. B., Bhogal, A. A., Hendrikse, J., Petersen, E. T., and Siero, J. C. W. (2018). Effect sizes of BOLD CVR, resting-state signal fluctuations and time delay measures for the assessment of hemodynamic impairment in carotid occlusion patients. Neuroimage 179, 530–539. doi: 10.1016/j.neuroimage.2018.06.017
Detre, J. A., Leigh, J. S., Williams, D. S., and Koretsky, A. P. (1992). Perfusion imaging. Magn. Reson. Med. 23, 37–45.
Dlamini, N., Shah-Basak, P., Leung, J., Kirkham, F., Shroff, M., Kassner, A., et al. (2018). Breath-hold blood oxygen level–dependent MRI: a tool for the assessment of cerebrovascular reserve in children with Moyamoya disease. Am. J. Neuroradiol. 39, 1717–1723. doi: 10.3174/ajnr.A5739
Donahue, M. J., Strother, M. K., Lindsey, K. P., Hocke, L. M., Tong, Y., and Frederick, B. D. (2016). Time delay processing of hypercapnic fMRI allows quantitative parameterization of cerebrovascular reactivity and blood flow delays. J. Cereb. Blood Flow Metab. 36, 1767–1779. doi: 10.1177/0271678X15608643
Draghici, A. E., and Taylor, J. A. (2016). The physiological basis and measurement of heart rate variability in humans. J. Physiol. Anthropol. 35:22. doi: 10.1186/s40101-016-0113-7
Duffin, J., Sobczyk, O., Crawley, A. P., Poublanc, J., Mikulis, D. J., and Fisher, J. A. (2015). The dynamics of cerebrovascular reactivity shown with transfer function analysis. Neuroimage 114, 207–216. doi: 10.1016/J.NEUROIMAGE.2015.04.029
Erdoğan, S. B., Tong, Y., Hocke, L. M., Lindsey, K. P., and deB Frederick, B. (2016). Correcting for blood arrival time in global mean regression enhances functional connectivity analysis of resting state fMRI-BOLD signals. Front. Hum. Neurosci. 10:311. doi: 10.3389/fnhum.2016.00311
Fierstra, J., Sobczyk, O., Battisti-Charbonney, A., Mandell, D. M., Poublanc, J., Crawley, A. P., et al. (2013). Measuring cerebrovascular reactivity: What stimulus to use? J. Physiol. 591, 5809–5821. doi: 10.1113/jphysiol.2013.259150
Fierstra, J., van Niftrik, C., Warnock, G., Wegener, S., Piccirelli, M., Pangalu, A., et al. (2018). Staging hemodynamic failure with blood oxygen-level–dependent functional magnetic resonance imaging cerebrovascular reactivity. Stroke 49, 621–629. doi: 10.1161/STROKEAHA.117.020010
Fisher, J. A., Sobczyk, O., Crawley, A., Poublanc, J., Dufort, P., Venkatraghavan, L., et al. (2017). Assessing cerebrovascular reactivity by the pattern of response to progressive hypercapnia. Hum. Brain Mapp. 38, 3415–3427. doi: 10.1002/hbm.23598
Frederick, B. B., Nickerson, L. D., and Tong, Y. (2012). Physiological denoising of BOLD fMRI data using Regressor Interpolation at Progressive Time Delays (RIPTiDe) processing of concurrent fMRI and near-infrared spectroscopy (NIRS). Neuroimage 60, 1913–1923. doi: 10.1016/j.neuroimage.2012.01.140
Friedman, L., Turner, J. A., Stern, H., Mathalon, D. H., Trondsen, L. C., and Potkin, S. G. (2008). Chronic smoking and the BOLD response to a visual activation task and a breath hold task in patients with schizophrenia and healthy controls. Neuroimage 40, 1181–1194. doi: 10.1016/j.neuroimage.2007.12.040
Friston, K. J., Williams, S., Howard, R., Frackowiak, R. S. J., and Turner, R. (1996). Movement-related effects in fMRI time-series. Magn. Reson. Med. 35, 346–355. doi: 10.1002/mrm.1910350312
Geranmayeh, F., Wise, R. J. S., Leech, R., and Murphy, K. (2015). Measuring vascular reactivity with breath-holds after stroke: a method to aid interpretation of group-level BOLD signal changes in longitudinal fMRI studies. Hum. Brain Mapp. 36, 1755–1771. doi: 10.1002/hbm.22735
Golestani, A. M., Chang, C., Kwinta, J. B., Khatamian, Y. B., and Jean Chen, J. (2015). Mapping the end-tidal CO2 response function in the resting-state BOLD fMRI signal: spatial specificity, test–retest reliability and effect of fMRI sampling rate. Neuroimage 104, 266–277. doi: 10.1016/j.neuroimage.2014.10.031
Golestani, A. M., Kwinta, J. B., Strother, S. C., Khatamian, Y. B., and Chen, J. J. (2016a). The association between cerebrovascular reactivity and resting-state fMRI functional connectivity in healthy adults: the influence of basal carbon dioxide. Neuroimage 132, 301–313. doi: 10.1016/j.neuroimage.2016.02.051
Golestani, A. M., Wei, L. L., and Chen, J. J. (2016b). Quantitative mapping of cerebrovascular reactivity using resting-state BOLD fMRI: validation in healthy adults. Neuroimage 138, 147–163. doi: 10.1016/j.neuroimage.2016.05.025
Gonzales, M. M., Tarumi, T., Mumford, J. A., Ellis, R. C., Hungate, J. R., Pyron, M., et al. (2014). Greater BOLD response to working memory in endurance-trained adults revealed by breath-hold calibration. Hum. Brain Mapp. 35, 2898–2910. doi: 10.1002/hbm.22372
Goode, S. D., Krishan, S., Alexakis, C., Mahajan, R., and Auer, D. P. (2009). Precision of cerebrovascular reactivity assessment with use of different quantification methods for hypercapnia functional MR imaging. AJNR Am. J. Neuroradiol. 30, 972–977. doi: 10.3174/ajnr.A1496
Greicius, M. D., Krasnow, B., Reiss, A. L., and Menon, V. (2003). Functional connectivity in the resting brain: a network analysis of the default mode hypothesis. Proc. Natl. Acad. Sci. U.S.A. 100, 253–258. doi: 10.1073/pnas.0135058100
Haight, T. J., Bryan, R. N., Erus, G., Davatzikos, C., Jacobs, D. R., D’Esposito, M., et al. (2015). Vascular risk factors, cerebrovascular reactivity, and the default-mode brain network. Neuroimage 115, 7–16. doi: 10.1016/j.neuroimage.2015.04.039
Hajjar, I., Zhao, P., Alsop, D., and Novak, V. (2010). Hypertension and cerebral vasoreactivity: a continuous arterial spin labeling magnetic resonance imaging study. Hypertension 56, 859–864. doi: 10.1161/HYPERTENSIONAHA.110.160002
Halani, S., Kwinta, J. B., Golestani, A. M., Khatamian, Y. B., and Chen, J. J. (2015). Comparing cerebrovascular reactivity measured using BOLD and cerebral blood flow MRI: the effect of basal vascular tension on vasodilatory and vasoconstrictive reactivity. Neuroimage 110, 110–123. doi: 10.1016/j.neuroimage.2015.01.050
Handwerker, D., Gazzaley, A., Inglis, B. A., and D’Esposito, M. (2007). Reducing vascular variability of fMRI data across aging populations using a breath holding task. Hum. Brain Mapp. 28, 846–859. doi: 10.1002/hbm.20307
Harvey, A. K., Pattinson, K. T. S., Brooks, J. C. W., Mayhew, S. D., Jenkinson, M., and Wise, R. G. (2008). Brainstem functional magnetic resonance imaging: disentangling signal from physiological noise. J. Magn. Reson. Imaging 28, 1337–1344. doi: 10.1002/jmri.21623
Hauser, T. K., Seeger, A., Bender, B., Klose, U., Thurow, J., Ernemann, U., et al. (2019). Hypercapnic BOLD MRI compared to H 215 O PET/CT for the hemodynamic evaluation of patients with Moyamoya Disease. Neuroimage Clin. 22:101713. doi: 10.1016/j.nicl.2019.101713
Heijtel, D. F. R., Mutsaerts, H. J. M. M., Bakker, E., Schober, P., Stevens, M. F., Petersen, E. T., et al. (2014). Accuracy and precision of pseudo-continuous arterial spin labeling perfusion during baseline and hypercapnia: a head-to-head comparison with 15O H2O positron emission tomography. Neuroimage 92, 182–192. doi: 10.1016/j.neuroimage.2014.02.011
Hou, X., Liu, P., Li, Y., Jiang, D., De Vis, J. B., Lin, Z., et al. (2020). The association between BOLD-based cerebrovascular reactivity (CVR) and end-tidal CO2 in healthy subjects. Neuroimage 207:116365. doi: 10.1016/j.neuroimage.2019.116365
Hsu, Y.-Y., Chang, C.-N., Jung, S.-M., Lim, K.-E., Huang, J.-C., Fang, S.-Y., et al. (2004). Blood oxygenation level-dependent MRI of cerebral gliomas during breath holding. J. Magn. Reson. Imaging 19, 160–167. doi: 10.1002/jmri.10447
Hua, J., Stevens, R. D., Huang, A. J., Pekar, J. J., and Van Zijl, P. C. (2011). Physiological origin for the BOLD poststimulus undershoot in human brain: vascular compliance versus oxygen metabolism. J. Cereb. Blood Flow Metab. 31, 1599–1611. doi: 10.1038/jcbfm.2011.35
Hund-Georgiadis, M., Zysset, S., Naganawa, S., Norris, D. G., and von Cramon, D. Y. (2003). Determination of cerebrovascular reactivity by means of fMRI signal changes in cerebral microangiopathy: a correlation with morphological abnormalities. Cerebrovasc. Dis. 16, 158–165. doi: 10.1159/000070596
Iranmahboob, A., Peck, K. K., Brennan, N. P., Karimi, S., Fisicaro, R., Hou, B., et al. (2016). Vascular reactivity maps in patients with gliomas using breath-holding BOLD fMRI. J. Neuroimaging 26, 232–239. doi: 10.1111/jon.12278
Ito, S., Mardimae, A., Han, J., Duffin, J., Wells, G., Fedorko, L., et al. (2008). Non-invasive prospective targeting of arterial P CO 2 in subjects at rest. J. Physiol. 586, 3675–3682. doi: 10.1113/jphysiol.2008.154716
Jahanian, H., Christen, T., Moseley, M. E., Pajewski, N. M., Wright, C. B., Tamura, M. K., et al. (2017). Measuring vascular reactivity with resting-state blood oxygenation level-dependent (BOLD) signal fluctuations: a potential alternative to the breath-holding challenge? J. Cereb. Blood Flow Metab. 37, 2526–2538. doi: 10.1177/0271678X16670921
Jahanian, H., Ni, W. W., Christen, T., Moseley, M. E., Tamura, M. K., and Zaharchuk, G. (2014). Spontaneous BOLD Signal fluctuataions in young healthy subjects and elderly patients with chronic kidney disease. PLoS One 9:e92539. doi: 10.1371/journal.pone.0092539
Julien, C. (2006). The enigma of Mayer waves: facts and models. Cardiovasc. Res. 70, 12–21. doi: 10.1016/j.cardiores.2005.11.008
Kalcher, K., Boubela, R. N., Huf, W., Biswal, B. B., Baldinger, P., Sailer, U., et al. (2013). RESCALE: voxel-specific task-fMRI scaling using resting state fluctuation amplitude. Neuroimage 70, 80–88. doi: 10.1016/j.neuroimage.2012.12.019
Kannurpatti, S. S., and Biswal, B. B. (2008). Detection and scaling of task-induced fMRI-BOLD response using resting state fluctuations. Neuroimage 40, 1567–1574. doi: 10.1016/j.neuroimage.2007.09.040
Kannurpatti, S. S., Motes, M. A., Biswal, B. B., and Rypma, B. (2014). Assessment of unconstrained cerebrovascular reactivity marker for large age-range FMRI studies. PLoS One 9:e88751. doi: 10.1371/journal.pone.0088751
Kannurpatti, S. S., Motes, M. A., Rypma, B., and Biswal, B. B. (2010). Neural and vascular variability and the fMRI-BOLD response in normal aging. Magn. Reson. Imaging 28, 466–476. doi: 10.1016/j.mri.2009.12.007
Kannurpatti, S. S., Motes, M. A., Rypma, B., and Biswal, B. B. (2011). Non-neural BOLD variability in block and event-related paradigms. Magn. Reson. Imaging 29, 140–146. doi: 10.1016/J.MRI.2010.07.006
Kassinopoulos, M., and Mitsis, G. D. (2019). Identification of physiological response functions to correct for fluctuations in resting-state fMRI related to heart rate and respiration. Neuroimage 202:116150. doi: 10.1016/j.neuroimage.2019.116150
Kastrup, A., Krüger, G., Glover, G. H., Neumann-Haefelin, T., and Moseley, M. E. (1999a). Regional variability of cerebral blood oxygenation response to hypercapnia. Neuroimage 10, 675–681. doi: 10.1006/nimg.1999.0505
Kastrup, A., Krüger, G., Neumann-Haefelin, T., and Moseley, M. E. (2001). Assessment of cerebrovascular reactivity with functional magnetic resonance imaging: comparison of CO(2) and breath holding. J. Magn. Reson. Imaging 19, 13–20. doi: 10.1016/s0730-725x(01)00227-2
Kastrup, A., Li, T.-Q., Takahashi, A., Glover, G. H., and Moseley, M. E. (1998). Functional magnetic resonance imaging of regional cerebral blood oxygenation changes during breath holding. Stroke 29, 2641–2645. doi: 10.1161/01.STR.29.12.2641
Kastrup, A., Li, T.-Q., Glover, G. H., and Moseley, M. E. (1999b). Cerebral blood flow-related signal changes during breath-holding. AJNR Am. J. Neuroradiol. 20, 1233–1238.
Kazan, S. M., Mohammadi, S., Callaghan, M. F., Flandin, G., Huber, L., Leech, R., et al. (2016). Vascular autorescaling of fMRI (VasA fMRI) improves sensitivity of population studies: a pilot study. Neuroimage 124, 794–805. doi: 10.1016/j.neuroimage.2015.09.033
Kiviniemi, V., Wang, X., Korhonen, V., Keinänen, T., Tuovinen, T., Autio, J., et al. (2016). Ultra-fast magnetic resonance encephalography of physiological brain activity-Glymphatic pulsation mechanisms? J. Cereb. Blood Flow Metab. 36, 1033–1045. doi: 10.1177/0271678X15622047
Kontos, H. A., Wei, E. P., Jarrell Raper, A., and Patterson, J. L. (1977). Local mechanism of CO2 action on cat pial arterioles. Stroke 8, 226–229. doi: 10.1161/01.STR.8.2.226
Krainik, A., Hund-Georgiadis, M., Zysset, S., and von Cramon, D. Y. (2005). Regional impairment of cerebrovascular reactivity and BOLD signal in adults after stroke. Stroke 36, 1146–1152. doi: 10.1161/01.STR.0000166178.40973.a7
Leoni, R. F., Mazzeto-Betti, K. C., Andrade, K. C., and de Araujo, D. B. (2008). Quantitative evaluation of hemodynamic response after hypercapnia among different brain territories by fMRI. Neuroimage 41, 1192–1198. doi: 10.1016/j.neuroimage.2008.03.035
Leoni, R. F., Mazzetto-Betti, K. C., Silva, A. C., Dos Santos, A. C., de Araujo, D. B., Leite, J. P., et al. (2012). Assessing cerebrovascular reactivity in carotid steno-occlusive disease using MRI BOLD and ASL techniques. Radiol. Res. Pract. 2012:268483. doi: 10.1155/2012/268483
Li, T.-Q., Kastrup, A., Takahashi, A. M., and Moseley, M. E. (1999a). Functional MRI of human brain during breath holding by BOLD and FAIR techniques. Neuroimage 9, 243–249. doi: 10.1006/nimg.1998.0399
Li, T.-Q., Moseley, M. E., and Glover, G. H. (1999b). A FAIR study of motor cortex activation under normo- and hypercapnia induced by breath challenge. Neuroimage 10, 562–569. doi: 10.1006/nimg.1999.0496
Lipp, I., Murphy, K., Caseras, X., and Wise, R. G. (2015). Agreement and repeatability of vascular reactivity estimates based on a breath-hold task and a resting state scan. Neuroimage 113, 387–396. doi: 10.1016/j.neuroimage.2015.03.004
Lipp, I., Murphy, K., Wise, R. G., and Caseras, X. (2014). Understanding the contribution of neural and physiological signal variation to the low repeatability of emotion-induced BOLD responses. Neuroimage 86, 335–342. doi: 10.1016/j.neuroimage.2013.10.015
Liu, H.-L., Huang, J.-C., Wu, C.-T., and Hsu, Y.-Y. (2002). Detectability of blood oxygenation level-dependent signal changes during short breath hold duration. Magn. Reson. Imaging 20, 643–648. doi: 10.1016/s0730-725x(02)00595-7
Liu, P., De Vis, J. B., and Lu, H. (2019). Cerebrovascular reactivity (CVR) MRI with CO2 challenge: a technical review. Neuroimage 187, 104–115. doi: 10.1016/j.neuroimage.2018.03.047
Liu, P., Hebrank, A. C., Rodrigue, K. M., Kennedy, K. M., Park, D. C., and Lu, H. (2013). A comparison of physiologic modulators of fMRI signals. Hum. Brain Mapp. 34, 2078–2088. doi: 10.1002/hbm.22053
Liu, P., Li, Y., Pinho, M., Park, D. C., Welch, B. G., and Lu, H. (2017a). Cerebrovascular reactivity mapping without gas challenges. Neuroimage 146, 320–326. doi: 10.1016/j.neuroimage.2016.11.054
Liu, P., Welch, B. G., King, D., Li, Y., Pinho, M., and Lu, H. (2015). Can resting state fMRI be used to map cerebrovascular reactivity? Proc. Intl. Soc. Mag. Reson. Med. 23:50.
Liu, P., Welch, B. G., Li, Y., Gu, H., King, D., Yang, Y., et al. (2017b). Multiparametric imaging of brain hemodynamics and function using gas-inhalation MRI. Neuroimage 146, 715–723. doi: 10.1016/j.neuroimage.2016.09.063
Liu, P., Xu, C., Lin, Z., Sur, S., Li, Y., Yasar, S., et al. (2020). Cerebrovascular reactivity mapping using intermittent breath modulation. Neuroimage 215:116787. doi: 10.1016/j.neuroimage.2020.116787
Lu, H., Golay, X., Pekar, J. J., and van Zijl, P. C. M. (2003). Functional magnetic resonance imaging based on changes in vascular space occupancy. Magn. Reson. Med. 50, 263–274. doi: 10.1002/mrm.10519
Lv, Y., Margulies, D. S., Cameron Craddock, R., Long, X., Winter, B., Gierhake, D., et al. (2013). Identifying the perfusion deficit in acute stroke with resting-state functional magnetic resonance imaging. Ann. Neurol. 73, 136–139. doi: 10.1002/ana.23763
MacIntosh, B. J., Klassen, L. M., and Menon, R. S. (2003). Transient hemodynamics during a breath hold challenge in a two part functional imaging study with simultaneous near-infrared spectroscopy in adult humans. Neuroimage 20, 1246–1252. doi: 10.1016/S1053-8119(03)00417-8
Magon, S., Basso, G., Farace, P., Ricciardi, G. K., Beltramello, A., and Sbarbati, A. (2009). Reproducibility of BOLD signal change induced by breath holding. Neuroimage 45, 702–712. doi: 10.1016/j.neuroimage.2008.12.059
Makedonov, I., Black, S. E., Macintosh, B. J., and Chao, L. (2013). BOLD fMRI in the white matter as a marker of aging and small vessel disease. PLoS One 8:e67652. doi: 10.1371/journal.pone.0067652
Makedonov, I., Chen, J. J., Masellis, M., MacIntosh, B. J. and Alzheimer’s Disease Neuroimaging Initiative. (2016). Physiological fluctuations in white matter are increased in Alzheimer’s disease and correlate with neuroimaging and cognitive biomarkers. Neurobiol. Aging 37, 12–18. doi: 10.1016/j.neurobiolaging.2015.09.010
Mäkiranta, M. J., Ruohonen, J., Suominen, K., Sonkajärvi, E., Salomäki, T., Kiviniemi, V., et al. (2004). BOLD-contrast functional MRI signal changes related to intermittent rhythmic delta activity in EEG during voluntary hyperventilation—simultaneous EEG and fMRI study. Neuroimage 22, 222–231. doi: 10.1016/j.neuroimage.2004.01.004
Mandell, D., Han, J., Poublanc, J., Crawley, A., Stainsby, J. A., Fisher, J. A., et al. (2008). Mapping cerebrovascular reactivity using blood oxygen level-dependent MRI in Patients with arterial steno-occlusive disease: comparison with arterial spin labeling MRI. Stroke 39, 2021–2028. doi: 10.1161/STROKEAHA.107.506709
Mark, C., Slessarev, M., Ito, S., Han, J., Fisher, J. A., and Pike, G. B. (2010). Precise control of end-tidal carbon dioxide and oxygen improves BOLD and ASL cerebrovascular reactivity measures. Magn. Reson. Med. 64, 749–756. doi: 10.1002/mrm.22405
Mark, C. I., Fisher, J. A., and Pike, G. B. (2011). Improved fMRI calibration: precisely controlled hyperoxic versus hypercapnic stimuli. Neuroimage 54, 1102–1111. doi: 10.1016/j.neuroimage.2010.08.070
Mcswain, D., Hamel, D. S., Faarc, R., Smith, B., Gentile, M. A., Srinivasan, S., et al. (2010). End-tidal and arterial carbon dioxide measurements correlate across all levels of physiologic dead space. Respir. Care 55, 288–293.
Moia, S., Stickland, R., Ayyagari, A., Termenon, M., Caballero-Gaudes, C., and Bright, M. G. (2020a). “Voxelwise optimization of hemodynamic lags to improve regional CVR estimates in breath-hold fMRI,” in Proceedings of the 42nd Annual International Conference of the IEEE Engineering in Medicine and Biology Society (EMBC), Montreal, 1489–1492. doi: 10.1109/EMBC44109.2020.9176225
Moia, S., Termenon, M., Uruñuela, E., Stickland, R. C., Bright, M. G., Caballero-Gaudes, C., et al. (2020b). ICA-based denoising strategies in breath-hold induced cerebrovascular reactivity mapping with multi Echo BOLD fMRI. bioRxiv [Preprint]. doi: 10.1101/2020.08.18.256479
Moreton, F. C., Dani, K. A., Goutcher, C., O’Hare, K., and Muir, K. W. (2016). Respiratory challenge MRI: practical aspects. Neuroimage Clin. 11, 667–677. doi: 10.1016/j.nicl.2016.05.003
Murphy, K., Birn, R. M., and Bandettini, P. A. (2013). Resting-state fMRI confounds and cleanup. Neuroimage 80, 349–359. doi: 10.1016/j.neuroimage.2013.04.001
Murphy, K., Harris, A. D., and Wise, R. G. (2011). Robustly measuring vascular reactivity differences with breath-hold: normalising stimulus-evoked and resting state BOLD fMRI data. Neuroimage 54, 369–379. doi: 10.1016/j.neuroimage.2010.07.059
Naganawa, S., Norris, D. G., Zysset, S., and Mildner, T. (2002). Regional differences of fMR signal changes induced by hyperventilation: comparison between SE-EPI and GE-EPI at 3-T. J. Magn. Reson. Imaging 30, 23–30. doi: 10.1002/jmri.10028
Ni, L., Li, J., Li, W., Zhou, F., Wang, F., Schwarz, C. G., et al. (2017). The value of resting-state functional MRI in subacute ischemic stroke: comparison with dynamic susceptibility contrast-enhanced perfusion MRI OPEN. Sci. Rep. 7:41586. doi: 10.1038/srep41586
Nishida, S., Aso, T., Takaya, S., Takahashi, Y., Kikuchi, T., Funaki, T., et al. (2019). Resting-state functional magnetic resonance imaging identifies cerebrovascular reactivity impairment in patients with arterial occlusive diseases: a pilot study. Neurosurgery 85, 680–688. doi: 10.1093/neuros/nyy434
Ogawa, S., Lee, T. M., Kay, A. R., and Tank, D. W. (1990). Brain magnetic resonance imaging with contrast dependent on blood oxygenation. Proc. Natl. Acad. Sci. U.S.A. 87, 9868–9872.
Pattinson, K. T. S., Governo, R. J., MacIntosh, B. J., Russell, E. C., Corfield, D. R., Tracey, I., et al. (2009). Opioids depress cortical centers responsible for the volitional control of respiration. J. Neurosci. 29, 8177–8186. doi: 10.1523/JNEUROSCI.1375-09.2009
Peebles, K., Celi, L., McGrattan, K., Murrell, C., Thomas, K., and Ainslie, P. N. (2007). Human cerebrovascular and ventilatory CO2 reactivity to end-tidal, arterial and internal jugular vein PCO2. J. Physiol. 584, 347–357. doi: 10.1113/jphysiol.2007.137075
Peng, S., Yang, H., Chen, C., and Shih, C. (2020). Short- and long-term reproducibility of BOLD signal change induced by breath-holding at 1.5 and 3 T. NMR Biomed. 33:e4195. doi: 10.1002/nbm.4195
Peng, T., Niazy, R., Payne, S. J., and Wise, R. G. (2013). The effects of respiratory CO2 fluctuations in the resting-state BOLD signal differ between eyes open and eyes closed. Magn. Reson. Imaging 31, 336–345. doi: 10.1016/j.mri.2012.06.013
Pillai, J. J., and Mikulis, D. J. (2014). Cerebrovascular reactivity mapping: an evolving standard for clinical functional imaging. AJNR Am. J. Neuroradiol. 36, 7–13. doi: 10.3174/ajnr.a3941
Pillai, J. J., and Zacá, D. (2011). Clinical utility of cerebrovascular reactivity mapping in patients with low grade gliomas. World J. Clin. Oncol. 2, 397–403. doi: 10.5306/wjco.v2.i12.397
Pinto, J., Chappell, M. A., Okell, T. W., Mezue, M., Segerdahl, A. R., Tracey, I., et al. (2020). Calibration of arterial spin labeling data—potential pitfalls in post-processing. Magn. Reson. Med. 83, 1222–1234. doi: 10.1002/mrm.28000
Pinto, J., Jorge, J., Sousa, I., Vilela, P., and Figueiredo, P. (2016). Fourier modeling of the BOLD response to a breath-hold task: optimization and reproducibility. Neuroimage 135, 223–231. doi: 10.1016/j.neuroimage.2016.02.037
Pinto, J., Nunes, S., Bianciardi, M., Dias, A., Silveira, L. M., Wald, L. L., et al. (2017). Improved 7 Tesla resting-state fMRI connectivity measurements by cluster-based modeling of respiratory volume and heart rate effects. Neuroimage 153, 262–272. doi: 10.1016/j.neuroimage.2017.04.009
Ponsaing, L. B., Lindberg, U., Rostrup, E., Iversen, H. K., Larsson, H. B. W., and Jennum, P. (2018). Impaired cerebrovascular reactivity in obstructive sleep apnea: a case-control study. Sleep Med. 43, 7–13. doi: 10.1016/J.SLEEP.2017.10.010
Posse, S., Olthoff, U., Weckesser, M., Jäncke, L., Müller-Gärtner, H. W., and Dager, S. R. (1997). Regional dynamic signal changes during controlled hyperventilation assessed with blood oxygen level-dependent functional MR imaging. AJNR Am. J. Neuroradiol. 18, 1763–1770.
Poulin, M. J., Liang, P. J., and Robbins, P. A. (1996). Dynamics of the cerebral blood flow response to step changes in end- tidal PCO2 and PO2 in humans. J. Appl. Physiol. 81, 1084–1095. doi: 10.1152/jappl.1996.81.3.1084
Prilipko, O., Huynh, N., Thomason, M. E., Kushida, C. A., and Guilleminault, C. (2014). An fMRI study of cerebrovascular reactivity and perfusion in obstructive sleep apnea patients before and after CPAP treatment. Sleep Med. 15, 892–898. doi: 10.1016/j.sleep.2014.04.004
Prisman, E., Slessarev, M., Han, J., Poublanc, J., Mardimae, A., Crawley, A., et al. (2008). Comparison of the effects of independently-controlled end-tidal PCO(2) and PO(2) on blood oxygen level-dependent (BOLD) MRI. J. Magn. Reson. Imaging 27, 185–191. doi: 10.1002/jmri.21102
Ratnatunga, C., and Adiseshiah, M. (1990). Increase in middle cerebral artery velocity on breath holding: a simplified test of cerebral perfusion reserve. Eur. J. Vasc. Surg. 4, 519–523. doi: 10.1016/s0950-821x(05)80795-9
Raut, R. V., Nair, V. A., Sattin, J. A., and Prabhakaran, V. (2016). Hypercapnic evaluation of vascular reactivity in healthy aging and acute stroke via functional MRI. Neuroimage Clin. 12, 173–179. doi: 10.1016/j.nicl.2016.06.016
Rebollo, I., Devauchelle, A.-D., Béranger, B., and Tallon-Baudry, C. (2018). Stomach-brain synchrony reveals a novel, delayed-connectivity resting-state network in humans. eLife 7:e33321. doi: 10.7554/ELIFE.33321.007
Riecker, A., Grodd, W., Klose, U., Schulz, J. B., Gröschel, K., Erb, M., et al. (2003). Relation between regional functional MRI activation and vascular reactivity to carbon dioxide during normal aging. J. Cereb. Blood Flow Metab. 23, 565–573. doi: 10.1097/01.WCB.0000056063.25434.04
Roberts, T., Jezzard, P., and Bulte, D. P. (2009). Comparison of breath holding techniques for the calibration of FMRI measurements of oxygen metabolism. Proc. Intl. Soc. Mag. Reason. Med. 17:1532.
Scouten, A., and Schwarzbauer, C. (2008). Paced respiration with end-expiration technique offers superior BOLD signal repeatability for breath-hold studies. Neuroimage 43, 250–257. doi: 10.1016/j.neuroimage.2008.03.052
Sobczyk, O., Battisti-Charbonney, A., Fierstra, J., Mandell, D. M., Poublanc, J., Crawley, A. P., et al. (2014). A conceptual model for CO2-induced redistribution of cerebral blood flow with experimental confirmation using BOLD MRI. Neuroimage 92, 56–68. doi: 10.1016/j.neuroimage.2014.01.051
Sousa, I., Vilela, P., and Figueiredo, P. (2014). Reproducibility of hypocapnic cerebrovascular reactivity measurements using BOLD fMRI in combination with a paced deep breathing task. Neuroimage 98, 31–41. doi: 10.1016/j.neuroimage.2014.04.049
Spano, V., Mandell, D., Sam, K., Battisti-charbonney, A., Pucci, O., Han, J., et al. (2013). CO2 blood oxygen level-dependent mr mapping of cerebrovascular reserve in a clinical population: safety, tolerability, and technical feasibility. Radiology 266, 592–598. doi: 10.1148/radiol.12112795
Stickland, R., Ayyagari, A., Zvolanek, K., and Bright, M. (2020). “Short breathing tasks at the start of a resting state scan: feasible measures of cerebrovascular reactivity,” in Proceedings of the International Society for Magnetic Resonance in Medicine Virtual Conference & Exhibition, Concord, CA.
Svaldi, D. O., Joshi, C., Robinson, M. E., Shenk, T. E., Abbas, K., Nauman, E. A., et al. (2015). Cerebrovascular Reactivity Alterations in Asymptomatic High School Football Players. Dev. Neuropsychol. 40, 80–84. doi: 10.1080/87565641.2014.973959
Svaldi, D. O., McCuen, E. C., Joshi, C., Robinson, M. E., Nho, Y., Hannemann, R., et al. (2017). Cerebrovascular reactivity changes in asymptomatic female athletes attributable to high school soccer participation. Brain Imaging Behav. 11, 98–112. doi: 10.1007/s11682-016-9509-6
Tancredi, F. B., Gauthier, C. J., Madjar, C., Bolar, D. S., Fisher, J. A., Wang, D. J. J., et al. (2012). Comparison of pulsed and pseudocontinuous arterial spin-labeling for measuring CO2 -induced cerebrovascular reactivity. J. Magn. Reson. Imaging 36, 312–321. doi: 10.1002/jmri.23658
Tancredi, F. B., and Hoge, R. D. (2013). Comparison of cerebral vascular reactivity measures obtained using breath-holding and CO2 inhalation. J. Cereb. Blood Flow Metab. 33, 1066–1074. doi: 10.1038/jcbfm.2013.48
Tancredi, F. B., Lajoie, I., and Hoge, R. D. (2014). A simple breathing circuit allowing precise control of inspiratory gases for experimental respiratory manipulations. BMC Res. Notes 7:235. doi: 10.1186/1756-0500-7-235
Taneja, K., Lu, H., Welch, B. G., Thomas, B. P., Pinho, M., Lin, D., et al. (2019). Evaluation of cerebrovascular reserve in patients with cerebrovascular diseases using resting-state MRI: a feasibility study. Magn. Reson. Imaging 59, 46–52. doi: 10.1016/j.mri.2019.03.003
Tchistiakova, E., Anderson, N. D., Greenwood, C. E., and MacIntosh, B. J. (2014). Combined effects of type 2 diabetes and hypertension associated with cortical thinning and impaired cerebrovascular reactivity relative to hypertension alone in older adults. Neuroimage Clin. 5, 36–41. doi: 10.1016/J.NICL.2014.05.020
Tchistiakova, E., Crane, D. E., Mikulis, D. J., Anderson, N. D., Greenwood, C. E., Black, S. E., et al. (2015). Vascular risk factor burden correlates with cerebrovascular reactivity but not resting state coactivation in the default mode network. J. Magn. Reson. Imaging 42, 1369–1376. doi: 10.1002/jmri.24917
Thomas, B., Logan, W., Donner, E. J., and Shroff, M. (2013). Assessment of cerebrovascular reactivity using real-time BOLD fMRI in children with moyamoya disease: a pilot study. Childs Nerv. Syst. 29, 457–463. doi: 10.1007/s00381-012-1952-0
Thomason, M. E., Burrows, B. E., Gabrieli, J. D. E., and Glover, G. H. (2005). Breath holding reveals differences in fMRI BOLD signal in children and adults. Neuroimage 25, 824–837. doi: 10.1016/j.neuroimage.2004.12.026
Thomason, M. E., Foland, L. C., and Glover, G. H. (2007). Calibration of BOLD fMRI using breath holding reduces group variance during a cognitive task. Hum. Brain Mapp. 28, 59–68. doi: 10.1002/hbm.20241
Thomason, M. E., and Glover, G. H. (2008). Controlled inspiration depth reduces variance in breath-holding-induced BOLD signal. Neuroimage 39, 206–214. doi: 10.1016/j.neuroimage.2007.08.014
Tong, Y., Bergethon, P. R., and Frederick, B. D. (2011). An improved method for mapping cerebrovascular reserve using concurrent fMRI and near-infrared spectroscopy with Regressor Interpolation at Progressive Time Delays (RIPTiDe). Neuroimage 56, 2047–2057. doi: 10.1016/j.neuroimage.2011.03.071
Tong, Y., Hocke, L. M., and Frederick, B. B. (2019a). Low frequency systemic hemodynamic “noise” in resting state BOLD fMRI: characteristics, causes, implications, mitigation strategies, and applications. Front. Neurosci. 13:787. doi: 10.3389/fnins.2019.00787
Tong, Y., Yao, J. F., Chen, J. J., and Frederick, B. D. (2019b). The resting-state fMRI arterial signal predicts differential blood transit time through the brain. J. Cereb. Blood Flow Metab. 39, 1148–1160. doi: 10.1177/0271678X17753329
Tong, Y., Lindsey, K. P., Hocke, L. M., Vitaliano, G., Mintzopoulos, D., and Frederick, B. D. (2017). Perfusion information extracted from resting state functional magnetic resonance imaging. J. Cereb. Blood Flow Metab. 37, 564–576. doi: 10.1177/0271678X16631755
Tsvetanov, K. A., Henson, R. N. A., Tyler, L. K., Davis, S. W., Shafto, M. A., Taylor, J. R., et al. (2015). The effect of ageing on fMRI: correction for the confounding effects of vascular reactivity evaluated by joint fMRI and MEG in 335 adults. Hum. Brain Mapp. 36, 2248–2269. doi: 10.1002/hbm.22768
Urback, A. L., MacIntosh, B. J., and Goldstein, B. I. (2017). Cerebrovascular reactivity measured by functional magnetic resonance imaging during breath-hold challenge: a systematic review. Neurosci. Biobehav. Rev. 79, 27–47. doi: 10.1016/j.neubiorev.2017.05.003
Urback, A. L., Metcalfe, A. W., Korczak, D. J., MacIntosh, B. J., and Goldstein, B. I. (2019). Reduced cerebrovascular reactivity among adolescents with bipolar disorder. Bipolar Disord. 21, 124–131. doi: 10.1111/bdi.12719
Urback, A. L., Metcalfe, A. W. S., Korczak, D. J., MacIntosh, B. J., and Goldstein, B. I. (2018). Magnetic resonance imaging of cerebrovascular reactivity in healthy adolescents. J. Neurosci. Methods 306, 1–9. doi: 10.1016/j.jneumeth.2018.06.001
Van de Moortele, P.-F., Pfeuffer, J., Glover, G. H., Ugurbil, K., and Hu, X. (2002). Respiration-induced B0 fluctuations and their spatial distribution in the human brain at 7 Tesla. Magn. Reson. Med. 47, 888–895. doi: 10.1002/mrm.10145
van Niftrik, C. H. B., Piccirelli, M., Bozinov, O., Maldaner, N., Strittmatter, C., Pangalu, A., et al. (2018). Impact of baseline CO 2 on Blood-Oxygenation-Level-Dependent MRI measurements of cerebrovascular reactivity and task-evoked signal activation. Magn. Reson. Imaging 49, 123–130. doi: 10.1016/j.mri.2018.02.002
van Niftrik, C. H. B., Piccirelli, M., Bozinov, O., Pangalu, A., Valavanis, A., Regli, L., et al. (2016). Fine tuning breath-hold-based cerebrovascular reactivity analysis models. Brain Behav. 6:e00426. doi: 10.1002/brb3.426
van Oers, C. A. M. M., van der Worp, H. B., Kappelle, L. J., Raemaekers, M. A. H., Otte, W. M., and Dijkhuizen, R. M. (2018). Etiology of language network changes during recovery of aphasia after stroke. Sci. Rep. 8:856. doi: 10.1038/s41598-018-19302-4
Vestergaard, M. B., and Larsson, H. B. (2019). Cerebral metabolism and vascular reactivity during breath-hold and hypoxic challenge in freedivers and healthy controls. J. Cereb. Blood Flow Metab. 39, 834–848. doi: 10.1177/0271678X17737909
Vogt, K. M., Ibinson, J. W., Schmalbrock, P., and Small, R. H. (2011). Comparison between end-tidal CO2 and respiration volume per time for detecting BOLD signal fluctuations during paced hyperventilation. Magn. Reson. Imaging 29, 1186–1194. doi: 10.1016/j.mri.2011.07.011
Wang, J., Chen, H., Liang, H., Wang, W., Liang, Y., Liang, Y., et al. (2019). Low-frequency fluctuations amplitude signals exhibit abnormalities of intrinsic brain activities and reflect cognitive impairment in leukoaraiosis patients. Med. Sci. Monit. 25, 5219–5228. doi: 10.12659/MSM.915528
Wang, P., Hou, P., Kesler, S., Colen, R., Kumar, A., Prabhu, S., et al. (2016). Resting-state fluctuation of BOLD signal amplitude for mapping cerebrovascular reactivity in presurgical functional MRI. Med. Phys. 43, 3646–3647. doi: 10.1118/1.4956971
Weckesser, M., Posse, S., Olthoff, U., Kemna, L., Dager, S., and Müller-Gärtner, H. W. (1999). Functional imaging of the visual cortex with bold-contrast MRI: hyperventilation decreases signal response. Magn. Reson. Med. 41, 213–216. doi: 10.1002/(sici)1522-2594(199901)41:1<213::aid-mrm31>3.0.co;2-s
Whittaker, J. R., Driver, I. D., Venzi, M., Bright, M. G., and Murphy, K. (2019). Cerebral autoregulation evidenced by synchronized low frequency oscillations in blood pressure and resting-state fMRI. Front. Neurosci. 13:433. doi: 10.3389/fnins.2019.00433
Williams, D. S., Detre, J. A., Leigh, J. S., and Koretsky, A. P. (1992). Magnetic resonance imaging of perfusion using spin inversion of arterial water. Proc. Natl. Acad. Sci. U.S.A. 89, 212–216.
Wise, R. G., Ide, K., Poulin, M. J., and Tracey, I. (2004). Resting fluctuations in arterial carbon dioxide induce significant low frequency variations in BOLD signal. Neuroimage 21, 1652–1664. doi: 10.1016/j.neuroimage.2003.11.025
Wu, P., Bandettini, P. A., Harper, R. M., and Handwerker, D. A. (2015). Effects of thoracic pressure changes on MRI signals in the brain. J. Cereb. Blood Flow Metab. 35, 1024–1032. doi: 10.1038/jcbfm.2015.20
Wu, P.-H., Rodríguez-Soto, A. E., Rodgers, Z. B., Englund, E. K., Wiemken, A., Langham, M. C., et al. (2019). MRI evaluation of cerebrovascular reactivity in obstructive sleep apnea. J. Cereb. Blood Flow Metab. 40, 1328–1337. doi: 10.1177/0271678X19862182
Yan, X., Zhang, J., Gong, Q., and Weng, X. (2011). Cerebrovascular reactivity among native-raised high altitude residents: an fMRI study. BMC Neurosci. 12:94. doi: 10.1186/1471-2202-12-94
Yezhuvath, U., Lewis-Amezcua, K., Varghese, R., Xiao, G., and Lu, H. (2009). On the assessment of cerebrovascular reactivity using hypercapnia BOLD MRI. NMR Biomed. 22, 779–786. doi: 10.1002/nbm.1392
Zacà, D., Jovicich, J., Nadar, S. R., Voyvodic, J. T., and Pillai, J. J. (2014). Cerebrovascular reactivity mapping in patients with low grade gliomas undergoing presurgical sensorimotor mapping with BOLD fMRI. J. Magn. Reson. Imaging 40, 383–390. doi: 10.1002/jmri.24406
Zang, Y.-F., He, Y., Zhu, C.-Z., Cao, Q.-J., Sui, M.-Q., Liang, M., et al. (2007). Altered baseline brain activity in children with ADHD revealed by resting-state functional MRI. Brain Dev. 29, 83–91. doi: 10.1016/j.braindev.2006.07.002
Zhao, P., Alsop, D. C., AbdulJalil, A., Selim, M., Lipsitz, L., Novak, P., et al. (2009). Vasoreactivity and peri-infarct hyperintensities in stroke. Neurology 72, 643–649. doi: 10.1212/01.wnl.0000342473.65373.80
Zou, Q.-H., Zhu, C.-Z., Yang, Y., Zuo, X.-N., Long, X.-Y., Cao, Q.-J., et al. (2008). An improved approach to detection of amplitude of low-frequency fluctuation (ALFF) for resting-state fMRI: fractional ALFF. J. Neurosci. Methods 172, 137–141. doi: 10.1016/j.jneumeth.2008.04.012
Keywords: cerebrovascular reactivity, MRI, breath-hold, paced deep breathing, resting-state
Citation: Pinto J, Bright MG, Bulte DP and Figueiredo P (2021) Cerebrovascular Reactivity Mapping Without Gas Challenges: A Methodological Guide. Front. Physiol. 11:608475. doi: 10.3389/fphys.2020.608475
Received: 30 September 2020; Accepted: 02 December 2020;
Published: 18 January 2021.
Edited by:
Itamar Ronen, Leiden University Medical Center, NetherlandsReviewed by:
Yunjie Tong, Purdue University, United StatesTommaso Gili, IMT School for Advanced Studies Lucca, Italy
Copyright © 2021 Pinto, Bright, Bulte and Figueiredo. This is an open-access article distributed under the terms of the Creative Commons Attribution License (CC BY). The use, distribution or reproduction in other forums is permitted, provided the original author(s) and the copyright owner(s) are credited and that the original publication in this journal is cited, in accordance with accepted academic practice. No use, distribution or reproduction is permitted which does not comply with these terms.
*Correspondence: Patrícia Figueiredo, cGF0cmljaWEuZmlndWVpcmVkb0B0ZWNuaWNvLnVsaXNib2EucHQ=