- 1Electrical Engineering Department, Centro de Investigación y de Estudios Avanzados del Instituto Politécnico Nacional, Guadalajara, Mexico
- 2Biomedical Sciences Department, Centro de Investigación Multidisciplinario en Salud, Universidad de Guadalajara, Tonalá, Mexico
- 3Biochemistry and Molecular Biology Department, Instituto de Investigaciones Básicas y Aplicadas, Universidad Autónoma del Estado de Morelos, Cuernavaca, Mexico
- 4Computer Sciences Department, Universidad de Guadalajara, Guadalajara, Mexico
- 5Electrical Engineering Department, City University of Hong Kong, Hong Kong, China
- 6Frankfurt Institute for Advanced Studies, Frankfurt, Germany
p53 regulates the cellular response to genotoxic damage and prevents carcinogenic events. Theoretical and experimental studies state that the p53-Mdm2 network constitutes the core module of regulatory interactions activated by cellular stress induced by a variety of signaling pathways. In this paper, a strategy to control the p53-Mdm2 network regulated by p14ARF is developed, based on the pinning control technique, which consists into applying local feedback controllers to a small number of nodes (pinned ones) in the network. Pinned nodes are selected on the basis of their importance level in a topological hierarchy, their degree of connectivity within the network, and the biological role they perform. In this paper, two cases are considered. For the first case, the oscillatory pattern under gamma-radiation is recovered; afterward, as the second case, increased expression of p53 level is taken into account. For both cases, the control law is applied to p14ARF (pinned node based on a virtual leader methodology), and overexpressed Mdm2-mediated p53 degradation condition is considered as carcinogenic initial behavior. The approach in this paper uses a computational algorithm, which opens an alternative path to understand the cellular responses to stress, doing it possible to model and control the gene regulatory network dynamics in two different biological contexts. As the main result of the proposed control technique, the two mentioned desired behaviors are obtained.
1. Introduction
Gene regulatory networks play key roles in every process of life, including cell cycle, metabolism, signal transduction, cell communication, and cellular differentiation. These complex biological networks use large amounts of data, necessary for modeling, analyzing, and controlling. Mathematical and computational methods are very helpful approaches for constructing network models at molecule level to predict cell behavior under normal conditions or pathological ones. Network topology and interactions between nodes (representing molecules, proteins, genes, mRNA, and others), and edges (establishing regulatory properties) describe the network dynamical behavior (Bolouri and Davidson, 2002). Different mathematical models have been developed for studying gene regulatory networks, which can be divided into four classes (De Jong, 2002): the first ones are logical models, which describe regulatory networks qualitatively, namely, Boolean networks (Kauffman, 1969; Akutsu et al., 1999; Wang et al., 2012), probabilistic Boolean networks (Shmulevich et al., 2002a,b), Bayesian networks (Friedman et al., 2000; Rau et al., 2010), and Petri nets (Chaouiya, 2007; Karlebach and Shamir, 2008); the second ones are defined by continuous models such as ordinary differential equations (Chen et al., 1999; Szallasi et al., 2006; Cao et al., 2012), and the S-system formalism (Kikuchi et al., 2003; Wang et al., 2010); the third ones are single-molecule level models (Cai et al., 2006; Elf et al., 2007; Selvin and Ha, 2008), which account for interactions among molecules; and the last ones are hybrid models combining different formulations like discrete-time and continuous-time frameworks (Ahmad et al., 2006; Fromentin et al., 2010). The continuous-time approach consists in connecting a group of dependent variables to biochemical reaction kinetics. In this case, it is essential to assume that molecules have constant concentrations with respect to cellular compartments, in which their variations are continuous functions of time (Chen et al., 1999; Szallasi et al., 2006; Cao et al., 2012). This approach is adopted in the present paper.
On the other hand, control theory has rapidly developed for complex networks (Wang and Chen, 2002; Sorrentino et al., 2007; Liu and Barabási, 2016). Recently, research focuses on the important issue of how to incorporate control techniques for biological systems and networks (Nowzari et al., 2016; Vinayagam et al., 2016; Gao et al., 2017; Jiao et al., 2018; Papatsenko et al., 2018; Wang et al., 2019), such as pinning control for gene regulatory networks (Lin et al., 2014; Chen et al., 2016; Yue et al., 2017; Li et al., 2018; Burbano et al., 2019). In Lin et al. (2014), a Boolean network model to reproduce the two-phase dynamics of the p53 network in response to DNA damage is developed. In particular, two types of Boolean attractors are presented; the first one is an apoptosis attractor and the second one is a repair attractor. Based on this model, practical control schemes for steering into the apoptosis attractor in presence of DNA damage by pinning the state of a single node or perturbing the weight of a single link are applied. In Chen et al. (2016), an Autonomous Boolean Control Networks (ABCNs) for designing and analyzing the therapeutic intervention strategies are introduced. An important issue in therapeutic intervention is to design a control sequence steering an ABCN from an undesirable location (implying a diseased condition) to a desirable one (corresponding to a healthy condition). Based on this motivation, pinning control strategy is proposed for steering an ABCN from any given condition to the desired one in the shortest time. Cluster synchronization of the coupled genetic regulatory network, represent with ABCN model is investigated in Yue et al. (2017) with a directed topology and using the event-based strategy and pinning control; for this network, a synthetic regulatory network analogous to that in Escherichia coli is proposed with twelve states, where three states are the pinned nodes. In Li et al. (2018), a single-input pinning controller design for reachability of Boolean networks is proposed; in addition, different nodes are selected as the pinning ones by solving logical matrix equations and Drosophila melanogaster gene regulatory network is used to illustrate the effectiveness and feasibility of the developed method. Finally, Burbano et al. (2019) pinning controllability analysis of multiagent networks subjected to three different types of noise diffusion processes; namely, noise affecting the node dynamics, the communication links, and the pinning control action is done, the effectiveness of the theoretical results is illustrated in the genetic Toggle Switch, originally introduced in E. coli. This last publication uses a model based on stochastic differential equations.
Additionally, gene regulatory networks present responses to DNA damage such as cell cycle arrest, DNA repair, senescence, apoptosis among others. Among the main regulators of these responses, tumor suppressor protein p53 (TP53) has been recognized as the “guardian of the genome” and is a key component of cellular responses to genotoxic stress (Lane, 1992). p53 regulates the cellular response to genotoxic damage and prevents tumorigenesis by post-translational modifications and gene transactivation (Ashcroft et al., 2000). Without cellular stress, p53 remains inactive and latent due to targeted degradation by the protein E3 ubiquitin-protein ligase Mdm2 (from MDM2 proto-oncogene). Mdm2 binds to p53 and marks it for proteasome degradation, preventing p53 accumulation in the nucleus and its transcriptional activity (Momand et al., 1992). In this way, the activity of p53 and its negative regulation by Mdm2 are widely recognized as one of the main regulatory mechanisms in genotoxic stress response (Sionov and Haupt, 1999). The p53-Mdm2 network models have been studied in Wagner et al. (2005), Geva-Zatorsky et al. (2006), Sykes et al. (2006), Wee et al. (2009), Wee et al. (2012), and Hafner et al. (2017), which describe different patterns of response as promotion of transcriptional activities, post-translational modifications, component interactions, and degradation rates. Another important regulator of the p53-Mdm2 network is the tumor suppressor p14ARF (Alternate Reading Frame), one product of the CDKN2A gene. p14ARF inhibits Mdm2-dependent p53 degradation, through Mdm2-p14ARF complex formation (Zhang et al., 1998). Thus, in response to genotoxic stress induced by gamma-radiation, p14ARF binds directly to Mdm2, leading to an inhibition of Mdm2-mediated p53 ubiquitination and degradation, which increases p53 levels. These events are coupled with downstream signaling pathways, promoting behaviors such as cell cycle arrest, DNA repair, senescence, or apoptosis induction (Zhang et al., 1998; Sionov and Haupt, 1999; Parisi et al., 2002).
The present paper uses a continuous-time approach in a deterministic model of the p53-Mdm2 network regulated by p14ARF under gamma-radiation response (Leenders and Tuszynski, 2013). Pinning control (Li et al., 2004; Chen, 2017) is applied to regulate feedback-loop caused by Mdm2 overexpression stimuli as carcinogenic initial condition (Oliner et al., 1992; Nilbert et al., 1994; Dei Tos et al., 2000; Rayburn et al., 2005), considering no influence of potential mutations. Two desired behaviors are expected; the first one, restoration of an oscillatory pattern, and the second one, the achievement of an increased p53 level expression. p14ARF as pinned node is selected according to the virtual leader methodology (Ren and Beard, 2008; Lewis et al., 2013).
The novelty of the present paper is summarized as follows:
1. The p53 and Mdm2 proteins are controlled by regulating the p14ARF level, based on sensitivity analysis (Dickinson and Gelinas, 1976; Hamby, 1994) as done below.
2. Spanning tree (Ren and Beard, 2008; Lewis et al., 2013) of the p53-Mdm2 network regulated by p14ARF is obtained in order to select the pinned nodes, which are determined based on the virtual leader methodology (Ren and Beard, 2008; Lewis et al., 2013).
3. Pinning control is introduced using a control systems approach where the control dynamics is solved in conjunction with the systems dynamics.
4. The proposed pinning control scheme does not require to apply control inputs to all nodes; in this paper, only one node (the pinned one), ensures oscillatory pattern under gamma-radiation and increased expression of p53 levels for the p53-Mdm2 network.
2. Methods
2.1. Model Description
The p53-Mdm2 network is key for determining cell behavior in response to cellular stress, such as DNA damage induced by gamma-radiation (Strigari et al., 2014; Hage-Sleiman et al., 2017), which can start a program of p53-dependent consequences such as cell cycle arrest, DNA repair, senescence, or apoptosis induction (Wagner et al., 2005; Geva-Zatorsky et al., 2006; Sykes et al., 2006; Wee et al., 2009, 2012; Hafner et al., 2017). In Figure 1, the interaction network between p53, Mdm2, and p14ARF is provided for an individual cell model in two cell compartments: nucleus and cytoplasm. This model includes DNA damage induced by gamma-radiation, which generates the p53 activation and the transactivation of both TP53 and MDM2 (among other transactivated genes, which are not considered in this model). Initially, the translocation process of mRNAs to the cytoplasm and its subsequent translation into proteins takes place; afterward, proteins are transported back to the nucleus. While p53 remains at high levels, Mdm2nuclear reduces its concentration levels, and vice versa, thus, producing an oscillatory pattern. Mdm2cytoplasmic moves to the nucleus at a constant rate, ignoring all other possible behaviors. The production and degradation rates of p14ARF remain constant (Leenders and Tuszynski, 2013).
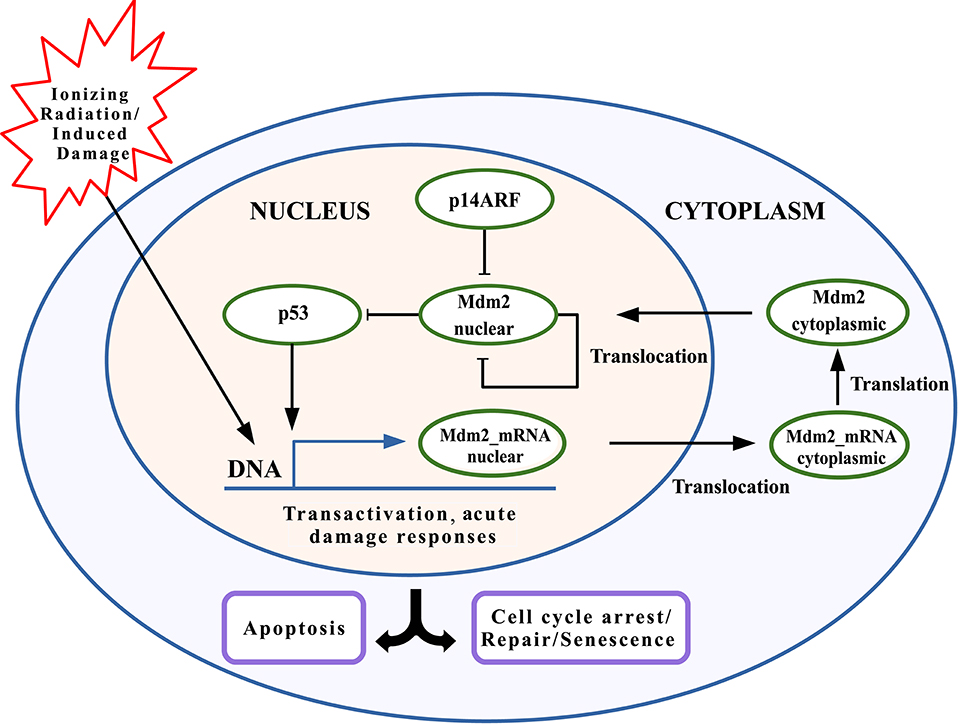
Figure 1. Schematic model of p53 network including Mdm2nuclear inhibition by p14ARF. p53 stabilization leads to transcriptional activity, inducing Mdm2mRNA expression. Mdm2cytoplasmic is transported to the nucleus. p14ARF is also upregulated under genotoxic stress, where binds to Mdm2nuclear and causing its suppression. p53 downstreaming possible responses include cell cycle arrest, DNA repair, senescence, and/or apoptosis.
Following assumptions for the p53-Mdm2 network regulated by p14ARF are presented:
1. The p53-Mdm2 network is one of the most explored biological mechanisms that exist, which provides adequate information.
2. The biological context requires different scenarios dependent on p53 and failure in the network of p53-Mdm2-p14ARF, which can be represented in equations for simulation output desired reference that have a biological explanation, such as regulation mechanisms in gene expression.
3. The biological responses of p53 that we are interested in exploring are related to the ability to generate cell cycle arrest, DNA repair, senescence, and/or apoptosis, especially the last one since it has a key role in the tumor suppressor response.
4. The model assumes the response to an ionizing radiation stimulus with p53-dependent responses, which presents an oscillatory pattern due to variation between p53 and its inhibitor Mdm2. The main objective is to simulate the p53 suppressed response when Mdm2 is overexpressed, which has been documented in several types of tumors.
2.2. Mathematical Description
Taken from Leenders and Tuszynski (2013), based on the principle of mass-action and the saturable transcription kinetics, the p53-Mdm2 network regulated by p14ARF without control action is mathematically described as follows:
The deterministic model (1–6) includes: p53 production and degradation (Equation 1), Mdm2_mRNAnuclear basal transcription (p53-dependent and Mdm2-independent production) in Equation (2); Mdm2_mRNAcytoplasmic transport from nucleus to cytoplasm (Equation 3), Mdm2_mRNAcytoplasmic translation rate and Mdm2cytoplasmic protein transport to nucleus (Equation 4), Mdm2cytoplasmic decay through Mdm2nuclear-p14ARF complex, which removes Mdm2 and stops Mdm2nuclear ubiquitination rate (Equation 5), p14ARF production and p14ARF decay in nucleus compartment (Equation 6). The parameter values used are presented in Table 1.
2.2.1. Model Characteristics of p53-Mdm2 Network Regulated by p14ARF
• The model (1–6) is based on response to gamma radiation in individual MCF-7 cells. This model does not represent all single cell line type responses to gamma radiation.
• It is important to emphasize that the model employed (1–6) describes the core components of the p53 network and are relevant to determine p53 dynamics in response to gamma radiation-induced DNA damage. Mathematical model considers several parameters with non-linear behaviors such as molecule production, degradation, the dissociation constant in the promoter region, translocation of network components, complex formation rate, and translation rate. Other genes/molecules regulations are ignored, gene mutations are not considered, and constant molecule concentrations in the cell are assumed.
• Note that this model includes both experimentally measured and unknown parameters, which are selected manually in order to fit the oscillatory behavior observed for one cell model, as reported in Leenders and Tuszynski (2013).
• Due to the scarcity of parameters used in equations, implications in future researches will involve eliciting responses to stimuli such as gamma radiation, also in other stimuli with a p53-dependent and independent responses, in single cells and multiple ones, including detailed characterizations about genome integrity so that a mathematical model reaches excellent precision.
2.3. Pinning Control Methodology
Consider a general network consisting of N nodes with nonlinear couplings, where each node is a scalar nonlinear dynamical system, which represents genes, concentrations of RNAs and proteins, given by
where xi ∈ ℝ is the state of node i, for i = 1, 2, …, N; fi:ℝ↦ℝ represents the self-dynamics of node i related to the degradation process of RNA and proteins, and so on; is the nonlinear coupling function between nodes, associated with the changes of xi due to transcription, translation, repression, activation, or other interaction processes, and N represents the all network nodes.
The control objective is that (7) tracks a desired output trajectory, given by
To achieve this objective, local feedback controllers are applied to a reduced number of network nodes, according to the pinning control methodology (Wang and Chen, 2002; Li et al., 2004; Chen, 2017). This methodology is composed of two parts: the first one, pinned nodes l are selected to apply control actions as in (8), where 1 ≤ l ≤ N, and l can be as small as one. The second one is the remained network nodes (N − l) without control action as in (9). Thus, the controlled network can be written as
where gi:ℝ↦ℝ is a nonlinear function of the node state i, for i = 1, 2, …, l and ui denotes the control on the node i ∈ l.
In the present paper, ui in (8), is proposed as a local positive discontinuous feedback control law, described by
where Ki is a positive control gain selected by the designer, ei is the tracking error between the desired output trajectory [yr(t)] and the controlled state (xi), is given by
with being a proposed auxiliary variable to reject chattering effect caused by sign(·) (signum function extracts the sign of a real number) (), and Si a signal filter given by
where αi and ωi are positive gains to be selected.
2.4. p14ARF as Pinned Node
To select the pinned nodes, the virtual leader methodology presented in Ren and Beard (2008) and Lewis et al. (2013) is used. The methodology consists on analyzing the interactions between proteins presented in Figure 1 using the mathematical model (1–6). The nodes that affect directly or indirectly the dynamical behavior everyone else, are candidates as pinned nodes. In this sense, the spanning tree of the p53-Mdm2 network regulated by p14ARF is developed, as in Figure 2; based on this analysis, p14ARF is the adequate biological selection as the pinned node.
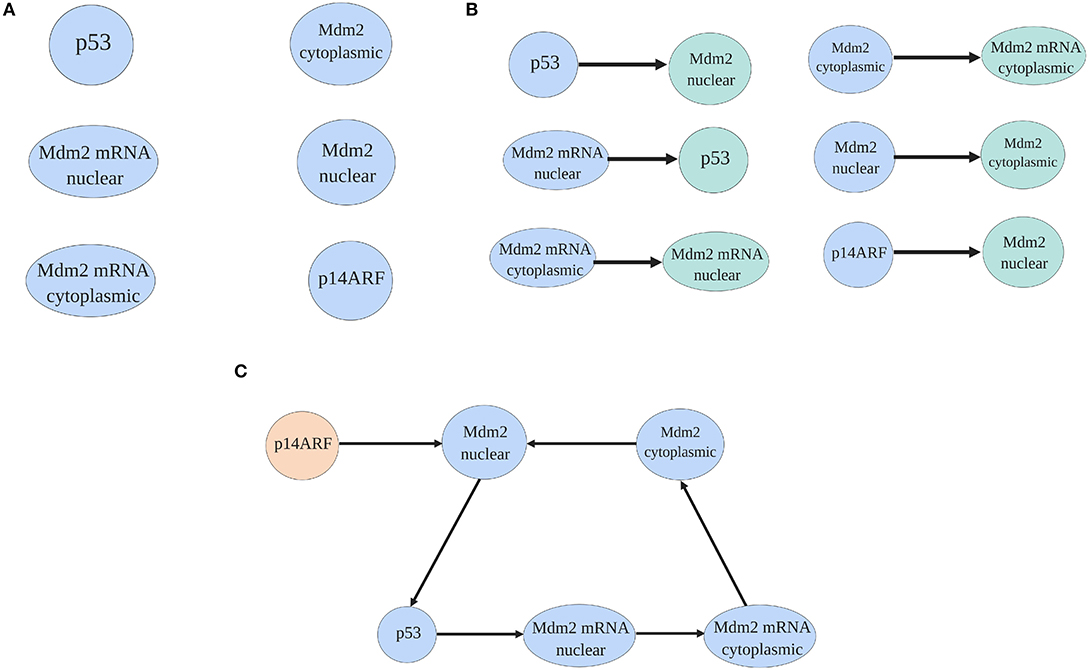
Figure 2. Spanning tree process of the p53-Mdm2 network regulated by p14ARF. In (A) proteins and mRNA of the network are presented. In (B) based on the mathematical model (1–6) and doing a biological analysis, proteins, and mRNA are illustrated with their dependent molecule. Finally, in (C) the spanning tree where p14ARF do not have direct dependence (virtual leader) from other protein/mRNA is presented.
From Equation (6), the differential equation for p14ARF (pinned node) is defined by three cellular processes, as follows
In order to control the p53-Mdm2 network, it is necessary to increase the p14ARF concentration levels to regulated Mdm2nuclear production. As can be seen degradation process and Mdm2nuclear − p14ARF complex formation have a negative sign; while, the production process has a positive sign. Due to this fact, we propose to modify the p14ARF production (Ka) process added the control law (10).
Thus, considering Equations (8) and (9), the p53-Mdm2 network regulated by p14ARF with control action is mathematically described as follows:
The p53-Mdm2 network regulated by p14ARF without control action (1–6) and with control action (13–18) are simulated using Matlab/Simulink and the fourth-order Runge-Kutta integration method with a fixed step size of 1 × 10−3.
3. Results
3.1. Behaviors of the p53-Mdm2 Network Regulated by p14ARF Without Control Action
Three different behaviors of the p53-Mdm2 network without control actions are presented in Figure 3.
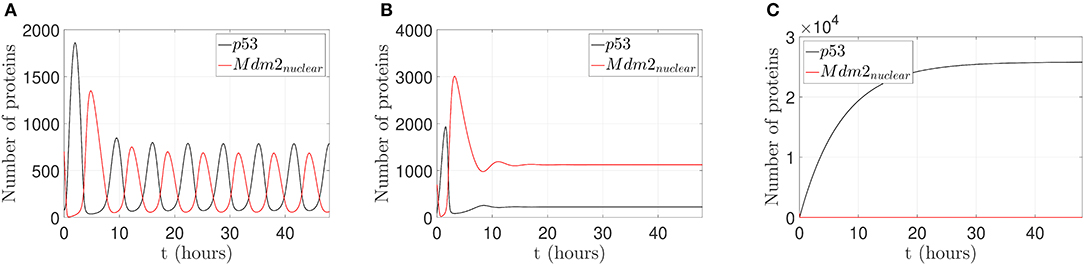
Figure 3. Behaviors of the p53-Mdm2 network regulated by p14ARF without control action. (A) p53-Mdm2nuclear oscillatory pattern under gamma-radiation within a lapse of 6.64 h. (B) Mdm2nuclear overexpression and p53 downregulation with /s and /s; once Mdm2nuclear is overexpressed, p53 is inhibited. It can be conjectured that Mdm2nuclear deregulation will lead to oncogenic behavior through p53 suppression. (C) Increased expression of p53 levels response under gamma-radiation induction with ka = 5 proteins/s and /s. It can be conjectured that p53 upregulation will lead to cell cycle arrest, DNA repair, senescence, and/or apoptotic response.
As displayed in Figure 3A, the p53-Mdm2 network presents an oscillatory pattern under gamma-radiation, for a lapse of 48 h, using the parameter values shown in Table 1. This response is due to post-translational modifications of p53 and the negative interactive loop of Mdm2-mediated ubiquitination, according to Ciliberto et al. (2005) and Geva-Zatorsky et al. (2006); this pattern has not been observed for all cell types and requires wild-type genes (Lahav et al., 2004; Leenders and Tuszynski, 2013).
Figure 3B illustrates that p53-Mdm2 dependent affinity is altered, producing a Mdm2nuclear overexpression when the parameters k1 and k2 are set to values five and ten times larger than the original ones, respectively. This behavior is reported in a variety of human soft tissue tumors and in hematological malignancies, as discussed in Oliner et al. (1992), Nilbert et al. (1994), Dei Tos et al. (2000), Bond et al. (2004), and Rayburn et al. (2005). In human tumors, Mdm2 overexpression can inhibit p53 normal regulatory activities and induce a loss of growth-inhibitory signals in cytostatic and apoptotic responses, which may favor a carcinogenic process.
Figure 3C displays an increased expression of p53 levels when the parameters Ka and drc are set to values ten and one hundred times larger than the original ones, respectively. This p53 dynamical behavior is related to downstream genes involved in signaling pathway, which can produce cell cycle arrest, DNA repair, senescence, and/or apoptotic response (El-Deiry, 1998; Hsing et al., 2000; Khan et al., 2004; Arya et al., 2010; Purvis et al., 2012).
3.2. Behaviors of the p53-Mdm2 Network With Nutlin-3
Several molecular inhibitors of p53-Mdm2 interaction have been proposed, including a small molecule called Nutlin-3 as in Vassilev et al. (2004). Due to Mdm2 deregulation has been reported in various tumor types, Nutlin-3 has been an option to block the p53-binding site of MDM2 competitively and induce the upregulation and activation of p53 pathway (Yee-Lin et al., 2018). These findings motivated us to observe the possible effect of the external stimulus of Nutlin-3 on the oscillatory pattern behavior in the p53-Mdm2 network regulated by p14ARF. If p53 stabilization is achieved and p53 degradation is avoided, as reported for Nutlin-3 in tumors, p53 can accomplish antiproliferative effects.
In this sense, a simulation to reduce p53-Mdm2 interaction and p53 degradation in the presence of Nutlin-3 is presented in Figure 4. To achieve this behavior, k1, which represents Mdm2-dependent p53 degradation, takes values between 25, 50, 75, and 90% less from the original value. It is possible to observe that simulating the effect of Nutlin-3, liberates p53 from the interaction and inhibition by Mdm2 in the network, allowing to desynchronize p53 and MDM2 oscillatory behavior, reaching a stable high concentration when the inhibition by Nutlin-3 is strong.
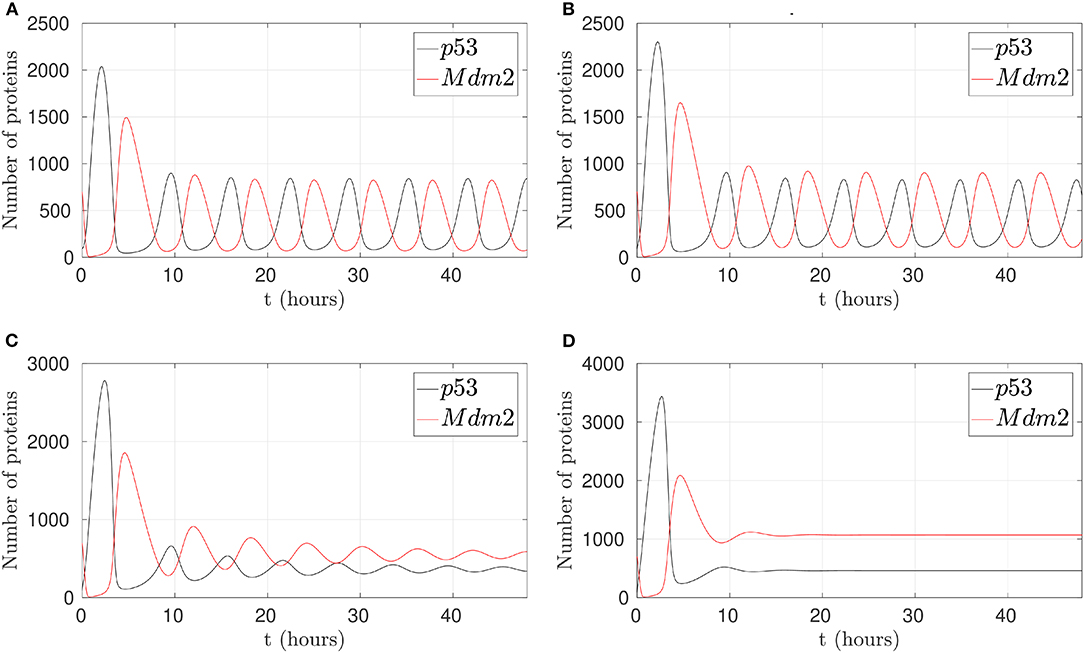
Figure 4. Behaviors of the p53-Mdm2 network regulated by p14ARF under Nutlin-3 effect. (A) With 25% activity in k1. (B) With 50% activity in k1. (C) With 75% activity in k1. (D) With 90% activity in k1. (C,D) Shows desynchronized p53-Mdm2 interaction.
3.3. Sensitivity Analysis for p14ARF Production (Ka)
This analysis is done on the basis of p14ARF production (Ka) value variation effects on p53 and Mdm2nuclear respectively as can be seen in Figure 5. Sensitivity analysis (Dickinson and Gelinas, 1976; Hamby, 1994) determines the Ka values for which the network can not achieve desired behaviors (0–1.5 proteins/s); the Ka value to reach p53-Mdm2nuclear oscillatory pattern (1.6–9.5 proteins/s), and the Ka value to generate an increased expression of p53 with Mdm2nuclear downregulation (9.6–50 proteins/s) are determined from Figure 5.
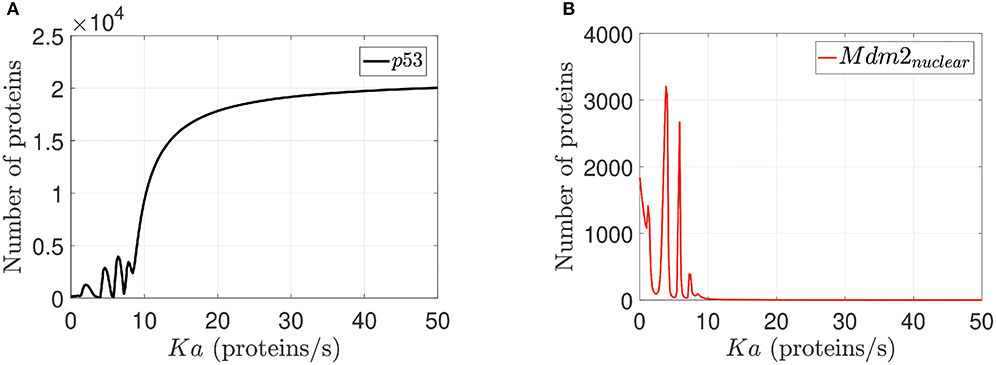
Figure 5. Sensitivity analysis for Ka. In (A) p53 response to Ka variations is illustrated. In (B) Mdm2nuclear response to Ka variations is presented. For both cases Ka varies between 0 to 50 proteins/s for all network proteins, but we select p53 and Mdm2nuclear because they are the output desired in the network.
3.4. Behaviors of the p53-Mdm2 Network Regulated by p14ARF With Control Action
To illustrate the p53 and Mdm2nuclear behavior under pinning control actions, two cases are considered: (1) to restore an oscillatory pattern under gamma-radiation, and (2) to achieve an increased expression of p53 level. For a 24 h lapse, the network runs without any control action and presents overexpressed Mdm2-mediated p53 degradation as carcinogenic initial behavior for both cases (Oliner et al., 1992; Nilbert et al., 1994; Dei Tos et al., 2000; Bond et al., 2004; Rayburn et al., 2005), which is displayed in Figure 3B above.
3.4.1. Case 1: Restoration of an Oscillatory Pattern Under Gamma-Radiation
For the first case, by considering p14ARF production (kau1) in a range between 0 and 1.5 proteins/s, the proposed controller is turned on after the 24 h initial lapse; however, the network can not achieves the oscillatory pattern as can be seen in Figure 6A. Due to the low value of kau1, the pinning control technique cannot achieve the desired behavior. Otherwise, with (kau1) in a range between 1.6 and 9.5 proteins/s, the proposed controller forces the network to gradually track the oscillatory pattern as can be seen in Figure 6B.
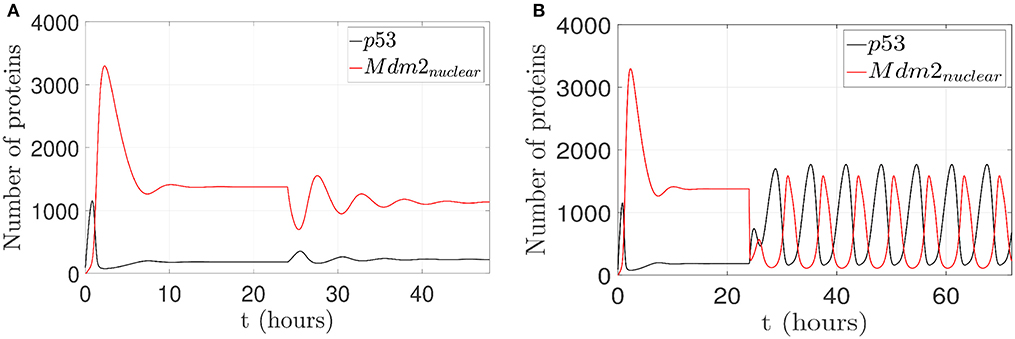
Figure 6. Proposed scenario simulations to achieve restoration of an oscillatory pattern under gamma-radiation in the p53-Mdm2 network regulated by p14ARF. For both tests is possible to observe overexpressed Mdm2-mediated p53 degradation as carcinogenic initial behavior at first 24 h. In (A), control action with (kau1) in a range between 0 and 1.5 proteins/s, the oscillatory pattern under gamma-radiation is not achieved (24–48 h) and likewise in (B) oscillatory pattern under gamma-radiation under pinning control (24–72 h) with (kau1) in a range between 1.6 and 9.5 proteins/s is achieved.
3.4.2. Case 2: Achievement of a p53 Level Increased Expression
For the second case, by considering p14ARF production (kau1) in a range between 0 and 1.5 proteins/s, the proposed controller is turned on; however, the network can not achieves increased expression of p53 as can be seen in Figure 7A. Due to the low value of kau1, the pinning control technique cannot achieve the desired behavior. Otherwise, with (kau1) in a range between 9.6 and 50 proteins/s, the proposed controller again is turned on, and the system gradually tracks the increased expression of p53 levels as can be seen in Figure 7B.
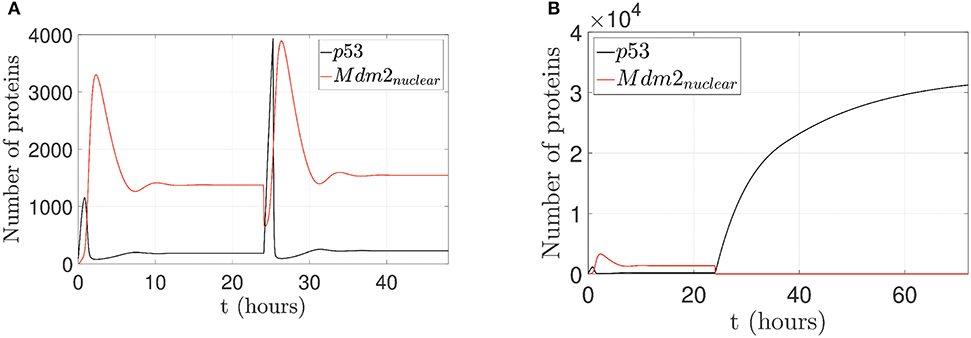
Figure 7. Proposed scenario simulations to achieve p53 level increased expression in the p53-Mdm2 network regulated by p14ARF. For both tests is possible to observe overexpressed Mdm2-mediated p53 degradation as carcinogenic initial behavior at first 24 h. In (A), control action with (kau1) in a range between 0 and 1.5 proteins/s, increased expression of p53 response is not achieved (24–48 h) and likewise in (B) increased expression of p53 response under pinning control (24–72 h) with (kau1) in a range between 9.6 and 50 proteins/s is achieved.
For both cases, the control law (u1(t) ∈ ℝ) is applied to p14ARF node as in Equation (14). The respective control actions are displayed in Figure 8A for the first case and Figure 8B for the second case. From the above results, it can be clearly seen that the pinning controller achieves regulation successfully for the p53-Mdm2 network.
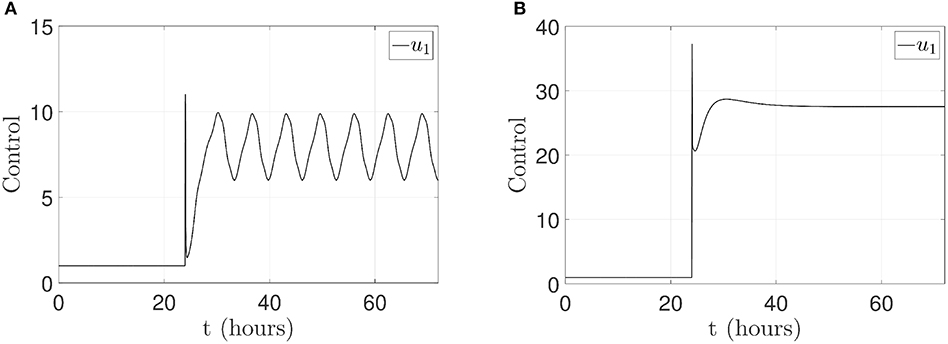
Figure 8. Control signal u1(t) applied in p14ARF. (A) Case 1: control action to achieve an oscillatory pattern under gamma-radiation. (B) Case 2: control action to achieve an increased expression of p53 response.
4. Discussion
In biological systems, gene regulatory networks, having complex interactions, present important challenges for the application of control strategies. A mixture of mechanisms such as gene expression patterns, post-translational modifications, translocation, components degradation, and the specific changes of internal and external signals of the cell, generate nonlinear behavior. Hence, to illustrate the applicability of complex network control, we present two cases for a deterministic network model corresponding to tumor suppressor p53, Mdm2, and p14ARF.
4.1. Behaviors Induced Without Control Action
4.1.1. p53-Mdm2nuclear Oscillatory Pattern
Levels of p53 and Mdm2nuclear proteins present oscillatory behavior, caused by pulses resulting from p53 activation, p53-dependent transactivation, Mdm2 production, Mdm2nuclear sequestration by p14ARF, and Mdm2-mediated ubiquitination. This process allows the cell to respond to double-strand breaks (DSBs) in DNA induced by gamma radiation, which correlates with the number of p53 pulses in individual cells (Lahav et al., 2004; Geva-Zatorsky et al., 2006). For the model used in this paper, there are regulatory factors which have not been considered, as the interaction of other potentially relevant genes transactivated by p53 (nearly 100 genes in pathways such as cell cycle arrest, DNA repair, senescence, and apoptosis; Riley et al., 2008). One of the simpler explanations for the p53 oscillatory pattern is due to repeated activation of ATM (Ataxia Telangiectasia Mutant), which dissociates the p53-Mdm2 complex and stabilizes an increase in p53 levels. These changes are driven by persistent DNA damage induced by radiation (Lahav et al., 2004; Geva-Zatorsky et al., 2006). Therefore, recovering the normal pattern response to DNA damage by the p53-Mdm2 network is fundamental for tumor suppression.
4.1.2. Mdm2nuclear Overexpression and p53 Downregulation
There are gene abnormalities in tumors, carcinogenesis driven by viral infections, and other mechanisms that contribute to inactivate p53 functions and its signaling outcomes (Scheffner et al., 1990; Camus et al., 2003; Rayburn et al., 2005). For example, Mdm2 overexpression leads to p53 downregulation, contributing to losses of tumor suppressor activity. Mdm2 overexpressed is a hallmark in several types of cancer (Nilbert et al., 1994; Dei Tos et al., 2000; Rayburn et al., 2005). As reviewed in Rayburn et al. (2005), Mdm2 is overexpressed in liposarcomas, osteosarcomas, testicular germ cell tumors, embryonic carcinomas, brain tumors (including glioblastomas and astrocytomas), hematological malignancies, bladder cancer, breast cancer, colorectal cancer among others. In this sense, the number of Mdm2 abnormalities is highly variable. Moreover, not all samples from the same type of tumor, show Mdm2 overexpression. Bond et al. (2004) reported other mechanisms that promote Mdm2 overexpression, where a single nucleotide polymorphism (SNP309) in the MDM2 promoter, increases the affinity of the transcriptional activator Sp1, resulting in higher levels of Mdm2 mRNA and Mdm2 translation rate. This behavior illustrates p53 downregulation resulting in a decreased response to DNA damaging agents and acceleration of tumorigenesis.
4.1.3. Increased Expression of p53 Levels
The stabilization and accumulation of p53 levels are part of the DNA damage response to maintain genome integrity. One of the possible response outputs of a fully functional p53 pathway is the induction of apoptosis generated by DNA damage. For apoptosis induction by radiation in certain tissues, a cascade of signaling is generated through pro-apoptotic proteins, such as response mediated by ATM protein (Bakkenist and Kastan, 2003). ATM leads to p53 stabilization, modifying the interaction capabilities with Mdm2 (El-Deiry, 1998). With the activation of p53, its degradation is limited, and p53 levels increase (Oliner et al., 1993). Downstream to ATM/p53 activation, an apoptosis program is carried out by a set of proteins, such as Bim, Puma, Bid, Bmf, Bad, Bik, Noxa, and Hrk, whereby Puma and Noxa can be directly regulated with p53 overexpression by gamma-radiation (Villunger et al., 2003; Chen et al., 2005). This set of proteins can bind and block survival proteins such as Bcl-2, which release death effectors like Bax and Bak. These effectors can lead to a change in the permeability of the outer mitochondria membrane. Furthermore, they can participate in the cell dismantling coupled with caspases (Green and Kroemer, 2004). There are other possible outcomes for the p53 activation pathway and p53 independent responses to DNA damage by gamma-radiation, which can lead the cell to cell cycle arrest, initiate DNA repair, or perform senescence. Experimental evidence indicates that the cellular level of p53 can dictate the response of the cell, such that lower levels of p53 result in arrest, whereas higher level results in apoptosis (Chen et al., 1996; Purvis et al., 2012).
4.1.4. p53-Mdm2 Network With Nutlin-3
Just as p14ARF can stabilize p53 by antagonizing Mdm2 effects (Weber et al., 1999), if we consider molecules or treatments that can function as Mdm2 inhibitors, the small molecule Nutlin-3, is a compound described that binds in the p53-binding pocket within Mdm2, inhibiting its interaction with p53, and preventing p53 tagging for proteasome-mediated degradation (Vassilev et al., 2004; Yee-Lin et al., 2018).
Nutlin-3 is considered cytotoxic in specific wild-type p53 cancer cells (Vassilev et al., 2004; Arya et al., 2010; Yee-Lin et al., 2018), and therefore, p53 executes p53-dependent genes transactivation. Unlike in p53 mutated cell lines, transactivation is defective, and induced Mdm2 expression is impaired (Kamijo et al., 1998). It is essential to consider TP53 mutational status, other p53-dependent responses, and the Nutlin 3 activity in a dose and time-dependent manner (Arya et al., 2010), to predict the effect of Nutlin 3 in Mdm2 binding interactions.
4.2. Behaviors Induced by the Pinning Control Technique
4.2.1. Case 1: Restoration of an Oscillatory Pattern Under Gamma-Radiation
The oscillatory pattern behavior (Geva-Zatorsky et al., 2006; Wee et al., 2009; Batchelor et al., 2011) induced by means of the pinning control technique is illustrated in Figure 6, with the purpose of restoring normal network behavior in presence of oncogenic overexpressed Mdm2; this overexpression avoids normal regulatory activities due to a suppressed wild-type p53. The pinning control technique (10) is located at the production of p14ARF node with Ki = 100, which allows to regenerate an oscillatory pattern and guarantees that p14ARF production kau1 achieves a range between 1.6 and 9.5 proteins/s. Enhanced p14ARF production induces a decrease in Mdm2nuclear which in turn increased p53 levels. This approach can help to analyze multiple interaction mechanisms, to induce different cell reprogramming responses. Therefore, the proposed approach motivates future researches on the interdependencies of cellular networks and new ways for treatment designs in tumor suppressor networks.
4.2.2. Case 2: Achievement of an Increased p53 Level Expression
The increased expression of p53 levels by means of the pinning control technique is achieved as shown in Figure 7. Such technique generates the desired behavior of p53 progressive accumulation, assuming that this behavior has a post-translational activation mediated by ATM, which could generate the activation of downstream proteins, contributing to the activation of apoptosis or cell cycle arrest (El-Deiry, 1998; Wagner et al., 2005; Geva-Zatorsky et al., 2006; Wee et al., 2009). The pinning control technique (10) is located at the production of p14ARF node with Ki = 5, which yields an increased p53 level expression and guarantees that p14ARF production kau1 achieves a range between 9.6 − 50proteins/s. p14ARF is moved from the nucleolus to nucleoplasm in response to DNA damage, where Mdm2nuclear-p14ARF complex promotes p53 tumor suppressor activity. For this case, it is possible to generate p53 accumulation, which is assumed to be activated by a mechanism linked to post-radiation activation of the ATM protein and p53-dependent induction of downstream proteins (Bakkenist and Kastan, 2003; Villunger et al., 2003; Pauklin et al., 2005). With the proposed pinning control law, it is possible to produce scenarios with different physiological or pathological responses of the p53-Mdm2 network.
For the two cases discussed above, the proposed pinning control strategy for the p53-Mdm2 network dynamics is applied on the p14ARF node based on sensitivity analysis as follows: In the first case, oscillatory pattern activity is achieved; in the second case p53 increased expression and accumulation are obtained. By means of the sensitivity analysis in Ka with respect to p53 and Mdm2nuclear, it can be established that with Ka low values, the network does not reach the desired behavior. However, for the adequate Ka values mentioned above, the network recovers its oscillatory pattern behavior, or an increase in p14ARF production leads causing a consistently increase p53 level. The proposed pinning control strategy as explained suppresses Mdm2 prooncogenic behavior and allows functional recovery of p53 physiological response.
4.2.3. p14ARF as Pinned Node for p53-Mdm2 Network Control
In this section, we explain the importance of p14ARF as pinned node for the p53-Mdm2 network. Pinned node p14ARF regulates p53 by promoting Mdm2 degradation, preventing the Mdm2-mediated p53 degradation (Kamijo et al., 1998; Zhang et al., 1998; Weber et al., 1999). Experimental evidence indicates that p14ARF can even induce apoptosis through the Bax protein independent of p53 (Suzuki et al., 2003) and activate p53 through phosphorylation in oncogenic damage (Ito et al., 2001). It has also been reported that p14ARF helps to regulate the expression of Rad51, a protein involved in DNA repair; such regulation is explained by the activation of the ATM/ATR pathway, which is activated in response to DNA DSBs damage induced by ionizing radiation (Pauklin et al., 2005). In an in vitro approach (Itoshima et al., 2000), esophageal cancer cells were transfected with a plasmid designed to rise ARF expression (exogenous), with the subsequent reduction of endogenous levels of Mdm2 and induced p53 accumulation. Also, Itoshima et al. (2000), observed that mutant form of p53 was also stabilized by ectopic ARF increased expression, consistent with another report where p53 mutated can also be bonded to Mdm2 (Haupt et al., 1997); however, mutant-p53 transcriptional activity is obliterated and the existence of an intact autoregulatory loop between ARF, Mdm2, and p53 is needed to observe full regulatory pathway responses, particularly, a response that leads to apoptosis in cancer cells.
Interestingly, according to a model of overexpression of p14ARF in glioblastoma and astrocytoma cells, p14ARF controls neovascularization, through upregulation of metalloproteinase-3 inhibitor (TIMP3) in a p53-independent signaling pathway, which suppresses angiogenesis (Zerrouqi et al., 2012). Moreover, the upregulation of P14ARF could generate activation or interaction with other factors such as Sp1, c-Jun, AP-1, and JNK that cooperate to prevent tumor-induced microthrombosis through activation of the anticoagulant factor TFPI-2. p14ARF also enhances the transcriptional activity of Sp1, using the interaction of Mdm2 with Sp1. If Mdm2 is inhibited, Sp1 activity can be enhanced and induce tumor suppressor effects independent of p53-mediated signaling, as stated by Zerrouqi et al. (2014).
As future work, it would be essential to generate a new model that includes these and other interactions (disruption of mutant p53 stabilization, p53 post-translational modifications, external inhibitors, or altered proteasome system within the network), which would allow applying these control techniques and other types of analysis to others cell signaling pathways, to computationally model and stir the dynamics of a gene regulatory network to the desired state mainly to reproduce the coordinated fluctuation behavior of core components in p53 network.
Finally, experimental validation for the p53-Mdm2 network regulated by p14ARF as pinned node is not possible to implement due to lack of a biosensor measure the activity in the nucleus and cytoplasm at the cell. Our group is currently developing such a sensor.
Data Availability Statement
The datasets generated for this study are available on request to the corresponding author.
Author Contributions
OS, CV, ES, and GC initiated research and provided knowledge about strategies of control in the methods section. AG-S and OR-J proposed the description model and discussion. EH-V and AA discussed simulations and prepared figures. All authors designed the research, analyzed data, prepared, wrote, and reviewed the paper.
Funding
This work was supported by CONACYT, Mexico, Project 257200 and by the Alfons und Gertrud Kassel-Stiftung.
Conflict of Interest
The authors declare that the research was conducted in the absence of any commercial or financial relationships that could be construed as a potential conflict of interest.
References
Ahmad, J., Bernot, G., Comet, J., Lime, D., and Roux, O. (2006). Hybrid modelling and dynamical analysis of gene regulatory networks with delays. ComPlexUs 3, 231–251. doi: 10.1159/000110010
Akutsu, T., Miyano, S., and Kuhara, S. (1999). Identification of genetic networks from a small number of gene expression patterns under the Boolean network model. Pac. Sympos. Biocomput. 4, 17–28. doi: 10.1142/9789814447300_0003
Arya, A., El-Fert, A., Devling, T., Eccles, R., Aslam, M., Rubbi, C., et al. (2010). Nutlin-3, the small-molecule inhibitor of MDM2, promotes senescence and radiosensitises laryngeal carcinoma cells harbouring wild-type p53. Br. J. Cancer 103:186. doi: 10.1038/sj.bjc.6605739
Ashcroft, M., Taya, Y., and Vousden, K. (2000). Stress signals utilize multiple pathways to stabilize p53. Mol. Cell. Biol. 20, 3224–3233. doi: 10.1128/MCB.20.9.3224-3233.2000
Bakkenist, C., and Kastan, M. (2003). DNA damage activates ATM through intermolecular autophosphorylation and dimer dissociation. Nature 421:499. doi: 10.1038/nature01368
Batchelor, E., Loewer, A., Mock, C., and Lahav, G. (2011). Stimulus-dependent dynamics of p53 in single cells. Mol. Syst. Biol. 7:488. doi: 10.1038/msb.2011.20
Bolouri, H., and Davidson, E. (2002). Modeling transcriptional regulatory networks. BioEssays 24, 1118–1129. doi: 10.1002/bies.10189
Bond, G., Hu, W., Bond, E., Robins, H., Lutzker, S., Arva, N., et al. (2004). A single nucleotide polymorphism in the MDM2 promoter attenuates the p53 tumor suppressor pathway and accelerates tumor formation in humans. Cell 119, 591–602. doi: 10.1016/j.cell.2004.11.022
Burbano, D., Russo, G., and Di Bernardo, M. (2019). Pinning controllability of complex network systems with noise. IEEE Trans. Control Netw. Syst. 6, 874–883. doi: 10.1109/TCNS.2018.2880300
Cai, L., Friedman, N., and Xie, X. (2006). Stochastic protein expression in individual cells at the single molecule level. Nature 440:358. doi: 10.1038/nature04599
Cai, X., and Yuan, Z. (2009). Stochastic modeling and simulation of the p53-MDM2/MDMX loop. J. Comput. Biol. 16, 917–933. doi: 10.1089/cmb.2008.0231
Camus, S., Higgins, M., Lane, D., and Lain, S. (2003). Differences in the ubiquitination of p53 by Mdm2 and the HPV protein E6. FEBS Lett. 536, 220–224. doi: 10.1016/S0014-5793(03)00054-1
Cao, J, Qi, X, and Zhao, H. (2012). “Modeling gene regulation networks using ordinary differential equations,” in Next Generation Microarray Bioinformatics. Methods in Molecular Biology (Methods and Protocols), Vol. 802, eds J. Wang, A. Tan and T. Tian (Humana Press) 185–197. doi: 10.1007/978-1-61779-400-1_12
Chaouiya, C. (2007). Petri net modelling of biological networks. Brief. Bioinformatics 8, 210–219. doi: 10.1093/bib/bbm029
Chen, G. (2017). Pinning control and controllability of complex dynamical networks. Int. J. Autom. Comput. 14, 1–9. doi: 10.1007/s11633-016-1052-9
Chen, H., Liang, J., and Wang, Z. (2016). Pinning controllability of autonomous Boolean control networks. Sci. China Inform. Sci. 59:070107. doi: 10.1007/s11432-016-5579-8
Chen, L., Willis, S., Wei, A., Smith, B., Fletcher, J., Hinds, M., et al. (2005). Differential targeting of prosurvival Bcl-2 proteins by their BH3-only ligands allows complementary apoptotic function. Mol. Cell 17, 393–403. doi: 10.1016/j.molcel.2004.12.030
Chen, T., He, H., and Church, G. (1999). “Modeling gene expression with differential equations,” in Biocomputing'99 (Mauna Lani, HI), 29–40. doi: 10.1142/9789814447300_0004
Chen, X., Ko, L., Jayaraman, L., and Prives, C. (1996). p53 levels, functional domains, and DNA damage determine the extent of the apoptotic response of tumor cells. Genes Dev. 10, 2438–2451. doi: 10.1101/gad.10.19.2438
Ciliberto, A., Novák, B., and Tyson, J. (2005). Steady states and oscillations in the p53/Mdm2 network. Cell Cycle 4, 488–493. doi: 10.4161/cc.4.3.1548
De Jong, H. (2002). Modeling and simulation of genetic regulatory systems: a literature review. J. Comput. Biol. 9, 67–103. doi: 10.1089/10665270252833208
Dei Tos, A., Doglioni, C., Piccinin, S., Sciot, R., Furlanetto, A., Boiocchi, M., et al. (2000). Coordinated expression and amplification of the MDM2, CDK4, and HMGI-C genes in atypical lipomatous tumours. J. Pathol. 190, 531–536. doi: 10.1002/(SICI)1096-9896(200004)190:5<531::AID-PATH579>3.0.CO;2-W
Dickinson, R., and Gelinas, R. (1976). Sensitivity analysis of ordinary differential equation systems–a direct method. J. Comput. Phys. 21, 123–143. doi: 10.1016/0021-9991(76)90007-3
El-Deiry, W. (1998). Regulation of p53 downstream genes. Semin. Cancer Biol. 8, 345–357. doi: 10.1006/scbi.1998.0097
Elf, J., Li, G., and Xie, X. (2007). Probing transcription factor dynamics at the single-molecule level in a living cell. Science 316, 1191–1194. doi: 10.1126/science.1141967
Friedman, N., Linial, M., Nachman, I., and Pe'er, D. (2000). Using Bayesian networks to analyze expression data. J. Comput. Biol. 7, 601–620. doi: 10.1089/106652700750050961
Fromentin, J., Eveillard, D., and Roux, O. (2010). Hybrid modeling of biological networks: mixing temporal and qualitative biological properties. BMC Syst. Biol. 4:79. doi: 10.1186/1752-0509-4-79
Gao, Z., Chen, X., and Başar, T. (2017). Controllability of conjunctive Boolean networks with application to gene regulation. IEEE Trans. Control Netw. Syst. 5, 770–781. doi: 10.1109/TCNS.2017.2746345
Geva-Zatorsky, N., Rosenfeld, N., Itzkovitz, S., Milo, R., Sigal, A., Dekel, E., et al. (2006). Oscillations and variability in the p53 system. Mol. Syst. Biol. 2, 1–13. doi: 10.1038/msb4100068
Green, D., and Kroemer, G. (2004). The pathophysiology of mitochondrial cell death. Science 305, 626–629. doi: 10.1126/science.1099320
Hafner, A., Stewart-Ornstein, J., Purvis, J., Forrester, W., Bulyk, M., and Lahav, G. (2017). p53 pulses lead to distinct patterns of gene expression albeit similar DNA-binding dynamics. Nat. Struct. Mol. Biol. 24:840. doi: 10.1038/nsmb.3452
Hage-Sleiman, R., Bahmad, H., Kobeissy, H., Dakdouk, Z., Kobeissy, F., and Dbaibo, G. (2017). Genomic alterations during p53-dependent apoptosis induced by γ-irradiation of Molt-4 leukemia cells. PLoS ONE 12:e0190221. doi: 10.1371/journal.pone.0190221
Hamby, D. (1994). A review of techniques for parameter sensitivity analysis of environmental models. Environ. Monitor. Assess. 32, 135–154. doi: 10.1007/BF00547132
Haupt, Y., Maya, R., Kazaz, A., and Oren, M. (1997). Mdm2 promotes the rapid degradation of p53. Nature 387, 296–299. doi: 10.1038/387296a0
Hsing, A., Faller, D., and Vaziri, C. (2000). DNA-damaging aryl hydrocarbons induce Mdm2 expression via p53-independent post-transcriptional mechanisms. J. Biol. Chem. 275, 26024–26031. doi: 10.1074/jbc.M002455200
Ito, A., Lai, C., Zhao, X., Saito, S., Hamilton, M., Appella, E., et al. (2001). p300/CBP-mediated p53 acetylation is commonly induced by p53-activating agents and inhibited by MDM2. EMBO J. 20, 1331–1340. doi: 10.1093/emboj/20.6.1331
Itoshima, T., Fujiwara, T., Waku, T., Shao, J., Kataoka, M., Yarbrough, W., et al. (2000). Induction of apoptosis in human esophageal cancer cells by sequential transfer of the wild-type p53 and E2F-1 genes: involvement of p53 accumulation via ARF-mediated MDM2 down-regulation. Clin. Cancer Res. 6, 285–2859.
Jiao, H., Zhang, L., Shen, Q., Zhu, J., and Shi, P. (2018). Robust gene circuit control design for time-delayed genetic regulatory networks without SUM regulatory logic. IEEE/ACM Trans. Comput. Biol. Bioinform. 15, 2086–2093. doi: 10.1109/TCBB.2018.2825445
Kamijo, T., Weber, J., Zambetti, G., Zindy, F., Roussel, M., and Sherr, C. (1998). Functional and physical interactions of the ARF tumor suppressor with p53 and MDM2. Proc. Natl. Acad. Sci. U.S.A. 95, 8292–8297. doi: 10.1073/pnas.95.14.8292
Karlebach, G., and Shamir, R. (2008). Modelling and analysis of gene regulatory networks. Nat. Rev. Mol. Cell Biol. 9:770. doi: 10.1038/nrm2503
Kauffman, S. (1969). Metabolic stability and epigenesis in randomly constructed genetic nets. J. Theor. Biol. 22, 437–467. doi: 10.1016/0022-5193(69)90015-0
Khan, S., Guevara, C., Fujii, G., and Parry, D. (2004). p14ARF is a component of the p53 response following ionizing irradiation of normal human fibroblasts. Oncogene 23, 6040–6046. doi: 10.1038/sj.onc.1207824
Kikuchi, S., Tominaga, D., Arita, M., Takahashi, K., and Tomita, M. (2003). Dynamic modeling of genetic networks using genetic algorithm and S-system. Bioinformatics 19, 643–650. doi: 10.1093/bioinformatics/btg027
Kuo, M., den Besten, W., Bertwistle, D., Roussel, M., and Sherr, C. (2004). N-terminal polyubiquitination and degradation of the ARF tumor suppressor. Genes Dev. 18, 1862–1874. doi: 10.1101/gad.1213904
Lahav, G., Rosenfeld, N., Sigal, A., Geva-Zatorsky, N., Levine, A., Elowitz, M., et al. (2004). Dynamics of the p53-MDM2 feedback loop in individual cells. Nat. Genet. 36:147. doi: 10.1038/ng1293
Leenders, G., and Tuszynski, J. (2013). Stochastic and deterministic models of cellular p53 regulation. Front. Oncol. 3:64. doi: 10.3389/fonc.2013.00064
Lewis, F., Zhang, H., Hengster-Movric, K., and Das, A. (2013). Cooperative Control of Multi-Agent Systems: Optimal and Adaptive Design Approaches. London: Springer-Verlag. doi: 10.1007/978-1-4471-5574-4
Li, F., Yan, H., and Karimi, H. (2018). Single-input pinning controller design for reachability of Boolean networks. IEEE Trans. Neural Netw. Learn. Syst. 29, 3264–3269. doi: 10.1109/TNNLS.2017.2705109
Li, X., Wang, X., and Chen, G. (2004). Pinning a complex dynamical network to its equilibrium. IEEE Trans. Circ. Syst. I 51, 2074–2087. doi: 10.1109/TCSI.2004.835655
Lin, G., Ao, B., Chen, J., Wang, W., and Di, Z. (2014). Modeling and controlling the two-phase dynamics of the p53 network: a Boolean network approach. New J. Phys. 16:125010. doi: 10.1088/1367-2630/16/12/125010
Liu, Y., and Barabási, A. (2016). Control principles of complex systems. Rev. Modern Phys. 88:035006. doi: 10.1103/RevModPhys.88.035006
Momand, J., Zambetti, G., Olson, D., George, D., and Levine, A. (1992). The mdm-2 oncogene product forms a complex with the p53 protein and inhibits p53-mediated transactivation. Cell 69, 1237–1245. doi: 10.1016/0092-8674(92)90644-R
Mor, A., Suliman, S., Ben-Yishay, R., Yunger, S., Brody, Y., and Shav-Tal, Y. (2010). Dynamics of single mRNP nucleocytoplasmic transport and export through the nuclear pore in living cells. Nat. Cell Biol. 12:543. doi: 10.1038/ncb2056
Nilbert, M., Mitelman, F., Mandahl, N., Rydholm, A., and Willén, H. (1994). MDM2 gene amplification correlates with ring chromosomes in soft tissue tumors. Genes Chromos. Cancer 9, 261–265. doi: 10.1002/gcc.2870090406
Northrup, S., and Erickson, H. (1992). Kinetics of protein-protein association explained by Brownian dynamics computer simulation. Proc. Natl. Acad. Sci. U.S.A. 89, 3338–3342. doi: 10.1073/pnas.89.8.3338
Nowzari, C., Preciado, V., and Pappas, G. (2016). Analysis and control of epidemics: a survey of spreading processes on complex networks. IEEE Control Syst. 36, 26–46. doi: 10.1109/MCS.2015.2495000
Oliner, J., Kinzler, K., Meltzer, P., George, D., and Vogelstein, B. (1992). Amplification of a gene encoding a p53-associated protein in human sarcomas. Nature 358:80. doi: 10.1038/358080a0
Oliner, J., Pietenpol, J., Thiagalingam, S., Gyuris, J., Kinzler, K., and Vogelstein, B. (1993). Oncoprotein MDM2 conceals the activation domain of tumour suppressor p53. Nature 362:857. doi: 10.1038/362857a0
Papatsenko, D., Waghray, A., and Lemischka, I. (2018). Feedback control of pluripotency in embryonic stem cells: signaling, transcription and epigenetics. Stem Cell Res. 29, 180–188. doi: 10.1016/j.scr.2018.02.012
Parisi, T., Pollice, A., Di Cristofano, A., Calabró, V., and La Mantia, G. (2002). Transcriptional regulation of the human tumor suppressor p14arf by E2F1, E2F2, E2F3, and Sp1-like factors. Biochem. Biophys. Res. Commun. 291, 1138–1145. doi: 10.1006/bbrc.2002.6591
Pauklin, S., Kristjuhan, A., Maimets, T., and Jaks, V. (2005). ARF and ATM/ATR cooperate in p53-mediated apoptosis upon oncogenic stress. Biochem. Biophys. Res. Commun. 334, 386–394. doi: 10.1016/j.bbrc.2005.06.097
Purvis, J., Karhohs, K., Mock, C., Batchelor, E., Loewer, A., and Lahav, G. (2012). p53 dynamics control cell fate. Science 336, 1440–1444. doi: 10.1126/science.1218351
Rau, A., Jaffrézic, F., Foulley, J., and Doerge, R. (2010). An empirical Bayesian method for estimating biological networks from temporal microarray data. Stat. Appl. Genet. Mol. Biol. 9, 1–26. doi: 10.2202/1544-6115.1513
Rayburn, E., Zhang, R., He, J., and Wang, H. (2005). MDM2 and human malignancies: expression, clinical pathology, prognostic markers, and implications for chemotherapy. Curr. Cancer Drug Targets 5, 27–41. doi: 10.2174/1568009053332636
Ren, W., and Beard, R. (2008). Distributed Consensus in Multi-Vehicle Cooperative Control. London: Springer-Verlag. doi: 10.1007/978-1-84800-015-5
Riley, T., Sontag, E., Chen, P., and Levine, A. (2008). Transcriptional control of human p53-regulated genes. Nat. Rev. Mol. Cell Biol. 9:402. doi: 10.1038/nrm2395
Scheffner, M., Werness, B., Huibregtse, J., Levine, A., and Howley, P. (1990). The E6 oncoprotein encoded by human papillomavirus types 16 and 18 promotes the degradation of p53. Cell 63, 1129–1136. doi: 10.1016/0092-8674(90)90409-8
Selvin, P., and Ha, T. (2008). Single-Molecule Techniques. Cold Spring Harbor, NY: Cold Spring Harbor Laboratory Press.
Shmulevich, I., Dougherty, E., Kim, S., and Zhang, W. (2002a). Probabilistic Boolean networks: a rule-based uncertainty model for gene regulatory networks. Bioinformatics 18, 261–274. doi: 10.1093/bioinformatics/18.2.261
Shmulevich, I., Dougherty, E., and Zhang, W. (2002b). From Boolean to probabilistic Boolean networks as models of genetic regulatory networks. Proc. IEEE 90, 1778–1792. doi: 10.1109/JPROC.2002.804686
Sionov, R., and Haupt, Y. (1999). The cellular response to p53: the decision between life and death. Oncogene 18:6145. doi: 10.1038/sj.onc.1203130
Sorrentino, F., Di Bernardo, M., Garofalo, F., and Chen, G. (2007). Controllability of complex networks via pinning. Phys. Rev. E 75:046103. doi: 10.1103/PhysRevE.75.046103
Strigari, L., Mancuso, M., Ubertini, V., Soriani, A., Giardullo, P., Benassi, M., et al. (2014). Abscopal effect of radiation therapy: interplay between radiation dose and p53 status. Int. J. Radiat. Biol. 90, 248–255. doi: 10.3109/09553002.2014.874608
Suzuki, H., Kurita, M., Mizumoto, K., Nishimoto, I., Ogata, E., and Matsuoka, M. (2003). p19ARF-induced p53-independent apoptosis largely occurs through BAX. Biochem. Biophys. Res. Commun. 312, 1273–1277. doi: 10.1016/j.bbrc.2003.11.071
Sykes, S., Mellert, H., Holbert, M., Li, K., Marmorstein, R., Lane, W., et al. (2006). Acetylation of the p53 DNA-binding domain regulates apoptosis induction. Mol. Cell 24, 841–851. doi: 10.1016/j.molcel.2006.11.026
Szallasi, Z., Stelling, J., and Periwal, V. (2006). System Modeling in Cellular Biology. From Concepts to Nuts and Bolts. Cambridge, MA: MIT Press. doi: 10.7551/mitpress/9780262195485.001.0001
Vassilev, L., Vu, B., Graves, B., Carvajal, D., Podlaski, F., Filipovic, Z., et al. (2004). In vivo activation of the p53 pathway by small-molecule antagonists of mdm2. Science 303, 844–848. doi: 10.1126/science.1092472
Villunger, A., Michalak, E., Coultas, L., Müllauer, F., Böck, G., Ausserlechner, M., et al. (2003). p53-and drug-induced apoptotic responses mediated by BH3-only proteins puma and noxa. Science 302, 1036–1038. doi: 10.1126/science.1090072
Vinayagam, A., Gibson, T., Lee, H., Yilmazel, B., Roesel, C., Hu, Y., et al. (2016). Controllability analysis of the directed human protein interaction network identifies disease genes and drug targets. Proc. Natl. Acad. Sci. U.S.A. 113, 4976–4981. doi: 10.1073/pnas.1603992113
Wagner, J., Ma, L., Rice, J., Hu, W., Levine, A., and Stolovitzky, G. (2005). p53-MDM2 loop controlled by a balance of its feedback strength and effective dampening using ATM and delayed feedback. IEEE Proc. Syst. Biol. 152, 109–118. doi: 10.1049/ip-syb:20050025
Wang, H., Qian, L., and Dougherty, E. (2010). Inference of gene regulatory networks using S-system: a unified approach. IET Syst. Biol. 4, 145–156. doi: 10.1049/iet-syb.2008.0175
Wang, L., Liu, Y., Wu, Z., Lu, J., and Yu, L. (2019). Stabilization and finite-time stabilization of probabilistic Boolean control networks. IEEE Trans. Syst. Man Cybernet. 1–8. doi: 10.1109/TSMC.2019.2898880
Wang, R., Saadatpour, A., and Albert, R. (2012). Boolean modeling in systems biology: an overview of methodology and applications. Phys. Biol. 9:055001. doi: 10.1088/1478-3975/9/5/055001
Wang, X., and Chen, G. (2002). Pinning control of scale-free dynamical networks. Phys. A 310, 521–531. doi: 10.1016/S0378-4371(02)00772-0
Weber, J., Taylor, L., Roussel, M., Sherr, C., and Bar-Sagi, D. (1999). Nucleolar ARF sequesters MDM2 and activates p53. Nat. Cell Biol. 1:20. doi: 10.1038/8991
Wee, K., Surana, U., and Aguda, B. (2009). Oscillations of the p53-AKT network: implications on cell survival and death. PLoS ONE 4:e4407. doi: 10.1371/journal.pone.0004407
Wee, K., Yio, W., Surana, U., and Chiam, K. (2012). Transcription factor oscillations induce differential gene expressions. Biophys. J. 102, 2413–2423. doi: 10.1016/j.bpj.2012.04.023
Weinberg, R., Veprintsev, D., Bycroft, M., and Fersht, A. (2005). Comparative binding of p53 to its promoter and DNA recognition elements. J. Mol. Biol. 348, 589–596. doi: 10.1016/j.jmb.2005.03.014
Yee-Lin, V., Pooi-Fong, W., and Soo-Beng, A. (2018). Nutlin-3, a p53-mdm2 antagonist for nasopharyngeal carcinoma treatment. Mini Rev. Med. Chem. 18, 173–183. doi: 10.2174/1389557517666170717125821
Yue, D., Guan, Z., Li, T., Liao, R., Liu, F., and Lai, Q. (2017). Event-based cluster synchronization of coupled genetic regulatory networks. Phys. A 482, 649–665. doi: 10.1016/j.physa.2017.04.024
Zerrouqi, A., Pyrzynska, B., Brat, D., and Van Meir, E. (2014). P14arf suppresses tumor-induced thrombosis by regulating the tissue factor pathway. Cancer Res. 74, 1371–1378. doi: 10.1158/0008-5472.CAN-13-1951
Zerrouqi, A., Pyrzynska, B., Febbraio, M., Brat, D., and Van Meir, E. (2012). P14arf inhibits human glioblastoma-induced angiogenesis by upregulating the expression of timp3. J. Clin. Invest. 122, 1283–1295. doi: 10.1172/JCI38596
Keywords: p53, Mdm2, p14ARF, pinning control, computational modeling
Citation: Suarez OJ, Vega CJ, Sanchez EN, González-Santiago AE, Rodríguez-Jorge O, Alanis AY, Chen G and Hernandez-Vargas EA (2020) Pinning Control for the p53-Mdm2 Network Dynamics Regulated by p14ARF. Front. Physiol. 11:976. doi: 10.3389/fphys.2020.00976
Received: 23 August 2019; Accepted: 17 July 2020;
Published: 28 August 2020.
Edited by:
George Bebis, University of Nevada, Reno, United StatesReviewed by:
Jaewhan Song, Yonsei University, South KoreaAbdessamad Zerrouqi, Medical University of Warsaw, Poland
Copyright © 2020 Suarez, Vega, Sanchez, González-Santiago, Rodríguez-Jorge, Alanis, Chen and Hernandez-Vargas. This is an open-access article distributed under the terms of the Creative Commons Attribution License (CC BY). The use, distribution or reproduction in other forums is permitted, provided the original author(s) and the copyright owner(s) are credited and that the original publication in this journal is cited, in accordance with accepted academic practice. No use, distribution or reproduction is permitted which does not comply with these terms.
*Correspondence: Edgar N. Sanchez, ZWRnYXIuc2FuY2hlekBjaW52ZXN0YXYubXg=; Esteban A. Hernandez-Vargas, dmFyZ2FzQGZpYXMudW5pLWZyYW5rZnVydC5kZQ==