- 1Centro de Investigação Marinha e Ambiental, Universidade do Algarve, Faro, Portugal
- 2Centre of Marine Sciences, Universidade do Algarve, Faro, Portugal
Within a scenario of increasing atmospheric CO2 and ocean acidification (OA), it is highly relevant to investigate its impacts not only on fish performance but also on fish intestinal microbiome and how that reflects on host performance and health. The main objective of this study was to establish if the intestinal microbiota of the sea bream (Sparus aurata) was affected by high level of CO2 in line with the predictions for this century. The bacterial communities of the intestinal fluid were characterized in animals kept at the present-day level of CO2 (400 μatm) and in animals switched to high CO2 (1200 μatm) for 1 month. Bacterial taxa identification was based on molecular methods, using the DNA coding for the 16S ribosomal RNA and primers targeting the regions V1–V3. Amplicons obtained from DNA samples of animals in the same tank were combined, cloned to obtain a bacterial DNA library, and the clones were sequenced. No significant differences were found between the two treatments for alpha diversity. However, beta diversity analysis revealed distinct dysbiosis in response to hypercapnia, with phylum Firmicutes absent from the bacterial communities of fish exposed to 1200 μatm CO2, whereas Proteobacteria relative abundance was increased at elevated CO2, due to the presence of Gammaproteobacteria (Vibrionaceae and Alteromonadaceae), a class not present in the control samples. This study provides a first glimpse at the impact of OA in fish intestinal microbiota and highlights potential downstream effects to the general condition of fishes under hypercapnia.
Introduction
In a global scenario, as a result of anthropogenic emissions in the atmosphere, mean oceanic CO2 values are expected to reach 1000 μatm CO2 by year 2100 and 1900 μatm CO2 by year 2300 (Caldeira and Wickett, 2003; Meehl et al., 2007). The ocean will act as a sinkhole of this CO2 resulting in a decrease of pH (IPCC, 2007, 2014; Kroeker et al., 2013), equivalent to a decline of 0.32 and 0.77 units, respectively, in the average ocean surface pH (Caldeira and Wickett, 2005). This shift in ocean chemistry is expected to have a profound impact in marine organisms (Secretariat of the Convention on Biological Diversity, 2014), as has been discussed in the literature with special emphasis on fish [see the review by Heuer and Grosell (2014) and references there in].
It has been shown that at higher partial pressure of CO2 (pCO2), the marine fish intestine has an increased metabolic demand (Heuer and Grosell, 2016), coupled to an increased intestinal ion transport and movement of plasma HCO3– into the intestinal lumen in exchange for Cl–. Compensation for elevated pCO2 typically occurs within days (Esbaugh et al., 2012) and, as a consequence, the mechanism of adaptation of marine fish to hyperosmotic environment, naturally present in the intestine, is intensified by hypercapnia. As such, an increase in HCO3– secretion into the intestinal lumen promotes a faster increase in pH levels in parallel with the accumulation of calcium and magnesium in the intestinal fluid. This results in increased formation of carbonate aggregates, reduced osmolality and facilitated water absorption (Gregório et al., 2019) at high pCO2. The augment in energetic cost for ion transport processes and the increased O2 consumption observed in the intestine at elevated pCO2, may diminish the energy available for digestive functions, by slowing the process of digestion which has been observed in different species (Tirsgaard et al., 2015; Heuer and Grosell, 2016).
The gastrointestinal tract (GIT) is a favorable ecological niche for microorganisms and several reviews have highlighted the roles played by the microbial communities, strongly suggesting that fish gut microbiota is involved in energy homeostasis by affecting processes such as feeding, digestion, metabolism and also the immune response (Nayak, 2010; Egerton et al., 2018; Wang et al., 2018; Butt and Volkoff, 2019). The fish gut bacterial communities may contain pathogenic, symbiotic and commensal bacteria and they can exert great effect on the host welfare which may result in mucosal tolerance or inflammation (Gomez and Balcázar, 2008; Merrifield et al., 2010). It is well accepted that the microbiota–gut–brain axis (MGB axis) has a pivotal role in host health, stress physiology and behavior (Mueller et al., 2012; Llewellyn et al., 2014; Foster et al., 2017).
In terms of structure there is a distinction between the allochthonous, or the free-living, microbiota associated with the digesta, and the autochthonous communities, which are able to colonize the mucosal surface of the digestive tract (Nayak, 2010; Banerjee and Ray, 2017). The density, composition and function of the autochthonous microbiota communities, in contrast with the allochthonous bacteria, varies distinctly in the different sections of the fish GIT (Nayak, 2010; Clements et al., 2014; Banerjee and Ray, 2017).
The intestinal allochthonous microbiota, more abundant and variable in composition than the autochthonous communities, has been suggested to play an important role in nutrient digestion and absorption (Ramirez and Dixon, 2003; Merrifield et al., 2010; Dimitroglou et al., 2011).
The dietary effect on fish gut microbiota has received special attention in the context of aquaculture of economically important species such as seabream (de Paula Silva et al., 2011; Estruch et al., 2015; Piazzon et al., 2017; Nikouli et al., 2018), salmon (Green et al., 2013; Dehler et al., 2017; Uren Webster et al., 2018) and cod (Ringø et al., 2006; Zhou et al., 2013), to name a few. Besides dietary and host-specific intrinsic factors (genetics, age, feeding habits), environmental factors such as wild vs. rearing conditions, seasonality, salinity, and temperature have also been studied and are known to influence fish gut microbiota structure (Nayak, 2010; Tarnecki et al., 2017; Egerton et al., 2018; Butt and Volkoff, 2019).
Given the intestinal environment and functioning modifications observed at elevated CO2, it is reasonable to expect that these changing conditions might affect the microbiota associated with the fish gut. As such, alterations in the quality of microorganisms in the GIT, which can be reflected in the host by mutualistic relation effects, could constitute another downstream impact of CO2 hitherto overlooked.
However, to the best of our knowledge, how the chemical and physiological compensatory processes that take place at elevated CO2 interact with the intestinal microbiota are still to be analyzed. In view of this, the aim of the present work was to investigate the effect of elevated CO2 in the bacterial community associated with intestinal fluid of sea bream (Sparus aurata), using molecular methods.
Materials and Methods
Ethics Statement
All the procedures were approved by CCMAR Animal Welfare Committee (ORBEA CCMAR-CBMR) and Direcção-Geral de Alimentação e Veterinária (DGAV) of the Portuguese Government, according to national legislation translation of EU Directive 2010/63/EU. Procedures were only applied to live animals by authorized users.
Animals, Experimental Conditions, and Sampling
Sea bream (S. aurata) juveniles were purchased from CUPIMAR SA (Cadiz, Spain) and transported to Ramalhete Marine Station (CCMAR, University of Algarve, Faro, Portugal). Fish were maintained for 60 days in 1000 L tanks with running seawater (36 ppt) at a density 9–10 kg m–3 and fed 2% ration (fish wet weight, Sorgal, S.A., Portugal: Balance 3) once daily until the start of the experiment (all food was consumed by the fish). The experiment was setup by transferring the fish into 100 L flow-through tanks (without re-circulation) at a density of four fish (250 g body weight) per tank, in a total of six tanks. Temperature and photoperiod were natural (September-October, Algarve, Portugal) and the feeding regime was maintained as above during the 30 days of the experiment. No mortality was observed during the experiments. Fish were weighted and measured at the beginning and at the end of the experiment.
Each treatment (control and high pCO2, herein named 400 μatm CO2 and 1200 μatm CO2, respectively) had three replicates (3 × 100 L flow-through tank with four fish per tank). To achieve elevated levels of CO2, the rate of injection in the water was controlled by the pH level of seawater using pH probes connected to CO2 injection controllers (EXAxt PH450G; Yokogawa Iberia-Portugal). Each independent header-tank was gassed with CO2, thus obtaining two groups constantly maintained at 400 (control, no CO2 injection), and 1200 (high) μatm CO2. Seawater pH (NBS scale) was measured daily to calibrate the automated negative feedback system for CO2 injection (details in Gregório et al., 2019). Total alkalinity (TA) was measured using a combination DL15 titrator and a DG115-SC probe (Mettler-Toledo) using certified acid titrant (0.1 M HCl, Fluka Analytical, Sigma-Aldrich). Based on the measurements of salinity, temperature, alkalinity and pH, water pCO2 was calculated using CO2Calc Software (version 1.0.4; Robbins et al., 2010).
After 30 days of the experiment onset, fish were fasted for 24 h, and sampled between 9:30 and 11:00 AM under anesthesia in 2-phenoxyethanol (1: 10,000 v/v; Sigma–Aldrich, St. Louis, MO, United States). Fish were sacrificed by decapitation. The whole intestine was isolated and clamped (from pyloric caeca to anal sphincter) with two hemostatic forceps. Next the intestinal fluid was collected into a sterile vial for DNA extraction. The whole procedure was done in a flow cabinet, on an ice tray covered with a fresh disposable sterile cloth and using fresh disposable sterile dissecting tools for each fish.
DNA Extraction
DNA extraction was conducted in fresh intestinal fluid samples, as soon as the animals were dissected, using the EZNA stool DNA kit (Omega Bio-tek, United States; 50 preps) following the manufacturer instructions in the Pathogen Detection Protocol. After extraction, DNA was stored at −20°C until used.
PCR, Cloning, and Sequencing
All DNA samples obtained from the 24 animals (4 fish per tank and three tanks per treatment) were subjected to PCR, targeting the 16S rRNA coding gene. For that the primer pair 27F and 533R (Piazzon et al., 2017) was used, allowing the amplification of a 507 bp fragment containing the hypervariable regions V1–V3. PCR reactions were prepared in a PCR workstation to a final volume of 50 μl, containing: 5 μl of MTP Taq 10× Buffer, 2 mM of MgCl2, 200 μM of each dNTP, 200 nM of each primer, 2.5 U of MTP Taq DNA polymerase (Sigma-Aldrich Inc., United States) and 5 μl of DNA. Dedicated micropipettes and filtered tips were used throughout. Amplification reactions were done in a T100 Thermal Cycler (Bio-Rad Laboratories, Inc.) with the following program: initial 5 min at 95°C, followed by 30 cycles of 30 s at 94°C, 30 s at 53°C, and 30 s at 72°C, and a final extension of 5 min at 72°C. The round of PCR reactions was prepared to include three negative controls and a positive control (Escherichia coli DNA). Ten microliters of each PCR product were visualized under UV light after agarose gel electrophoresis (1.5% agarose in TAE 1x) and staining with ethidium bromide. All the negative controls showed no amplification band and the PCR products were processed further.
The amplicons obtained from each of the 4 fish from each tank were combined and ligated into the cloning vector using the pGEM-T Easy System I kit (Promega, United States) according to the manufacturer’s instructions. Each of these ligations, in a total of six ligations, was used to transform competent XL1 Blue E. coli cells. Transformations were plated onto LB-agar plates containing 50 μg.ml–1 ampicillin and to which surface 40 μL of X-Gal solution (20 mg/ml solution, Thermo Scientific) and 40 μL of IPTG solution (100 mM solution, Thermo Scientific) had been evenly spread. After overnight incubation, the white colonies were transferred to PCR tubes containing the following mix: 5 μl of Dream Taq 10x Buffer, 2 mM of MgCl2, 200 μM of each dNTP, 200 nM of each primer (primers M13F and M13R from the pGEM vector) and 1 U of dream Taq DNA polymerase (Thermo Fischer Scientific, Inc., United States). Amplification reactions, including a negative control, were done in a T100 Thermal Cycler (Bio-Rad Laboratories, Inc.) with the following program: initial 5 min at 95°C, followed by 30 cycles of 30s at 94°C, 30s at 60°C, and 30 s at 72°C, and a final extension of 5 min at 72°C. After agarose gel electrophoresis (1.5% agarose in TAE 1×) and staining with ethidium bromide, the PCR products were visualized under UV light.
PCR products corresponding to the expected size fragment were purified using the EZNA Cycle Pure kit (Omega Bio-tek, United States), and selected for commercial sequencing (CCMAR, Portugal; Sanger sequencing) using primers T7 and SP6 from the pGEM vector. Because some of the amplified fragments (less than 10% of the total clones screened per combined sample) were either longer or shorter than the expected size, these were also purified and sequenced for subsequent analysis.
Sequence Data Analysis and Taxonomic Identification
The sequences obtained were visualized with the sequence editor BioEdit (Hall, 1999) and cut by the primers (27F and 533R). Each sequence obtained was individually subjected to a BLAST (Basic Local Alignment Search Tool) analysis (blastn), with the application BLASTN 2.8.0 available at NCBI1. Sequences resulting from amplicons longer or shorter than the expected size were also analyzed in the BLAST nucleotide database. It was verified they corresponded to low complexity sequences and were hence removed from subsequent analysis. The software Decipher version 2.11.3 (Wright et al., 2012) was used to identify possible chimeras, which were also removed from the datasets. At least 30 quality sequences were obtained per combined sample (F1–F4, F9–F12, F17–F20 for 400 μatm CO2 and F5–F8, F13–F16, F21–F24for 1200 μatm CO2).
Following this initial screening, FASTA files were generated with the sequences obtained for each combined sample, in a total of 6 files. Taxonomy assignment was done using the RDP classifier (Wang et al., 2007), with a confidence threshold of 80%. Each FASTA file was also submitted to a BLASTN analysis using the database for 16S ribosomal RNA sequences (Bacteria and Archaea) and excluding non-cultured organisms. The files obtained from RDP and from BLASTN were imported into MEGAN version 6.12.0 (Huson et al., 2016), to estimate the taxonomic composition of each sample microbiota. MEGAN is a software package used to calculate and explore the taxonomic content of metagenomic data, namely of data obtained through the sequencing of microbiomes. In MEGAN the assignment of reads to taxa based on the BLASTN files (100 matches per read) was computed using the naive LCA (Lowest Common Ancestor) algorithm (Huson et al., 2007), with a minimum threshold of 50 for the bit disjointScore of alignments or “hits,” and a maximum threshold of 0.01 for the E-value. No discrepancies were found between the two taxonomic assignments.
Statistics
Using the pipeline implemented in MEGAN, rarefaction curves were first obtained for each of the six combined samples, by plotting the number of observed OTUs (OTU-operational taxonomic unit), or number of leaves in the taxonomy tree, against the number of sequences, or number of reads sampled from leaves. Subsequently, diversity indexes (Shannon–Weaver and Simpson reciprocal) were calculated for each combined sample, as a measure of alpha-diversity. The hit maps [number of reads allocated to each OTU (Family level)] were imported to PRIMER-e, version 7 software (Massey University, Albany, New Zealand) to explore the variance in microbial communities and test beta-diversity through multivariate analyses, using PERMANOVA + add-on (Clarke et al., 2014). All data were square root transformed and a Bray-Curtis dissimilarity coefficient was used to construct a resemblance matrix, with data visualized using non-metric multidimensional scaling analysis (nMDS). SIMPROF analyses were also conducted to further test for structure in the data. SIMPER (Similarity percentages) was used to identify significant alteration in the composition of OTUs and which OTUs were mainly responding to the effect of elevated CO2.
Results
Experimental conditions and seawater physicochemical parameters are given in Table 1, as mean values (with standard deviations) of the three tanks per CO2 concentration used.
The effect of exposure to elevated pCO2 during the 30 days of the experiment did not significantly affect fish weight nor fish length (Supplementary Figure S1).
The data presented here are based on the assigned sequences (reads) obtained for each sample (Table 2). The classification of the sequences resulted in 19 OTUs assignments (family level) from 6 phyla (Figure 1A, Supplementary Figure S2, and Supplementary Files S1–S6). Based on the rarefaction curves (Supplementary Figure S2) and the Chao1 index (Table 2), satisfactory coverage of the existing bacterial OTUs was reached for the majority of the samples.

Table 2. Alpha diversity estimates through the determination of diversity indexes of the microbiota of the intestinal fluid of Sparus aurata.
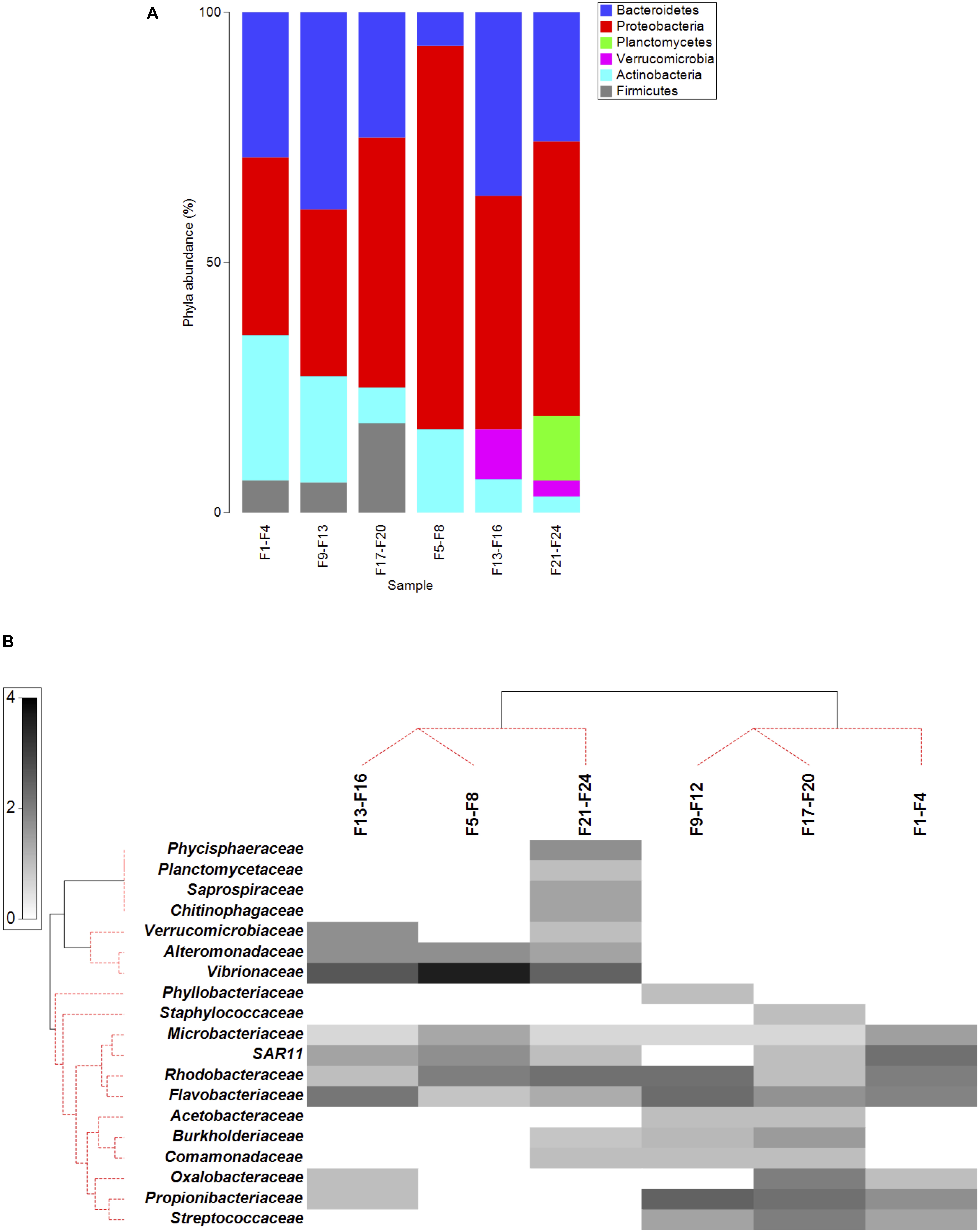
Figure 1. (A) Phyla abundance (%) in the microbiota of the intestinal fluid of Sparus aurata at 400 μatm CO2 (F1–F4, F9–F12, F17–F20) and at 1200 μatm CO2 (F5–F8, F13–F16, F21–F24). (B) Shade plot of abundance of the 19 OTUs (in the rows; Family level) identified in the six samples (in the columns) of S. aurata intestinal fluid. White space denotes absence of that OUT in that sample. Depth of gray scale is linearly proportional to a square-root transformation of abundance. The same transform was used for sample clustering and for OUT clustering. Samples were grouped using Bray–Curtis similarities on the transformed data, by hierarchical, unconstrained divisive clustering (UNCTREE), together with Type I SIMPROF tests. Two separated groups of samples (continuous lines), corresponding to the two CO2 levels under study, were supported by the SIMPROF test (significance level 5%). OTUs were also clustered, with the standard agglomerative method (group average linked), based on index of association resemblances computed on the transformed data, together with level Type 3 SIMPROF tests. Three coherent groups (continuous lines; cophenetic correlation: 0.86) were supported by the SIMPROF test (significance level 5%).
Proteobacteria, Bacteroidetes, and Actinobacteria, were the dominant phyla across the intestinal fluid microbial communities of the six samples, averaging 40, 31, and 19%, respectively, at 400 μatm CO2, and 60, 23, and 9%, respectively, at 1200 μatm CO2 (Figure 1A).
Four families from class Alpha-proteobacteria were detected in this work, Acetobacteraceae, Phyllobacteriaceae, SAR11 and Rhodobacteraceae, although only the last was found in all of the six samples.
The Beta-proteobacteria, Burkholderiaceae, Comamon- adaceae, and Oxalobacteraceae, were detected in both treatments but not in all samples (Figure 1B).
The Gammaproteobacteria, Vibrionaceae and Alteromon- adaceae, were only present at elevated pCO2.
From phyla Bacteroidetes, the families Saprospiraceae and Chitinophagaceae were only found in one of the samples at elevated pCO2, whereas Flavobacteriaceae was found in all samples of both treatments.
Two families from phylum Actinobacteria were detected, Propionibacteriacea and Microbacteriaceae, with the last present in all samples.
Phylum Firmicutes was not detected at elevated pCO2, neither were phyla Planctomycetes and Verrucomicrobia, at 400 μatm CO2, although these two phyla were only detected in one of the high pCO2 samples.
The Shannon and Simpson diversity indexes (Table 2), as a measure of alpha diversity, were not significantly affected (t-test of the averaged values of the three samples per treatment) by elevated pCO2. However, microbial community compositions of the intestinal fluid at the two CO2 concentrations were found significantly different from each other (Bray-Curtis resemblance matrix, Monte–Carlo test P = 0.022, Supplementary Table S1), with no significant difference in dispersion of data within samples from the same treatment (PERMDISP, p > 0.05, Supplementary Table S1). Note that with a simple experimental design such as the present, the number of possible permutations is not sufficient for a proper PERMANOVA analysis. Instead the Monte Carlo test should be used (Clarke et al., 2014), as was done here. The differences between the two groups of samples were also supported by the LINKTREE (linkage tree) based on the Bray-Curtis similarity matrix, with samples clustering according to treatment (Figure 1B) (SIMPROF Type 1 test, p < 5%). Figure 1B also shows the OTUs clustering, with the standard agglomerative method (group average linked), based on the index of association resemblances. Three coherent groups (continuous lines) were supported by the SIMPROF Type 3 test (p < 5%; cophenetic correlation: 0.86), with two of the groups containing the OTUs only found at elevated pCO2.
The results of SIMPER (similarity percentages – species contributions, One-Way analysis) are shown in Supplementary Table S2. The average dissimilarity between samples from the two treatments was 60.97% whereas the similarity between samples from the same treatment was 65.24 and 60.93% for 400 μatm CO2 and 1200 μatm CO2, respectively. This analysis also revealed that the main OTUs driving the difference between the two treatments were Vibrionaceae (17.84%), Propionibacteriaceae (10.86%), Alteromonadaceae (9.94%), and Streptococcaceae (9.69%). This information was overlaid onto the result of the nMDS analysis (Figure 2), showing the significant difference in microbial community composition of the intestinal fluid between the two CO2 treatments.
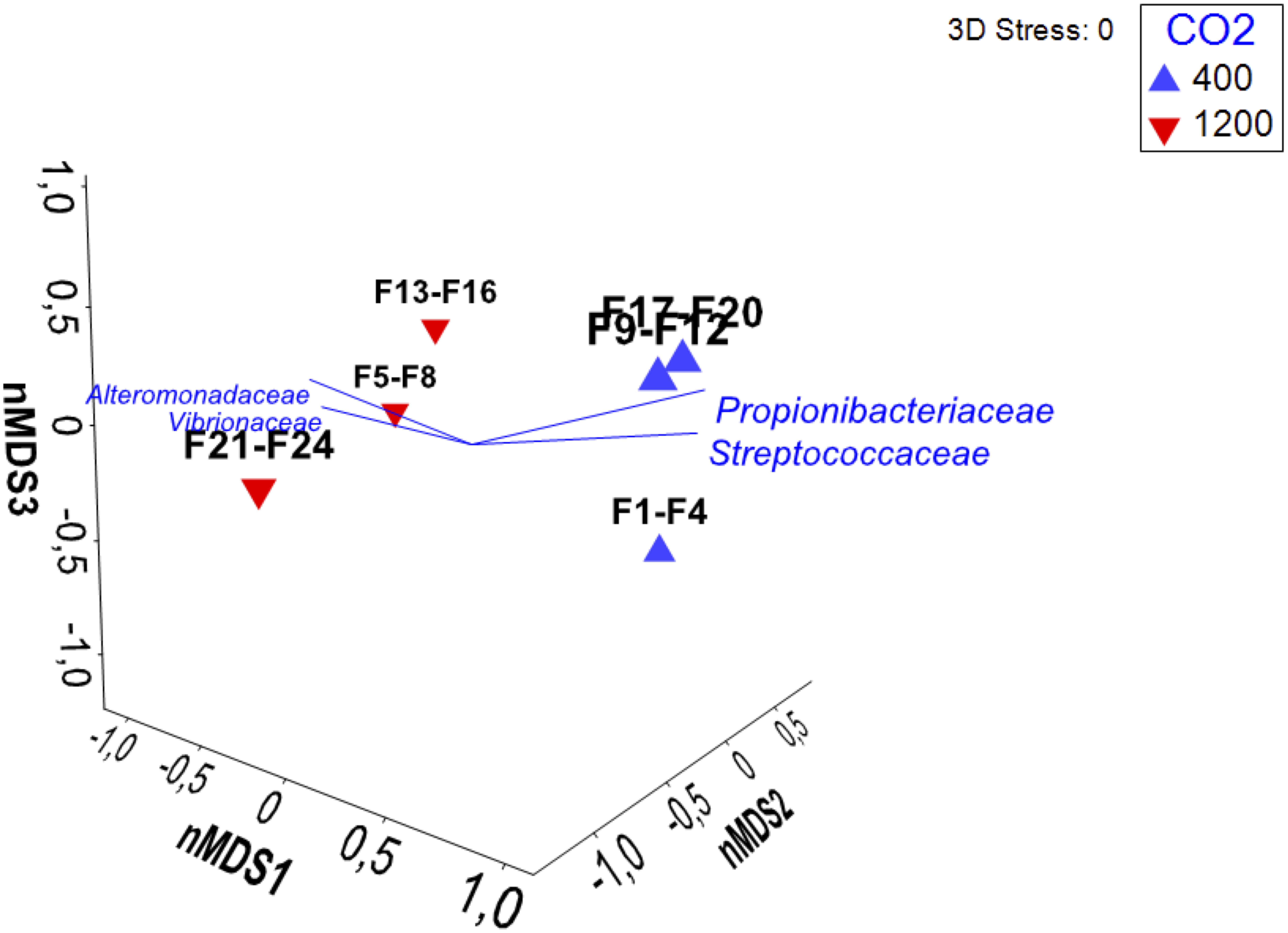
Figure 2. Non-metric multidimensional scaling (nMDS) plot based on Bray–Curtis similarity among microbial communities of S. aurata intestinal fluid samples, obtained from fish grown at 400 μatm CO2 (F1–4; F9–12; F17–20) and at 1200 μatm CO2 (F5–F8; F13–F16; F21–F24). The plot shows a clear change in community structure with the increase in pCO2 (p = 0.022, Monte–Carlo test). The vectors displayed indicate those OTUs identified as contributing most strongly toward microbiota similarity of samples from the same experimental group using SIMPER analysis. The vector direction for each OUT reflects the Pearson correlations of their square-root transformed counts with the two ordination axes, and length gives the multiple correlation coefficient from this linear regression on the ordination points.
Discussion
This study was conducted as a preliminary approach to verify the putative effect of elevated pCO2 on the microbiota of the intestinal fluid.
For 30 days fish were exposed to control (400 μatm) and elevated (1200 μatm) CO2, with four fish in 100 L flow-through tanks (circa 1 kg fish/tank), allowing for complete water volume renovation every hour. Each treatment had three tanks and its own water circuit and fish were fed the same diet. Thus, the only difference between treatments was the injection of CO2 in the 1200 μatm tanks. Since the water microbial communities between the control and elevated pCO2 tanks were not expected to be altered with this experimental setup, we did not analyse water samples in our work.
The OTUs detected in the fish intestinal fluid belonged to 6 phyla, commonly found in marine fish gut (Sullam et al., 2012; Givens et al., 2015; Merrifield and Rodiles, 2015; Tran et al., 2017; Wang et al., 2018).
The observation that Proteobacteria, Bacteroidetes Actinobacteria and Firmicutes were the phyla detected in the control CO2 concentration (400 μatm CO2) is consistent with previously published studies on seabream GIT microbiota, either including the luminal and the mucosa-associated microbiota (Estruch et al., 2015), only the mucosa (Kormas et al., 2014) or only the luminal (Nikouli et al., 2018).
The remaining two phyla, Planctomycetes and Verrucomicrobia, were only detected at elevated pCO2 although not in all of the samples. These results showed some variation in intestinal fluid microbiota between replicate tanks, mainly at elevated pCO2, but no significant difference in dispersion of data was found (PERMDISP, p > 0.05, Supplementary Table S1). Both phyla are known to sporadically occur as subdominant phyla in microbiota (Ikeda-Ohtsubo et al., 2018) but their contribution to the structure and function of fish microbiota is not further explored in the literature.
Despite the differences in bacterial communities between the two treatments, three families from three different phyla were present in all of the samples: Flavobacteriaceae (Bacteroidetes), Rhodobacteraceae (Alphaproteobacteria), and Microbacteriaceae (Actinobacteria). These families were also identified in seabream by other authors (Estruch et al., 2015; Nikouli et al., 2018). A closer examination at the genus level (Supplementary Files S1–S6) shows a great dispersion in the genera present even in samples from the same treatment, with most of the genera identified with a low CT. In microbiota studies, OTUs present across a range of samples being compared are usually considered as the core biome (Jones et al., 2018), so we propose those three families might constitute the core microbiota of seabream in our experimental conditions.
The consistent differences in intestinal fluid microbial communities between the two CO2 treatments were that phylum Firmicutes was not found in the bacterial communities of fish exposed to 1200 μatm CO2, whereas Proteobacteria relative abundance was increased at elevated pCO2, due to the presence of Gammaproteobacteria (Vibrionaceae and Alteromonadaceae), a class not present in the control samples. Similarly, an increase in Vibrionaceae and Alteromonadaceae at elevated pCO2 was also reported in tissues, skeleton, and mucus of colonies of the coral Acropora eurystoma, exposed to pH 7.3 for 10 weeks, compared with those exposed to ambient pH 8.2 (Meron et al., 2011). An increase in antibacterial activity was also seen and was attributed mostly to an increase in Vibrio sp., suggesting a mechanism for host invasion (Meron et al., 2011).
Vibrionaceae, Alteromonadaceae, and different genera of lactic acid bacteria in phylum Firmicutes have been described in marine fish (Hovda et al., 2007; Denev et al., 2009; Pérez et al., 2010; Roeselers et al., 2011). In the study by Piazzon et al. (2017) on the intestinal mucus of seabream, in fish farm rearing conditions, Vibrionaceae (Photobacterium and Vibrio) was the dominant family in all diet groups tested. However, in a previous study by Estruch et al. (2015), using closed recirculation systems, Vibrionaceae was found in the stomach but not in the foregut, midgut or hindgut of seabream. In another study with seabream (Nikouli et al., 2018), from different commercial aquaculture farms in Greece, only Pseudomonadales, from the Gammaproteobacteria, were present.
These three studies differ in the allochthonous vs. autochthonous proportion of microbiota that was sampled, in experimental setup (water circulation, diet, feeding time, duration of the experiment) and in the environmental conditions (salinity, temperature). Having in view how sensitive the GIT microbiota is to any of those variables (Wang et al., 2018), these differences are most probably at the root of the apparently conflicting results and show that comparison between microbiota data sets needs to be made with caution and studies need to report the details of the sample preparation.
In our work, the Vibrionaceae present were attributed to genera Vibrio and Lucibacterium (Vibrio harveyi, Farmer et al., 2005), albeit with a confidence threshold (CT) frequently lower that 80% (Supplementary Files S4–S6). Photobacterium was not found in our samples.
Vibrio is one of the most important bacterial genera in aquaculture, with both pathogenic and probiotic species (Vandenberghe et al., 2003). Vibrio species are motile and facultative anaerobes. Although some Vibrio are clearly identified pathogens of marine organisms, as is Vibrio harveyi, it has been postulated that many Vibrio species could be not true pathogens, but rather opportunistic pathogens, whose virulence is accentuated under intensive aquaculture conditions (Thompson et al., 2004). Many Vibrio species are known to produce hydrolytic enzymes (amylase, lipase, chitinase) and in this way they can act as symbionts assisting in the breakdown of dietary components (Ray et al., 2012). However, whether or not the Vibrio detected at elevated pCO2 can behave as pathogens clearly needs further attention as this could add to the already described impact of elevated pCO2 on the immune system of fish (Kaya et al., 2013; Bresolin de Souza et al., 2014).
All the Alteromonadaceae present in our samples were attributed to genus Aliiglaciecola (CT 78%). Alteromonadaceae are aerobic and motile and related to polysaccharide degradation. It was observed that SAR11 abundance is increased in the presence of Alteromonadaceae (Wietz et al., 2015) an effect attributed to cross-feeding on alginate monomers and other metabolic products released by alginolytic Alteromonadaceae (Wietz et al., 2015). In our study SAR11 (CT 100%, Candidatus pelagibacter, Supplementary Files S1–S6) was consistently detected in all of the samples from elevated pCO2, but in only two of the control samples. SAR11 are chemoheterotrophic marine bacteria with a large impact on the cycling of carbon and other important nutrients in the oceans, capable of withstanding and adjust quickly to fluctuations in the availability of nutrients (Sowell et al., 2008).
The presence of Gammaproteobacteria at elevated pCO2 was coincident with the absence of Firmicutes. Firmicutes and Bacteroidetes are known to contribute to carbohydrates and/or proteins fermentation in the intestine to help the host acquire nutrients from the diet (Spor et al., 2011). In our study phylum Bacteroidetes was present at 1200 μatm CO2, but phylum Firmicutes could not be detected in this treatment. However, family Streptococcaceae, genus Streptoccocus (CT 100%, Supplementary Files 1–3) was detected in all control samples. Streptococcus was also the most abundant Firmicutes genus found in seabream by Estruch et al. (2015). Lactic acid bacteria (LAB) such as Streptococcus, and Firmicutes in general, are thought to be important to host carbohydrate metabolism through fermentation and have been associated with efficient dietary energy harvest (Turnbaugh et al., 2006; Guo et al., 2008; Krajmalnik-Brown et al., 2012). Intestinal composition of LAB is known to vary with season in different species (Wang et al., 2018, and references therein) and also with the feeding regime (Parris et al., 2019). LAB are among the microbiota bacteria most responsive to feeding, with many taxa showing a positive response, a factor that may also contribute to their role in energy extraction from food. Interestingly, Propionibacteriaceae, were also negatively affected by elevated pCO2. Although present in all of the control tanks, this family was found in only one of the elevated pCO2 samples. Propionibacterium, the genus detected (CT 100%, Supplementary Files S1–S3, S5), is a lipophilic facultative anaerobe that feeds on lactate, and is used in calves as a probiotic to counteract low ruminal pH that might cause subacute ruminal acidosis or SARA (Brugman et al., 2018).
Conclusion
Our observations provide a first glimpse of how the bacterial community in fish gut can be affected by exposure to elevated pCO2. The data suggests that the chemical and physiological compensatory processes, taking place in the intestinal lumen of the fish, provoke the significant dysbiosis seen at elevated pCO2, by affecting mainly the LAB in the intestinal fluid bacterial communities, while favoring bacteria with other pathways of polysaccharide and protein degradation. This shift might be related to the delayed digestion observed in fish under hypercapnia. Since the bacterial groups replacing LAB can harbor putative pathogens, future studies characterizing the immune response of fishes to elevated pCO2 will need to analyze and take into account alterations in fish microbiota.
Data Availability Statement
The datasets generated for this study can be found in the NCBI Nucleotide Database F1–F4: MN240895–MN240926, F9–F12: MN240969–MN241001, F17–F20: MN240937–MN240968, F5–F8: MN243925–MN243954, F13–F16: MN241002–MN241031, and F21–F24: MN257910–MN257940.
Author Contributions
FF and JF planned the work and designed the study. JF sampled the animals. FF and RC performed the laboratory work and the data analyses. FF, RC, and JF wrote the manuscript and read and approved the content of the manuscript.
Funding
This work was supported by the Ministry of Science and Higher Education and European Social Funds through the Portuguese National Science Foundation (FCT) by Project PTDC/MAR-BIO/3034/2014 to JF. CCMAR and CIMA were supported by national funds from the Portuguese Foundation for Science and Technology (FCT) through projects UID/Multi/04326/2019 and UID/MAR/00350/2013, respectively.
Conflict of Interest
The authors declare that the research was conducted in the absence of any commercial or financial relationships that could be construed as a potential conflict of interest.
Supplementary Material
The Supplementary Material for this article can be found online at: https://www.frontiersin.org/articles/10.3389/fphys.2019.01446/full#supplementary-material
Footnotes
References
Banerjee, G., and Ray, A. K. (2017). Bacterial symbiosis in the fish gut and its role in health and metabolism. Symbiosis 72, 1–11. doi: 10.1007/s13199-016-0441-8
Bresolin de Souza, K., Jutfelt, F., Kling, P., Förlin, L., and Sturve, J. (2014). Effects of increased CO2 on fish gill and plasma proteome. PLoS One 9:e102901. doi: 10.1371/journal.pone.0102901
Brugman, S., Ikeda-Ohtsubo, W., Braber, S., Folkerts, G., Pieterse, C. M. J., and Bakker, P. A. H. M. (2018). A comparative review on microbiota manipulation: lessons from fish, plants, livestock, and human research. Front. Nutr. 5:80. doi: 10.3389/fnut.2018.00080
Butt, R. L., and Volkoff, H. (2019). Gut microbiota and energy homeostasis in fish. Front. Endocrinol. 10:9. doi: 10.3389/fendo.2019.00009
Caldeira, K., and Wickett, M. E. (2003). Anthropogenic carbon and ocean pH. Nature 425, 365–365. doi: 10.1038/425365a
Caldeira, K., and Wickett, M. E. (2005). Ocean model predictions of chemistry changes from carbon dioxide emissions to the atmosphere and ocean. J. Geophys. Res. 110, 2156–2202. doi: 10.1038/nature13798
Clarke, K., Gorley, R., Somerfield, P., and Warwick, R. (2014). Change in Marine Communities: An Approach to Statistical Analysis and Interpretation, 3rd Edn. Plymouth: PRIMER-E.
Clements, K. D., Angert, E. R., Montgomery, W. L., and Choat, J. H. (2014). Intestinal microbiota in fishes: what’s known and what’s not. Mol. Ecol. 23, 1891–1898. doi: 10.1111/mec.12699
de Paula Silva, F. C., Nicoli, J. R., Zambonino-Infante, J. L., Kaushik, S., and Gatesoupe, F.-J. (2011). Influence of the diet on the microbial diversity of faecal and gastrointestinal contents in gilthead sea bream (Sparus aurata) and intestinal contents in goldfish (Carassius auratus). FEMS Microbiol. Ecol. 78, 285–296. doi: 10.1111/j.1574-6941.2011.01155.x
Dehler, C. E., Secombes, C. J., and Martin, S. A. M. (2017). Environmental and physiological factors shape the gut microbiota of Atlantic salmon parr (Salmo salar L.). Aquaculture 467, 149–157. doi: 10.1016/j.aquaculture.2016.07.017
Denev, S., Staykov, Y., Moutafchieva, R., and Beev, G. (2009). Microbial ecology of the gastrointestinal tract of fish and the potential application of probiotics and prebiotics in finfish aquaculture. Int. Aquat. Res. 1, 1–29.
Dimitroglou, A., Merrifield, D. L., Carnevali, O., Picchietti, S., Avella, M., Daniels, C., et al. (2011). Microbial manipulations to improve fish health and production– a mediterranean perspective. Fish Shellfish Immunol. 30, 1–16. doi: 10.1016/j.fsi.2010.08.009
Egerton, S., Culloty, S., Whooley, J., Stanton, C., and Ross, R. P. (2018). The gut microbiota of marine fish. Front. Microbiol. 9:873. doi: 10.3389/fmicb.2018.00873
Esbaugh, A. J., Heuer, R., and Grosell, M. (2012). Impacts of ocean acidification on respiratory gas exchange and acid-base balance in a marine teleost. Opsanus beta. J. Comp. Physiol. B 182, 921–934. doi: 10.1007/s00360-012-0668-5
Estruch, G., Collado, M. C., Peñaranda, D. S., Tomás Vidal, A., Jover Cerdá, M., and Pérez Martínez, G. (2015). Impact of fishmeal replacement in diets for gilthead sea bream (Sparus aurata) on the gastrointestinal microbiota determined by pyrosequencing the 16S rRNA Gene. PLoS One 10:e0136389. doi: 10.1371/journal.pone.0136389
Foster, J. A., Rinaman, L., and Cryan, J. F. (2017). Stress & the gut-brain axis: regulation by the microbiome. Neurobiol. Stress 7, 124–136. doi: 10.1016/j.ynstr.2017.03.001
Givens, C. E., Ransom, B., Bano, N., and Hollibaugh, J. T. (2015). Comparison of the gut microbiomes of 12 bony fish and 3 shark species. Mar. Ecol. Prog. Ser. 518, 209–223. doi: 10.3354/meps11034
Gomez, G. D., and Balcázar, J. L. (2008). A review on the interactions between gut microbiota and innate immunity of fish. FEMS Immunol. Med. Microbiol. 52, 145–154. doi: 10.1111/j.1574-695x.2007.00343.x
Green, T. J., Smullen, R., and Barnes, A. C. (2013). Dietary soybean protein concentrate-induced intestinal disorder in marine farmed Atlantic salmon, Salmo salar is associated with alterations in gut microbiota. Vet. Microbiol. 166, 286–292. doi: 10.1016/j.vetmic.2013.05.009
Gregório, S. F., Ruiz-Jarabo, I., Carvalho, E. M., and Fuentes, J. (2019). Increased intestinal carbonate precipitate abundance in the sea bream (Sparus aurata L.) in response to ocean acidification. PLoS One 14:e0218473. doi: 10.1371/journal.pone.0218473
Guo, X., Xia, X., Tang, R., Zhou, J., Zhao, H., and Wang, K. (2008). Development of a real-time PCR method for firmicutes and bacteroidetes in faeces and its application to quantify intestinal population of obese and lean pigs. Lett. Appl. Microbiol. 47, 367–373. doi: 10.1111/j.1472-765X.2008.02408.x
Hall, T. A. (1999). BioEdit: a user-friendly biological sequence alignment editor and analysis program for Windows 95/98/NT. Nucl. Acids. Symp. Ser. 41, 95–98.
Heuer, R. M., and Grosell, M. (2014). Physiological impacts of elevated carbon dioxide and ocean acidification on fish. Am. J. Physiol. Regul. Integr. Comp. Physiol. 307, R1061–R1084. doi: 10.1152/ajpregu.00064.2014
Heuer, R. M., and Grosell, M. (2016). Elevated CO2 increases energetic cost and ion movement in the marine fish intestine. Sci. Rep. 6:34480. doi: 10.1038/srep34480
Hovda, M. B., Lunestadt, B. T., Fontanillas, R., and Rosnes, J. T. (2007). Molecular characterisation of the intestinal microbiota of farmed Atlantic salmon (Salmo salar L.). Aquaculture 272, 581–588. doi: 10.1016/j.aquaculture.2007.08.045
Huson, D. H., Auch, A. F., Qi, J., and Schuster, S. C. (2007). MEGAN analysis of metagenomic data. Genome Res. 17, 377–386. doi: 10.1101/gr.5969107
Huson, D. H., Beier, S., Flade, I., Górska, A., El-Hadidi, M., Mitra, S., et al. (2016). MEGAN community edition – interactive exploration and 2 analysis of large-scale microbiome sequencing data. PLoS Comput. Biol. 12:e1004957. doi: 10.1371/journal.pcbi.1004957
Farmer, J. J. III., Janda, J. M., Brenner, F. W., Cameron, D. N., and Birkhead, K. M. (2005). “Genus 1. Vibrio Pacini 1854, 411AL,” in Bergey’s Manual of Systematic Bacteriology, The Proteobacteria Part B The Gammaproteobacteria, 2nd Edn, Vol. 2, eds D. J. Brenner, N. R. Krieg, and J. T. Staley, (New York NY: Springer), 494–546.
Ikeda-Ohtsubo, W., Brugman, S., Warden, C. H., Rebel, J. M. J., Folkerts, G., and Pieterse, C. M. J. (2018). How can we define “optimal microbiota?”: A comparative review of structure and functions of microbiota of animals. Fish, and plants in agriculture. Front. Nutr. 5:90. doi: 10.3389/fnut.2018.00090
IPCC (2007). “Climate change 2007: synthesis report,” in Contribution of Working Groups I, II and III to the Fourth Assessment Report of the Intergovernmental Panel on Climate Change, eds Core Writing Team, R. K. Pachauri and A. Reisinger, (Geneva: IPCC), 104.
IPCC (2014). “Climate change 2014: synthesis report,” in Contribution of Working Groups I, II and III to the Fifth Assessment Report of the Intergovernmental Panel on Climate Change, eds Core Writing Team, R. K. Pachauri and L. A. Meyer, (Geneva: IPCC), 151.
Jones, J., DiBattista, J. D., Stat, M., Bunce, M., Boyce, M. C., Fairclough, D. V., et al. (2018). The microbiome of the gastrointestinal tract of a range-shifting marine herbivorous fish. Front. Microbiol. 9:2000. doi: 10.3389/fmicb.2018.02000
Kaya, H., Yılmaz, S., Gürkan, M., and Hisar, O. (2013). Effects of environmental hypercapnia on hemato-immunological parameters, carbonic anhydrase, and Na+, K+-ATPase enzyme activities in rainbow trout (Oncorhynchus mykiss) tissues. Toxicol. Environ. Chem. 95, 1395–1407. doi: 10.1080/02772248.2013.869332
Kormas, K. A., Meziti, A., Mente, E., and Frentzos, A. (2014). Dietary differences are reflected on the gut prokaryotic com-munity structure of wild and commercially reared sea bream (Sparus aurata). Microbiol. Open 3, 718–728. doi: 10.1002/mbo3.202
Krajmalnik-Brown, R., Ilhan, Z. E., Kang, D. W., and DiBaise, J. K. (2012). Effects of gut microbes on nutrient absorption and energy regulation. Nutr. Clin. Pract. 27, 201–214. doi: 10.1177/0884533611436116
Kroeker, K. J., Kordas, R. L., Crim, R., Hendriks, I. E., Ramajo, L., Singh, G. S., et al. (2013). Impacts of ocean acidification on marine organisms: quantifying sensitivities and interaction with warming. Glob. Chang. Biol. 19, 1884–1896. doi: 10.1111/gcb.12179
Llewellyn, M. S., Boutin, S., Hoseinifar, S. H., and Derome, N. (2014). Teleost microbiomes: the state of the art in their characterization, manipulation and importance in aquaculture and fisheries. Front. Microbiol. 5:207. doi: 10.3389/fmicb.2014.00207
Meehl, G. A., Stocker, T. F., Collins, W. D., Friedlingstein, P., Gaye, A. T., Gregory, J. M., et al. (2007). “Global Climate Projections,” in Climate Change 2007: The Physical Science Basis. Contribution of Working Group I to the Fourth Assessment Report of the Intergovernmental Panel on Climate Change 2007, (Cambridge: Cambridge University).
Meron, D., Atias, E., Iasur Kruh, L., Elifantz, H., Minz, D., Fine, M., et al. (2011). The impact of reduced pH on the microbial community of the coral Acropora eurystoma. ISME J. 5, 51–60. doi: 10.1038/ismej.2010.102
Merrifield, D. L., Dimitroglou, A., Foey, A., Davies, S. J., Baker, R. R., Bøgwald, J., et al. (2010). The current status and future focus of probiotic and prebiotic applications for salmonids. Aquaculture 302, 1–18. doi: 10.1016/j.aquaculture.2010.02.007
Merrifield, D. L., and Rodiles, A. (2015). “The fish microbiome and its interactions with mucosal tissues,” in Mucosal Health in Aquaculture, ed. B. H. B. Peatman, (San Diego: Academic Press), 273–295. doi: 10.1016/b978-0-12-417186-2.00010-8
Nayak, S. K. (2010). Role of gastrointestinal microbiota in fish. Aquac. Res. 41, 1553–1573. doi: 10.1111/j.1365-2109.2010.02546.x
Nikouli, E., Meziti, A., Antonopoulou, E., Eleni Mente, E., and Kormas, K. A. (2018). Gut bacterial communities in geographically distant populations of farmed sea bream (Sparus aurata) and Sea Bass (Dicentrarchus labrax). Microorganisms 2018:92. doi: 10.3390/microorganisms6030092
Parris, D. J., Morgan, M. M., and Stewart, F. J. (2019). Feeding rapidly alters microbiome composition and gene transcription in the clownfish gut. Appl. Environ. Microbiol. 85:e02479-18. doi: 10.1128/AEM.02479-18
Pérez, T., Balcázar, J. L., Ruiz-Zarzuela, I., Halaihel, N., Vendrell, D., De Blas, I., et al. (2010). Host-microbiota interactions within the fish intestinal ecosystem. Mucosal. Immunol. 3, 355–360. doi: 10.1038/mi.2010.12
Piazzon, M. C., Calduch-Giner, J. A., Fouz, B., Estensoro, I., Simó-Mirabet, P., Puyalto, M., et al. (2017). Under control: how a dietary additive can restore the gut microbiome and proteomic profile and improve disease resilience in a marine teleostean fish fed vegetable diets. Microbiome 5:164. doi: 10.1186/s40168-017-0390-3
Ramirez, R. F., and Dixon, B. A. (2003). Enzyme production by obligate intestinal anaerobic bacteria isolated from oscars (Astronotus ocellatus), angelfish (Pterophyllum scalare) and southern flounder (Paralichthys lethostigma). Aquaculture 227, 417–426. doi: 10.1016/S0044-8486(03)00520-9
Ray, A. K., Ghosh, K., and Ringø, E. (2012). Enzyme-producing bacteria isolated from fish gut: a review. Aquac. Nutr. 18, 465–492. doi: 10.1111/j.1365-2095.2012.00943.x
Ringø, E., Sperstad, S., Myklebust, R., Refstie, S., and Krogdahl, A. (2006). Characterisation of the microbiota associated with intestine of Atlantic cod (Gadus morhua L.): the effect of fish meal, standard soybean meal and a bioprocessed soybean meal. Aquaculture 261, 829–841. doi: 10.1016/j.aquaculture.2006.06.030
Robbins, L. L., Hansen, M. E., Kleypas, J. A., and Meylan, S. C. (2010). CO2calc: a user-friendly seawater carbon calculator for Windows. Mac OS X and iOS (iPhone). US Geol. Surv. Open File 201:17.
Roeselers, G., Mittge, E. K., Stephens, W. Z., Parichy, D. M., Cavanaugh, C. M., Guillemin, K., et al. (2011). Evidence for a core gut microbiota in the zebrafish. ISME J. 5, 1595–1608. doi: 10.1038/ismej.2011.38
Secretariat of the Convention on Biological Diversity (2014). An updated Synthesis of the Impacts of Ocean Acidification on Marine Biodiversity Montreal, Technical Series 75. eds S. Hennige, J. M. Roberts, and P. Williamson Montreal: Secretariat of the Convention on Biological Diversity. 99.
Sowell, S. M., Norbeck, A. D., Lipton, M. S., Nicora, C. D., Callister, S. J., Smith, R. D., et al. (2008). Proteomic analysis of stationary phase in the marine bacterium “candidatus pelagibacter ubique”. Appl. Environ. Microbiol. 74, 4091–4100. doi: 10.1128/AEM.00599-08
Spor, A., Koren, O., and Ley, R. (2011). Unravelling the effects of the environment and host genotype on the gut microbiome. Nat. Rev. Microbiol. 9, 279–290. doi: 10.1038/nrmicro2540
Sullam, K. E., Essinger, S. D., Lozupone, C. A., O’Connor, M. P., Rosen, G. L., Knight, R., et al. (2012). Environmental and ecological factors that shape the gut bacterial communities of fish: a meta-analysis. Mol. Ecol. 21, 3363–3378. doi: 10.1111/j.1365-294X.2012.05552.x
Tarnecki, A. M., Burgos, F. A., Ray, C. L., and Arias, C. R. (2017). Fish intestinal microbiome: diversity and symbiosis unraveled by metagenomics. J. Appl. Microbiol. 123, 2–17. doi: 10.1111/jam.13415
Thompson, F. L., Iida, T., and Swings, J. (2004). Biodiversity of vibrios. Microbiol. Mol. Biol. Rev. 68, 403–431. doi: 10.1128/MMBR.68.3.403-431.2004
Tirsgaard, B., Moran, D., and Steffensen, J. F. (2015). Prolonged SDA and reduced digestive efficiency under elevated CO2 may explain reduced growth in Atlantic cod (Gadus morhua). Aquat. Toxicol. 158, 171–180. doi: 10.1016/j.aquatox.2014.11.009
Tran, N. T., Wang, G.-T., and Wu, S.-G. A. (2017). Review of intestinal microbes in grass carp Ctenopharyngodon idellus (Valenciennes). Aquac. Res. 48, 3287–3297. doi: 10.1111/are.13367
Turnbaugh, P. J., Ley, R. E., Mahowald, M. A., Magrini, V., Mardis, E. R., and Gordon, J. I. (2006). An obesity-associated gut microbiome with increased capacity for energy harvest. Nature 444:1027. doi: 10.1038/nature05414
Uren Webster, T. M., Consuegra, S., Hitchings, M., and Garcia de Leaniz, C. (2018). Interpopulation variation in the Atlantic salmon microbiome reflects environmental and genetic diversity. Appl. Environ. Microbiol. 84:e00691-18. doi: 10.1128/AEM.00691-18
Vandenberghe, J., Thompson, F. L., Gomez-Gil, B., and Swings, J. (2003). Phenotypic diversity amongst Vibrio isolates from marine aquaculture systems. Aquaculture 219, 9–20. doi: 10.1016/S0044-8486(02)00312-5
Wang, A. R., Ran, C., Ringø, E., and Zhou, Z. G. (2018). Progress in fish gastrointestinal microbiota research. Rev. Aquac. 10, 626–640. doi: 10.1016/j.dci.2017.02.024
Wang, Q., Garrity, G. M., Tiedje, J. M., and Cole, J. R. (2007). Naïve bayesian classifier for rapid assignment of rRNA sequences into the new Bacterial Taxonomy. Appl. Environ. Microbiol. 73, 5261–5267. doi: 10.1128/AEM.00062-07
Wietz, M., Wemheuer, B., Simon, H., Giebel, H.-A., Seibt, M. A., Daniel, R., et al. (2015). Bacterial community dynamics during polysaccharide degradation at contrasting sites in the Southern and Atlantic Oceans. Environ. Microbiol. 17, 3822–3831. doi: 10.1111/1462-2920.12842
Wright, E. S., Yilmaz, L. S., and Noguera, D. R. (2012). DECIPHER, A search-based approach to chimera identification for 16S rRNA sequences. Appl. Environ. Microbiol. 78, 717–725. doi: 10.1128/AEM.06516-11
Keywords: sea bream, intestinal, microbiota, ocean acidification, Vibrio
Citation: Fonseca F, Cerqueira R and Fuentes J (2019) Impact of Ocean Acidification on the Intestinal Microbiota of the Marine Sea Bream (Sparus aurata L.). Front. Physiol. 10:1446. doi: 10.3389/fphys.2019.01446
Received: 08 August 2019; Accepted: 08 November 2019;
Published: 28 November 2019.
Edited by:
Joy Becker, The University of Sydney, AustraliaReviewed by:
Tiziano Verri, University of Salento, ItalyEduardo Sampaio, Marine and Environmental Sciences Centre (MARE), Portugal
Copyright © 2019 Fonseca, Cerqueira and Fuentes. This is an open-access article distributed under the terms of the Creative Commons Attribution License (CC BY). The use, distribution or reproduction in other forums is permitted, provided the original author(s) and the copyright owner(s) are credited and that the original publication in this journal is cited, in accordance with accepted academic practice. No use, distribution or reproduction is permitted which does not comply with these terms.
*Correspondence: Juan Fuentes, amZ1ZW50ZXNAdWFsZy5wdA==