- 1Institute of Sport Science, Friedrich Schiller University of Jena, Jena, Germany
- 2Department of Statistics, University of Bonn, Bonn, Germany
A stable gait pattern is a prerequisite to successfully master various activities of daily living. Furthermore, reduced gait stability is associated with a higher risk of falling. To provide specific intervention strategies to improve gait stability, gaining detailed knowledge of the underlying mechanism and influencing factors is of utmost importance. The effects of relevant influencing factors on gait stability are poorly examined, yet. Therefore, the aim of the current study was to quantify the effects of various influencing factors on gait stability. In a cross-sectional study, we assessed dynamic gait stability and relevant influencing factors in 102 older adults (age >65 years). In addition to dynamic gait stability (largest Lyapunov exponent [LLE] and gait variability measures) during normal over-ground (single-task: ST) and dual-task (DT) walking, we registered the following influencing factors: health status (SF12), pain status (painDETECT, SES), fear of falling (falls efficacy scale), depression (CES-D), cognition performance (Stroop test), physical activity (Freiburger Fragebogen zur körperlichen Aktivität), proprioception (joint position sense), peripheral sensation (mechanical and vibration detection threshold), balance performance (static balance on force plate) and muscular fitness (instrumented sit-to-stand test). We used a principal components regression to link the identified principal components with the gait stability and gait variability responses. The four principal components “strength and gender” (e.g., p = 0.001 for LLE during ST), “physical activity” (e.g., p = 0.006 for LLE during ST), “pain” (e.g., p = 0.030 for LLE during DT) and “peripheral sensation” (e.g., p = 0.002 for LLE during ST) were each significantly associated with at least two of the analyzed gait stability/variability measures. The dimension “balance” was a significant predictor in only one gait measure. While “proprioception” tends to correlate with a gait variability measure, we did not find a dependency of mental health on any gait measure. In conclusion, the participants' ability to recover from small perturbations (as measured with the largest Lyapunov exponent) seems to be related to gender and strength, the amount of physical activity the participants spent every week, peripheral sensation and pain status. Since the explained variance is still rather low, there could be more relevant factors that were not addressed, yet.
Introduction
Falls in the elderly occur quite frequently. 30–60% of older adults fall at least once a year (Rubenstein, 2006; Inouye et al., 2009) and up to 20% of falls result in injuries (Rubenstein, 2006), hospitalization or death in older adults (Rubenstein, 2006; Pfortmueller et al., 2014). Western health care systems spend 0.85 to 1.5% of their total health care expenditures (Heinrich et al., 2010) on the consequences of falls, rendering this issue socio-economically relevant. Considering the effect on the individual's quality of life, the prevention of falls is of utmost importance.
Risk factors for falls and fall-related injuries can be categorized into (I) environmental risk factors, (II) behavioral risk factors, (III) biological risk factors, and (IV) socioeconomic risk factors. However, besides standing quietly and sitting down, walking is one of the most common daily activities leading to falls (Robinovitch et al., 2013). Thus, it is not surprising that an unstable gait pattern is associated with a higher risk of falling in older adults (Hamacher et al., 2011; Bruijn et al., 2013). In addition, walking is a prerequisite to handle a wide range of daily activities (Bramble and Lieberman, 2004). Since gait measures are predictors of mobility (Brach et al., 2007), a stable gait also ensures social participation not only in the elderly. This highlights the importance of a stable gait in various contexts.
According to the literature, age is a strong predictor of falls (Lord et al., 2003; Ambrose et al., 2013; Pfortmueller et al., 2014) as well as of an unstable gait (Terrier and Reynard, 2015). Other (predominantly age-related) intrinsic determinants are frequently discussed as risk factors for falls. These intrinsic determinants include gender, muscle strength or muscle power, balance, peripheral sensation (proprioception, vibration sense, tactile sensitivity), cognition, and diseases, such as diabetes mellitus (Lord et al., 2003; Ambrose et al., 2013; Pfortmueller et al., 2014). Even depression seems to be a factor related to an altered gait pattern and the probability of falling (Paleacu et al., 2007; Ambrose et al., 2013). Furthermore, gait characteristics (Ambrose et al., 2013) and in particular gait variability and gait stability measures (Hamacher et al., 2011; Bruijn et al., 2013) or the ability to dual-task during gait (Ambrose et al., 2013) are also highly associated with the risk of falling. Although this may indicate interactions between the above-mentioned person-related determinants and walking abilities in older adults, the effects of intrinsic factors on gait variability or gait stability itself were barely reported in the literature. Since knowledge of relevant factors affecting a stable gait is a prerequisite to (1) develop and to evaluate specific intervention strategies, (2) improve gait stability, (3) reduce the number of falls, or (4) ensure save social participation in older adults, we aimed to assess the effects of intrinsic factors associated with falling on gait stability and gait variability as well as on dual-task walking in older adults.
In general, exercise interventions are capable to improve gait variability (Wollesen et al., 2015) and, furthermore, an improved gait pattern enhances physical functioning, physical activity and social participation (VanSwearingen et al., 2011). However, to be able to deduce individually adjusted fall prevention programs (Pfortmueller et al., 2014) that are more efficient and to better understand the underlying mechanisms of gait stability, influencing factors must be identified.
In a broader sense, a stable gait is a gait pattern that does not lead to falls. There are different types of gait stability (Bruijn et al., 2013): for example, dealing with small internal (e.g., neuromuscular noise) and external perturbations (e.g., surface friction) during normal overground walking or recovering from larger perturbations (e.g., a trip or a slip). This study will focus on the former type. Here, regarding fall risk, variability measures and the largest Lyapunov exponent depict the best construct, predictive, and convergent validity (Bruijn et al., 2013) and are, thus, chosen for this study.
Therefore, the aim of the current study was to explore the effect of all above-mentioned intrinsic risk factors on gait stability and gait variability during normal and dual-task walking in older adults. Additionally, factors that interfere with a stable gait, e.g., pain (Hamacher et al., 2016), osteoarthritis and having a joint replacement (Yakhdani et al., 2010; Hamacher, 2014), were also considered.
Materials and Methods
Study Design and Participants
In a cross-sectional study, 102 (52 female and 50 male) healthy older adults with a mean age of 72 years (SD = 4.5 years) and a mean body mass index of 27 (SD = 3.6) were recruited using a newspaper announcement. Inclusion criteria were an age of at least 65 years and the ability to walk for 10 min without any aids. Acute neurological, orthopedic or cardiovascular diseases lead to exclusion. Participants with common age-related diseases, such as diabetes mellitus, osteoarthritis, high blood pressure or an implanted prosthesis, were included in the study. This study was carried out in accordance with the recommendations of the Declaration of Helsinki with written informed consent from all subjects. The protocol was approved by the Ethical Commission of the Faculty of Social and Behavioral Sciences, Friedrich Schiller University of Jena (no. FSV 16/05).
Testing Procedure
Each participant came at 2 different days within 2 weeks to complete the tests. At the first test day, a standardized gait analysis was conducted. At the second test day, influencing factors were registered. In total, all tests lasted 3.5 h. A summary of all outcomes is given in Tables 1, 2.
Anamnesis
To check the inclusion and exclusion criteria, diseases, motor-functional complaints, and medication were registered. The participants were explicitly asked if they have diabetes mellitus, osteoarthritis in any joint of the lower extremities or any kind of prosthesis at the lower extremities. To be able to better describe our cohort, we also asked the participants how frequently they have fallen (while walking or standing) within the last 12 months.
Gait Analysis
To assess gait parameters, a standardized gait analysis was conducted in an empty sports hall. Thereto, inertial sensors (MTw2, Xsens Technologies B.V., Enschede, The Netherlands, range of measurement of angular velocity: ±1,200 deg/s, sampling rate: 100 Hz) were attached to the dominant forefoot with tape and to the thorax (strap underneath the arms, thus sensor at upper thoracic spine) with an elastic strap. The dominant foot was identified using the German version of the Lateral Preference Inventory (Ehrenstein and Arnold-Schulz-Gahmen, 1997). To improve the reliability of gait measures, the participants walked on a 25 m track up and down once with their comfortable walking pace to familiarize to the test setup (Hamacher et al., 2017). Thereafter, the participants completed the following conditions in randomized and balanced order: (a) Motor single-task condition: walking up and down the 25 m track with their comfortable walking pace for 4 min; (b) Dual-task condition: Walking with comfortable walking pace while reciting serial three subtractions (starting from a random three-digit number) for 4 min.
From the kinematic walking time series, the following gait parameters were calculated: stride length and stride time as well as the intra-individual stride-to-stride variability (standard deviations) of stride length and stride time as measures of gait variability. The reliability of the measurement system is verified (Hamacher et al., 2014).
As a measure of local dynamic gait stability (LDS), the short-time largest Lyapunov exponent was determined for foot and trunk kinematics separately using an evaluated algorithm (Hamacher et al., 2015). Since for normal overground walking, the highest effects comparing young vs. older adults were observed when analyzing time series derived from three-dimensional angular velocity data of the foot (Hamacher et al., 2015), we used those time series, too. For each participant, the three-dimensional angular velocity data of 100 strides were time-normalized to 10,000 samples, resulting in an average of 100 samples per stride. Thereafter, a state space was built upon on the time-normalized data using the method of time-delayed embedding. The time delay and embedded dimension were chosen based on the first minimal mutual information (Fraser and Swinney, 1986) and the false nearest neighbors analysis (Kennel et al., 1992), respectively. A fixed time-delay τ (mean across all participants: τfoot = 9, τtrunk = 11) and embedded dimension dE (maximum across all participants: dEfoot = dEtrunk = 12) was used for all participants. The largest Lyapunov exponent was calculated using Rosenstein and coworkers' algorithm (Rosenstein et al., 1993). Thereto, the Euclidean distances of each point in state space of initially nearest neighbors were tracked in time and the mean of the logarithm of this divergence curves was calculated. The largest Lyapunov exponent was defined as the slope of the linear fit through approximately 0–0.5 strides. Larger values indicate lower local dynamic gait stability. The largest Lyapunov exponent quantifies the ability of a dynamic system (human gait) to recover from small perturbations (Bruijn et al., 2013).
Compared to the gait variability measures, the largest Lyapunov exponent depict a slightly better construct validity (Bruijn et al., 2013). Therefore, the outcomes local dynamic stability (LDS) of trunk and foot during single-task and dual-task walking were considered primary criterions to be predicted by the assumed influencing outcomes. In a secondary analysis, the gait variability parameters SD of stride length and SD of stride time during single-task and dual-task walking were analyzed.
Muscular Fitness
To measure muscular power of the lower extremities, the sit-to-stand transfer has already been successfully conducted in older adults (Lindemann et al., 2003; Zech et al., 2011; Zijlstra et al., 2012). We used an instrumented version to assess muscular power (Zijlstra et al., 2010). Thereto, an inertial sensor (MTw2, Xsens Technologies B.V., Enschede, The Netherlands, sampling rate: 100 Hz) was fixed to the back of the pelvis. Compared to a force plate based approach, power calculated from inertial sensor data fixed to the pelvis depict high correlations (r = 0.95 for fast movements, Zijlstra et al., 2010). The participants were placed on the front part of a chair (height: 0.47 m, no armrests, arms crossed over the chest). They were asked to stand up as fast as possible. The participants were asked to sit/stand motionless immediately before and after the sit-to-stand transition. As described below, this was used as a boundary condition for parameter calculation.
Using the sensors orientation (quaternions) and the three-dimensional accelerometer data, the vertical component of the acceleration data was extracted, and gravitational acceleration was removed by subtracting 9.81 m/s2. Vertical movement velocity was calculated by numerical integration (Heun's method). Prior to and after the sit-to-stand transition, the movement velocity is considered zero. Any deviations from zero (e.g., due to the numerical integration) were removed by subtracting a straight line which was fitted through two points: the pre-test and the post-test vertical velocity value. The Power was calculated as the arithmetic product of the vertical movement velocity, gravitational acceleration g, and the body weight. Power was then normalized to the subject's body weight. Based on the resulting power-time curve, peak power and mean power (mean power during the sit-to-stand transition where the vertical movement velocity was at least 0.1 m/s) were calculated as outcomes.
Balance
Balance performance was assessed during double-leg stance (feet together) and semi-tandem stance (toe of the dominant foot slightly touching the contralateral heel) on a force platform (type 9260AA6, Kistler Instrumente GmbH, Winterthur, Swiss). During the test, the participants did not wear shoes but socks. The two stance conditions were completed with eyes open and eyes closed for 30 s each stance condition. In all stance conditions, hands were held on hips. During the open eyes conditions, the participants were asked to look at a cross placed at eye level 1 m in front of the participant. With the aid of the MARS software (Kistler Instrumente GmbH, Sindelfingen, Germany) the mean velocity of the two-dimensional velocity of the center of pressure was calculated for each balance condition.
Pain
The pain status was assessed using the painDETECT questionnaire and the Pain Experience Scale (German: Schmerzempfindungsskala, SES). The painDETECT questionnaire quantifies the neuropathic component of pain. A higher score depicts a higher likelihood of neuropathic pain being present (Freynhagen et al., 2006).
The SES assesses the (a) affective and (b) sensory dimension of pain. Furthermore, information on (c) rhythmicity, (d) local depth, and (e) temperature are quantified as sub-scales of the sensory dimension of pain (Geissner, 1995).
Cognition
The Color-Word Interference Test (Stroop) is a frequently used test to quantify executive functioning. More specifically, the selective allocation of attention is rated (Lamers et al., 2010). The participants (a) must read color names, (b) name ink colors (no writings), and (c) name ink colors of words. However, in this last condition, the ink color and the color words do not match (incongruent stimulus). As outcomes, we used the time needed for condition c (incongruent stimulus) as well as the time difference of conditions b & c (time costs of the incongruent stimulus). Both outcomes are commonly assessed (Uttl and Graf, 1997).
Fear of Falling
We rated fear of falling using the Falls Efficacy Scale International (FES-I) (Dias et al., 2006). The questionnaire assesses the subjective fear to fall during various common daily activities. The German version of the FES-I was validated and depict good quality criteria (Delbaere et al., 2010). As the outcome, a single value reflecting the amount of fear of falling was calculated.
Depression
To register depression symptoms, we deployed the German version of the Center for Epidemiologic Studies-Depression Scale (CES-D) for screening purposes. This validated questionnaire is suitable for a self-assessment of depressive symptoms in the general population (Meyer and Hautzinger, 2001; Lehr et al., 2008). High outcome scores indicate a high probability of having a depression.
Health Status
The overall health status was assessed with the 12-Item Short-Form Health Survey (SF-12 Ware et al., 1996). Based on that 12 items a physical component summary, as well as a mental health summary score, were analyzed. High scores are interpreted as a good health status.
Peripheral Sensation
Mechanical and vibration detection thresholds were assessed as described in the “quantitative sensory testing” battery (Rolke et al., 2006). To test the mechanical detection threshold, we used Frey filaments (0.5, 1, 2, 4, 8, 16, 32, 64, 128, 256, 512 mN; MARSTOCKnervtest, Schriesheim, Germany). The force was applied to the lateral malleolus of the dominant foot. A series of descending, and ascending stimulus intensities was applied. The threshold was calculated as the geometric mean from five repetitions.
To test the vibration detection threshold, the Rydel–Seiffer graded tuning fork (64 Hz, 8/8 scale) was applied at a proximal elevation of the tibia (tibia tuberositas). The participants were asked to indicate once they did not feel any vibrations anymore. The threshold was then defined as the mean of three repetitions.
Proprioception
Proprioception was tested with an inertial sensor-based reproduction test using an active-active procedure as described by Arvin et al. (2015). To measure the knee angle in real-time using an in-house software, inertial sensors (MTw2, Xsens Technologies B.V., Enschede, The Netherlands, sampling rate: 100 Hz) were fixed to the dominant shank (medial and distal to the tibia tuberositas) and to the iliotibial tract at the middle of the thigh. The participants stood on a wooden platform keeping their eyes closed. The participants were then asked to slowly flex their knee. The examiner said “stop” once a knee flexion angle of about 40° was reached. Deviations from the target angle up to ±5° were allowed and not corrected to reduce fatiguing effects in the older adults. The participants memorized the knee angle, returned to the start position and reproduced that angle. The mismatch error was registered. This procedure was repeated 10 times, but the first two times were considered learning trials and, thus, not analyzed. As outcomes, we calculated the mean of the absolute error and the standard deviation of the signed error.
Physical Activity
The “Freiburger Fragebogen zur körperlichen Aktivität” (FFkA Frey et al., 1999) is a validated instrument to register health-related physical activity (PA, in hours per week) during the last seven days. Since physical activities generally follow a yearly seasonal pattern (Cepeda et al., 2018), we adjusted, where appropriate, for this seasonal component using a sine/cosine regression with a yearly period. This seasonal regression model is often used in time series analysis and allows for an accurate description (R2 = 0.99) of the yearly PA mean pattern of old-elderly adults (aged ≥75 years) reported in Figure 1 of Cepeda et al. (2018). The data used to build and validate the seasonal regression model was extracted from Figure 1 in Cepeda et al. (2018) using the open source software Engauge Digitizer (version 10.7 by Mark Mitchell).
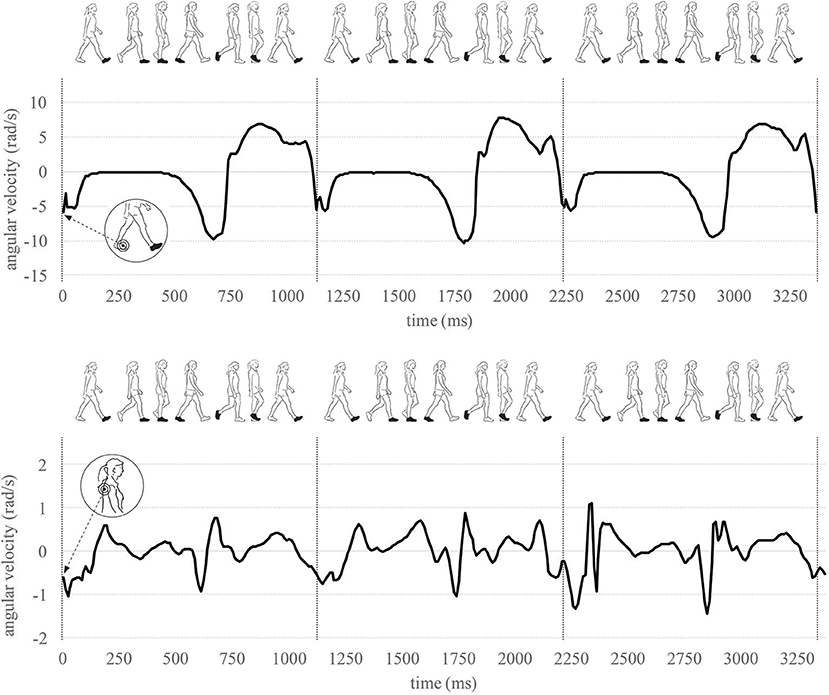
Figure 1. Sensor placement at the foot and trunk. As an example, one part of the sensor signal across three strides is illustrated.
Statistics
The number of predictor variables (Table 2) was relatively large in comparison to the sample size and collinearities between predictor variables would cause variance inflations in the estimators of a classical regression analysis. Therefore, we used a principal component regression (PCR) analysis to reduce the dimension of the predictor space, to gain orthogonalized predictors and to link the identified principal components (PC) with the gait stability responses (primary outcomes) as well as the gait variability responses (secondary outcomes, Table 4). The number of factors was determined using the Kaiser criterion (percentage of variance explained: 75.3%).
To facilitate the interpretation, we used VARIMAX-rotated PCs and considered their largest (≥0.5) standardized factor loadings (Table 3). Gender and muscular fitness are included in the same factor (PC three). To get further insights into the relationship of gender and muscular fitness with the response variables, we analyzed the correlations (Pearson's r) between each of the muscular fitness outcomes (S2SPeakV and S2SMeanV) and each of the gait stability and gait variability outcomes separately for male and female participants (Table 5). Additionally, we tested for gender differences in the gait stability and gait variability outcomes using t-tests for independent samples (Table 6).
Results
For 90 participants, all outcome measures were analyzed. Test data of 12 participants could not be included due to technical problems of the inertial sensor system (n = 5) or some participants did not want to answer all questionnaires (n = 7). Since only 9 participants self-reported having diabetes, this factor was not included in the statistical analysis. Of the 90 participants analyzed, 41 (46%) reported having at least one fall and 19 (21%) having two falls within the last 12 months.
Pronounced seasonal patterns were found and corrected for the overall PA [F(2, 99) = 8.62, p < 0.001], the basic PA [F(2, 99) = 13.85, p < 0.001], and the sports-related PA [F(2, 99) = 3.55, p = 0.03]. No significant seasonal pattern was found for the extracurricular PA.
The results of the principal components analyses are displayed in Table 3. Out of the 31 outcome measures, 10 factors were extracted in total: (1) “pain,” (2) “balance,” (3) “strength and gender,” (4) “physical activity,” (5) “cognition,” (6) “proprioception,” (7) “mental health,” (8) “Osteoarthritis and prosthesis,” (9) “BMI,” and (10) “peripheral sensation.”
The results of the regression analysis are depicted in Table 4. The factor “strength and gender” is a significant predictor for LDS foot (LDSfoot, ST: p = 0.001, LDSfoot, DT: p = 0.050) and trunk (LDStrunk, ST: p = 0.001, LDStrunk, DT: p < 0.001). A higher relative muscular fitness and/or being male correlated with a lower largest Lyapunov exponent (better LDS). Furthermore, more physical activity improved LDS of the foot during single (p = 0.006) and dual-task walking (p = 0.040) as well as to LDS of the trunk during single task walking (p = 0.007). A low peripheral sensation diminished the foot LDS (LDSfoot, ST: p = 0.002, LDSfoot, DT: p < 0.001) but there was not even a trend regarding the LDS of the trunk. BMI (LDSfoot, ST: p = 0.043), pain (LDSfoot, DT: p = 0.030) and the factor “Osteoarthritis and prosthesis” (LDStrunk, DT: p = 0.006) were each a significant predictor but only in one model. Good balance abilities (p = 0.094) and a lower BMI (p = 0.075) tended to improve LDSfoot, DT.
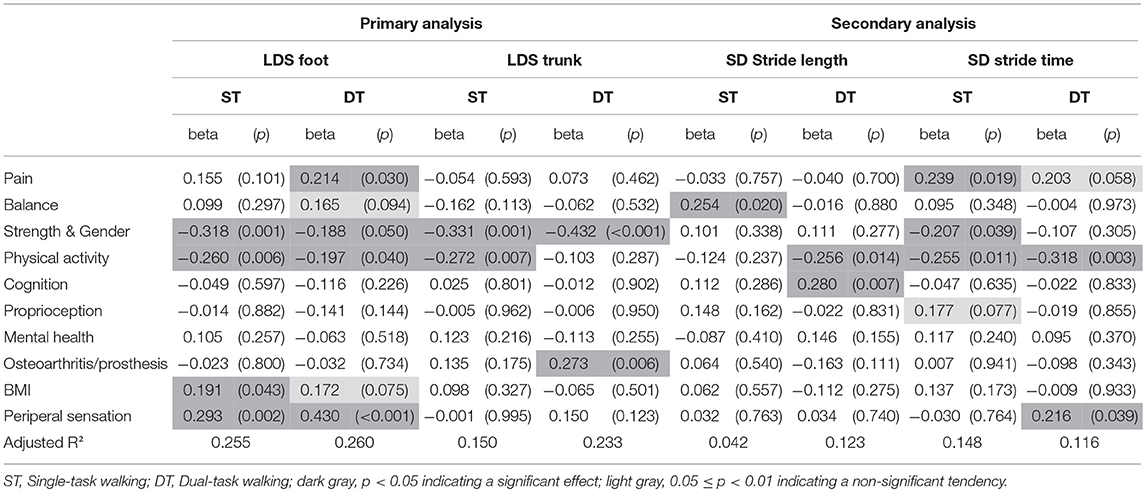
Table 4. Regression analyses were used to link the identified principal components (Table 3) with the gait stability responses (primary outcomes) and the gait variability responses (secondary outcomes).
Within the secondary analysis, Stride-to-stride gait variability was analyzed. Again, “strength and gender” (SDStrideTime, ST: p = 0.039) and “physical activity” (SDStrideLength, DT: p = 0.014, SDStrideTime, ST: p = 0.011, SDStrideTime, DT: p = 0.003,) were significant predictors. “Balance” (SDStrideLength, ST: p = 0.020), “pain” (SDStrideTime, ST: p = 0.019), “cognition” (SDStrideLength, DT: p = 0.007) and “peripheral sensation” (SDStrideTime, DT: p = 0.039) were only in one model of the secondary analysis significant predictors. Good “proprioception” or less “pain” tended to improve SDStrideTime, ST (p = 0.077) and SDStrideTime, DT (p = 0.058), respectively.
Knowing, that muscular fitness and gender were included into one factor, we determined the relation of muscular fitness (outcomes S2SPeakV and S2SMwV) with the gait stability and gait variability outcomes for men and women, separately (Table 5). For men, a higher muscular fitness improveed LDSfoot, ST (S2SPeakV: r = −0.33, p = 0.010), LDSfoot, DT (S2SPeakV: r = −0.30, p = 0.019) and SDStrideLength, ST (S2SPeakV: r = −0.24, p = 0.049; S2SMeanV: r = −0.25, p = 0.043). Furthermore, S2SPeakV (LDStrunk, DT: r = −0.21, p = 0.077) and S2SMeanV (LDSfoot, ST: r = −0.23, p = 0.052; LDSfoot, DT: r = −0.19, p = 0.092) tended to increase LDS. For women, SDStrideTime, ST was correlated with S2SPeakV (r = −0.36, p = 0.005) and S2SMeanV (r = −0.40, p = 0.002). LDStrunk, DT (S2SMeanV: r = −0.23, p = 0.059) tended to be correlated with muscular fitness measures.
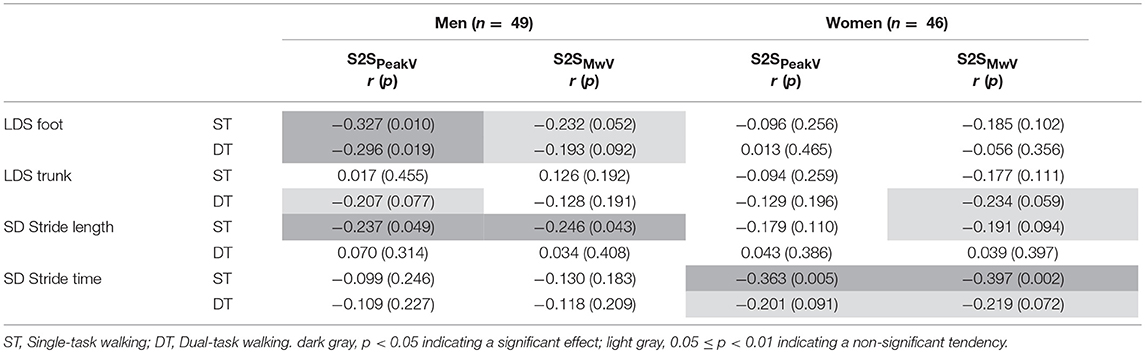
Table 5. The correlations (Pearson's r) between each of the muscular fitness outcomes (S2SPeakV and S2SMeanV) and each of the gait stability and gait variability outcomes were separately assessed for male and female participants.
Gait stability and gait variability measures of male vs. female participants are given in Table 6. Men depicted better LDS of foot (LDSfoot, ST: p = 0.017, d = 0.48; LDSfoot, DT: p = 0.029, d = 0.45) and trunk (LDStrunk, ST: p < 0.001, d = 0.82; LDStrunk, DT: p < 0.001, d = 0.99) but higher gait variability (SDStrideLength, ST: p < 0.001, d = 0.85) than women.
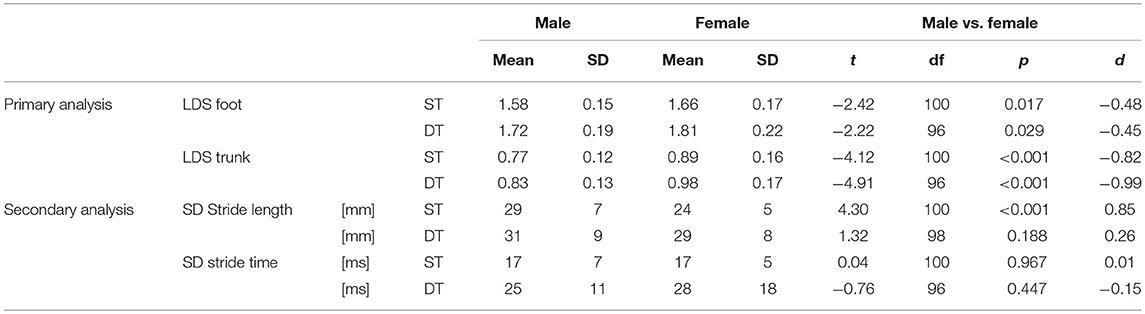
Table 6. We tested for gender differences in the gait stability and gait variability outcomes using t-tests for independent samples (ST, single-task walking; DT, Dual-task walking).
Discussion
The aim of the cross-sectional study was to explore influencing intrinsic factors on local dynamic gait stability and gait variability in an older population. The four dimensions (factors of a principal component analysis) (1) “strength and gender,” (2) “physical activity,” (3) “pain,” and (4) “peripheral sensation” were each associated with at least two of the analyzed gait stability/variability measures. Dimension (5) “balance” was a significant predictor in only one gait measure. While dimension (6) “proprioception” tends to correlate with a gait variability measure, we did not find a dependency of mental health on any gait measure. Hereafter, we will discuss these dimensions one after another:
1) The results suggest that participants with higher relative muscle performance or men walk more stable. Since both muscular fitness (sit-to-stand test) and gender were merged into one factor, we analyzed their individual contributions on gait stability and gait variability, separately. Comparing male and female participants, we observed significant differences in gait stability. Regarding the stability measures of the primary analysis, men walk more stable than women. This could be a reason why women are more likely to fall (WHO, 2008; Robinovitch et al., 2013). Despite this, the correlation of relative muscle performance with these primary measures was, on the one hand, stronger in men than in women regarding the gait stability measures but on the other hand, more pronounced in women as compared to men regarding the gait variability measures. Overall, the strongest correlations depicted only medium effects. The gait measures used in the current study reflect the system's capacity to recover from small perturbations (i.e., neuromuscular noise and wind, Bruijn et al., 2013). However, other types of stability, such as the recovery from larger perturbations (e.g., after tripping), might require more strength. This would also explain the comparatively low effect sizes of the correlation analysis. This result suggests that lower extremity muscular fitness is less relevant for LDS but could be more relevant for other kinds of gait stability (e.g., recovery from larger perturbations). This is in line with the recommendation to include strength exercises into fall prevention programs (Sherrington et al., 2011).
2) The amount of physical activity is also a strong predictor of most gait stability and gait variability measures during both, single-task and dual-task walking. This finding is in agreement with the broad evidence for beneficial effects of regular physical activity for enhancing and maintaining older adults' fitness as well as mental and physical health-related quality of life (Taylor et al., 2004; Netz et al., 2005; Nelson et al., 2007).
3) In our study, pain predicted a few gait outcomes. This is in line with previously reported data (Hamacher et al., 2016). In the paper of Hamacher et al. dual-task costs of gait depended on pain severity. In fact, pain is known to affect muscle activity and biomechanical behavior (Hodges, 2011) and pain disrupt cognitive functions and executive control (Keogh et al., 2013). Thus, it is surprising that the effects of pain on gait stability or gait variability were not more pronounced. A reason could be that the pain questionnaires were not assessed during the test day of the gait analysis which could have reduced the measured effect of pain on gait stability or variability measures.
4) Peripheral sensation was a significant predictor of foot LDS. In another study, touch and vibration sense were correlated with static balance performance (Lord et al., 1991) confirming our results. Interestingly, peripheral sensation did primarily effect foot LDS but not trunk LDS. In further studies, phase-dependent local dynamic stability (Ihlen et al., 2015) should be used to reveal if such effects are restricted to the stance or swing phase of gait.
5) We did not reveal any significant effect of balance in the primary analysis and only one within the secondary analysis. This is surprising since, in most studies, balance deficits being discussed to be a relevant risk factor for falls (Lord et al., 2003; Ambrose et al., 2013; Pfortmueller et al., 2014) and exercises to improve balance have been suggested to be included into fall prevention programs (Sherrington et al., 2008, 2011). Our results could imply that (static) balance is not that important for gait as it is for preventing falls in general (e.g., during quiet standing). It is known that posture control concepts are fundamentally different for standing and walking (Winter, 1995). Furthermore, it is known, that balance abilities are context-specific (Sibley et al., 2015; Kümmel et al., 2016). Our result could also be a limitation of the methods chosen. We only assessed static balance measures but no dynamic balance parameters and it is known that the predictive value for falls depends on the method (Muir et al., 2010) and that there is a high intrasubject variability in individual concepts maintaining postural stability (Pasma et al., 2014).
6) We did not find a significant dependency of the active joint position sense on gait stability or gait variability. This is in contrast with the result of Lord et al. (1991), who reported a relation of proprioception and static and dynamic balance measures. Non-questionable, proprioception plays a key role in motor control and functional joint stability (Riemann and Lephart, 2002; Proske and Gandevia, 2012) but the quality criteria (reliability and validity) have been questioned, in general (Riemann et al., 2002; Benjaminse et al., 2009; Hillier et al., 2015). Low quality criteria could explain the missing relationship between proprioception and gait stability or gait variability.
Overall, the explained variance of the regression model is rather low (adjusted R2 range from 0.04 to 0.26). In models predicting static or dynamic balance, the multiple R ranged from 0.24 to 0.37 (Lord et al., 1991) which would be an R2 of 0.06 to 0.14. These R2 values are comparable to ours (between 0.15 and 0.26 for the models predicting LDS of the foot and trunk, Table 4). However, the low explained variance highlights the fact that there could be more relevant factors for gait stability and gait variability (as well as for balance) that were not addressed, yet. However, a strength of our study is the large number of considered parameters. Furthermore, the study sample seems to be sufficient to reveal effects relevant to the practice. On the other hand, this is a cross-sectional study. Thus, the results should be confirmed with experimental designs, for example with intervention studies designed to improve gait stability. Additionally, we did not assess vision, vestibular functioning, peripheral nerve tests and reflexes or Vitamin D deficiency and other variables that also have been discussed to be relevant risk factors for falls (Tinetti et al., 1988; Lord et al., 2003; Ambrose et al., 2013; Pfortmueller et al., 2014). At last, we assessed the participants' ability to recover from small perturbations. There are other types of gait stability that should be addressed in further studies.
In conclusion, the participants' ability to recover from small perturbations (as measured with the largest Lyapunov exponent) seems to be related to (1) gender and muscular fitness, (2) the amount of physical activity the participants spent every week, (3) peripheral sensation (mechanical and vibration detection threshold), and (4) pain status. No or minor effects were found for balance, proprioception, cognition or mental health. Since the explained variance is still rather low, there could be more relevant factors that were not addressed, yet.
Author Contributions
DH and AZ planned and designed the study. DH, CH, VH, CK, and TT collected the data. DL and DH analyzed the data. DH, DL, and AZ drafted the manuscript. All authors critically revised the manuscript. All authors approved the final version of the manuscript.
Conflict of Interest Statement
The authors declare that the research was conducted in the absence of any commercial or financial relationships that could be construed as a potential conflict of interest.
Acknowledgments
We would like to thank all the participants of our study. Furthermore, we would like to thank Elke Rieger for helping with the graphics.
References
Ambrose, A. F., Paul, G., and Hausdorff, J. M. (2013). Risk factors for falls among older adults: a review of the literature. Maturitas 75, 51–61. doi: 10.1016/j.maturitas.2013.02.009
Arvin, M., Hoozemans, M. J., Burger, B. J., Verschueren, S. M., van Dieën, J. H., and Pijnappels, M. (2015). Reproducibility of a knee and hip proprioception test in healthy older adults. Aging Clin. Exp. Res. 27, 171–177. doi: 10.1007/s40520-014-0255-6
Benjaminse, A., Sell, T. C., Abt, J. P., House, A. J., and Lephart, S. M. (2009). Reliability and precision of hip proprioception methods in healthy individuals. Clin. J. Sport Med. 19, 457–463. doi: 10.1097/JSM.0b013e3181bcb155
Brach, J. S., Studenski, S. A., Perera, S., VanSwearingen, J. M., and Newman, A. B. (2007). Gait variability and the risk of incident mobility disability in community-dwelling older adults. J. Gerentol. 62A, 983–988. doi: 10.1093/gerona/62.9.983
Bramble, D. M., and Lieberman, D. E. (2004). Endurance running and the evolution of homo. Nature 432, 345–352. doi: 10.1038/nature03052
Bruijn, S. M., Meijer, O. G., Beek, P. J., and Van Dieën, J. H. (2013). Assessing the stability of human locomotion: a review of current measures. J. R. Soc. 10:20120999. doi: 10.1098/rsif.2012.0999
Cepeda, M., Koolhaas, C. M., van Rooij, Frank, J. A., Tiemeier, H., Guxens, M., Franco, O. H., et al. (2018). Seasonality of physical activity, sedentary behavior, and sleep in a middle-aged and elderly population: the Rotterdam study. Maturitas 110, 41–50. doi: 10.1016/j.maturitas.2018.01.016
Delbaere, K., Close, J. C., Mikolaizak, A. S., Sachdev, P. S., Brodaty, H., and Lord, S. R. (2010). The Falls Efficacy Scale International (FES-I). A comprehensive longitudinal validation study. Age Ageing 39, 210–216. doi: 10.1093/ageing/afp225
Dias, N., Kempen, G. I. J. M., Todd, C. J., Beyer, N., Freiberger, E., Piot-Ziegler, C., et al. (2006). Die Deutsche Version der Falls Efficacy Scale-International Version (FES-I) [The German version of the Falls Efficacy Scale-International Version (FES-I)]. Z Gerontol Geriatr. 39, 297–300. doi: 10.1007/s00391-006-0400-8
Ehrenstein, W. H., and Arnold-Schulz-Gahmen, B. E. (1997). Seitenbevorzugung von Auge, Ohr, Hand und Fuß. Available online at: http://www.sport.uni-mainz.de/physio/FragebogenIfADoVI04.html (Accessed June 26, 2018).
Fraser, A., and Swinney, H. (1986). Independent coordinates for strange attractors from mutual information. Phys. Rev. A 33, 1134–1140. doi: 10.1103/PhysRevA.33.1134
Frey, I., Berg, A., Grathwohl, D., and Keul, J. (1999). Freiburger Fragebogen zur körperlichen Aktivität-Entwicklung, Prüfung und Anwendung. Sozial- und Präventivmedizin 44, 55–64. doi: 10.1007/BF01667127
Freynhagen, R., Baron, R., Gockel, U., and Tölle, T. R. (2006). painDETECT: a new screening questionnaire to identify neuropathic components in patients with back pain. Curr. Med. Res. Opin. 22, 1911–1920. doi: 10.1185/030079906X132488
Geissner, E. (1995). Die Schmerzempfindungsskala SES. Ein differenziertes und veränderungssensitives Verfahren zur Erfassung chronischer und akuter Schmerzen [The Pain Perception Scale–a differentiated and change-sensitive scale for assessing chronic and acute pain]. Rehabilitation 34, XXXV–XLIII.
Hamacher, D. (2014). Evaluation von Augmented Feedback in der Gangschulung bei Patientinnen nach totalendoprothetischer Versorgung des Hüftgelenks. Hamburg: Kovač.
Hamacher, D., Hamacher, D., Krowicki, M., and Schega, L. (2017). Between-day test-retest reliability of gait variability in older individuals improves with a familiarization trial. Aging Clin. Exp. Res. 29, 327–329. doi: 10.1007/s40520-016-0536-3
Hamacher, D., Hamacher, D., Singh, N. B., Taylor, W. R., and Schega, L. (2015). Towards the assessment of local dynamic stability of level-grounded walking in an older population. Med. Eng. Phys. 37, 1152–1155. doi: 10.1016/j.medengphy.2015.09.007
Hamacher, D., Hamacher, D., Taylor, W. R., Singh, N. B., and Schega, L. (2014). Towards clinical application: repetitive sensor position re-calibration for improved reliability of gait parameters. Gait Posture 39, 1146–1148. doi: 10.1016/j.gaitpost.2014.01.020
Hamacher, D., Rudolf, M., Lohmann, C., and Schega, L. (2016). Pain severity reduction in subjects with knee osteoarthritis decreases motor-cognitive dual-task costs. Clin. Biomech. 39, 62–64. doi: 10.1016/j.clinbiomech.2016.09.009
Hamacher, D., Singh, N. B., Van Dieën, J. H., Heller, M. O., and Taylor, W. R. (2011). Kinematic measures for assessing gait stability in elderly individuals: a systematic review. J. R. Soc. 8, 1682–1698. doi: 10.1098/rsif.2011.0416
Heinrich, S., Rapp, K., Rissmann, U., Becker, C., and König, H.-H. (2010). Cost of falls in old age: a systematic review. Osteoporos. Int. 21, 891–902. doi: 10.1007/s00198-009-1100-1
Hillier, S., Immink, M., and Thewlis, D. (2015). Assessing proprioception: a systematic review of possibilities. Neurorehabil. Neural Repair 29, 933–949. doi: 10.1177/1545968315573055
Hodges, P. W. (2011). Pain and motor control: from the laboratory to rehabilitation. J. Electromyogr. Kinesiol. 21, 220–228. doi: 10.1016/j.jelekin.2011.01.002
Ihlen, E. A. F., Weiss, A., Helbostad, J. L., and Hausdorff, J. M. (2015). The Discriminant value of phase-dependent local dynamic stability of daily life walking in older adult community-dwelling fallers and nonfallers. Biomed Res. Int. 2015:402596. doi: 10.1155/2015/402596
Inouye, S. K., Brown, C. J., and Tinetti, M. E. (2009). Medicare nonpayment, hospital falls, and unintended consequences. N. Engl. J. Med. 360, 2390–2393. doi: 10.1056/NEJMp0900963
Kennel, M., Brown, R., and Abarbanel, H. (1992). Determining embedding dimension for phase-space reconstruction using a geometrical construction. Phys. Rev. A 45, 3403–3411. doi: 10.1103/PhysRevA.45.3403
Keogh, E., Moore, D. J., Duggan, G. B., Payne, S. J., and Eccleston, C. (2013). The disruptive effects of pain on complex cognitive performance and executive control. PLoS ONE 8:e83272. doi: 10.1371/journal.pone.0083272
Kümmel, J., Kramer, A., Giboin, L.-S., and Gruber, M. (2016). Specificity of balance training in healthy individuals: a systematic review and meta-analysis. Sports Med. 46, 1261–1271. doi: 10.1007/s40279-016-0515-z
Lamers, M. J. M., Roelofs, A., and Rabeling-Keus, I. M. (2010). Selective attention and response set in the Stroop task. Mem. Cognit. 38, 893–904. doi: 10.3758/MC.38.7.893
Lehr, D., Hillert, A., Schmitz, E., and Sosnowsky, N. (2008). Screening depressiver Störungen mittels Allgemeiner Depressions-Skala (ADS-K) und State-Trait Depressions Scales (STDS-T). Diagnostica 54, 61–70. doi: 10.1026/0012-1924.54.2.61
Lindemann, U., Claus, H., Stuber, M., Augat, P., Muche, R., Nikolaus, T., et al. (2003). Measuring power during the sit-to-stand transfer. Eur. J. Appl. Physiol. 89, 466–470. doi: 10.1007/s00421-003-0837-z
Lord, S. R., Clark, R. D., and Webster, W. (1991). Postural stability in the elderly. J. Gerentol. 46, M69–M76.
Lord, S. R., Menz, H. B., and Tiedemann, A. (2003). A physiological profile approach to falls risk assessment and prevention. Phys. Ther. 83, 237–252. doi: 10.1093/ptj/83.3.237
Meyer, T. D., and Hautzinger, M. (2001). Allgemeine Depressions-Skala (ADS). Diagnostica 47, 208–215. doi: 10.1026//0012-1924.47.4.208
Muir, S. W., Berg, K., Chesworth, B., Klar, N., and Speechley, M. (2010). Balance impairment as a risk factor for falls in community-dwelling older adults who are high functioning: a prospective study. Phys. Ther. 90, 338–347. doi: 10.2522/ptj.20090163
Nelson, M. E., Rejeski, W. J., Blair, S. N., Duncan, P. W., Judge, J. O., King, A. C., et al. (2007). Physical activity and public health in older adults: recommendation from the American College of Sports Medicine and the American Heart Association. Circulation 116, 1094–1105. doi: 10.1161/CIRCULATIONAHA.107.185650
Netz, Y., Wu, M.-J., Becker, B. J., and Tenenbaum, G. (2005). Physical activity and psychological well-being in advanced age: a meta-analysis of intervention studies. Psychol. Aging 20, 272–284. doi: 10.1037/0882-7974.20.2.272
Paleacu, D., Shutzman, A., Giladi, N., Herman, T., Simon, E. S., and Hausdorff, J. M. (2007). Effects of pharmacological therapy on gait and cognitive function in depressed patients. Clin. Neuropharmacol. 30, 63–71. doi: 10.1097/01.wnf.0000240949.41691.95
Pasma, J. H., Engelhart, D., Schouten, A. C., van der Kooij, H., Maier, A. B., and Meskers, C. G. M. (2014). Impaired standing balance: the clinical need for closing the loop. Neuroscience 267, 157–165. doi: 10.1016/j.neuroscience.2014.02.030
Pfortmueller, C. A., Lindner, G., and Exadaktylos, A. K. (2014). Reducing fall risk in the elderly: risk factors and fall prevention, a systematic review. Minerva Med. 105, 275–281.
Proske, U., and Gandevia, S. C. (2012). The proprioceptive senses. Their roles in signaling body shape, body position and movement, and muscle force. Physiol. Rev. 92, 1651–1697. doi: 10.1152/physrev.00048.2011
Riemann, B. L., and Lephart, S. M. (2002). The sensorimotor system, part II. The role of proprioception in motor control and functional joint stability. J. Athl. Train. 37, 80–84.
Riemann, B. L., Myers, J. B., and Lephart, S. M. (2002). Sensorimotor system measurement techniques. J. Athl. Train. 37, 85–98.
Robinovitch, S. N., Feldman, F., Yang, Y., Schonnop, R., Leung, P. M., Sarraf, T., et al. (2013). Video capture of the circumstances of falls in elderly people residing in long-term care. An observational study. Lancet 381, 47–54. doi: 10.1016/S0140-6736(12)61263-X
Rolke, R., Baron, R., Maier, C., Tölle, T. R., Treede, R.-D., Beyer, A., et al. (2006). Quantitative sensory testing in the German Research Network on Neuropathic Pain (DFNS): standardized protocol and reference values. Pain 123, 231–243. doi: 10.1016/j.pain.2006.01.041
Rosenstein, M. T., Collins, J. J., and De Luca, C. J. (1993). A practical method for calculating largest Lyapunov exponents from small data sets. Physica D Nonlinear Phenomena 65, 117–134. doi: 10.1016/0167-2789(93)90009-P
Rubenstein, L. Z. (2006). Falls in older people: epidemiology, risk factors and strategies for prevention. Age Ageing 35(Suppl. 2), ii37–ii41. doi: 10.1093/ageing/afl084
Sherrington, C., Tiedemann, A., Fairhall, N., Close, J. C. T., and Lord, S. R. (2011). Exercise to prevent falls in older adults: an updated meta-analysis and best practice recommendations. N. S. W. Public Health Bull. 22, 78–83. doi: 10.1071/NB10056
Sherrington, C., Whitney, J. C., Lord, S. R., Herbert, R. D., Cumming, R. G., and Close, J. C. T. (2008). Effective exercise for the prevention of falls: a systematic review and meta-analysis. J. Am. Geriatr. Soc. 56, 2234–2243. doi: 10.1111/j.1532-5415.2008.02014.x
Sibley, K. M., Beauchamp, M. K., van Ooteghem, K., Straus, S. E., and Jaglal, S. B. (2015). Using the systems framework for postural control to analyze the components of balance evaluated in standardized balance measures: a scoping review. Arch. Phys. Med. Rehabil. 96, 122–132. doi: 10.1016/j.apmr.2014.06.021
Taylor, A. H., Cable, N. T., Faulkner, G., Hillsdon, M., Narici, M., and van der Bij, A. K. (2004). Physical activity and older adults: a review of health benefits and the effectiveness of interventions. J. Sports Sci. 22, 703–725. doi: 10.1080/02640410410001712421
Terrier, P., and Reynard, F. (2015). Effect of age on the variability and stability of gait: a cross-sectional treadmill study in healthy individuals between 20 and 69 years of age. Gait Posture 41, 170–174. doi: 10.1016/j.gaitpost.2014.09.024
Tinetti, M. E., Speechley, M., and Ginter, S. F. (1988). Risk factors for falls among elderly persons living in the community. N. Engl. J. Med. 319, 1701–1707. doi: 10.1056/NEJM198812293192604
Uttl, B., and Graf, P. (1997). Color-Word Stroop test performance across the adult life span. J. Clin. Exp. Neuropsychol. 19, 405–420. doi: 10.1080/01688639708403869
VanSwearingen, J. M., Perera, S., Brach, J. S., Wert, D., and Studenski, S. A. (2011). Impact of exercise to improve gait efficiency on activity and participation in older adults with mobility limitations: a randomized controlled trial. Phys. Ther. 91, 1740–1751. doi: 10.2522/ptj.20100391
Ware, J., Kosinski, M., and Keller, S. D. (1996). A 12-item short-form health survey: construction of scales and preliminary tests of reliability and validity. Med. Care 34, 220–233. doi: 10.1097/00005650-199603000-00003
WHO (2008). WHO Global Report on Falls Prevention in Older Age. Geneva: World Health Organization Ageing and Life Course Family and Community Health.
Winter, D. A. (1995). Human balance and posture control during standing and walking. Gait Posture 3, 193–214. doi: 10.1016/0966-6362(96)82849-9
Wollesen, B., Voelcker-Rehage, C., Willer, J., Zech, A., and Mattes, K. (2015). Feasibility study of dual-task-managing training to improve gait performance of older adults. Aging Clin. Exp. Res. 27, 447–455. doi: 10.1007/s40520-014-0301-4
Yakhdani, H. R. F., Bafghi, H. A., Meijer, O. G., Bruijn, S. M., van den Dikkenberg, N., Stibbe, A. B., et al. (2010). Stability and variability of knee kinematics during gait in knee osteoarthritis before and after replacement surgery. Clin. Biomech. 25, 230–236. doi: 10.1016/j.clinbiomech.2009.12.003
Zech, A., Steib, S., Freiberger, E., and Pfeifer, K. (2011). Functional muscle power testing in young, middle-aged and community-dwelling nonfrail and prefrail older adults. Arch. Phys. Med. Rehabil. 92, 967–971. doi: 10.1016/j.apmr.2010.12.031
Zijlstra, A., Mancini, M., Lindemann, U., Chiari, L., and Zijlstra, W. (2012). Sit-stand and stand-sit transitions in older adults and patients with Parkinson's disease: event detection based on motion sensors versus force plates. J. Neuroeng. Rehabil. 9:75. doi: 10.1186/1743-0003-9-75
Keywords: balance, muscular fitness, physical activity, pain, peripheral sensation, gender, proprioception, gait variability
Citation: Hamacher D, Liebl D, Hödl C, Heßler V, Kniewasser CK, Thönnessen T and Zech A (2019) Gait Stability and Its Influencing Factors in Older Adults. Front. Physiol. 9:1955. doi: 10.3389/fphys.2018.01955
Received: 25 July 2018; Accepted: 22 December 2018;
Published: 24 January 2019.
Edited by:
Lars Donath, German Sport University Cologne, GermanyReviewed by:
Sridhar Poosapadi Arjunan, RMIT University, AustraliaEduard Kurz, University Hospital in Halle, Germany
Copyright © 2019 Hamacher, Liebl, Hödl, Heßler, Kniewasser, Thönnessen and Zech. This is an open-access article distributed under the terms of the Creative Commons Attribution License (CC BY). The use, distribution or reproduction in other forums is permitted, provided the original author(s) and the copyright owner(s) are credited and that the original publication in this journal is cited, in accordance with accepted academic practice. No use, distribution or reproduction is permitted which does not comply with these terms.
*Correspondence: Daniel Hamacher, daniel.hamacher@uni-jena.de