- Institute of Sport Science, University of Lausanne, Lausanne, Switzerland
Alpine ski analysis has always been very challenging, mainly due to the environmental conditions, large field and rapid and dynamic skiers’ movements. Global navigation satellite system (GNSS) offers a solution adapted to outdoor testing, but the relationship between the point where the antenna is attached and the real centre of mass (CoM) position is still unknown. This article proposes to compare different points of the body used to quantify the performance of alpine skiers. 3D models of seven elite skiers performing giant slalom (GS) were built using multiple camera system and dedicated motion tracking software. CoM as well as pelvis, head and feet trajectories were deduced from the data. The potential and kinetic energies corresponding to these points were calculated, as well as the evolution of the turn radius during the turn cycle. Differences between values given by the CoM and the other morphological points were analyzed. The pelvis offered the best estimation of the CoM: No differences were found for the biomechanical parameters, except for the kinetic energy, where 2% of the turn cycle had significant different values. The head was less accurate compared to the pelvis, showing significant differences with CoM between 7 and 20% of the turn cycle depending on the parameter. Finally, the feet offered the worst results, with significant differences between 16 and 41% of the turn cycle. Energies and turn radius calculated by using pelvis in place of CoM offered similar patterns, allowing the analysis of mechanical and dissipation energy in GS. This may potentially enable easier testing methods to be proposed and tested.
Introduction
Human movement analyses are usually based on the body centre of mass (CoM) position determination. Mechanics of different sports have widely been studied, showing the necessity to calculate the CoM with a good accuracy to perform precise analysis [e.g., walking (Cavagna et al., 1963; Saibene and Minetti, 2003; Willems et al., 1995), running (Kyröläinen et al., 2001), cycling (Chèze et al., 1995)]. However, CoM calculations usually require large infrastructures such as 3D camera system (Richards, 1999) or a force platform (Barbier et al., 2003). Kinematic arms (Belli et al., 1993) and global navigation satellite system (GNSS) (Terrier et al., 2005) have also been used in running and walking analysis, but these methods use a point situated on the back of the subject to approximate the CoM. Slawinski et al. (2004) analyzed the use of a lumbar point for the estimation of potential and kinetic mechanical power in running. With this method, they found an overestimation of the kinetic power and underestimation of the potential power. Nevertheless, results obtained by using either a fixed point on the back or the CoM were well correlated. Gard et al. (2004) compared three methods (i.e., force platform, marker on the sacrum and full body model) to determine vertical displacement of the CoM during walking. They highlighted an overestimation of the vertical displacement of the CoM with the sacrum marker. In alpine skiing, the CoM has also been used as a reference to perform technical analysis (Kagawa, 2001; Schiefermüller et al., 2005), trajectories and speed analysis (Lešnik and Zvan, 2003) and to analyze energy balance of skiers performing turns both in giant slalom (GS) (Supej et al., 2005; Supej, 2008) and in slalom (Reid et al.2009). More recently, Fasel et al. (2016) used both GNSS and inertial sensors to determine CoM in alpine skiing. An accuracy and precision of 0.08 and 0.04 m respectively were reported for the CoM position.
Multiple camera systems are commonly used to reconstruct 3D models of the athlete, and CoM is then calculated, with de Leva adjustments (De Leva, 1996), using mathematical models of the body based on Hanavan (1964), Clauser et al. (1969), or Zatsiorsky (1983). However, this method only enables the recording of a small acquisition volume (usually one or two gates) and suffers from the approximation induced by the model. Alternatively, the use of low cost, high accuracy GNSS have expanded, allowing analyzing trajectories during a whole run (Waegli and Skaloud, 2007; Gomez-Lopez et al., 2009; Waegli and Skaloud, 2009; Waegli et al., 2009). However, since the CoM is not a fixed body point, the link between the antenna trajectory and the real CoM of the skier need to be determined. Gilgien et al. (2013) used the pendulum principle to estimate the distance between the real CoM position and the position given by a GNSS antenna placed on the helmet. Another solution could be to place the antenna to different positions. Therefore, the aim of this work was to compare the use of either the CoM or other morphological points to determine delta of potential energy (ΔEpot), kinetic energy (Ekin) and turn radius (Trad) of alpine skiers performing GS.
Materials and Methods
Participants
Seven European Cup and FIS racers [mean ± standard deviation (SD): body mass 98.8 ± 9.1 kg; height 1.82 ± 0.07 m; GS FIS points 26.45 ± 14.58] participated in the study. All participants were healthy males without any joint motion problems (World Medical Association, 2013).
Experimental Design and Setting
A GS run was set up with a total of six gates, with a linear gate distance of 24 m and a lateral offset of 9 m. The first three gates were used to initiate the rhythm, and the next three were recorded. The slope angle was approximately 22 degrees. Six panning and tilting cameras, 1004∗1004 pixels resolution, 48 Hz (PiA1000, Basler, Switzerland) were positioned around the GS run, about 35 m from the center of the zone of acquisition (i.e., video captured). Each camera was mounted on a special tripod head, especially built to always keep the sensor center of the camera at the same 3D coordinate, even when the camera was rotated to track the skier. Reference markers mounted on poles were positioned around the run to act as calibration and reference points for the panning and tilting reconstruction. The capture volume was around 60 ∗ 20 ∗ 2 m (Figure 1A). The positions of each reference marker, gate and camera were measured with a reflectorless total station (theodolite + laser range finder, LQTS-522D, Longqiang, China). The cameras’ 3D coordinates were calculated as the median of two points on either side of the tilting axis of the camera. Each camera was connected with Gigabit Ethernet to a dedicated laptop which directly recorded the frames in the RAM memory of the computer, using a software developed for this specific purpose (Swistrack, Thomas Lochmatter, Switzerland). Cameras were also connected to battery packs and dedicated synchronization boxes (Meyer et al., 2012). These boxes use GNSS signal to achieve wireless synchronization of the cameras recording system and ensure images from the six cameras are taken simultaneously with an error of less than 2.00 μs.
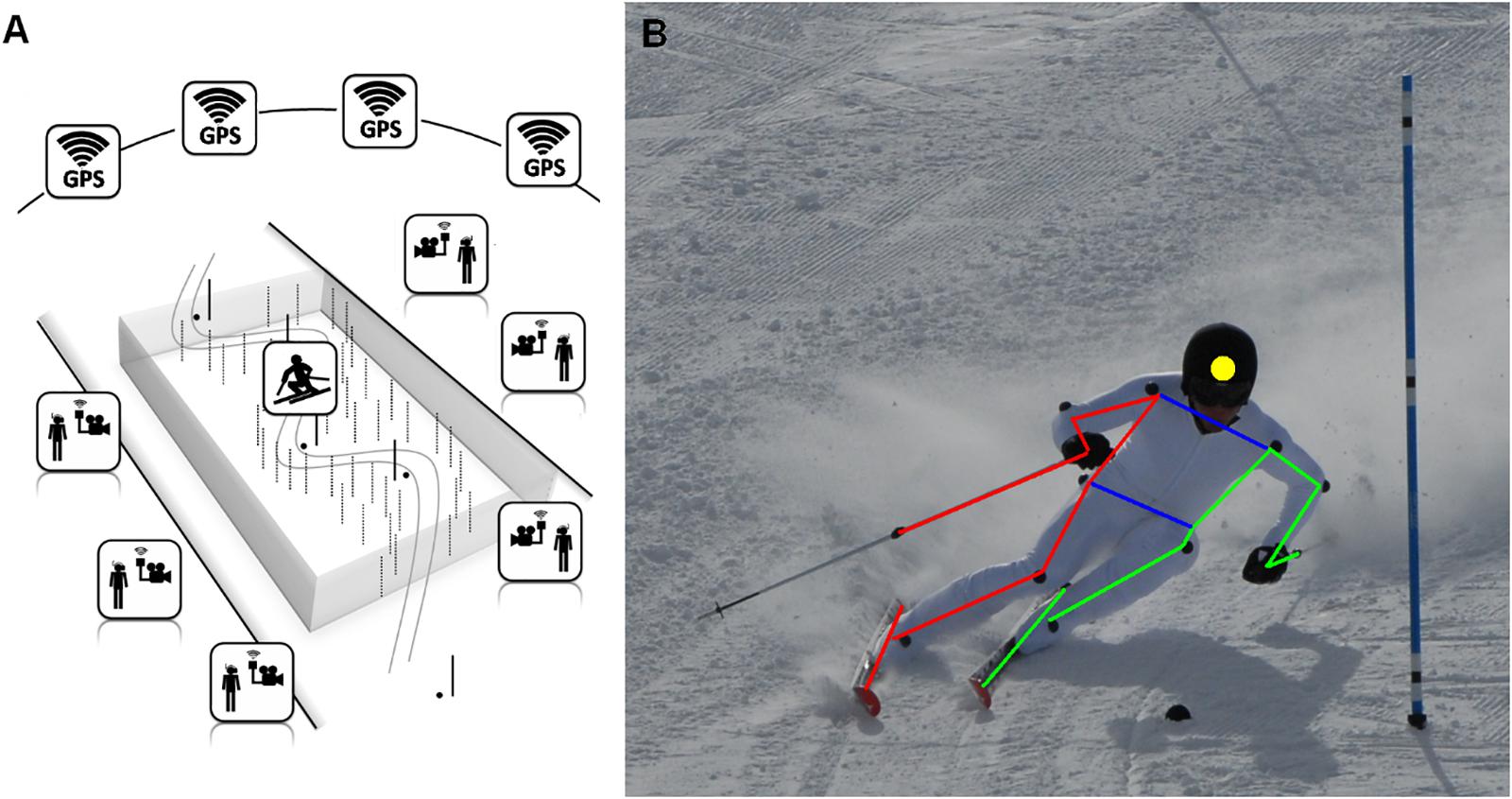
FIGURE 1. (A) Slope setup showing the cameras, the gates and the reference points positions, (B) skier suit, markers and body segments.
The athletes used their own GS skis to completed three trials of the GS. The runs were recorded and the time needed to go through three considered gates was estimated by counting the number of images captured on video. The fastest run of each skier was then analyzed (typical speed around 20 m/s). The selected runs were processed with SIMI motion software (SIMI motion, SIMI, Germany), using the panning and tilting modules. The camera’s internal (e.g., focal length, image format and principal point) and external (e.g., camera position and orientation) parameters needed for the analysis were determined using the DLT 11 calibration method (Hatze, 1988; Abdel-Aziz and Karara, 2015).
Participants had to wear a white racing suit previously equipped with 14 black markers, a black helmet, and black gloves. CoM of both ski poles were also marked with black markers. In total, 19 markers were identified, and 3D models composed of 14 segments were built (Figure 1B). The CoM of the skier was calculated using the model proposed by Clauser et al. (1969) modified to take the material’s weight into account. Tree morphological points that could be used for further analysis (e.g., GNSS antenna placement) were also defined: The Pelvis position was defined as the middle point between the 2 trochanters’ markers, the Feet position as the middle point between the 2 ankle-bone markers and the Head position as the center of the helmet.
The accuracy of the reconstruction method was measured in two different ways. First, the positions of three gates as given by the total station were compared with the positions calculated by the software. Second, the error in the length of each body segment was determined.
Parameters Analysis
The ΔEpot (J/kg), Ekin (J/kg), and Trad (1/m), were calculated for the CoM and the three morphological points (i, with i = {CoM, Head, Pelvis, Feet}). For analysis purposes, each trial was normalized to fit a 100% temporal turn cycle, where 0 and 100% were the time points when the projection of the CoM was between the two skis. A cubic B-splines interpolation method was used to achieve the normalization (Greville, 1964; Lee et al., 1997).
Potential Energy
As the different morphological points are positioned at different heights of the body, the ΔEpoti was calculated at each percent of the turn cycle, using the mass of the skier including equipment (M), the acceleration due to gravity (g) and the delta height (ΔHi(t)) of the analyzed point in a global reference system:
Kinetic Energy
The Ekini evolution during the turn was calculated using the speed (Vi) of the analyzed points (calculated as the time derivative of the point coordinate) and M, using the following equation:
The mean Ekin (Ekinm) was also calculated over the whole turn cycle, to show the overall error when using a morphological point instead of the CoM.
Turn Radius
The Tradi were calculated directly with SIMI motion, using the Frenet-Serret formula (Serret, 1851; Frenet, 1852). The turn entry (Tentryi) was defined as the instant where the Tradi dropped below the natural radius of the skis (i.e., 25 m) and the turn exit (Texiti) as the instant where the turn radius went over 25 m again.
Statistical Analysis
Statistical parametric mapping (SPM) was used on paired Student T-Tests (Pataky, 2010; Pataky et al., 2015) to analyze data over the whole turn cycle, to compare ΔEpot, Ekin and Trad obtained for the morphological points to the CoM reference. The fit between the curves was assessed by summing percent of time of a turn cycle where SPM indicate significant differences (tt values with p < .05). Paired Student T-tests were also used to assess statistical differences between the CoM and the morphological points for the Ekinm, Tentry, and Texit, given as mean ±SD. For all statistical analyses, significance was accepted at p < .05.
Results
3D Accuracy
For the global gates position reconstruction using the 3D reconstruction software, a horizontal mean error of 14.0 ± 8.0 mm was calculated, giving a 95% limit of agreement of 27.1 mm. For the vertical error, the absolute mean of 5.9 ± 3.5 mm gave a 95% limit of agreement of 11.6 mm. Adding the horizontal and the vertical errors led to a total 3D reconstruction error of 15.7 ± 7.8 mm, and a 95% limit of agreement of 28.3 mm. The segments lengths mean error of 13.0 ± 12.0 mm led to a 95% limit of agreement of 32.7 mm.
Potential Energy
Using the Head instead of the CoM to estimate ΔEpot led to significantly different values for 12% of the time course of the turn during the turn cycle. The corresponding curves are plotted on Figure 2A with the corresponding SPM results on Figure 2B (tt threshold at ±5.90).
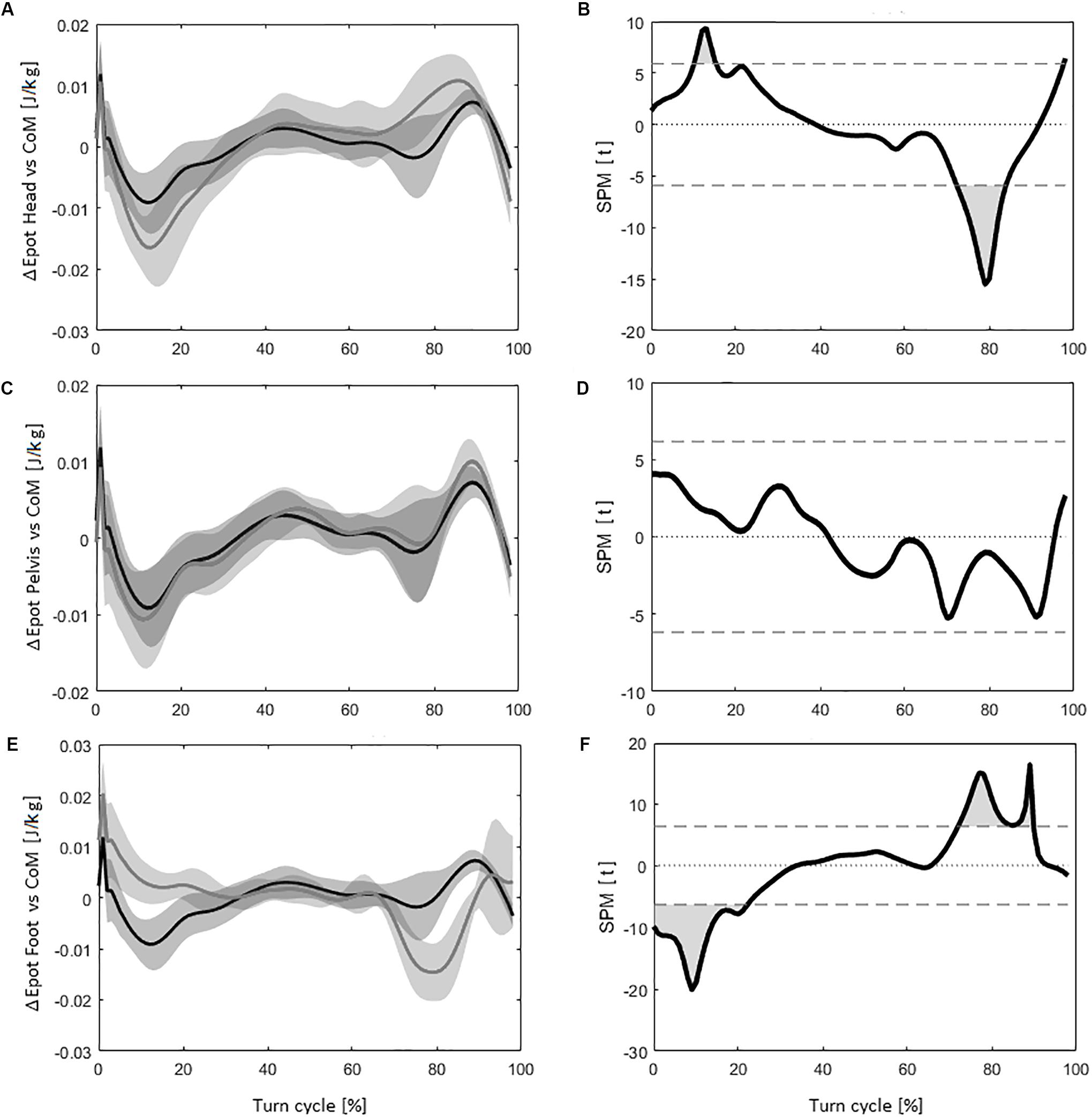
FIGURE 2. (A) The evolution of the delta of potential energy (ΔEpot) for the CoM (in black) and the Head (in dark gray) during the turn cycle, with the corresponding standard deviation (SD) (in light gray). (B) Evolution of statistical parametric mapping (SPM) values (tt) during the turn cycle, with light gray area representing the portions of the turns were it is statistically possible to differentiate the CoM with the Head (the horizontal dash lines correspond to tt = 6.79, p = 0.05). (C) The evolution of ΔEpot for the CoM (in black) and the Pelvis (in dark gray) during the turn cycle, with the corresponding SD (in light gray). (D) Evolution of SPM values (tt), with light gray area representing the portions of the turns were it is statistically possible to differentiate the CoM with the Pelvis. (E) The evolution of ΔEpot for the CoM (in black) and the Feet (in dark gray) during the turn cycle, with the corresponding SD (in light gray). (F) Evolution of SPM values (tt), with light gray area representing the portions of the turns were it is statistically possible to differentiate the CoM with the Feet.
When the Pelvis was used instead of the CoM to calculate ΔEpot, no significantly different values were obtained. Figure 2C shows the evolution of the ΔEpot between the CoM and the Pelvis with the corresponding SPM curve on Figure 2D (tt threshold at ±6.16).
Concerning the use of the Feet to estimate the ΔEpot, 41% of the measures during the turn cycle had significantly different values (tt threshold at ±6.33) compared to the values obtained using the CoM, as seen in Figures 2E,F.
Kinetic Energy
From the Ekin calculation, it can be seen that the Head induced significantly different values for 20% of the measures during the turn cycle compared to the results obtained using the CoM. Figure 3A represents the evolution of the curves during the turn cycle, while Figure 3B shows the corresponding SPM results (tt threshold at ±5.99). Compared to the CoM, the Head induced a significant underestimation of −2.57 ± 1.22 J/kg (p < 0.001) when calculating Ekinm.
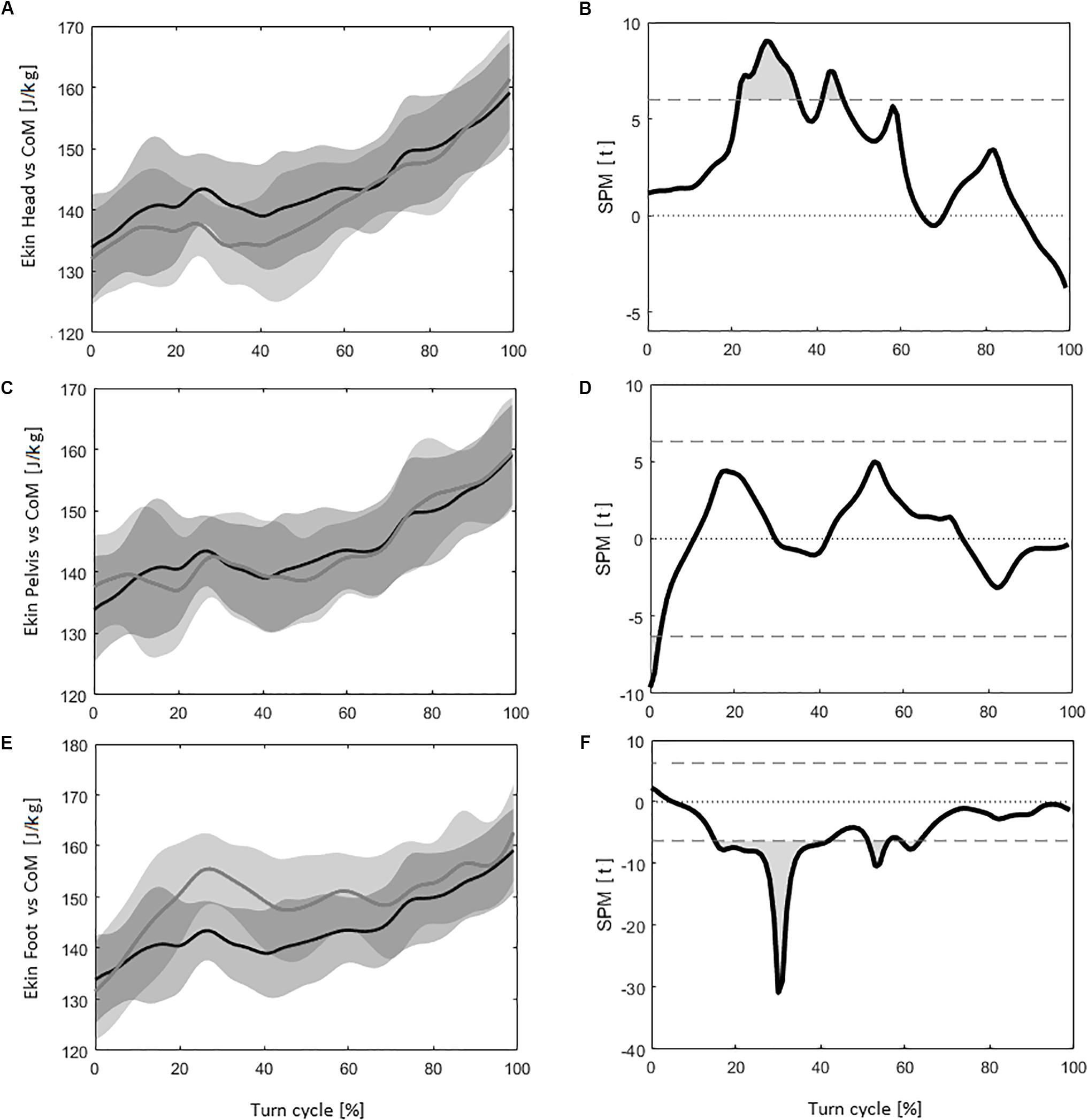
FIGURE 3. (A) The evolution of the kinetic energy (Ekin) for the CoM (in black) and the Head (in dark gray) during the turn cycle, with the corresponding standard deviation (SD) (in light gray). (B) Evolution of statistical parametric mapping (SPM) values (tt) during the turn cycle, with light gray area representing the portions of the turns were it is statistically possible to differentiate the CoM with the Head (the horizontal dash lines correspond to tt = 6.79, p = 0.05). (C) The evolution of Ekin for the CoM (in black) and the Pelvis (in dark gray) during the turn cycle, with the corresponding SD (in light gray). (D) Evolution of SPM values (tt), with light gray area representing the portions of the turns were it is statistically possible to differentiate the CoM with the Pelvis. (E) The evolution of Ekin for the CoM (in black) and the Feet (in dark gray) during the turn cycle, with the corresponding SD (in light gray). (F) Evolution of SPM values (tt), with light gray area representing the portions of the turns were it is statistically possible to differentiate the CoM with the Feet.
Compared to the CoM, The Pelvis induced significantly different values for only 2% of the Ekin measurement during the turn cycle (Figures 3C,D) (tt threshold at ±6.31). No significant difference were found between the CoM and the Pelvis for Ekinm (−0.22 ± 0.93 J/kg, p = 1.000).
For the Feet, Ekin calculation led to significantly different values for 36% of the turn cycle compared to the CoM. Figures 3E,F displays the evolution of the Ekin curve and SPM results (tt threshold at ±6.29). The Feet induced a significant overestimation of Ekinm [5.77 ± 4.00 J/kg (p < 0.001)] compared to the result obtained with the CoM.
Turn Radius
The results obtained using the Head instead of the CoM for the calculation of Trad indicated significant differences for 7% of the turn cycle. Evolution of Trad is described in Figure 4A, with the corresponding SPM values Figure 4B (tt threshold at ±6.67).
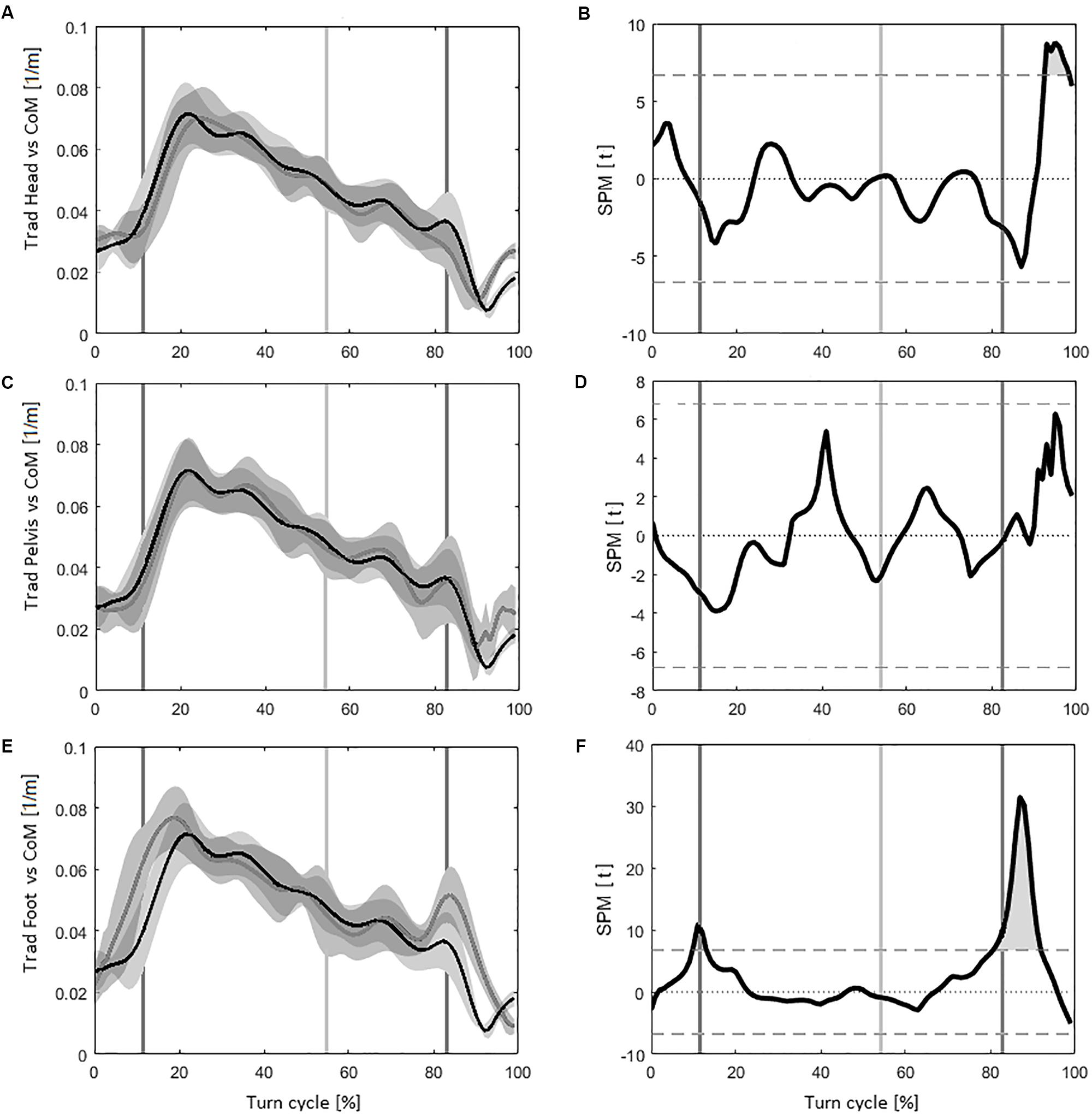
FIGURE 4. (A) The evolution of the turn radius (Trad) for the CoM (in black) and the Head (in dark gray) during the turn cycle, with the corresponding standard deviation (SD) (in light gray). (B) Evolution of statistical parametric mapping (SPM) values (tt) during the turn cycle, with light gray area representing the portions of the turns were it is statistically possible to differentiate the CoM with the Head (the horizontal dash lines correspond to tt = 6.79, p = 0.05). (C) The evolution of Trad for the CoM (in black) and the Pelvis (in dark gray) during the turn cycle, with the corresponding SD (in light gray). (D) Evolution of SPM values (tt), with light gray area representing the portions of the turns were it is statistically possible to differentiate the CoM with the Pelvis. (E) The evolution of Trad for the CoM (in black) and the Feet (in dark gray) during the turn cycle, with the corresponding SD (in light gray). (F) Evolution of SPM values (tt), with light gray area representing the portions of the turns were it is statistically possible to differentiate the CoM with the Feet.
The calculation of Trad using the Pelvis instead of the CoM induced no significant differences during the whole turn cycle (tt threshold at ±6.79). The corresponding curves are plotted on Figures 4C,D.
Using the Feet instead of the CoM to estimate Trad revealed that 16% of the measures had significantly different values during the turn cycle. Figures 4E,F displays the evolution of the Trad curve and SPM results for the comparison of the CoM and the Feet during the turn cycle (tt threshold at ±6.76).
Comparison of Tentry and Texit between the CoM and the morphological points can be found in Table 1.
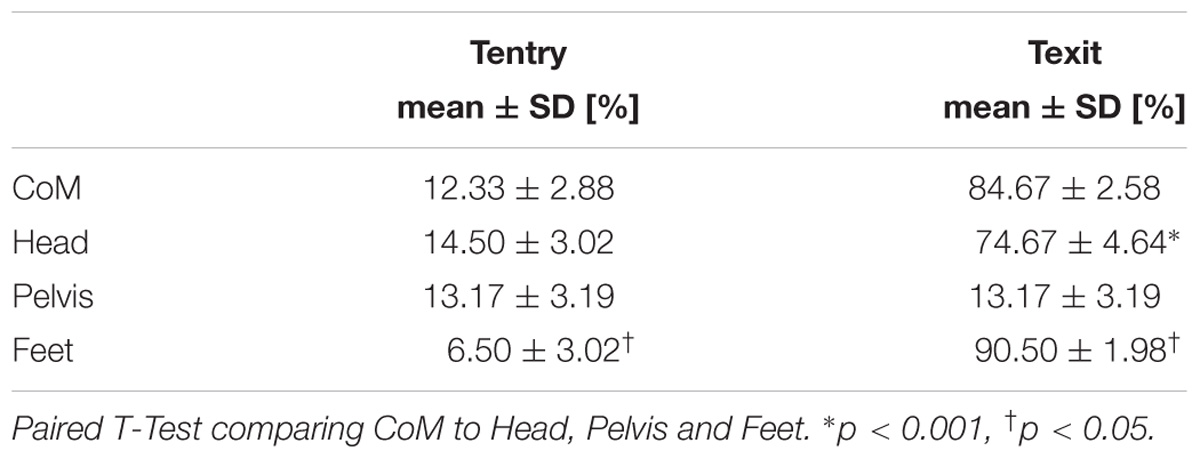
TABLE 1. Moment of the turn cycle (in %) when the radius falls below 25 m (Tentry) and exceed 25 m again (Texit).
Discussion
The most important finding of this study was the high level of agreement between the Pelvis and the CoM. Indeed, when looking at the different parameters analyzed, the Pelvis offered the best estimation for the ΔEpot, Ekin and Trad calculation. No significant differences were found for the ΔEpot and Trad during the whole turn whilst only 2% of the turn cycle significantly differed in the case of the Ekin. The difference was encountered only at the beginning of the turn.
As a global observation, it is quite intuitive to see the Feet and the Head as extreme points of the skier, while the Pelvis is more centered and near the CoM. Nevertheless, the Head allowed slightly better estimations than the Feet for the analyzed parameters showing more similar patterns of the CoM. The angulation of the hips during the second half of the turn can probably explain this result, as the Head is more centered vertically on the CoM while the Feet follow an external trajectory. The best morphological point to estimate ΔEpot and Ekin is therefore the Pelvis, followed by the Head and finally by the Feet that offer poor reliability.
Energy
As Epot is directly correlated to vertical displacement, the curves of ΔEpot obtained in this study can be compared to the work proposed by Pozzo et al. (2005), who calculated the vertical displacement of the CoM compared to the ground. As expected, the CoM was higher during transitions between turns and lower at gate crossings. This corresponds well to the interpretation of ΔEpot curves calculated using the CoM and the Feet in the present study.
As the Ekin values depend on the square power of the speed, the shape of the curves obtained in this study can also be compared to those obtained by Pozzo et al. (2005) for the speed of the skiers during the turns. The measured speed attained its maximal value during gate transition, as it does in the present study.
Supej (2008) and Reid et al. (2009) analyzed the mechanical energy of skiers (Emech), which involved addition of the Ekin and the Epot. They also calculated the corresponding dissipated energy (Edissip) as the change in mechanical energy per change of vertical distance (Supej et al., 2005). To allow comparison with these studies, Figures 5A,B show the Emech and the Edissip respectively, calculated using the CoM and the morphological points of the present study.
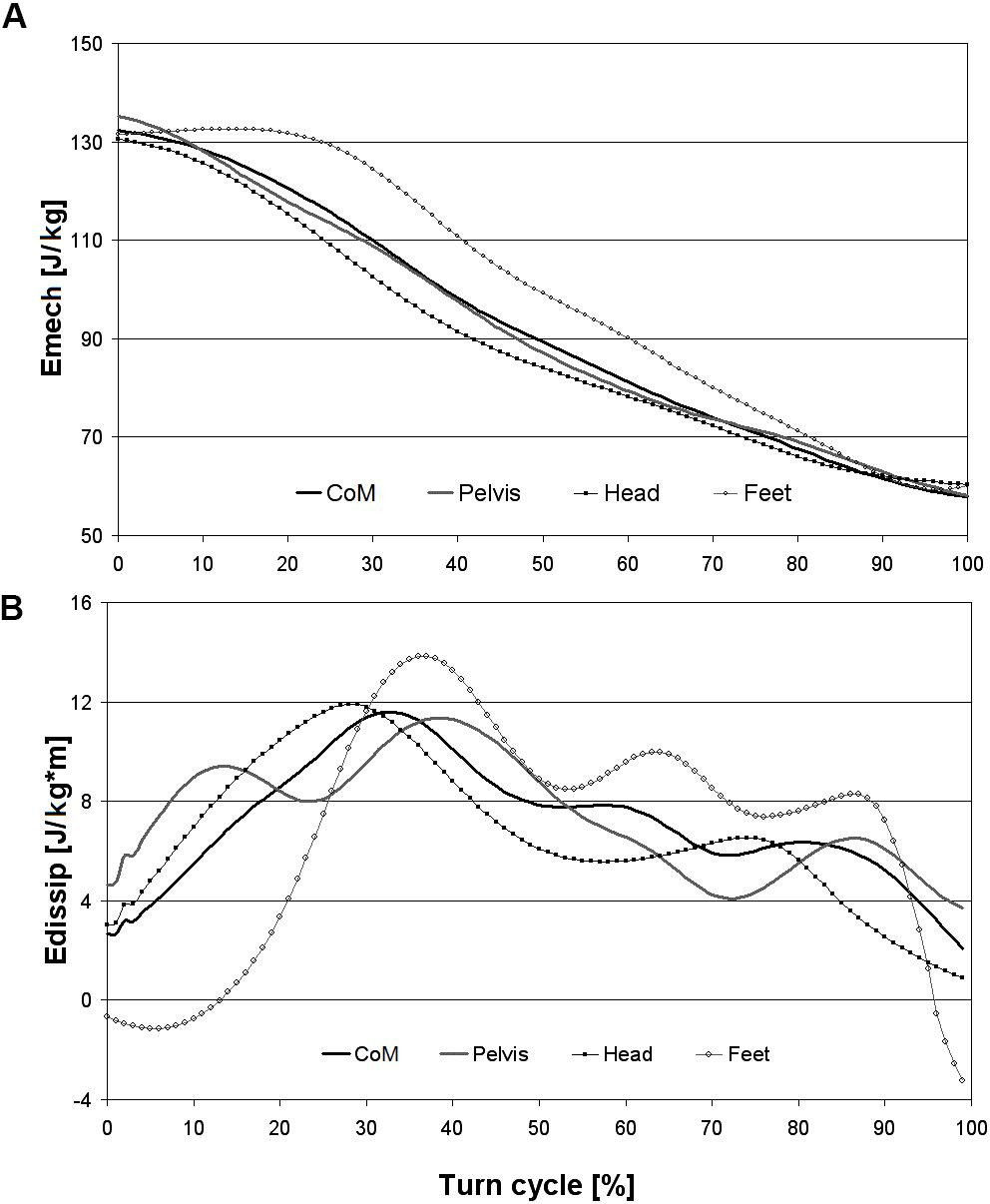
FIGURE 5. (A) Mechanical energy (Emech) calculated using the CoM and the morphological points, (B) Energy dissipation (Edissip) during the turn.
The curves obtained for the CoM are very similar to those obtained by Supej (2008) in GS and Reid et al. (2009) in slalom. The minimum energy dissipation occurred at the turn transition and the maximum during the first steering phase, between 20 and 40% of the turn cycle.
Turn Radius
The Trad described by the Feet trajectory began earlier and ended after the Trad of the CoM. The Head also finished the turn earlier than the CoM. Therefore, the Head had the longer time interval between two turns where its trajectory was almost straight, and the Feet had the shortest time interval with a straight trajectory. It was interesting to note that around the gate crossing, inter-athlete variability increased, suggesting that the gates induced perturbation. If the radius decreased during the transition phase to reach its minimum, it increased gradually during the steering phases. Supej (2008) obtained a curve of a similar shape when calculating the CoM’s turn radius of four athletes performing GS. For slalom turns, Reid et al. (2009) obtained a different curve in slalom, where the radius decreased slowly during the first part of the turn and increased rapidly at the end of the turn. This indicates a different choice of trajectory in giant compared to slalom.
The Feet trajectory radii showed a small reduction between the second steering phase and the transition phase, when the skier decided to engender the new turn. It was at this same moment that the skier made a longitudinal extension, when the Epot_diff between the CoM and the Head increased, at approximately 80% of the turn cycle (Figure 3A).
Once again, the Pelvis gave the best approximation of the CoM concerning turn radius, followed by the Head. The Feet, with a time lag in the turn radius did not offer a good approximation of the CoM’s Trad, but it could be interesting to further explore the radius reduction around 85% of the turn. Indeed, it may be possible that this radius reduction coincides with an increase in the force and an extension of the skier to trigger the next turn.
Conclusion
It is the first time that different morphological points of the body are used to estimate energetic parameters of alpine skiers. The results obtained with the Pelvis offered very accurate approximations of the CoM, with an equivalent accuracy than the pendulum method used by Gilgien et al. (2013). The Head also offered a good approximation for overall energy analysis and is a very accessible point for 3D video tracking or GNSS antenna placement, but side leaning profiles induced inaccurate estimations in the middle part of the turn. Finally, the Feet did not allow for a good estimation of the CoM as most of the parameters did not even have curves that look like those described by the CoM.
Data Availability Statement
The raw data supporting the conclusions of this manuscript will be made available by the authors, without undue reservation, to any qualified researcher.
Ethics Statement
This study was carried out in accordance with the recommendations of the HRO Guidelines from the “Commission cantonale (VD) d’éthique de la recherche sur l’être humain.” The protocol was approved by the “Commission cantonale (VD) d’éthique de la recherche sur l’être humain.” All subjects gave written informed consent in accordance with the Declaration of Helsinki.
Author Contributions
All authors listed have made a substantial, direct and intellectual contribution to the work, and approved it for publication.
Funding
This work was supported by a Grant from the Swiss Federal Office for Sports.
Conflict of Interest Statement
The authors declare that the research was conducted in the absence of any commercial or financial relationships that could be construed as a potential conflict of interest.
Acknowledgments
The authors wants to thanks the athletes for their participation to this study. Parts of the present manuscript were included in the Ph.D. thesis of the first author (Meyer, 2012).
References
Abdel-Aziz, Y. I., and Karara, H. M. (2015). Direct linear transformation from comparator coordinates into object space coordinates in close-range photogrammetry. Photogramm. Eng. Remote Sens. 81, 103–107. doi: 10.14358/PERS.81.2.103
Barbier, F., Allard, P., Guelton, K., Colobert, B., and Godillon-Maquinghen, A.-P. (2003). Estimation of the 3-D center of mass excursion from force-plate data during. IEEE Trans. Neural Syst. Rehabil. 11, 31–37. doi: 10.1109/TNSRE.2003.810433
Belli, A., Avela, J., and Komi, P. V. (1993). Mechanical energy assessment with different methods during running. Int. J. Sports Med. 14, 252–256. doi: 10.1055/s-2007-1021173
Cavagna, G., Saibene, F. P., and Margaria, R. (1963). External work in walking. J. Appl. Physiol. 18, 1–9. doi: 10.1152/jappl.1963.18.1.1
Chèze, L., Fregly, B. J., and Dimnet, J. (1995). A solidification procedure to facilitate kinematic analyses based on video system data. J. Biomech. 28, 879–884. doi: 10.1016/0021-9290(95)95278-D
Clauser, C. E., McConville, J. T., and Young, J. W. (1969). Weight, Volume, and Center of Mass of Segments of the Human Body. Final Report, No. TR-69-70. Fort Belvoir, VA: Defense Technical Information Center.
De Leva, P. (1996). Adjustments to zatsiorsky-seluyanov’s segment inertia parameters. J. Biomech. 29, 1223–1230. doi: 10.1016/0021-9290(95)00178-6
Fasel, B., Spörri, J., Gilgien, M., Boffi, G., Chardonnens, J., Müller, E., et al. (2016). Three-dimensional body and centre of mass kinematics in alpine ski racing using differential GNSS and inertial sensors. Remote Sens. 8:671. doi: 10.3390/rs8080671
Gard, S. A., Miff, S. C., and Kuo, A. D. (2004). Comparison of kinematic and kinetic methods for computing the vertical motion of the body center of mass during walking. Hum. Mov. Sci. 22, 597–610. doi: 10.1016/j.humov.2003.11.002
Gilgien, M., Spörri, J., Chardonnens, J., Kröll, J., and Müller, E. (2013). Determination of external forces in alpine skiing using a differential global navigation satellite system. Sensors 13, 9821–9835. doi: 10.3390/s130809821
Gomez-Lopez, P. J., Hernan, O., and Ramirez, J. V. (2009). “Analysis of skiers’ performance using GPS,” in Science and Skiing IV, eds E. Müller and T. Stöggl (Maidenhead: Meyer & Meyer Sport), 207–215.
Greville, T. (1964). Numerical procedures for interpolation by spline functions. J. Soc. Ind. Appl. Math. B Numer. Anal. 1, 53–68.
Hatze, H. (1988). High-precision three-dimensional photogrammetric calibration and object space reconstruction using a modified DLT-approach. J. Biomech. 21, 533–538. doi: 10.1016/0021-9290(88)90216-3
Kagawa, H. Y. T. (2001). “Effective action of skier’s center of mass in skiing,” in Science and Skiing II, eds H. S. E. Müller, C. Raschner, S. Lindinger, and E. Kornexl (Oxford: Meyer & Meyer Sport).
Kyröläinen, H., Belli, A., and Komi, P. (2001). Biomechanical factors affecting running economy. Med. Sci. Sports Exerc. 33, 1330–1337. doi: 10.1097/00005768-200108000-00014
Lee, S., Wolberg, G., and Shin, S. Y. (1997). Scattered data interpolation with multilevel b-splines. IEEE Trans. Vis. Comput. Graph. 3, 228–244. doi: 10.1109/2945.620490
Lešnik, B., and Zvan, M. (2003). Comparison of centre of mass trajectories in modern giant slalom techniques. Kinesiology 35, 191–200.
Meyer, F. (2012). Biomechanical Analysis of Alpine Skiers Performing Giant Slalom Turns. Ph.D. thesis, Univ. Lausanne, Lausanne.
Meyer, F., Bahr, A., Lochmatter, T., and Borrani, F. (2012). Wireless GPS-based phase-locked synchronization system for outdoor environment. J. Biomech. 45, 188–190. doi: 10.1016/j.jbiomech.2011.09.014
Pataky, T. (2010). Generalized n-dimensional biomechanical field analysis using statistical parametric mapping. J. Biomech. 43, 1976–1982. doi: 10.1016/j.jbiomech.2010.03.008
Pataky, T., Vanrenterghem, J., and Robinson, M. (2015). Zero- vs. one-dimensional, parametric vs. non-parametric, and confidence interval vs. hypothesis testing procedures in one-dimensional biomechanical trajectory analysis. J. Biomech 48, 1277–1285. doi: 10.1016/j.jbiomech.2015.02.051
Pozzo, R., Canclini, A., Cotelli, C., and Barroni, G. (2005). “3D kinematics and kinetic analysis of geant slalom in elite skiers at Val Badia world cup race in 2002,” in Science and Skiing III, eds D. B. E. Müller, R. Klika, S. Lindinger, and H. Schwameder (Oxford: Meyer & Meyer Sport (Uk), Ltd), 125–135.
Reid, R., Gilgien, M., Moger, T., Tjorhom, H., Haugen, P., Kipp, R., et al. (2009). “Turn characteristics and energy dissipation in slalom,” in Science and Skiing IV, eds S. L. E. Müller and T. Stöggl (Maidenhead: Meyer & Meyer Sport), 419–429.
Richards, J. G. (1999). The measurement of human motion: a comparison of commercially available systems. Hum. Mov. Sci. 18, 589–602. doi: 10.1016/S0167-9457(99)00023-8
Saibene, F., and Minetti, A. E. (2003). Biomechanical and physiological aspects of legged locomotion in humans. Eur. J. Appl. Physiol. 88, 297–316. doi: 10.1007/s00421-002-0654-9
Schiefermüller, C., Lindinger, S., and Mueller, E. (2005). “The skier’s centre of gravity as a reference point in movement analysis for different designated systems,” in Science and Skiing III, eds D. B. E. Müller, R. Klika, S. Lindinger, and H. Schwameder (Aachen: Meyer & Meyer Sport), 172–185.
Serret, J. A. (1851). Sur quelques formules relatives à la théorie des courbes à double courbure. J. Math. 16, 193–207.
Slawinski, J., Billat, V., Koralsztein, J. P., and Tavernier, M. (2004). Use of lumbar point for the estimation of potential and kinetic mechanical power in running. J. Appl. Biomech. 20, 324–331. doi: 10.1123/jab.20.3.324
Supej, M. (2008). Differential specific mechanical energy as a quality parameter in racing alpine skiing. J. Appl. Biomech. 24, 121–129. doi: 10.1123/jab.24.2.121
Supej, M., Kugovnik, O., and Nemec, B. (2005). “Energy principle used for estimating the quality of a racing ski turn,” in Science and Skiing III, eds D. B. E. Müller, R. Klika, S. Lindinger, and H. Schwameder (Aachen: Meyer & Meyer Verlag), 228–237.
Terrier, P., Turner, V., and Schutz, Y. (2005). GPS analysis of human locomotion: Further evidence for long-range correlations in stride-to-stride fluctuations of gait parameters. Hum. Mov. Sci. 24, 97–115. doi: 10.1016/j.humov.2005.03.002
Waegli, A., Meyer, F., Ducret, S., Skaloud, J., and Pesty, R. (2009). “Assessment ot timing and performance based on trajectories from low-cost GPS/INS positioning,” in Science and Skiing IV, eds S. L. E. Müller and T. Stöggl (Maidenhead: Meyer & Meyer Sport), 556–564.
Waegli, A., and Skaloud, J. (2007). “Assessment of GPS/MEMS-IMU integration performance in ski racing,” in Proceedings of the ENC GNSS 2007 (TimeNav’07), Geneva.
Waegli, A., and Skaloud, J. (2009). Optimization of two GPS/MEMS-IMU integration strategies with application to sports. GPS Solut. 13, 315–326. doi: 10.1007/s10291-009-0124-5
Willems, P. A., Cavagna, G. A., and Heglund, N. C. (1995). External, internal and total work in human locomotion. J. Exp. Biol. 198, 379–393.
World Medical Association (2013). World Medical Association Declaration of Helsinki: ethical principles for medical research involving human subjects. JAMA 310, 2191–2194. doi: 10.1001/jama.2013.281053
Keywords: centre of mass, potential energy, kinetic energy, GNSS, giant slalom
Citation: Meyer F and Borrani F (2018) Estimating Alpine Skiers’ Energetics and Turn Radius Using Different Morphological Points. Front. Physiol. 9:1541. doi: 10.3389/fphys.2018.01541
Received: 30 May 2018; Accepted: 15 October 2018;
Published: 13 November 2018.
Edited by:
Gianluca Vernillo, Università degli Studi di Milano, ItalyReviewed by:
Thomas Leonhard Stöggl, University of Salzburg, AustriaTed Polglaze, University of Western Australia, Australia
Copyright © 2018 Meyer and Borrani. This is an open-access article distributed under the terms of the Creative Commons Attribution License (CC BY). The use, distribution or reproduction in other forums is permitted, provided the original author(s) and the copyright owner(s) are credited and that the original publication in this journal is cited, in accordance with accepted academic practice. No use, distribution or reproduction is permitted which does not comply with these terms.
*Correspondence: Frédéric Meyer, ZnJlZGVyaWMubWV5ZXJAdW5pbC5jaA==