- Human Performance and Sports Science Laboratory, Faculty of Sport Sciences, University of Murcia, Murcia, Spain
The aims of this study were (1) to establish the best fit between ventilatory and lactate exercise performance parameters in running and (2) to explore novel alternatives to estimate the maximal aerobic speed (MAS) in well-trained runners. Twenty-two trained male athletes (O2max 60.2 ± 4.3 ml·kg·min−1) completed three maximal graded exercise tests (GXT): (1) a preliminary GXT to determine individuals' MAS; (2) two experimental GXT individually adjusted by MAS to record the speed associated to the main aerobic–anaerobic transition events measured by indirect calorimetry and capillary blood lactate (CBL). Athletes also performed several 30 min constant running tests to determine the maximal lactate steady state (MLSS). Reliability analysis revealed low CV (<3.1%), low bias (<0.5 km·h−1), and high correlation (ICC > 0.91) for all determinations except V-Slope (ICC = 0.84). Validity analysis showed that LT, LT+1.0, and LT+3.0 mMol·L−1 were solid predictors of VT1 (−0.3 km·h−1; bias = 1.2; ICC = 0.90; p = 0.57), MLSS (−0.2 km·h−1; bias = 1.2; ICC = 0.84; p = 0.74), and VT2 (<0.1 km·h−1; bias = 1.3; ICC = 0.82; p = 0.9l9), respectively. MLSS was identified as a different physiological event and a midpoint between VT1 (bias = −2.0 km·h−1) and VT2 (bias = 2.3 km·h−1). MAS was accurately estimated (SEM ± 0.3 km·h−1) from peak velocity (Vpeak) attained during GXT with the equation: MASEST (km·h−1) = Vpeak (km·h−1) * 0.8348 + 2.308. Current individualized GXT protocol based on individuals' MAS was solid to determine both maximal and submaximal physiological parameters. Lactate threshold tests can be a valid and reliable alternative to VT and MLSS to identify the workloads at the transition from aerobic to anaerobic metabolism in well-trained runners. In contrast with traditional assumption, the MLSS constituted a midpoint physiological event between VT1 and VT2 in runners. The Vpeak stands out as a powerful predictor of MAS.
Introduction
Numerous studies have embraced the question of how training programs based on individualized physiological parameters may increase cardiorespiratory performance in endurance sports like running or cycling. Evidence suggests establishing exercise workloads or intensities based on the individual physiological events (i.e., setting training zones) allows athletes to minimize injury and fatigue risks, but above all to enhance individual adaptations and respond to the training plan (Scharhag-Rosenberger et al., 2012; Mann et al., 2014; Wolpern et al., 2015). A recent review (Stöggl and Sperlich, 2015) addressed the fact that similar training intensity distribution shows different efficacy and adaptations depending on the competitive stage, the endurance discipline, and the athlete's performance levels. Thus, the more individualized and accurate the thresholds and training zones, the more precise the exercise prescription and the greater the athletes' adaptation and performance enhancement (García-Pallarés et al., 2009; Wolpern et al., 2015). From a competition point of view, exercise test performance parameters are useful to track cardiopulmonary and specific adaptations to the entire season training plan, and to explain performance (Lucía et al., 2000; Esteve-Lanao et al., 2007; García-Pallarés et al., 2009, 2010).
Physiological variables such as maximal oxygen uptake (O2max), submaximal metabolic inflection points like the pulmonary ventilation thresholds (VT) and lactate thresholds (LT), the maximal aerobic speed (MAS: the speed associated with O2max), or the peak velocity (Vpeak: the highest speed attained at the end of the test) are regular variables used by coaches and scientists to estimate and monitor running performance during training and competition events (Farrell et al., 1979; di Prampero et al., 1986; Stratton et al., 2009; McLaughlin et al., 2010). For the evaluation of these parameters in runners, it is common to use graded exercise tests (GXTs) on the treadmill, consisting of a series of stages lasting 1–5 min. Differences in the duration of each stage and the load increments can alter the cardiorespiratory and metabolic response, and therefore the measurement (Bentley et al., 2007; Julio et al., 2017). As suggested by pioneering studies (Buchfuhrer et al., 1983; Lukaski et al., 1989), recent investigations (Midgley et al., 2007) and reviews (Julio et al., 2017), traditional longer GXTs (i.e., 20–30 min) to determine LT including increments each 3–5 min would prevent the athlete from achieving their MAS due to accumulative fatigue, dehydration, muscle acidosis, and cardiovascular drift. This is critical because MAS is a pertinent and widespread criterion to set training intensities for endurance disciplines (Billat and Koralsztein, 1996; Jones and Carter, 2000). An interesting approach carried out with cyclists revealed that shorter protocols (12–14 min) including 1-min stages are valid both, to estimate submaximal metabolic inflection points (VT and LT), and to identify true values for O2max and MAS in cyclists (Lucía et al., 1999, 2000; Gaskill et al., 2001; Midgley et al., 2007; Pallarés et al., 2016). However, the validity and reliability of GXT with 1-min stages protocol in runners needs to be fully verified.
Physiological response to exercise in endurance sports is commonly assessed though measurements based on ventilatory and lactate methods. However, the relationship between the two methods is not yet clear (Pallarés et al., 2016). Recent findings support the idea that a training model based on ventilatory thresholds (VT1 and VT2) could be very effective to set individual exercise intensity in endurance sports given that it takes into account individual metabolic responses (Wolpern et al., 2015). One of the most accurate systems to obtain these ventilatory responses is on the basis of gas exchange parameters using indirect calorimetry (Lucía et al., 2000; Gaskill et al., 2001; Pallarés et al., 2016). In VT1, the O2 and carbon dioxide production (CO2) increase proportionally, while HCO3− acts to buffer lactic acid concentration in blood (Wasserman et al., 1973; Del Coso et al., 2009); this intensity is ideal for high-volume low-intensity exercise (Stöggl and Sperlich, 2014). In turn, in VT2, the blood lactate accumulation boosts and rises considerably and the system collapses due to the homeostatic compromise and metabolic acidosis (Wasserman et al., 1973; Jones et al., 2007); this intensity sets a critical limit for high-intensity interval training (Stöggl and Sperlich, 2014). However, gas exchange systems require the use of expensive equipment and laboratory conditions which most teams, coaches, and athletes are not equipped with or cannot afford.
A further method to set individual exercise intensity is based on capillary blood lactate (CBL) measurements (Beneke et al., 2011). A number of authors have defined a list of CBL parameters associated with specific exercise intensities such as LT (Wasserman et al., 1973), maximal lactate steady state (MLSS, Beneke and von Duvillard, 1996), OBLA (onset of blood lactate accumulation, Sjödin and Jacobs, 1981), or the DMAX (Cheng et al., 1992). An accurate detection of MLSS is particularly important due to it being considered the highest intensity in which glycogen stores are the main exercise limiting factor (Coyle et al., 1986) and constitutes a prominent part of aerobic training in world-class athletes (García-Pallarés et al., 2009, 2010). Although CBL methods are commonly used for coaches to set individual training workloads, the relationship between lactate-based parameters and VTs load intensities is still an open debate. In cyclists, it seems clear that workloads at the VT1 are very related to LT (Lucía et al., 1999; Amann et al., 2006; Pallarés et al., 2016). However, the estimation of VT2 from lactate methods generates some controversy. Traditionally, VT2 intensities have been associated with MLSS (Svedahl and MacIntosh, 2003). In contrast with this assumption, recent evidence in cyclists demonstrates that MLSS encompasses a different metabolic pathway and limiting factor than VT, and constitutes a midpoint between VT1 and VT2 (Pallarés et al., 2016; Peinado et al., 2016). The determination of VT2 is essential due to represents a turn point at which metabolic acidosis cannot be buffered by ventilation (Lucía et al., 2000) and sets a critical limit for high-intensity training (Stöggl and Sperlich, 2014). One previous study conducted with cyclists has attempted to clarify the relationship between VTs and CBL methods, and reported a high reliability and validity of the following relationships: (1) VT1 and LT, (2) VT2 and LT+2 mMol·L−1, and (3) MLSS and LT+0.5 mMol·L−1 (Pallarés et al., 2016). To the best of our knowledge, there are no previous studies examining these relationships in runners. This is an important gap considering the existing differences between cycling and running, such as the more impaired ventilation in cycling and the higher muscle mass involved, greater muscle pump efficiency, and the implication of eccentric muscle actions in running (Bijker et al., 2002; Millet et al., 2009). Given that these differences may alter the physiological response to exercise, prescribing training plans for runners based on cyclists' reference values could be imprecise. Thus, the relationships between CBL and VTs intensities in runners need to be fully clarified.
In addition to the aerobic–anaerobic transition, another ventilation parameter to predict running performance is the MAS (McLaughlin et al., 2010), considered as the minimum speed at which O2max is reached (Lacour et al., 1991). As a rule of thumb, high intensity training in endurance athletes is established at 90–105% of the MAS (Stöggl and Sperlich, 2015). Given its importance for training plans and workload distribution, coaches and researchers have invested effort in designing maximal field tests to estimate the MAS and predict O2max in endurance athletes to establish the aerobic performance limits (Léger and Boucher, 1980; Berthon et al., 1997). However, these tests have important limitations: (1) the equations proposed to estimate the MAS from field tests are not based on accurate measurements such as gas exchange systems using indirect calorimetry (Lucía et al., 2000; Gaskill et al., 2001), and (2) maximal efforts criteria were not tested to ensure reaching values of O2max (ACSM, 2013). As stated above, a valid alternative to these field tests is to determine the MAS through GXT with 1-min increments using gas exchange systems. These short protocols allow the athletes to reach their maximal cardiac output, and therefore make possible obtaining a true Vpeak-value (Pallarés et al., 2016; Julio et al., 2017). Given that both MAS and Vpeak correspond to very similar intensities (Lacour et al., 1991) the calculation of an estimated MAS (MASEST) from the Vpeak, when gas exchange systems are not available, seems promising. However, this hypothesis is still to be proven.
Therefore, the aims of this study were (1) to establish the best fit between ventilatory and lactate exercise performance parameters in running and (2) to explore novel alternatives to estimate essential running performance indicators such as the MAS from similar intensity parameters like the Vpeak when gas exchange systems are not available.
Methods
Participants
Twenty-two trained male athletes (runners and triathletes) volunteered to participate in this study (age 25.9 ± 8.0 years, body mass 68.2 ± 6.1 kg, height 174.8 ± 5.8 cm, body fat 11.4 ± 1.9%, O2max 60.2 ± 4.3 ml·kg·min−1, endurance training experience 7.1 ± 4.0 years). All participants were competing at regional and national level races and following a regular training load of 4–6 days per week, 1–2 h per day. Measurements were obtained during the pre-competitive season. All participants underwent a complete medical examination (including ECG) that showed all were in good health. No physical limitations or musculoskeletal injuries that could affect testing procedures were reported. None of the subjects were taking drugs, medications, or dietary supplements known to influence physical performance. The Bioethics Commission of the University of Murcia approved the study, which was carried out according to the declaration of Helsinki. Subjects were verbally informed about the experimental procedures and possible risk and benefits. Written informed consent was obtained from all subjects.
Experimental Design
Participants visited the lab 5–7 times separated by 2–7 days. All participants had at least 6 months of familiarization with the testing procedures used in this investigation. On the first day, participants completed a preliminary GXT with 1-min increments (GXTPRE) to determine individuals' MAS and Vpeak, including 48–72 h rest before the next session. In the following two sessions, separated by 48 h, athletes performed two identical experimental GXT (GXTEXP 1 and GXTEXP 2). For these two GXTEXP protocols, initial running speed and workload increments were individually set according to participants' Vpeak previously determined in the GXTPRE.
The GXTEXP started with a 5-min warm-up at 13 km·h−1 less than each athlete's Vpeak followed, without a break, by a GXT 1-min (i.e., increments of 1 km·h−1·min−1) until exhaustion. Lastly, athletes came back to the lab two to three more times to perform a 30 min submaximal constant running test to determine the speed associated with the MLSS (Beneke, 2003). To maintain physical performance during the investigation period (2–3 weeks) participants followed an individual training protocol consisting in: running sessions (runners) or swimming, cycling, and running sessions (triathletes) of 90 min at individual VT1 intensity interspersed with efforts of 5–7 min at 90–95% of VT2 intensity each 20 min. Training sessions were repeated each 48 h with 24 h rest before each evaluation to ensure a full recovery.
Individualized Maximal Treadmill GXT Protocol
All the running trials were performed on the same treadmill (HP Cosmos Pulsar, HP Cosmos Sports and Medical GMBH, Nussdorf Traunstein, Germany) with an incline of 1.0% (Jones and Doust, 1996). Evaluations were performed under similar environmental conditions (21–24°C and 45–55% relative humidity) at the same time of day (16:00 to 19:00 h) to minimize the circadian rhythm effects (Mora-Rodríguez et al., 2015). Air ventilation was controlled with a fan positioned 1.5 m from the subject's chest at a wind velocity of 2.55 m·s−1.
The GXTPRE under medical supervision to fulfill three objectives: (1) discard cardiovascular diseases, (2) to minimize the bias of progressive learning on test reliability, and (3) to determine the athletes' MAS and Vpeak subsequently used to set up the individualized GXTEXP workload (i.e., treadmill speed). Participants' HR was monitored by standard 12 lead ECG (Quark T12, Cosmed, Italy), ventilatory performance (O2, O2max, and VE) was recorded on a breath-by-breath basis using a metabolic cart averaging data every 5 s (MetaLyzer 3B-R3, Cortex Biophysik GmbH, Leipzig, Germany) and the rate of perceived exertion (RPE) was assessed using the 6–20 Borg Scale (Borg, 1998) every 2 min. The MAS was determined from metabolic cart measurements as the first running velocity where O2max was reached (Billat and Koralsztein, 1996). The Vpeak was automatically obtained from the treadmill software using the Kuipers et al.'s formula (Kuipers et al., 2003): Vpeak = Vcomplete + (Inc * t/T), in which Vcomplete is the running velocity of the last complete stage, Inc is the speed increment (i.e., 1 km·h−1), t is the number of seconds sustained during the incomplete stage and T is the number of seconds required to complete a stage (i.e., 60 s).
The two GTXEXP were individually set up according to the MAS previously determined in the preliminary test (GXTPRE), as follows: starting with a 5-min warm-up at 13 km·h−1 less than each athlete's Vpeak, followed, without a break, by a GXT 1-min (i.e., increments of 1 km·h−1·min−1) until exhaustion. Ventilatory parameters and RPE were assessed as aforementioned in the GXTPRE. The HR was continuously monitored (V800, Polar, Finland). Capillary blood lactate samples from the finger were collected (Lactate Pro, Arkray, Japan) every 2 min (i.e., each 2 km·h−1 increments). The design of this particular protocol and its duration (min–max) were deliberate, given that: (1) It allows a clear detection of ventilatory thresholds (VT1 and VT2) by indirect calorimetry (Lucía et al., 1999, 2000; Pallarés et al., 2016); (2) It is effective in determining a true O2max (Midgley et al., 2007); (3) The protocol duration was short enough (12–14 min) to avoid the local acidosis and HR rise (cardiac drift) to obtain a true maximum cardiovascular performance (Dawson et al., 2005); (4) The short duration allows athletes to achieve a true MAS and Vpeak (Julio et al., 2017); and (5) By the end of the test, seven to nine capillary blood samples can be collected from each participant before exhaustion, which enable the plotting of a complete lactate curve. In particular, fingerprint blood samples were collected by a specialist placed beside the treadmill without any pause during the participants' running test (i.e., in movement) to make the process less invasive and ensure a constant effort during the GXT protocols.
Maximal effort criteria (ACSM, 2013) were considered to verify the outcomes, from which participants must reach at least three from the list: (i) failure of HR to increase with further increases in exercise intensity; (ii) a plateau in O2 (or failure to increase O2 by 150 mL·min−1) with increased workload; (iii) a respiratory exchange ratio (RER) ≥1.10; CBL >8 mmol·L−1; (iv) a rating of perceived exertion (RPE) >17 on the 6–20 scale. If verified, physiological parameters were determined, and the individuals' treadmill speed at each of the physiological parameters studied were considered for subsequent analysis. Blood lactate analyzer and indirect calorimetry devices were calibrated before each test according to the manufacturer's instructions.
Determination of MLSS
Several 30 min constant workloads on a treadmill were performed to identify the highest workload (km·h–1) at which CBL increased >1 mMol·L–1 between the 10th and 30th min of exercise (Beneke, 2003). After 7 days from the second GXTEXP, all participants performed the first MLSS trial at the individual workload associated to their 85% of VT2, based on previous studies (Llodio et al., 2016; Pallarés et al., 2016). Depending on the results of the first MLSS-test, successive trials with a 48-h rest between sessions were increased or decreased 0.5 km·h–1 until MLSS criteria was fulfilled (Pallarés et al., 2016).
Determination of Ventilation Parameters
VT1 was determined using the criteria of an increase in both ventilatory equivalent of oxygen (E/O2) and end-tidal pressure of oxygen (PETO2) with no concomitant increase in ventilatory equivalent of carbon dioxide (E/CO2). VT2 was determined using the criteria of an increase in both the E/O2 and E/CO2 and a decrease in PETCO2 (Lucía et al., 2000; Figures 1A,B). V-Slope load was identified in that intensity of exercise which, in a plot of the minute production of CO2 over the minute utilization of oxygen (O2), shows an increase in the slope above 1.0 (Wasserman et al., 1973; Gaskill et al., 2001). The O2max was defined as the highest plateau (two successive maximal within 150 mL·min−1, averaging the data every 5 s) reached. MAS was defined as the minimum speed at which maximum oxygen uptake O2max is reached (Lacour et al., 1991). Vpeak was taken from the highest velocity reached during this GXT protocol and calculated according to the Kuipers et al. (2003).
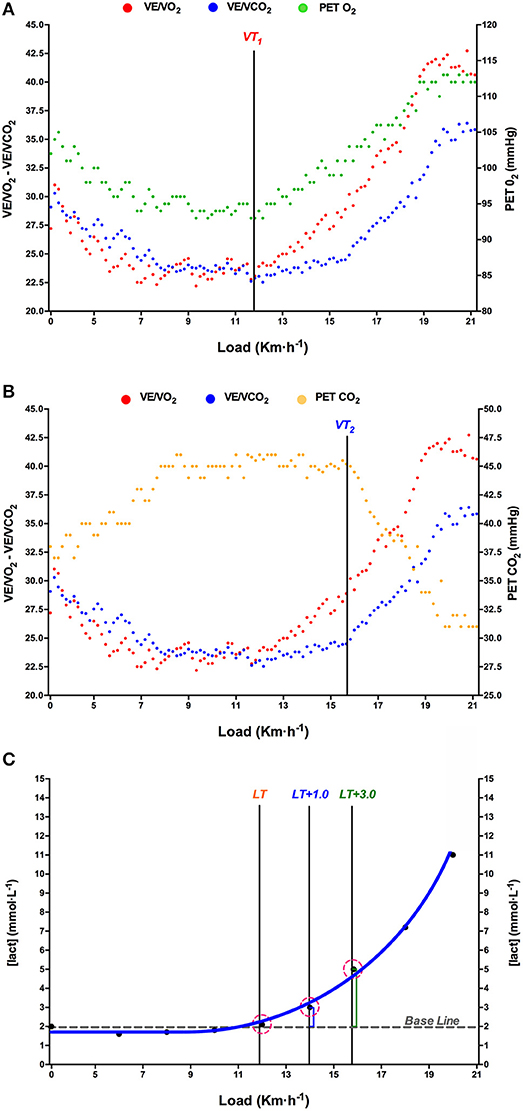
Figure 1. Example of determination of ventilatory thresholds (VT1, A; VT2, B), and lactate threshold (LT, C) in one test. Each gas-exchange data point corresponds to a 5-s interval. E/O2, ventilatory equivalent for oxygen; E/CO2, ventilatory equivalent for carbon dioxide; PETCO2, end-tidal pressure of oxygen; end-tidal pressure of carbon dioxide (PETCO2).
Determination of Lactate Parameters
LT was determined by examining the CBL speed relationship ([Lact]blood/ km·h−1) during the GXT as the highest speed not associated with a rise in CBL above baseline (Weltman et al., 1990). Baseline CBL was the average during the initial stages with values 0.8 mMol·L−1 above rest state. This always occurred just before the curvilinear increase in blood lactate observed at subsequent exercise intensities (Coyle et al., 1983; Lucía et al., 2000). Lactate Threshold + 1.0 mMol·L−1 (LT+1.0) represents the speed which causes an increase of 1 mMol·L−1 above baseline measurements (Coyle et al., 1983). Following this criterion, five LT-based events were established as previously described (Pallarés et al., 2016): LT+0.5, LT+1, LT+1.5, LT+2.0, LT+2.5, and LT+3.0 mMol·L−1. DMAX method was determined by plotting the lactate response to exercise intensity in a third-order polynomial regression curve. DMAX was defined as the point on the regression curve that yields the maximal distance to the straight line formed by the two end points of the curve (Cheng et al., 1992). Onset of blood lactate accumulation (OBLA4mM) was defined as the exercise intensity identified by interpolation across the 4 mMol·L−1 point in the plot of [Lact]blood during incremental exercise (Sjödin and Jacobs, 1981). Two independent observers detected all ventilatory and LT following the criteria previously described. If they did not agree, the opinion of a third investigator was sought (Lucía et al., 1999; Figure 1C).
Statistical Analyses
Standard statistical methods were used for the calculation of means, standard deviations (SD), and 95% confidence interval. The reliability of ventilation and lactate parameters was analyzed comparing the consistence among trials (i.e., GXTEXP 1 vs. GXTEXP 2) by calculating the coefficient of variation (CV), intraclass correlation coefficient (ICC), and Bland–Altman plots. Linear regression analysis was employed to estimate a theoretical MAS (MASEST) from the average of the Vpeak achieved at the end of the two GXTEXP trials. Validity analysis of the ventilatory thresholds (VT1 and VT2), MLSS, and MAS against the other parameters was conducted over the means obtained in the trials by ANOVA, ICC, and Bland-Altman bias. Analyses were performed using GraphPad Prism 6.0 (GraphPad Software, Inc., CA, USA) and SPSS software version 19.0 (IBM Corp., Armonk, NY, USA).
Results
All participants reached at least two of the criteria for achievement of maximal efforts during all the GXT-tests, therefore maximal ventilation and cardiovascular performance was verified. The initial speed ranged from 6 to 10 km·h−1 and no fatigue was detected following the warm-up (i.e., all participants maintained a RER < 0.85 and CBL under the baseline). The Vpeak reached during the GXTPRE ranged from 18 to 22 km·h−1. Linear regression analysis (Figure 2) revealed a very strong association between MAS and Vpeak (p < 0.01; r = 0.954; SEM = 0.3 km·h−1) and yielded the equation:
Intra-subject reliability between GXTEXP trials (Table 1) revealed low CV (<3.1%), low bias (<0.5 km·h−1), and high correlation (ICC > 0.91) for all determinations except V-Slope (ICC = 0.84).
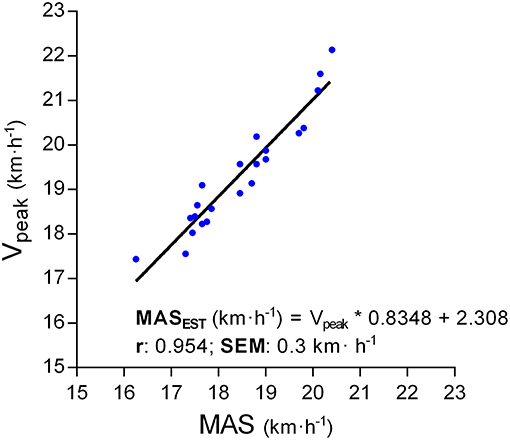
Figure 2. Linear regression estimating a theoretical maximal aerobic speed (MASEST) from the average of the fastest velocity achieved at the end of the GXTEXP trials (Vpeak).
Table 2 shows the validity analysis comparing VT1, MLSS, VT2, and MAS workloads against the rest of the parameters. The strongest associations (ICC > 0.82, p > 0.57) were: VT1 with LT (−0.3 ± 1.2 km·h−1), MLSS with LT+1.0 (−0.2 ± 1.2 km·h−1), VT2 with LT+3.0 (<0.1 ± 1.3 km·h−1), and MAS with MASEST (<0.1 ± 0.4 km·h−1).
Table 3 shows the 95% confidence interval for main physiological parameters under study.
Discussion
The main findings of the current study were that (1) LT obtained during a 12–14 min, 1 km·h−1 per minute GXT is a valid method to determine the main physiological parameters of the aerobic–anaerobic transition, (2) LT, LT+1 and LT+3.0 are solid predictors of VT1, MLSS, and VT2, respectively, (3) the MLSS was identified as a midpoint between VT1 and VT2, and (4) an estimated maximal aerobic speed (MASEST) can be accurately obtained (error ± 0.3 km·h−1) from the fastest speed achieved during the current GXT (Vpeak). This study adds to the existing literature by providing a valid alternative test based on blood lactate to obtain performance workloads without the need of using indirect calorimetry (less affordable technology). In addition, we contribute with an accurate method to estimate the MAS, which is one of the most used indicators to set training intensities in running. To our knowledge, this is the first report examining the validity and reliability of such an extensive battery of tests and parameters to determine critical workloads in runners.
The high reliability values found in physiological measurements between the two GXTEXP treadmill trials concurs with those previously reported in cycle ergometer (Pallarés et al., 2016). In addition, our results allow us to discourage using V-Slope when other parameters are available. Although the causes that might explain these effects are very difficult to isolate and quantify, it is arguable that an individualized workload adjustment approach accounted for these increments (García-Pallarés et al., 2009; Wolpern et al., 2015). In the current GXT with 1-min increments, athletes started at 13 km·h−1 below their Vpeak, previously determined during the GXTPRE session. By doing this, it is guaranteed that the athlete is running at the optimal intensity to end up at their maximum workload after 12–14 min avoiding cardiac drift, local acidosis, and allowing a clear detection of ventilatory and LT, additionally getting maximal values of O2max Considering this information, individual GXT protocols based on athletes' maximal speed should be developed to enhance the consistency of data during physiological evaluations.
A number of studies have investigated the relationship between ventilatory threshold and blood lactate concentration in endurance athletes. Authors agreed that workloads at the first ventilatory threshold (i.e., VT1) are strongly related to the workload at which lactate starts to increase above resting values (LT; Wasserman et al., 1973; Lucía et al., 2000; Pallarés et al., 2016). Our findings corroborate this association between VT1 and LT but showing a greater external workload in runners (VT1 = 59–65% of MAS) compared to cyclists [VT1 ~ 51.5% of maximal aerobic power (MAP)]. These findings suggest that running describes a great relative external workload associated with the VT1 response. Therefore, smaller errors in detecting ventilatory thresholds may have a greater negative impact on the running performance compared to other disciplines like cycling, for instance, misguided training prescription, undesirable physical adaptations, and a greater probability of the appearance of the interference phenomenon during concurrent training (García-Pallarés and Izquierdo, 2011). In turn, there is no clear agreement about which LT better reflects VT2 intensities. A previous experiment in cyclists (Pallarés et al., 2016) determined a high correlation between VT2 and LT+2 mMol·L−1, followed by the OBLA4mM, which established high intensities at ~80% of their maximal aerobic power (MAP). Interestingly enough, we identified a greater CBL in runners during the transition phase, locating the VT2 at LT+3 mMol·L−1 intensities, setting the high intensity limit at 84–87% of the MAS. The existing physiological differences between running and cyclists may explain these disparities. Millet et al. (2009) reviewed the literature and identified a list of potential distinguishing factors between running and cycling physiological demands. The authors pointed out differences on ventilatory responses to exercise in terms of exercise-induced arterial hypoxaemia, O2 diffusion capacity, ventilatory fatigue, and pulmonary mechanics. Moreover, other factors like running/cycling economy (higher delta efficiency in running), muscle recruitment patterns (greater muscle mass involved and eccentric phase activity in running), and ventilation impairment (higher in cycling) may account for these differences.
The MLSS constitutes another essential physiological event in endurance performance, as it is the maximal workload that can be maintained without elevations in blood lactate concentration (MLSS). Previous authors have proposed that MLSS workload coincides with the one for VT2 (Smekal et al., 2012). In contrast to this assumption, recent investigations in cyclists elucidated that MLSS may correspond to a lower exercise intensity of VT2 and matches better with the midpoint between both ventilatory thresholds (Pallarés et al., 2016; Peinado et al., 2016). In support of this theory, our findings revealed that MLSS intensity (72–74% of MAS) constitutes a transition between VT1 (59–65% of MAS) and VT2 (84–87% of MAS). Moreover, MLSS was highly associated with LT+1 mMol·L−1. In cyclists, MLSS has been associated with LT+0.5 mMol·L−1 (Pallarés et al., 2016). These increments on CBL at the same relative intensity might indicate a greater energy cost in running at MLSS workload, which may imply earlier fatigue and lower performance by accelerating glycogen depletion (Coyle et al., 1986).
A main contribution of the current study is to provide an estimated MAS (MASEST) from the maximal speed achieved (Vpeak) at the end of the GXT protocol with a minimal error of ±0.3 km·h−1. Main physiological events (VT and MLSS) are related to a given percentage of MAS (Pallarés et al., 2016), therefore the estimation of MAS would allow coaches to determine effective working ranges (Table 3) and training zones (Table 4) with an error of <0.5%. Although there are other track tests to estimate the MAS (e.g., Léger and Boucher, 1980), the current protocol adds to the existing methods the possibility to design individualized training routines based on athletes' MAS without the need of indirect calorimetry o CBL records. It is important to mention that, given the originality of the proposal, the current outcomes have been shown to be valid only for the subject group that was tested. Future research should extend these findings to examine the validity of the MASEST compared to estimations from existing field tests.
It is noteworthy that, given the high inter-individual response of training adaptations in endurance exercise (Bouchard et al., 1986), a similar workload distribution may not have the same effect among athletes, even if they are from the same discipline and compete at high level (Stöggl and Sperlich, 2015). In this case, an individual assessment is required to detect specific workloads. However, this implies indirect calorimeters methods which are expensive and out of reach for the majority of coaches and athletes. In this study, we provide a valid and reliable alternative to estimate critical workloads (VT1, MLSS, and VT2) using a cheaper and affordable method such as CBL. Furthermore, the current GXT individualized protocol (i.e., starting at 13 km·h–1 below the athlete's MAS with increments of 1 km·h–1/min until) appears to be a promising method to determine training zones in well-trained runners. What is now required is to test the effectiveness of training plans according to the current 5-zone proposal (Table 4). In addition, future investigations should examine the validity of this protocol in amateur and female runners to enhance its applicability within the endurance sport community.
Ethics Statement
All procedures performed in this study involving human participants were in accordance with the ethical standards of the institutional Human Research Ethics Committee and with the 1964 Helsinki declaration and its later amendments or comparable ethical standards. The study was approved by the Bioethics Commission of the University of Murcia. Written informed consent was obtained from all subjects prior to participation.
Author Contributions
JP and VC-E: Conception and design of the experiments; JP, VC-E, RM-N, and AM-C: Pre-testing, experimental preparation, and data collection; VC-E, JC-I, and JP: data analysis. The first draft of the manuscript was written by VC-E, JC-I, and JP. All co-authors edited and proofread the manuscript and approved the final version.
Conflict of Interest Statement
The authors declare that the research was conducted in the absence of any commercial or financial relationships that could be construed as a potential conflict of interest.
Acknowledgments
The authors wish to thank the subjects for their invaluable contribution to the study.
References
ACSM (2013). ACSM's Guidelines for Exercise Testing and Prescription, 9th Edn. Philadelphia, PA: Lippincott Williams and Wilkins.
Amann, M., Subudhi, A. W., and Foster, C. (2006). Predictive validity of ventilatory and lactate thresholds for cycling time trial performance. Scand. J. Med. Sci. Sports 16, 27–34. doi: 10.1111/j.1600-0838.2004.00424.x
Beneke, R. (2003). Methodological aspects of maximal lactate steady state-implications for performance testing. Eur. J. Appl. Physiol. 89, 95–99. doi: 10.1007/s00421-002-0783-1
Beneke, R., Leithäuser, R. M., and Ochentel, O. (2011). Blood lactate diagnostics in exercise testing and training. Int. J. Sports Physiol. Perform. 6, 8–24. doi: 10.1123/ijspp.6.1.8
Beneke, R., and von Duvillard, S. P. (1996). Determination of maximal lactate steady state response in selected sports events. Med. Sci. Sports Exerc. 28, 241–246. doi: 10.1097/00005768-199602000-00013
Bentley, D. J., Newell, J., and Bishop, D. (2007). Incremental exercise test design and analysis: implications for performance diagnostics in endurance athletes. Sports Med. 37, 575–586. doi: 10.2165/00007256-200737070-00002
Berthon, P., Fellmann, N., Bedu, M., Beaune, B., Dabonneville, M., Coudert, J., and Chamoux, A. (1997). A 5-min running field test as a measurement of maximal aerobic velocity. Eur. J. Appl. Physiol. Occup. Physiol. 75, 233–238. doi: 10.1007/s004210050153
Bijker, K. E., de Groot, G., and Hollander, A. P. (2002). Differences in leg muscle activity during running and cycling in humans. Eur. J. Appl. Physiol. 87, 556–561. doi: 10.1007/s00421-002-0663-8
Billat, L. V., and Koralsztein, J. P. (1996). Significance of the velocity at VO2max and time to exhaustion at this velocity. Sports Med. 22, 90–108. doi: 10.2165/00007256-199622020-00004
Bouchard, C., Lesage, R., Lortie, G., Simoneau, J. A., Hamel, P., Boulay, M. R., et al. (1986). Aerobic performance in brothers, dizygotic and monozygotic twins. Med. Sci. Sports Exerc. 18, 639–646. doi: 10.1249/00005768-198612000-00006
Buchfuhrer, M. J., Hansen, J. E., Robinson, T. E., Sue, D. Y., Wasserman, K., and Whipp, B. J. (1983). Optimizing the exercise protocol for cardiopulmonary assessment. J. Appl. Physiol. 55, 1558–1564. doi: 10.1152/jappl.1983.55.5.1558
Cheng, B., Kuipers, H., Snyder, A. C., Keizer, H. A., Jeukendrup, A., and Hesselink, M. (1992). A new approach for the determination of ventilatory and lactate thresholds. Int. J. Sports Med. 13, 518–522. doi: 10.1055/s-2007-1021309
Coyle, E. F., Coggan, A. R., Hemmert, M. K., and Ivy, J. L. (1986). Muscle glycogen utilization during prolonged strenuous exercise when fed carbohydrate. J. Appl. Physiol. 61, 165–172. doi: 10.1152/jappl.1986.61.1.165
Coyle, E. F., Martin, W. H., Ehsani, A. A., Hagberg, J. M., Bloomfield, S. A., Sinacore, D. R., and Holloszy, J. O. (1983). Blood lactate threshold in some well-trained ischemic heart disease patients. J. Appl. Physiol. 54, 18–23. doi: 10.1152/jappl.1983.54.1.18
Dawson, E. A., Shave, R., George, K., Whyte, G., Ball, D., Gaze, D., and Collinson, P. (2005). Cardiac drift during prolonged exercise with echocardiographic evidence of reduced diastolic function of the heart. Eur. J. Appl. Physiol. 94, 305–309. doi: 10.1007/s00421-005-1318-3
Del Coso, J., Hamouti, N., Aguado-Jimenez, R., and Mora-Rodriguez, R. (2009). Respiratory compensation and blood pH regulation during variable intensity exercise in trained versus untrained subjects. Eur. J. Appl. Physiol. 107, 83–93. doi: 10.1007/s00421-009-1101-y
di Prampero, P. E., Atchou, G., Brückner, J. C., and Moia, C. (1986). The energetics of endurance running. Eur. J. Appl. Physiol. Occup. Physiol. 55, 259–266.
Esteve-Lanao, J., Foster, C., Seiler, S., and Lucia, A. (2007). Impact of training intensity distribution on performance in endurance athletes. J. Strength Cond. Res. 21, 943–949. doi: 10.1519/R-19725.1
Farrell, P. A., Wilmore, J. H., Coyle, E. F., Billing, J. E., and Costill, D. L. (1979). Plasma lactate accumulation and distance running performance. Med. Sci. Sports 11, 338–344.
García-Pallarés, J., García-Fernández, M., Sánchez-Medina, L., and Izquierdo, M. (2010). Performance changes in world-class kayakers following two different training periodization models. Eur. J. Appl. Physiol. 110, 99–107. doi: 10.1007/s00421-010-1484-9
García-Pallarés, J., and Izquierdo, M. (2011). Strategies to optimize concurrent training of strength and aerobic fitness for rowing and canoeing. Sports Med. 41, 329–343. doi: 10.2165/11539690-000000000-00000
García-Pallarés, J., Sánchez-Medina, L., Carrasco, L., Díaz, A., and Izquierdo, M. (2009). Endurance and neuromuscular changes in world-class level kayakers during a periodized training cycle. Eur. J. Appl. Physiol. 106, 629–638. doi: 10.1007/s00421-009-1061-2
Gaskill, S. E., Ruby, B. C., Walker, A. J., Sanchez, O. A., Serfass, R. C., and Leon, A. S. (2001). Validity and reliability of combining three methods to determine ventilatory threshold. Med. Sci. Sports Exerc. 33, 1841–1848. doi: 10.1097/00005768-200111000-00007
Jones, A. M., and Carter, H. (2000). The effect of endurance training on parameters of aerobic fitness. Sports Med. 29, 373–386. doi: 10.2165/00007256-200029060-00001
Jones, A. M., and Doust, J. H. (1996). A 1% treadmill grade most accurately reflects the energetic cost of outdoor running. J. Sports Sci. 14, 321–327. doi: 10.1080/02640419608727717
Jones, A. M., Wilkerson, D. P., DiMenna, F., Fulford, J., and Poole, D. C. (2007). Muscle metabolic responses to exercise above and below the “critical power” assessed using 31P-MRS. Am. J. Physiol. Regul. Integr. Comp. Physiol. 294, R585–R593. doi: 10.1152/ajpregu.00731.2007
Julio, U. F., Panissa, V. L. G., Shiroma, S. A., and Franchini, E. (2017). Effect of protocol manipulation for determining maximal aerobic power on a treadmill and cycle ergometer: a brief review. Strength Cond. J. 5, 58–71. doi: 10.1519/SSC.0000000000000350
Kuipers, H., Rietjens, G., Verstappen, F., Schoenmakers, H., and Hofman, G. (2003). Effects of stage duration in incremental running tests on physiological variables. Int. J. Sports Med. 24, 486–491. doi: 10.1055/s-2003-42020
Lacour, J. R., Padilla-Magunacelaya, S., Chatard, J. C., Arsac, L., and Barthélémy, J. C. (1991). Assessment of running velocity at maximal oxygen uptake. Eur. J. Appl. Physiol. Occup. Physiol. 62(2), 77–82. doi: 10.1007/BF00626760
Léger, L., and Boucher, R. (1980). An indirect continuous running multistage field test: the Université de Montréal track test. Can. J. Appl. Sport Sci. 5, 77–84.
Llodio, I., Gorostiaga, E., Garcia-Tabar, I., Granados, C., and Sánchez-Medina, L. (2016). Estimation of the maximal lactate steady state in endurance runners. Int. J. Sports Med. 37, 539–546. doi: 10.1055/s-0042-102653
Lucía, A., Hoyos, J., Pérez, M., and Chicharro, J. L. (2000). Heart rate and performance parameters in elite cyclists: a longitudinal study. Med. Sci. Sports Exerc. 32, 1777–1782. doi: 10.1097/00005768-200010000-00018
Lucía, A., Sánchez, O., Carvajal, A., and Chicharro, J. L. (1999). Analysis of the aerobic-anaerobic transition in elite cyclists during incremental exercise with the use of electromyography. Br. J. Sports Med. 33, 178–185. doi: 10.1136/BJSM.33.3.178
Lukaski, H. C., Bolonchuk, W. W., and Klevay, L. M. (1989). Comparison of metabolic responses and oxygen cost during maximal exercise using three treadmill protocols. J. Sports Med. Phys. Fitness 29, 223–229. doi: 10.1519/JSC.0000000000001193
Mann, T. N., Lamberts, R. P., and Lambert, M. I. (2014). High responders and low responders: factors associated with individual variation in response to standardized training. Sports Med. 44, 1113–1124. doi: 10.1007/s40279-014-0197-3
McLaughlin, J. E., Howley, E. T., Bassett, D. R., Thompson, D. L., and Fitzhugh, E. C. (2010). Test of the classic model for predicting endurance running performance. Med. Sci. Sports Exerc. 42, 991–997. doi: 10.1249/MSS.0b013e3181c0669d
Midgley, A. W., McNaughton, L. R., and Carroll, S. (2007). Time at VO2max during intermittent treadmill running: test protocol dependent or methodological artefact? Int. J. Sports Med. 28, 934–939. doi: 10.1055/s-2007-964972
Millet, G. P., Vleck, V. E., and Bentley, D. J. (2009). Physiological differences between cycling and running: lessons from triathletes. Sports Med. 39, 179–206. doi: 10.2165/00007256-200939030-00002
Mora-Rodríguez, R., Pallarés, J. G., López-Gullón, J. M., López-Samanes, Á, Fernández-Elías, V. E., and Ortega, J. F. (2015). Improvements on neuromuscular performance with caffeine ingestion depend on the time-of-day. J. Sci. Med. Sport 18, 338–342. doi: 10.1016/j.jsams.2014.04.010
Pallarés, J. G., Moran-Navarro, R., Fernando Ortega, J., Emilio Fernandez-Elias, V., and Mora-Rodriguez, R. (2016). Validity and reliability of ventilatory and blood lactate thresholds in well-trained cyclists. PLoS ONE 11:e0163389. doi: 10.1371/journalpone/e0163389
Peinado, A. B., Filho, D. P., Díaz, V., Benito, P. J., Álvarez-Sánchez, M., Zapico, A. G., and Calderón, F. J. (2016). The midpoint between ventilatory thresholds approaches maximal lactate steady state intensity in amateur cyclists. Biol. Sport 33, 373–380. doi: 10.5604/20831862.1221812
Scharhag-Rosenberger, F., Walitzek, S., Kindermann, W., and Meyer, T. (2012). Differences in adaptations to 1 year of aerobic endurance training: individual patterns of nonresponse. Scand. J. Med. Sci. Sports 22, 113–118. doi: 10.1111/j.1600-0838.2010.01139.x
Sjödin, B., and Jacobs, I. (1981). Onset of blood lactate accumulation and marathon running performance. Int. J. Sports Med. 2, 23–26. doi: 10.1055/s-2008-1034579
Smekal, G., von Duvillard, S. P., Pokan, R., Hofmann, P., Braun, W. A., Arciero, P. J., et al. (2012). Blood lactate concentration at the maximal lactate steady state is not dependent on endurance capacity in healthy recreationally trained individuals. Eur. J. Appl. Physiol. 112, 3079–3086. doi: 10.1007/s00421-011-2283-7
Stöggl, T., and Sperlich, B. (2014). Polarized training has greater impact on key endurance variables than threshold, high intensity, or high volume training. Front. Physiol. 5:33. doi: 10.3389/fphys.2014.00033
Stöggl, T., and Sperlich, B. (2015). The training intensity distribution among well-trained and elite endurance athletes. Front. Physiol. 6:295. doi: 10.3389/fphys.2015.00295
Stratton, E., O'Brien, B. J., Harvey, J., Blitvich, J., McNicol, A. J., Janissen, D., et al. (2009). Treadmill velocity best predicts 5000-m run performance. Int. J. Sports Med. 30, 40–45. doi: 10.1055/s-2008-1038761
Svedahl, K., and MacIntosh, B. R. (2003). Anaerobic threshold: the concept and methods of measurement. Can. J. Appl. Physiol. 28, 299–323. doi: 10.1139/h03-023
Wasserman, K., Whipp, B. J., Koyl, S. N., and Beaver, W. L. (1973). Anaerobic threshold and respiratory gas exchange during exercise. J. Appl. Physiol. 35, 236–243. doi: 10.1152/jappl.1973.35.2.236
Weltman, A., Snead, D., Stein, P., Seip, R., Schurrer, R., Rutt, R., and Weltman, J. (1990). Reliability and validity of a continuous incremental treadmill protocol for the determination of lactate threshold, fixed blood lactate concentrations, and VO2max. Int. J. Sports Med. 11, 26–32. doi: 10.1055/s-2007-1024757
Keywords: blood lactate, ventilation threshold, maximal aerobic speed, VO2max, endurance, maximal lactate steady state
Citation: Cerezuela-Espejo V, Courel-Ibáñez J, Morán-Navarro R, Martínez-Cava A and Pallarés JG (2018) The Relationship Between Lactate and Ventilatory Thresholds in Runners: Validity and Reliability of Exercise Test Performance Parameters. Front. Physiol. 9:1320. doi: 10.3389/fphys.2018.01320
Received: 21 March 2018; Accepted: 31 August 2018;
Published: 25 September 2018.
Edited by:
Martin Burtscher, Universität Innsbruck, AustriaReviewed by:
Hannes Gatterer, EURAC Research, ItalyRicardo Mora-Rodriguez, Universidad de Castilla-La Mancha, Spain
Copyright © 2018 Cerezuela-Espejo, Courel-Ibáñez, Morán-Navarro, Martínez-Cava and Pallarés. This is an open-access article distributed under the terms of the Creative Commons Attribution License (CC BY). The use, distribution or reproduction in other forums is permitted, provided the original author(s) and the copyright owner(s) are credited and that the original publication in this journal is cited, in accordance with accepted academic practice. No use, distribution or reproduction is permitted which does not comply with these terms.
*Correspondence: Jesús G. Pallarés, amdwYWxsYXJlc0B1bS5lcw==