- 1 College of Engineering, Nihon University, Koriyama, Japan
- 2 Department of Medical Education, Nagoya City University Graduate School of Medical Sciences, Nagoya, Japan
- 3 Department of Neurology, Graduate School of Medicine, University of Tokyo, Tokyo, Japan
- 4 Department of Cardiology, Fujita Health University School of Medicine, Toyoake, Japan
- 5 Educational Physiology Laboratory, Graduate School of Education, University of Tokyo, Tokyo, Japan
The correlates of indices of long-term ambulatory heart rate variability (HRV) of the autonomic nervous system have not been completely understood. In this study, we evaluated conventional HRV indices, obtained from the daytime (12:00–18:00) Holter recording, and a recently proposed non-Gaussianity index (λ; Kiyono et al., 2008) in 12 patients with multiple system atrophy (MSA) and 10 patients with Parkinson disease (PD), known to have varying degrees of cardiac vagal and sympathetic dysfunction. Compared with the age-matched healthy control group, the MSA patients showed significantly decreased HRV, most probably reflecting impaired vagal heart rate control, but the PD patients did not show such reduced variability. In both MSA and PD patients, the low-to-high frequency (LF/HF) ratio and the short-term fractal exponent α1, suggested to reflect the sympathovagal balance, were significantly decreased, as observed in congestive heart failure (CHF) patients with sympathetic overdrive. In contrast, the analysis of the non-Gaussianity index λ showed that a marked increase in intermittent and non-Gaussian HRV observed in the CHF patients was not observed in the MSA and PD patients with sympathetic dysfunction. These findings provide additional evidence for the relation between the non-Gaussian intermittency of HRV and increased sympathetic activity.
Introduction
The correlates of indices of long-term ambulatory heart rate variability (HRV) of the autonomic nervous system have not been completely understood. In particular, there is yet no established index for sympathetic activation, and most HRV indices proposed primarily reflect reduced or impaired vagal function (Camm et al., 1996; Marine et al., 2002; Bauer et al., 2006). Considering a key role played by the sympathetic overdrive as one of the universal precipitating factors for various chronic illnesses (McEwen, 1998, 2007) and as a factor responsible for cardiac electrical instability (Schwartz et al., 1984), the quest for HRV indices probing sympathetic activation would be of great importance.
As a marker potentially related to the sympathetic cardiac overdrive, we have recently proposed increased non-Gaussianity of HRV (Kiyono et al., 2008). This form of non-Gaussianity has been used in fluid dynamics for characterizing intermittency of turbulence (Castaing et al., 1990). When applied to HRV analysis, it captures the occurrence of intermittent heart rate increments (Kiyono et al., 2004, 2007). In a cohort of congestive heart failure (CHF), Kiyono et al. (2008) initially observed that the increased non-Gaussianity of HRV predicts increased mortality risk, while none of the conventional HRV indices, including those reflecting vagal heart rate control, were predictive of death among these patients. More recently, Hayano et al. (2011) also reported that the increased non-Gaussianity index, λ25s, which captures intermittent heart rate increments within a scale of 25 s similar to that used in the study by Kiyono et al. (2008), is associated with increased cardiac mortality risk in a cohort of acute myocardial infarction (AMI), with the predictive power independent of other HRV indices. As heart rate fluctuations in the scale within a minute are mediated almost exclusively by neural autonomic activities (Camm et al., 1996), but λ25s showed no substantial correlation with vagally mediated HRV indices and the patients taking β-blockers had lower λ25s, Hayano et al. (2011) conjectured that the non-Gaussianity index in this scale probably captures heart rate fluctuations mediated by intermittent activations of cardiac sympathetic activity, affecting independently the mortality of cardiac patients.
In the present study, this conjecture is more directly tested by studying long-term ambulatory HRV in patients with multiple system atrophy (MSA). MSA is a sporadic and rapidly progressive neurodegenerative disorder that presents with autonomic failure in combination with Parkinsonism or cerebellar ataxia (Wenning et al., 2008; Stefanova et al., 2009). The autonomic symptoms are believed to be due to neuropathological abnormalities in both preganglionic sympathetic (Sone et al., 2005) and vagal (Benarroch et al., 2006) neurons. In previous HRV studies, decreased high frequency, vagally mediated HRV was observed in MSA patients than in age-matched healthy controls (Gurevich et al., 2004; Kuriyama et al., 2005), resembling the reduced or impaired vagal function in cardiac patients (Camm et al., 1996; Bauer et al., 2006). In contrast, because of degeneration of the preganglionic sympathetic neurons, it is hypothesized that the non-Gaussianity of HRV fails to markedly increase, such as that observed in cardiac patients (Kiyono et al., 2008; Hayano et al., 2011), in MSA patients. We test this hypothesis by comparing the results for MSA with those for CHF (Kiyono et al., 2008); the results were reanalyzed in the same methodological framework.
In the present study, we also studied ambulatory HRV in patients with Parkinson disease (PD) in which autonomic failure is commonly observed (Lipp et al., 2009). As the autonomic pathology of PD is different from that of MSA, being primarily postganglionic as evidenced by decreased uptake of adrenergic markers such as iodine-123 metaiodobenzylguanidine (Braune et al., 1998, 1999), the degree, and balance of sympathetic and vagal impairments could be different. Thus, it would be intriguing to examine if the lack of increased non-Gaussianity is still observed in PD.
Materials and Methods
Study Patients
Twelve MSA patients (six male and six female subjects; 61.9 ± 7.1, 54–76 years) and 10 patients with PD (two male and eight female subjects; 71.1 ± 6.0, 63–81 years) at the Department of Neurology of the University of Tokyo Hospital participated in this study (Tables 1 and 2, respectively). Diagnosis was made according to the UK Parkinson’s Disease Society Brain Bank Clinical Diagnostic Criteria (Hughes et al., 1992) and the second consensus statement on MSA diagnosis (Gilman et al., 2008). All patients were examined by neurologists, and all PD patients exhibited a response to L-DOPA without remarkable MRI findings. All MSA patients fulfilled the criteria for probable MSA (Gilman et al., 2008), and most of them took adrenergic stimulants for controlling severe orthostatic hypotension and anti-adrenergic or anti-muscarinic medications for their neurogenic bladder.
In addition, we studied 108 patients who were consecutively referred for evaluation or treatment of CHF (61 male and 47 female subjects; 66.1 ± 14.8, 21–92 years). Of these patients, 39 (36.1%) died within the follow-up period of 33 ± 17 months (range, 1–59 months). The medication status before discharge from the hospital was not significantly different between survivors and non-survivors. The clinical details of the CHF patients were reported previously (Kiyono et al., 2008).
The results were compared with data from age-matched healthy subjects; the details of which were reported elsewhere (Kiyono et al., 2004). All individuals within ±2 years of each patient’s age were selected from a pool of 122 healthy subjects.
Measurements and Protocol
The original electrocardiogram (ECG) data were derived from 24-h Holter recordings. The ECG signals were digitized at 125 Hz and 12 bits and processed offline using a personal computer equipped with a dedicated software. All QRS complexes in each recording were detected and labeled automatically. The results of automatic analysis were reviewed, and any errors in R wave classification were corrected manually. Computer files were generated containing the duration of individual R–R intervals and morphology classifications of individual QRS complexes (normal, supraventricular, and ventricular premature complexes). The series of intervals between two successive R waves of sinus rhythm [normal-to-normal (NN) intervals] was analyzed. To avoid the adverse effects of any remaining errors in the detection of the R wave, large (>20%) consecutive R–R interval differences were thoroughly reviewed until all errors were corrected. In addition, when atrial or ventricular premature complexes were encountered, the corresponding R–R intervals were interpolated by the median of the two successive beat-to-beat intervals. We also confirmed that no sustained tachyarrhythmias were present in our HRV recordings. In this study, all HRV indices were obtained from the daytime (12:00–18:00) data.
Analysis of Conventional HRV Indices
The following HRV indices were calculated: mean NN intervals, standard deviation (SD) of all NN intervals (SDNN), SD of 5 min averaged NN intervals (SDANN), root mean square of successive difference of NN intervals (RMSSD), the variances corresponding to ultra-low frequency (ULF; 0–0.0033 Hz), very low frequency (VLF; 0.0033–0.04 Hz), low frequency (LF; 0.04–0.15 Hz), and high frequency (HF; 0.15–0.40 Hz) bands, and LF/HF ratio, all of which were proposed by the Task Force of the European Society of Cardiology and the North American Society of Pacing and Electrophysiology (Camm et al., 1996). The variances of these frequency components were transformed to natural logarithmic values (ln ms2).
In addition, we also computed the deceleration and acceleration capacity (DC and AC) based on the phase rectified signal averaging of NN intervals (Bauer et al., 2006), and the fractal scaling exponents, the short-term exponent α1 and the long-term α2, using detrended fluctuation analysis (DFA; Peng et al., 1995).
Multiscale Probability Density Function Analysis
Recent studies from our group have shown that human HRV exhibits the intermittent dynamics or temporal heterogeneity of variance leading to non-Gaussian probability density function (PDF) of heart rate increments (Kiyono et al., 2004), especially in cardiac patients within timescales corresponding to LF and VLF ranges (Kiyono et al., 2008; Hayano et al., 2011). As such a feature, called heteroscedasticity, cannot be captured by conventional HRV indices, we conducted multiscale PDF analysis to characterize intermittent large deviations and the resultant non-Gaussianity of HRV.
The procedure starts from interpolating observed series of NN intervals with a cubic spline function and resampling at an interval (Δt) of 250 ms (4 Hz), yielding interpolated time series b(t). Next after subtracting average interval bave, integrated time series B(t) are obtained by integrating b(t) over the entire length,
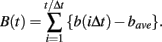
As in previous studies (Kiyono et al., 2004, 2008; Hayano et al., 2011), the local trend of B(t) is eliminated by a third-order polynomial fit to B(t) within moving windows of length 2s, where s is the scale of analysis. Thereafter, intermittent deviation ΔsB(t) is measured as the increment with a time lag s of the detrended time series. For instance, in a window from time T − s to T + s, the increments are calculated as follows:

where T − s/2 ≤ t < T + s/2 and ffit(t) is the polynomial representing the local trend of B(t). ΔsB(t) reflects an average degree of tachycardia if negative [b(t) < bave] or bradycardia if positive [b(t) > bave] over a moving window with length s (in seconds) after detrending. To quantitatively characterize the non-Gaussian property of ΔsB(t) at scale s, the standardized PDF (variance set to one) constructed from all ΔsB(t) values is approximated by the Castaing’s model (Castaing et al., 1990) with a single parameter λs, which we refer to as the non-Gaussianity index. A greater λs indicates a fatter non-Gaussian tail and a sharper peak of PDF compared to the Gaussian distribution. On the other hand, if λs is close to zero, PDF is close to a Gaussian distribution. The parameter λs is estimated as follows:

where q ≠ 0 or 2, q > -1, and denotes the estimated value of the q-th order absolute moment of ΔsB (Kiyono et al., 2007).
In the present study, we calculated λs using the 0.25-th order moment (q = 0.25) to emphasize the center part of PDF and reduce the effects of large outliers such as those resulting from ectopic beats. This implies that our non-Gaussianity index with q = 0.25 more strongly characterizes peak PDF around the center of the observed non-Gaussian distribution, as opposed to higher-order moments, such as kurtosis based on the fourth moment, emphasizing heavy tails and extreme deviations. Based on our recent findings that increased λs at scale s = 25 s is associated with increased cardiac mortality risk and that this predictive power is independent of clinical risk factors in CHF and AMI patients (Kiyono et al., 2008; Hayano et al., 2011), we evaluated the non-Gaussianity index λ25s at s = 25 s, which is at the edge of LF and VLF ranges.
An important feature of this multiscale PDF analysis is that if a time series has temporally homogeneous and finite variance, the increment PDF of the integrated series rapidly converges to a Gaussian distribution as the time-scale s increases because of the well-known statistical law called the central limit theorem. On the other hand, if neither condition is fulfilled, slow convergence to a Gaussian distribution or a scale-dependent λs and non-Gaussian fat tail can arise, suggestive of increased intermittency as observed in hydrodynamic turbulence (Castaing et al., 1990; Ghashghaie et al., 1996). Indeed, in the so-called multiplicative cascade model (Monin and Yaglom, 1975), one of the representative models describing intermittency of hydrodynamic turbulence and also used as a model of heart rate intermittency (Lin and Hughson, 2001), λs is known to have scale dependence in the form of (Kiyono et al., 2007; Figure 1). In the cascade model, multiscaling properties of the increments called structure functions also exist in the corresponding scales (Kiyono et al., 2007). To evaluate such a dynamic (cascade-like) aspect of intermittent fluctuations, we calculated the slope of
vs. ln s (λ2-slope) in the range 20 < s < 200 s (mainly covering LF and VLF ranges).
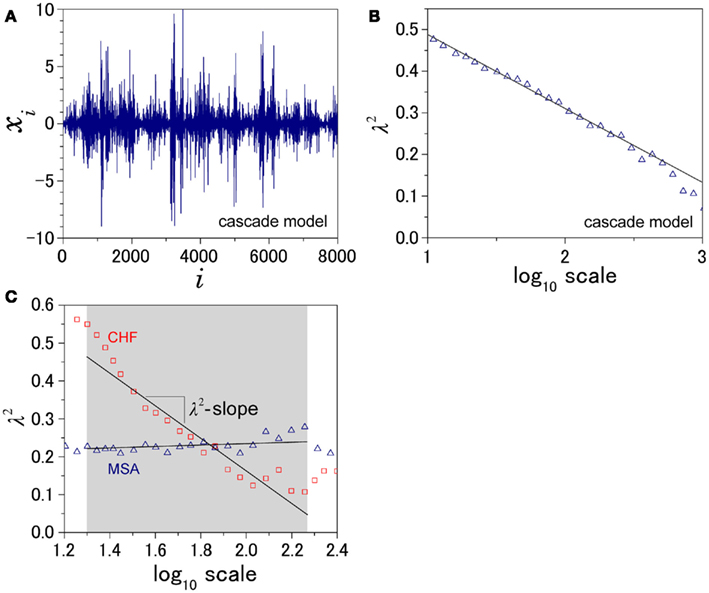
Figure 1. Illustration of the definition of λ2-slope. (A) An example of intermittent fluctuation generated by a cascade model (Kiyono et al., 2007) and (B) the scale dependence of λ2, which is proportional to the logarithmic scale. To quantify this kind of intermittent behavior of HRV, λ2-slope is defined as the slope of a regression line between λ2 and the logarithmic scale in the range between 20 and 200 s. (C) Scale dependence of λ2 for a patient with multiple system atrophy (MSA) and a patient with congestive heart failure (CHF).
Statistical Analysis
The data are reported as the mean ± SD. One-way ANOVA was used to test for statistical differences across groups, and Tukey’s honestly significant difference test was used for pair-wise comparisons. For variables with skewed distributions, values were transformed to natural logarithms. The Kolmogorov–Smirnov test was used to assess differences in age distribution between groups. In addition, the bootstrap method (Efron and Tibshirani, 1993) was used to assess possible selection biases of age-matched control groups. Bootstrap samples having the same size as each of MSA and PD groups were generated by randomly drawing age-matched subjects with replacement from a pool of healthy subjects. P < 0.05 was considered significant.
Results
Indices of autonomic function were derived from HRV recordings from MSA, PD, and CHF patients as well as from the three separate age-matched control groups (MSA controls, 63.6 ± 8.6 years vs. MSA patients, 62.3 ± 7.4 years; PD controls, 68.5 ± 8.3 years vs. PD patients, 68.6 ± 7.9 years; CHF controls, 59.1 ± 16.0 years vs. CHF patients, 66.1 ± 14.8 years). The age distributions for the control groups were not significantly different from those for the patients’ groups. Mean duration since MSA diagnosis was 3.3 ± 2.1 years (range, 1–9 years; Table 1). Mean duration since PD diagnosis was 10.7 ± 9.1 years (range, 2–31 years), and the mean Hoehn and Yahr score was 3.6 ± 0.7 (range, 3–5; Table 2).
Conventional HRV Indices
Table 3 presents HRV indices derived from HRV recordings from MSA patients and age-matched healthy control subjects, together with the bootstrap estimators for the healthy controls. Compared with the control group, the MSA patients showed significantly decreased HRV as indicated by lower SDNN, SDANN, and RMSSD values, reduced power in all spectral bands (HF, LF, VLF, ULF), and lower DC and AC. Indices such as LF/HF and DFA α1 were also significantly decreased. Compared with the control group, the PD patients showed significant decreases only in LF and VLF power and significantly lower DC and AC (Table 4). LF/HF and DFA α1 were significantly decreased. As shown in Tables 3 and 4, these findings were largely supported also by comparing mean values for the patient groups with 95%-confidence intervals of the bootstrap estimators. Table 5 presents the HRV indices in CHF patients and age-matched healthy control subjects. Compared with the control group, both surviving and non-surviving CHF patients exhibited significantly decreased HRV as indicated by lower SDNN and SDANN, reduced power in LF, VLF, and ULF ranges, and lower DC and AC. Indices such as LF/HF, and DFA α1 and α2 were also significantly decreased.
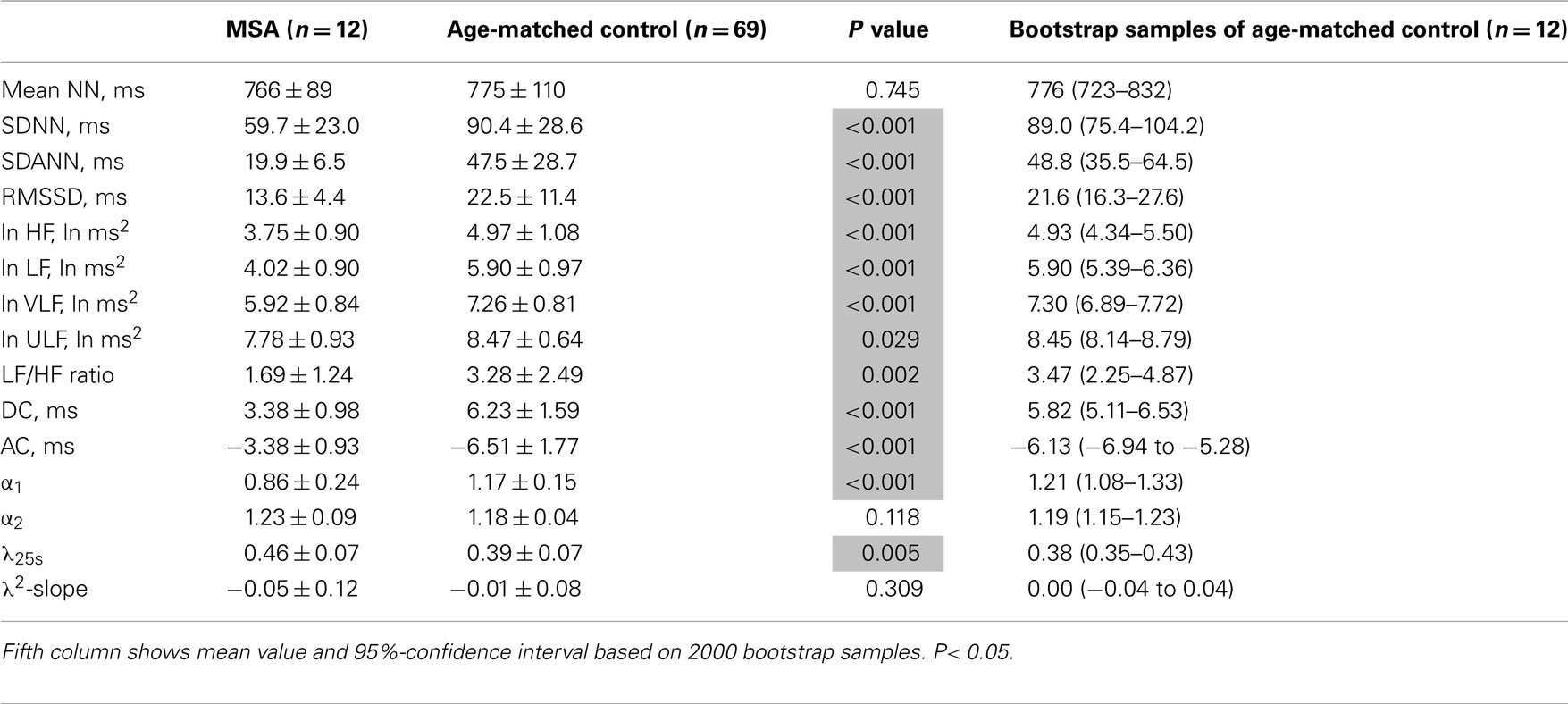
Table 3. Heart rate variability measures in patients with multiple system atrophy (MSA) and age-matched controls.
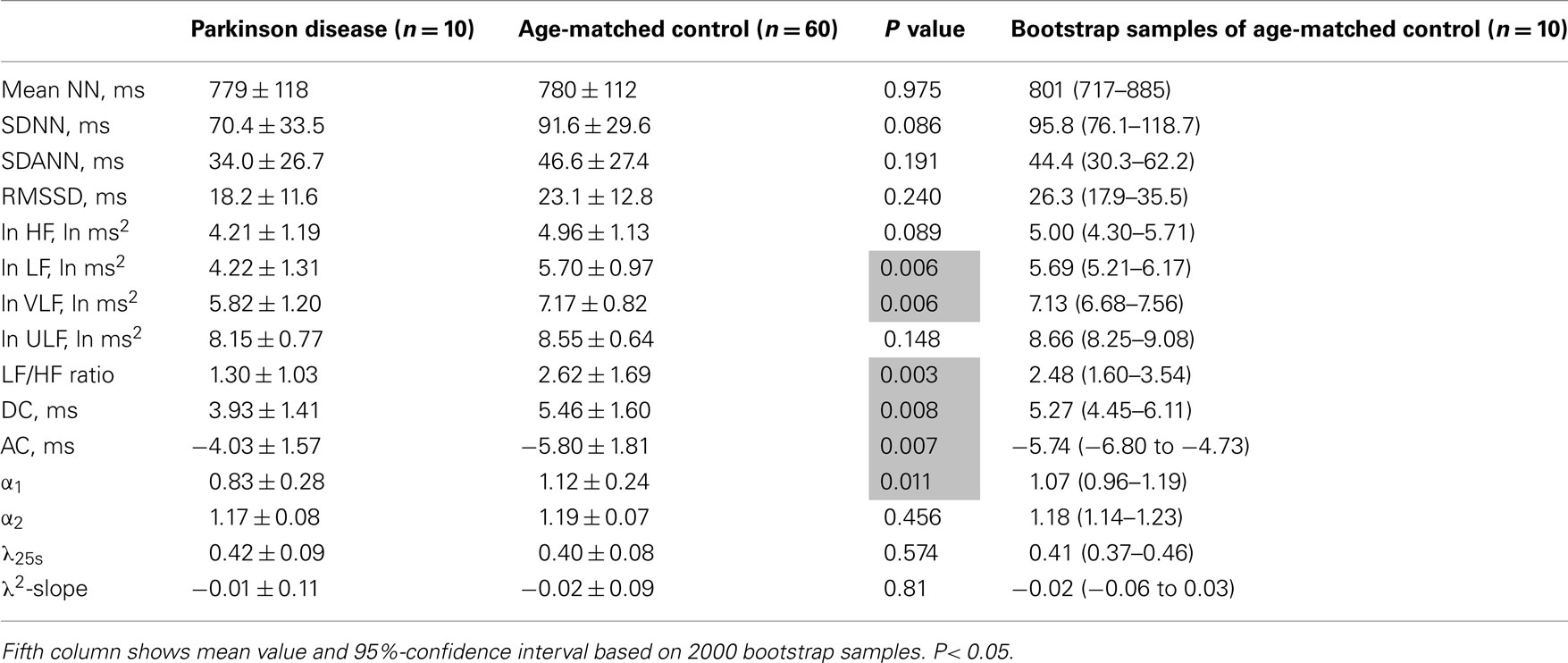
Table 4. Heart rate variability measures in patients with Parkinson disease and age-matched controls.
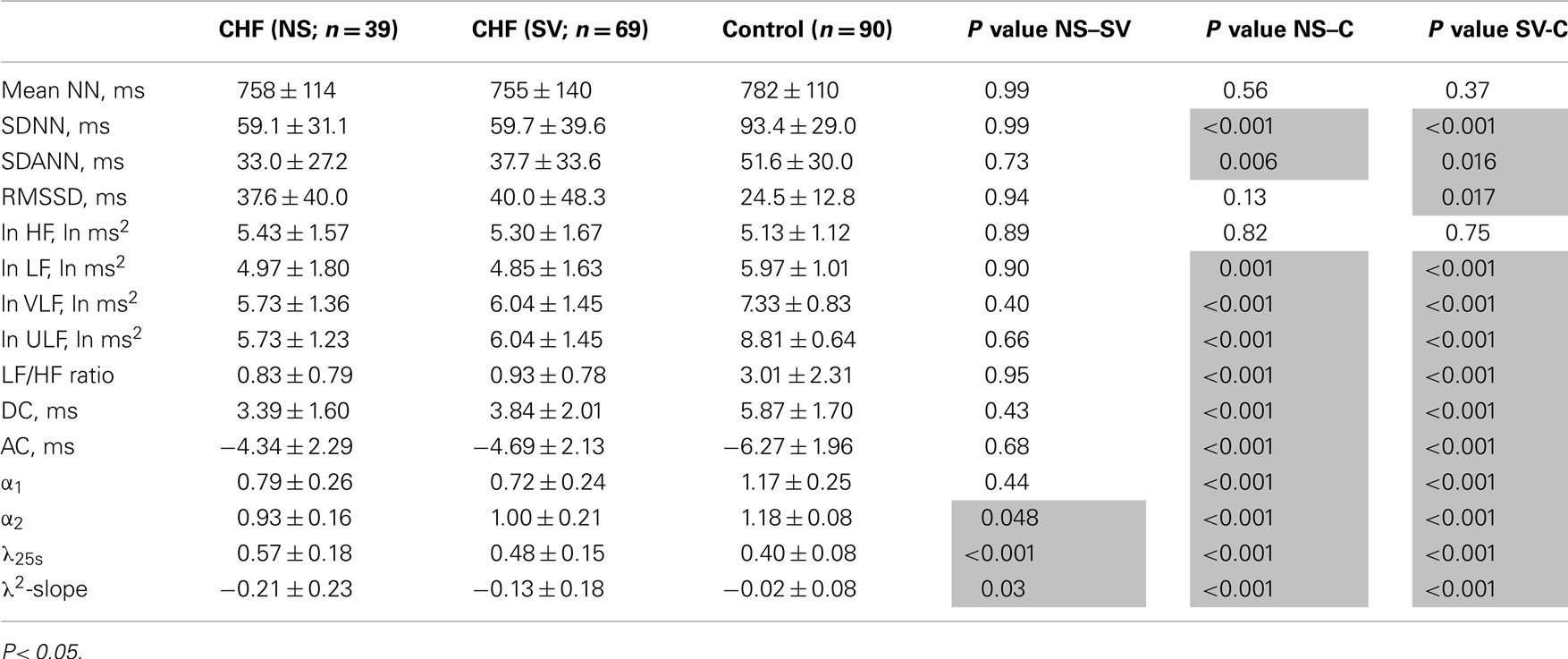
Table 5. Heart rate variability indices in patients with congestive heart failure and age-matched controls.
In the MSA patients, the pattern of changes in conventional HRV indices was similar to that observed in the CHF patients. While the decreased HRV in both MSA and CHF patients might reflect reduced vagal heart rate control, decreases in LF/HF and DFA α1 were observed for both MSA, a disease with reported preganglionic sympathetic failure (Sone et al., 2005), and for CHF, a pathology associated with sympathetic overdrive (Packer, 1988; Ciarka et al., 2008). In contrast, no decreases in SDNN and HF power, indices of reduced HRV, were observed in the PD patients, which might reflect relatively intact vagal heart rate control. However, decreases in LF/HF and DFA α1 were also observed in PD, a disease with reported postganglionic sympathetic failure (Braune et al., 1998, 1999).
Non-Gaussian and Intermittent Properties of HRV
Figure 2 shows representative results of the multiscale PDF analysis for MSA, CHF, and PD patients (one patient from each group) and a healthy subject. As shown in Figures 2M–P, HRV data from the MSA and PD patients and the healthy subject yielded similar PDF curves at each scale. In contrast, recordings from the CHF patient yielded a PDF curve with a more tapered center and fatter tails at relatively smaller scales. This reflects intermittent large deviations or bursts observed at s = 20 s in CHF patients (Figure 2G), while this increased intermittency was not observed in the MSA and PD patients. In addition, as the scale s increases, deformation of PDFs toward a Gaussian distribution was clearly observed only in the CHF patient. The deformation process of the non-Gaussian PDF can be described by the relation between the non-Gaussianity index λs and scale s. As shown in Figure 3, the MSA and PD patient groups and the healthy subject groups showed nearly constant values across a wide range of scales s, resulting in an almost zero value λ2-slope. In contrast, the CHF patient group, particularly non-survivors, was characterized by almost linear increases in
as the log scale decreased from 200 to 20 s, similar to that observed for a cascade model of intermittent turbulence (Figure 1B). Consequently, the λ2-slope for the CHF patients was significantly more negative than that for the healthy controls.
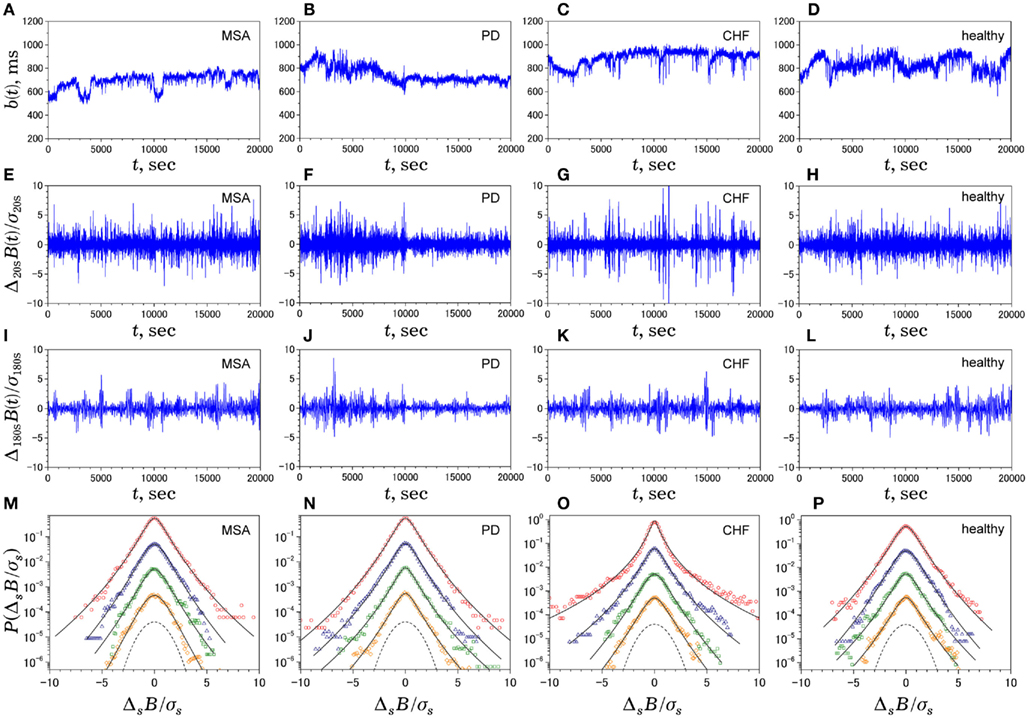
Figure 2. Multiscale PDF characterization of heart rate variability. Illustrative examples of time series of NN intervals b(t) (A–D), time series of Δ20sB(i) (E–H), time series of Δ180sB(i) (I–L), and standardized PDFs (in logarithmic scale) of ΔsB(i) for (from the top to bottom) s = 20, 60, 180, 300 s (M–P), where σs denotes the SD of ΔsB(i). In solid lines, we superimpose the PDF approximated by Castaing’s model (Castaing et al., 1990). The panels on the leftmost side (A,E,I,M) are data for a 60-year-old male patient with multiple system atrophy (MSA). The panels on the left-of-center side (B,F,J,N) are data for a 68-year-old male patient with Parkinson disease (PD). The panels on the right-of-center side (C,G,K,O) are data for a 83-year-old female patient with congestive heart failure (CHF) who died 54 days after the measurement. The panels on the rightmost side (D,H,L,P) are data for a control subject (84-year-old male). For comparison, the dashed line denotes a Gaussian distribution. Note that, while the raw b(t) for the patients looks much different from that for the control subject, the degrees of non-Gaussianity (i.e., the shapes of PDF) remain unaltered across scales for MSA and PD patients and the healthy control, except for those for the CHF patient at shorter scales (G,O).
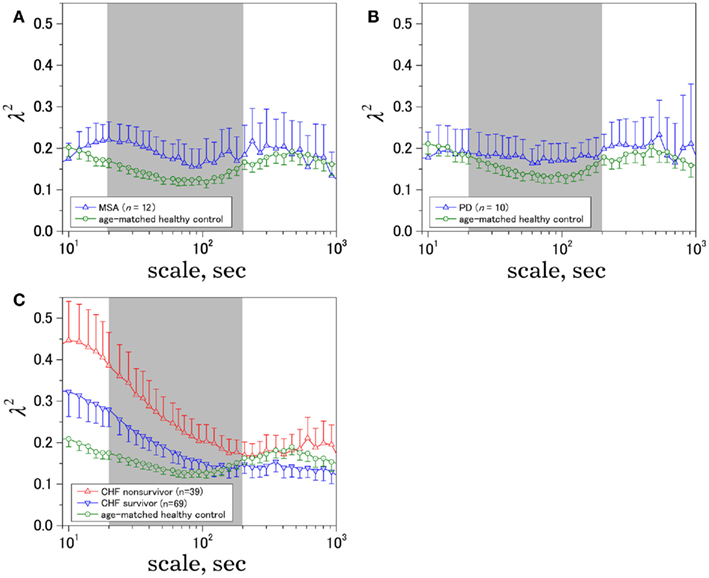
Figure 3. Time-scale dependence of the non-Gaussianity index, λ2. The results for (A) multiple system atrophy (MSA), (B) Parkinson disease (PD), and (C) congestive heart failure (CHF) patients, both for survivor (SV; n = 69) and non-survivor (NS; n = 39). Age-matched controls were selected from a database of healthy subjects. Error bars indicate 95%-confidence intervals of the group averages.
λ25s for the MSA patients was slightly but significantly higher than that for healthy controls (Table 3), although the level was much lower than that for CHF non-survivors (Table 5). λ25s for the PD patients failed to increase compared with that for healthy controls (Table 4). Both MSA and PD patients with sympathetic failure had λ2-slopes of almost zero, which were not significantly different from those of healthy controls (Tables 3 and 4). Only CHF patients with known sympathetic overdrive had significantly negative λ2-slopes (Table 5).
Discussion
Long-term ambulatory HRV continues to attract clinical interest as a useful tool for risk stratification in AMI (Kleiger et al., 1987; Bigger et al., 1996; La Rovere et al., 1998; Schmidt et al., 1999; Huikuri et al., 2000; Bauer et al., 2006) and chronic heart failure (Ho et al., 1997; Nolan et al., 1998; Mäkikallio et al., 2001). Patients at higher mortality risk frequently have higher heart rates with reduced and less complex (or monotonic) HRV, and most indices used to characterize such HRV dynamics primarily reflect reduced or impaired vagal function (Camm et al., 1996; Marine et al., 2002; Bauer et al., 2006). In contrast, few HRV indices are related to sympathetic function and their autonomic correlates and prognostic significance are still uncertain. For example, a decrease, but not the increase, in LF/HF, believed to reflect the sympathovagal balance (Pagani et al., 1986), is associated with increased mortality risk (Tsuji et al., 1994; Huikuri et al., 2000) in patients exhibiting sympathetic activation (Ciarka et al., 2008). Similarly, a decrease in DFA α1, known to be correlated with LF/HF and sensitive to changes in the sympathovagal balance (Huikuri et al., 2009), is associated with increased mortality risk (Huikuri et al., 2000; Mäkikallio et al., 2001). The present study further demonstrated that both of these indices are also decreased in MSA, a neurodegenerative disorder associated with preganglionic sympathetic failure (Sone et al., 2005), and in PD, which is often accompanied by postganglionic sympathetic failure (Braune et al., 1998, 1999).
As a marker potentially related to sympathetic cardiac overdrive, we have recently introduced increased non-Gaussianity of HRV within LF and VLF ranges in patients with CHF and AMI (Kiyono et al., 2008; Hayano et al., 2011), cardiopathologies known to be associated with sympathetic overdrive (Packer, 1988; Ciarka et al., 2008). In the present study, we further demonstrated that a marked increase in intermittent and non-Gaussian HRV was not observed in MSA and PD patients with sympathetic failure. We still have not determined why λ25s for the MSA patients was slightly but significantly higher than that for healthy controls; this enhanced non-Gaussianity may be due to adrenergic stimulants administered to ameliorate severe orthostatic symptoms in the MSA patients. However, the scale-dependent increase in with decreasing log scales mainly within the VLF range, leading to a markedly higher λ25s in the CHF patients (Table 5), was not observed in MSA. Therefore, we suggest that the systematically increased non-Gaussianity of HRV within LF and VLF ranges could be a hallmark of sympathetic cardiac overdrive and that indices such as λ25s and λ2-slope could be used to measure the degree of sympathetic activation. Indeed, we recently observed decreased λ25s in the AMI patients taking (anti-sympathetic) β-blockers (Hayano et al., 2011).
Using concepts developed in statistical and non-linear physics, it has been demonstrated that the healthy human heart rate fluctuates in a complex manner even under resting conditions, exhibiting fractal long-range correlations (Peng et al., 1993; Yamamoto and Hughson, 1994) and multifractal properties (Ivanov et al., 1999; Amaral et al., 2001; Ching and Tsang, 2007). Based on these findings, Lin and Hughson (2001) proposed an analogy between heart rate dynamics and hydrodynamic turbulence because a phenomenological model of hydrodynamic turbulence, called the multiplicative cascade model (Monin and Yaglom, 1975), can also have multifractal properties. Using multiscale PDF analysis, we later demonstrated that the healthy human HRV does not show slow and gradual convergence to a Gaussian distribution (Kiyono et al., 2004; Figure 3), an important requirement of the multiplicative cascade model (Figure 1B). In contrast, the present study and previous work (Kiyono et al., 2008) suggest that HRV within LF and VLF ranges of CHF patients, especially non-survivors, is more compatible with the multiplicative cascade model.
The multiplicative (log-normal) cascade model used to generate fluctuations with intermittent bursts such as those shown in Figure 1A is given by
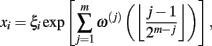
where ξi is Gaussian white noise with zero mean, ω(j)(k) are independent Gaussian random variables with zero mean and constant variance, and (·( is the floor function (Kiyono et al., 2007). The m is the total number of cascade steps, yielding the total number of data points 2m (i = 1, …, 2m). An essential part of the model is that ξi is modulated by multiplication of random (log-normal) weights exp[ω(j)(k)] (k = 0, 1, …, 2j− 1) at the j-th step every 2m−j subintervals; therefore, large fluctuations are observed only when the momentary weights for (many) different steps with varying timescales are simultaneously large (refer to Figure 5 of Kiyono et al., 2007). Using multiscale PDF analysis, Kiyono et al. (2007) further showed that this model exhibits the scale dependence of a non-Gaussianity index in the form of (Figure 1B).
The fact that heart rate dynamics of CHF patients with sympathetic activation exhibit a non-Gaussianity index which decays with scales within LF and VLF ranges suggests a sympathetic origin for HRV intermittency. In these scales (20–200 s), heart rate dynamics reflect cardiovascular regulation by neural, humoral, and thermal influences (Kitney and Rompelman, 1980). These subsystems are considered to be compensatory; therefore, it is likely that only simultaneous failure of all these subsystems operating at multiple timescales, compatible with the reciprocal of cascade steps “j” in the above example, could result in sympathetic overdrive, leading to large and intermittent heart rate deviations. We propose that such a multiplicative picture would provide a deeper physiological understanding of the nature of sympathetic function. In addition, it would provide a reason why methods requiring stationary, not intermittent, dynamics have not been successful in finding the sympathetic correlates of ambulatory HRV.
In the present study, we focused on daytime HRV for the following reasons. First, as reported in our previous study (Kiyono et al., 2005), there are large differences in non-Gaussianity and its scale dependence between day and night in healthy humans, presumably because of the difference in the sympathovagal balance. Second, disorders of sleep and sleep breathing are common in MSA (Colosimo, 2011); therefore, incorporating nighttime data would inevitably introduce additional complexity. Third, one of our goals is to assess sympathetic activity, which is predominant during the day. Note that this shift from 24-h HRV to daytime HRV does not change our previous finding of the increased non-Gaussianity of low frequency HRV in CHF patients than in healthy controls (Kiyono et al., 2008).
In agreement with previous studies (Gurevich et al., 2004; Kuriyama et al., 2005), our MSA patients showed significantly decreased HRV, as evidenced by lower SDNN and HF power. This decrease is probably related to the known abnormalities in central vagal (Benarroch et al., 2006) and sympathetic function in these patients (Sone et al., 2005). On the other hand, changes in SDNN and HF power were not significant in PD patients, implying relatively intact vagal heart rate control despite the impaired peripheral, cardiac sympathetic function (Braune et al., 1998, 1999). Thus, analyses of ambulatory HRV may facilitate discriminative diagnosis between MSA and PD, particularly the difficult distinction between early stage PD and MSA with predominant Parkinsonian symptoms (Lipp et al., 2009).
Conflict of Interest Statement
The authors declare that the research was conducted in the absence of any commercial or financial relationships that could be construed as a potential conflict of interest.
Abbreviations
AC, acceleration capacity; AMI, acute myocardial infarction; CHF, congestive heart failure; DC, deceleration capacity; DFA, detrended fluctuation analysis; ECG, electrocardiogram; HF, high frequency; HRV, heart rate variability; LF, low frequency; LF/HF, LF-to-HF ratio; MRI, magnetic resonance imaging; MSA, multiple system atrophy; NN, normal-to-normal; PD, Parkinson disease; PDF, probability density function; RMSSD, root mean square of successive difference of NN intervals; SD, standard deviation; SDANN, standard deviation of 5 min averaged NN intervals; SDNN, standard deviation of all NN intervals; ULF, ultra-low frequency; VLF, very low frequency.
Acknowledgments
This research was supported in part by a Grant-in-Aid for Scientific Research (A) (23240094; to Yoshiharu Yamamoto) from the Japan Society for the Promotion of Science (JSPS) and by Core Research for Evolutional Science and Technology from Japan Science and Technology Agency (JST; to Yoshiharu Yamamoto).
References
Amaral, L. A. N., Ivanov, P., Aoyagi, N., Hidaka, I., Tomono, S., Goldberger, A. L., Stanley, H. E., and Yamamoto, Y. (2001). Behavioral-independent features of complex heartbeat dynamics. Phys. Rev. Lett. 86, 6026–6029.
Bauer, A., Kantelhardt, J. W., Barthel, P., Schneider, R., Mäkikallio, T., Ulm, K., Hnatkova, K., Schömig, A., Huikuri, H. V., Bunde, A., Malik, M., and Schmidt, G. (2006). Deceleration capacity of heart rate as a predictor of mortality after myocardial infarction: cohort study. Lancet 367, 1674–1681.
Benarroch, E. E., Schmeichel, A. M., Sandroni, P., Low, P. A., and Parisi, J. E. (2006). Involvement of vagal autonomic nuclei in multiple system atrophy and Lewy body disease. Neurology 66, 378–383.
Bigger, J. T., Steinman, R. C., Rolnitzky, L. M., Fleiss, J. L., Albrecht, P., and Cohen, R. J. (1996). Power law behavior of RR-interval variability in healthy middle-aged persons, patients with recent acute myocardial infarction, and patients with heart transplants. Circulation 93, 2142–2151.
Braune, S., Reinhardt, M., Bathmann, J., Krause, T., Lehmann, M., and Lücking, C. H. (1998). Impaired cardiac uptake of meta-[123I]iodobenzylguanidine in Parkinson’s disease with autonomic failure. Acta Neurol. Scand. 97, 307–314.
Braune, S., Reinhardt, M., Schnitzer, R., Riedel, A., and Lücking, C. H. (1999). Cardiac uptake of [123I]MIBG separates Parkinson’s disease from multiple system atrophy. Neurology 53, 1020–1025.
Camm, A. J., Malik, M., Bigger, J. T. Jr., Breithardt, G., Cerutti, S., Cohen, R. J., Coumel, P., Fallen, E. L., Kleiger, R. E., Lombardi, F., Malliani, A., Moss, A. J., Rottman, J. N., Schmidt, G., Schwartz, P. J., and Singer, D. H. (1996). Heart rate variability: standards of measurement, physiological interpretation and clinical use. Task Force of the European Society of Cardiology and the North American Society of Pacing and Electrophysiology. Circulation 93, 1043–1065.
Castaing, B., Gagne, Y., and Hopfinger, E. (1990). Velocity probability density functions of high Reynolds number turbulence. Physica D 46, 177–200.
Ching, E. S. C., and Tsang, Y.-K. (2007). Multifractality and scale invariance in human heartbeat dynamics. Phys. Rev. E Stat. Nonlin. Soft Matter Phys. 76, 041910.
Ciarka, A., van de Borne, P., and Pathak, A. (2008). Myocardial infarction, heart failure and sympathetic nervous system activity: new pharmacological approaches that affect neurohumoral activation. Expert Opin. Investig. Drugs 17, 1315–1330.
Colosimo, C. (2011). Nonmotor presentations of multiple system atrophy. Nat. Rev. Neurol. 7, 295–298.
Efron, B., and Tibshirani, R. J. (1993). An Introduction to the Bootstrap. New York: Chapman & Hall.
Ghashghaie, S., Breymann, W., Peinke, J., Talkner, P., and Dodge, Y. (1996). Turbulent cascades in foreign exchange markets. Nature 381, 767–770.
Gilman, S., Wenning, G. K., Low, P. A., Brooks, D. J., Mathias, C. J., Trojanowski, J. Q., Wood, N. W., Colosimo, C., Dürr, A., Fowler, C. J., Kaufmann, H., Klockgether, T., Lees, A., Poewe, W., Quinn, N., Revesz, T., Robertson, D., Sandroni, P., Seppi, K., and Vidailhet, M. (2008). Second consensus statement on the diagnosis of multiple system atrophy. Neurology 71, 670–676.
Gurevich, T. Y., Groozman, G. B., Giladi, N., Drory, V. E., Hausdorff, J. M., and Korczyn, A. D. (2004). R-R interval variation in Parkinson’s disease and multiple system atrophy. Acta Neurol. Scand. 109, 276–279.
Hayano, J., Kiyono, K., Struzik, Z. R., Yamamoto, Y., Watanabe, E., Stein, P. K., Watkins, L. L., Blumenthal, J. A., and Carney, R. M. (2011). Increased non-Gaussianity of heart rate variability predicts cardiac mortality after an acute myocardial infarction. Front. Physiol. 2:65. doi:10.3389/fphys.2011.00065
Ho, K. K. L., Moody, G. B., Peng, C.-K., Mietus, J. E., Larson, M. G., Levy, D., and Goldberger, A. L. (1997). Predicting survival in heart failure case and control subjects by use of fully automated methods for deriving nonlinear and conventional indices of heart rate dynamics. Circulation 96, 842–848.
Hughes, A. J., Daniel, S. E., Kilford, L., and Lees, A. J. (1992). Accuracy of clinical diagnosis of idiopathic Parkinson’s disease: a clinico-pathological study of 100 cases. J. Neurol. Neurosurg. Psychiatr. 55, 181–184.
Huikuri, H. V., Mäkikallio, T. H., Peng, C.-K., Goldberger, A. L., Hintze, U., and Møller, M. (2000). Fractal correlation properties of R-R interval dynamics and mortality in patients with depressed left ventricular function after an acute myocardial infarction. Circulation 101, 47–53.
Huikuri, H. V., Perkiömäki, J. S., Maestri, R., and Pinna, G. D. (2009). Clinical impact of evaluation of cardiovascular control by novel methods of heart rate dynamics. Philos. Transact. A Math. Phys. Eng. Sci. 367, 1223–1238.
Ivanov, P. C., Amaral, L. A. N., Goldberger, A. L., Havlin, S., Rosenblum, M. G., Struzik, Z. R., and Stanley, H. E. (1999). Multifractality in human heart rate dynamics. Nature 399, 461–465.
Kitney, R. I., and Rompelman, O. (eds). (1980). The Study of Heart-Rate Variability. Oxford: Clarendon Press.
Kiyono, K., Hayano, J., Watanabe, E., Struzik, Z. R., and Yamamoto, Y. (2008). Non-Gaussian heart rate as an independent predictor of mortality in patients with chronic heart failure. Heart Rhythm 5, 261–268.
Kiyono, K., Struzik, Z. R., Aoyagi, N., Sakata, S., Hayano, J., and Yamamoto, Y. (2004). Critical scale invariance in a healthy human heart rate. Phys. Rev. Lett. 93, 178103.
Kiyono, K., Struzik, Z. R., Aoyagi, N., Togo, F., and Yamamoto, Y. (2005). Phase transition in a healthy human heart rate. Phys. Rev. Lett. 95, 058101.
Kiyono, K., Struzik, Z. R., and Yamamoto, Y. (2007). Estimator of a non-Gaussian parameter in multiplicative log-normal models. Phys. Rev. E Stat. Nonlin. Soft Matter Phys. 76, 041113.
Kleiger, R. E., Miller, J. P., Bigger, J. T., Moss, A. J., The Multicenter Post-Infarction Research Group. (1987). Decreased heart rate variability and its association with increased mortality after acute myocardial infarction. Am. J. Cardiol. 59, 256–262.
Kuriyama, N., Mizuno, T., Iida, A., Watanabe, Y., and Nakagawa, M. (2005). Autonomic nervous evaluation in the early stages of olivopontocerebellar atrophy. Auton. Neurosci. 123, 87–93.
La Rovere, M. T., Bigger, J. T., Marcus, F. I., Mortara, A., and Schwartz, P. J. (1998). Baroreflex sensitivity and heart-rate variability in prediction of total cardiac mortality after myocardial infarction. Lancet 351, 478–484.
Lin, D., and Hughson, R. L. (2001). Modeling heart rate variability in healthy humans: a turbulence analogy. Phys. Rev. Lett. 86, 1650–1653.
Lipp, A., Sandroni, P., Ahlskog, J. E., Fealey, R. D., Kimpinski, K., Iodice, V., Gehrking, T. L., Weigand, S. D., Sletten, D. M., Gehrking, J. A., Nickander, K. K., Singer, W., Maraganore, D. M., Gilman, S., Wenning, G. K., Shults, C. W., and Low, P. A. (2009). Prospective differentiation of multiple system atrophy from Parkinson disease, with and without autonomic failure. Arch. Neurol. 66, 742–750.
Mäkikallio, T. H., Huikuri, H. V., Hintze, U., Videbaek, J., Mitrani, R. D., Castellanos, A., Myeburg, R. J., Møller, M., and Myerburg, R. J. (2001). Fractal analysis and time- and frequency-domain measures of heart rate variability as predictors of mortality in patients with heart failure. Am. J. Cardiol. 87, 178–182.
Marine, J. E., Watanabe, M. A., Smith, T. W., and Monahan, K. M. (2002). Effect of atropine on heart rate turbulence. Am. J. Cardiol. 89, 767–769.
McEwen, B. S. (1998). Protective and damaging effects of stress mediators. N. Engl. J. Med. 338, 171–179.
McEwen, B. S. (2007). Physiology and neurobiology of stress and adaptation: central role of the brain. Physiol. Rev. 87, 873–904.
Nolan, J., Batin, P. D., Andrews, R., Lindsay, S. J., Brooksby, P., Mullen, M., Baig, W., Flapan, A. D., Cowley, A., Prescott, R. J., Neilson, J. M., and Fox, K. A. (1998). Prospective study of heart rate variability and mortality in chronic heart failure. Results of the United Kingdom heart failure evaluation and assessment of risk trial (UK-Heart). Circulation 98, 1510–1516.
Packer, M. (1988). Neurohormonal interactions and adaptations in congestive heart failure. Circulation 77, 721.
Pagani, M., Lombardi, F., Guzzetti, S., Rimoldi, O., Furlan, R., Pizzinelli, P., Sandrone, G., Malfatto, G., Dell’Orto, S., Piccaluga, E., Turiel, M., Baselli, G., Cerutti, S., and Malliani, A. (1986). Power spectral analysis of heart rate and arterial pressure variabilities as a marker of sympatho-vagal interaction in man and conscious dog. Circ. Res. 59, 178–193.
Peng, C. K., Havlin, S., Stanley, H. E., and Goldberger, A. L. (1995). Quantification of scaling exponents and crossover phenomena in nonstationary heartbeat time series. Chaos 5, 82–87.
Peng, C.-K., Mietus, J., Hausdorff, J. M., Havlin, S., Stanley, H. E., and Goldberger, A. L. (1993). Long-range anticorrelations and non-Gaussian behavior of the heartbeat. Phys. Rev. Lett. 70, 1343–1346.
Schmidt, G., Malik, M., Barthel, P., Schneider, R., Ulm, K., Rolnitzky, L., and Camm, A. J. (1999). Heart-rate turbulence after ventricular premature beats as a predictor of mortality after acute myocardial infarction. Lancet 353, 1390–1396.
Schwartz, P. J., Billman, G. E., and Stone, H. L. (1984). Autonomic mechanisms in ventricular fibrillation induced by myocardial ischemia during exercise in dogs with healed myocardial infarction. An experimental preparation for sudden cardiac death. Circulation 69, 790–800.
Sone, M., Yoshida, M., Hashizume, Y., Hishikawa, N., and Sobue, G. (2005). alpha-Synuclein-immunoreactive structure formation is enhanced in sympathetic ganglia of patients with multiple system atrophy. Acta Neuropathol. 110, 19–26.
Stefanova, N., Bücke, P., Duerr, S., and Wenning, G. K. (2009). Multiple system atrophy: an update. Lancet Neurol. 8, 1172–1178.
Tsuji, H., Venditti, F. J., Manders, E. S., Evans, J. C., Larson, M. G., Feldman, C. L., and Levy, D. (1994). Reduced heart rate variability and mortality risk in an elderly cohort. The Framingham Heart Study. Circulation 90, 878–883.
Wenning, G. K., Stefanova, N., Jellinger, K. A., Poewe, W., and Schlossmacher, M. G. (2008). Multiple system atrophy: a primary oligodendrogliopathy. Ann. Neurol. 64, 239–246.
Keywords: heart rate variability, ambulatory ECG, multiple system atrophy, Parkinson disease, autonomic failure
Citation: Kiyono K, Hayano J, Kwak S, Watanabe E and Yamamoto Y (2012) Non-Gaussianity of low frequency heart rate variability and sympathetic activation: lack of increases in multiple system atrophy and Parkinson disease. Front. Physio. 3:34. doi: 10.3389/fphys.2012.00034
Received: 09 December 2011; Accepted: 07 February 2012;
Published online: 22 February 2012.
Edited by:
Riccardo Barbieri, Massachusetts General Hospital, USAReviewed by:
Der Chyan Bill Lin, Ryerson University, CanadaRoberto Sassi, Università degli Studi di Milano, Italy
Copyright: © 2012 Kiyono, Hayano, Kwak, Watanabe and Yamamoto. This is an open-access article distributed under the terms of the Creative Commons Attribution Non Commercial License, which permits non-commercial use, distribution, and reproduction in other forums, provided the original authors and source are credited.
*Correspondence: Yoshiharu Yamamoto, Graduate School of Education, The University of Tokyo, 7-3-1 Hongo, Bunkyo-ku, Tokyo 113-0033, Japan. e-mail: yamamoto@p.u-tokyo.ac.jp