- Institute of Clinical Medicine, Division of Cardiology, Department of Internal Medicine, Centre of Excellence in Research, University of Oulu, Oulu, Finland
The time-domain measures and power–spectral analysis of heart rate variability (HRV) are classic conventional methods to assess the complex regulatory system between autonomic nervous system and heart rate and are most widely used. There are abundant scientific data about the prognostic significance of the conventional measurements of HRV in patients with various conditions, particularly with myocardial infarction. Some studies have suggested that some newer measures describing non-linear dynamics of heart rate, such as fractal measures, may reveal prognostic information beyond that obtained by the conventional measures of HRV. An ideal risk indicator could specifically predict sudden arrhythmic death as the implantable cardioverter-defibrillator (ICD) therapy can prevent such events. There are numerically more sudden deaths among post-infarction patients with better preserved left ventricular function than in those with severe left ventricular dysfunction. Recent data support the concept that HRV measurements, when analyzed several weeks after acute myocardial infarction, predict life-threatening ventricular tachyarrhythmias in patients with moderately depressed left ventricular function. However, well-designed prospective randomized studies are needed to evaluate whether the ICD therapy based on the assessment of HRV alone or with other risk indicators improves the patients’ prognosis. Several issues, such as the optimal target population, optimal timing of HRV measurements, optimal methods of HRV analysis, and optimal cutpoints for different HRV parameters, need clarification before the HRV analysis can be a widespread clinical tool in risk stratification.
Introduction
Heart rate variability (HRV) describes the complex regulatory system between heart rate and the autonomic nervous system. There are several methods to measure HRV (Task Force of the European Society of Cardiology and the North American Society of Pacing and Electrophysiology, 1996). The traditional statistical methods and the power–spectral analysis of HRV are classic methods to measure HRV and are most widely used. The conventional measures of HRV have been shown to provide prognostic information in several patient populations (Kleiger et al., 1987; Bigger et al., 1992, 1993; Fei et al., 1996; Zuanetti et al., 1996; Nolan et al., 1998). The conventional measures of HRV cannot reveal delicate changes in heart rate beat-to-beat dynamics. Therefore several non-linear methods for measuring heart rate dynamics have been developed (Saul et al., 1987; Goldberger, 1990b, 1996; Skinner et al., 1993; Pincus and Goldberger, 1994; Peng et al., 1995; Voss et al., 1998; Tuzcu et al., 2006; Norris et al., 2008a). Few of these newer methods of HRV, such as the fractal-like scaling property and the complexity, have been tested in well-designed studies, which have included a relevant number of patients and have had well-defined endpoints. Some of these studies have suggested that some of the non-linear measures of HRV work better than the traditional measures of HRV in predicting future adverse events in various patient groups. The physiological background of the non-linear measures of HRV is much less well understood than that of the conventional measures of HRV. The non-linear methods of HRV assess qualitative properties rather than the magnitude of the heart rate dynamics.
Several other factors than the type of the parameter influence the prognostic value of HRV measurements. The timing of the HRV measurement after an acute myocardial infarction (AMI) has a direct influence on the prognostic significance of HRV due to substantial electrical and mechanical remodeling after AMI (Exner et al., 2007; Huikuri et al., 2009a). The prognostic value of HRV variables is also dependent on the left ventricular function and the severity of heart failure (Mäkikallio et al., 2001a, 2005). HRV parameters analyzed from short-term recordings obtained during controlled conditions yield somewhat different prognostic information than the HRV variables analyzed from long-term ambulatory 24-h recordings. It is also important to select appropriate preprocessing methods for editing premature depolarizations and irrelevant oscillations from RR interval time series in order to obtain reliable and reproducible prognostic data for clinical purposes (this is dealt in another review in the present issue). It is noteworthy that HRV parameters work prognostically differently in patients with different diseases and healthy subjects. The predictive power of HRV variables varies also depending on whether total mortality, different modes of mortality, or other adverse events are selected as endpoints. Several novel methods to describe heart rate dynamics, such as heart rate turbulence, have been developed (Schmidt et al., 1999). Their role in risk stratification is discussed in other reviews in this issue as is the influence of exercise on heart rate dynamics.
During the past two decades the number of publications dealing with HRV has exploded reaching at least over 14,000 at the moment. The present review is focusing on some of these studies, of which the majority have relevant number of patients and well-defined endpoints, and which elucidate the value of conventional and non-linear methods of HRV in risk assessment.
Heart Rate Variability in Risk Evaluation
Classic Studies Applying Conventional Methods of Heart Rate Variability Analysis
The prognostic significance of the conventional measures of HRV in post-AMI patients is well established. Schneider and Costiloe (1965) first proposed that HRV is reduced in patients with AMI and is associated with adverse prognosis. Wolf et al. (1978) found a significantly lower in-hospital mortality rate among the patients with AMI, who had more pronounced sinus arrhythmia. Kleiger et al. (1987) published the cornerstone study, where they showed that decreased HRV measured by the SD of all normal-to-normal RR-intervals (SDNN) analyzed from 24-h electrocardiographic recordings predicts mortality in post-infarction patients. The combination of reduced HRV and the occurrence of late potentials was found to have a sensitivity of 58%, a positive predictive accuracy of 33%, and a relative risk of 18.5 for arrhythmic events in post-AMI patients (Farrell et al., 1991). In the study by Cripps et al. (1991), which included 177 patients after AMI, the relative risk of sudden death or symptomatic sustained ventricular tachycardia during a median follow-up of 16 months was found to be seven times greater in those with low baseline values of the triangular index of HRV. In another study, after adjustment for relevant clinical risk markers, the total, ultralow-frequency and very-low-frequency powers of HRV remained a significant and powerful predictor of mortality after AMI (Bigger et al., 1992). In 433 survivors of first AMI, Odemuyiwa et al. (1994) observed that HRV was an independent predictor of sudden death and total cardiac mortality only during the first 6 months of follow-up. The results of the study including 226 consecutive patients with AMI confirmed the previous observations concerning the association between decreased HRV and mortality after AMI and suggested the importance of disturbance in sympathovagal regulation unrelated to left ventricular function or infarct location as a mechanism of high-risk (Vaishnav et al., 1994). Reduced HRV has been found to be related to both arrhythmic and non-arrhythmic death in post-AMI patients (Hartikainen et al., 1996). Fei et al. (1996) showed in their study including 700 consecutive patients after AMI that although the predictive accuracy of SDNN analyzed from 5-min short-term RR interval data for 1-year total cardiac mortality was lower than that of the HRV index analyzed from 24-h period, the SDNN short-term data could be used in preselection of high-risk patients. Bigger et al. (1993) studied the predictive value of the power–spectral measures of HRV obtained from short from 2 to 15 min electrocardiographic recordings in 715 post-AMI patients. They concluded that the power–spectral measures of HRV obtained from short recordings are remarkably similar to those obtained from 24-h recordings and predict all-cause mortality and sudden cardiac death. Zuanetti et al. (1996) evaluated the predictive value of several time-domain measures of HRV for total and cardiovascular mortality in 567 patients with AMI, who were treated with thrombolytic therapy and followed up for 1000 days. They concluded that time-domain indexes of HRV retain their independent prognostic significance even in post-AMI patients of all ages treated with fibrinolysis. Heart rate and HRV analyzed from predischarge 24-h electrocardiographic recordings were found to be more powerful predictors of mortality than ejection fraction in post-AMI patients in the study by Copie et al. (1996). Disturbed autonomic modulation of heart rate seems to be specifically related to susceptibility to ventricular fibrillation, but not to stable monomorphic ventricular tachycardia, suggesting that autonomic influences modify the presentation of malignant ventricular tachyarrhythmia after previous myocardial infarction (Perkiömäki et al., 1997).
The analysis of HRV can give an insight into various pathophysiological processes including the progression of focal coronary atherosclerosis (Huikuri et al., 1999a) and the angiographic severity of coronary artery disease (Hayano et al., 1990). Patients with coronary artery disease may have decreased vagal activity (Airaksinen et al., 1987) and abnormal circadian rhythm of HRV (Huikuri et al., 1994). Furthermore, ischemia may be preceded by increased sympathetic tone (Bernardi et al., 1988).
It is noteworthy that the HRV counterparts of autonomic drives are very different in patients with heart failure and in normal subjects (van de Borne et al., 1997). However, there are a lot of publications, which show that HRV is decreased in patients with congestive heart failure (Saul et al., 1988; Casolo et al., 1989, 1991; Kienzle et al., 1992; Nolan et al., 1992; Mortara et al., 1994). Saul et al. (1988) observed decreased vagal, but relatively well preserved sympathetic modulation of heart rate in patients with chronic stable congestive heart failure. On the contrary, reduction of all spectral components of HRV were found in patients with congestive heart failure in the study by Casolo et al. (1991). Brouwer et al. (1996) observed in 95 patients with mild to moderate heart failure that abnormal Poincare plots were independent predictors of all-cause and sudden cardiac death.
Hypertensive patients have been found to have increased low-frequency components and reduced high-frequency components of HRV (Guzetti et al., 1988). On the other hand, lower values of low- and high-frequency power of HRV were observed in hypertensive patients with left ventricular hypertrophy than in controls, while ultralow- and very-low-frequency components were similar in the groups (Petretta et al., 1995). Left ventricular hypertrophy is a well known risk indicator, and HRV has been shown to be inversely related to left ventricular mass index (Mandawat et al., 1994, 1995; Petretta et al., 1995). However, not all studies have confirmed this finding (Perkiömäki et al., 1996).
Disturbances in cardiac autonomic regulation assessed by HRV have been observed in several other conditions. Patients with diabetic neuropathy have decreased HRV (Wheeler and Watkins, 1973; Pfeifer et al., 1982; Smith, 1982). A reduced HRV in patients with diabetes still yields long-term prognostic information (Wheeler et al., 2002). In post-AMI patients the association between decreased HRV and long-term mortality has been observed to be at least as strong in diabetic patients as in non-diabetic patients (Whang and Bigger, 2003). However, there are also some data to suggest that including patients with diabetes decreases the association between HRV and mortality after AMI (Stein et al., 2004). The measurement of HRV offers also prognostic information in an elderly population beyond that provided by the assessment of conventional risk factors (Tsuji et al., 1994). Patients with chronic renal failure (Axelrod et al., 1987; Cloarec-Blanchard et al., 1992) have decreased HRV. Patients with different neurological conditions, such as Parkinsonism (Kuroiwa et al., 1983), multiple sclerosis (Neubauer and Gundersen, 1978), and quadriplegia (Inoue et al., 1990), have also been observed to have reduced HRV. Decreased HRV has been found in heart transplant recipients, but allograft rejection may lead to increased HRV (Sands et al., 1989).
Studies Applying Non-Linear Methods of Heart Rate Variability and Newer Studies
The fractal measures are the non-linear measures of HRV, whose prognostic significance has most widely been assessed in clinical studies including relevant number of patients and using well-defined endpoints. The fractal measures of HRV analysis have been shown to provide incremental prognostic information compared with the conventional measures of heart rate fluctuations. A basic feature of a fractal system is scale-invariance, i.e., same features repeat themselves on different measurement scales (Goldberger, 1996). Healthy subjects´ erratic fluctuations of sinus rhythm have fractal-like characteristics (Denton et al., 1990; Goldberger, 1990a).
Power-law HRV analysis is as a qualitative measure of the power spectrum in the region of the ultralow- and very-low-frequency bands obtained from long-term recordings. A plot of spectral power and frequency on bi-logarithmic scale shows linear portion between 10−4 and 10−2 Hz, and the slope of this relationship reflects long-term fractal-like scaling characteristics of HRV (Saul et al., 1987). Healthy subjects have power-law exponent values around −1 (Saul et al., 1987; Bigger et al., 1996), and the value of this slope has been observed to decrease with advancing age (Pikkujämsä et al., 1999). The finding that denervated hearts have a substantially steeper power-law slope emphasizes the important role of the autonomic nervous system in determining the steepness of the slope (Bigger et al., 1996). A steep power-law slope has been shown to be a better predictor of all-cause mortality or arrhythmic death than the traditional power–spectral bands in post-AMI patients (Bigger et al., 1996), and a better predictor of mortality than the traditional measurements of HRV in the elderly (Huikuri et al., 1998).
Detrended fluctuation analysis (DFA) quantifies intrinsic fractal-like correlation properties of dynamic systems (Peng et al., 1994). Peng et al. (1995) have described the details of this method. Briefly, the RR interval variability in relation to a local trend is analyzed in observation windows of different sizes in preprocessed and integrated RR interval time series. The RR interval variability is shown on a log–log scale as a function of the observation window size. In the presence of scaling, this relationship has a linear portion. The short-term scaling exponent (DFA1; for window sizes <11 beats) describes short-term scaling properties, and the intermediate-term scaling exponent (DFA2; for window sizes >11 beats) longer term scaling properties of the signal. Healthy middle-aged subjects have the short-term scaling exponent values somewhat over or around 1 (Pikkujämsä et al., 2001). The values of the short-term scaling exponent are determined by a complex interplay of the parasympathetic and sympathetic autonomic nervous system. Concomitant activation of both vagal and sympathetic outflow has been shown to decrease the short-term fractal scaling exponent resulting in a random heart rate behavior (Tulppo et al., 2005). The physiological background of the short-term scaling exponent has been discussed in detail elsewhere (Huikuri et al., 2009b). Healthy elderly subjects may have changes in the fractal correlation properties of heart rate dynamics (Iyengar et al., 1996; Pikkujämsä et al., 1999), and the short-term scaling exponent has also been observed to predict cardiac death in the elderly (Mäkikallio et al., 2001b).
There is some evidence that the short-term fractal-like scaling properties of heart rate dynamics analyzed by the DFA technique can yield prognostic information beyond that obtained by the conventional measures of HRV. Studies in post-AMI patients have suggested that decreased short-term scaling exponent is a better predictor of mortality than the conventional measurements of HRV (Mäkikallio et al., 1999a; Huikuri et al., 2000) and that decreased short-term scaling exponent values are associated with vulnerability to ventricular tachycardia (Mäkikallio et al., 1997), ventricular fibrillation (Mäkikallio et al., 1999b), arrhythmic death, and non-arrhythmic cardiac death (Huikuri et al., 2000). The prognostic value of the short-term scaling exponent has also been shown in a general post-AMI population without a marked left ventricular dysfunction and high proportion of patients on beta-blocking medication (Tapanainen et al., 2002). Fractal HRV has been observed to retain its prognostic value even when the vast majority of the patients were taking beta-blockers after AMI (Jokinen et al., 2003). Among the autonomic risk markers, the short-term scaling exponent has been found to be the strongest predictor of recurrent non-fatal coronary events after AMI (Perkiömäki et al., 2008). Disturbed cardiac autonomic regulation represented by reduced values of the short-term scaling exponent has been found to predict perpetuating ventricular tachyarrhythmias, but not self-terminating ventricular tachyarrhythmias in post-AMI patients with moderate left ventricular dysfunction suggesting that perpetuating and self-terminating ventricular tachyarrhythmias may have differences in factors, which modify arrhythmias (Perkiömäki et al., 2011; Figure 1). Figure 2 shows two typical cases of RR interval behavior in high-risk patients with low short-term fractal scaling exponent and one case with normal fractal scaling exponent.
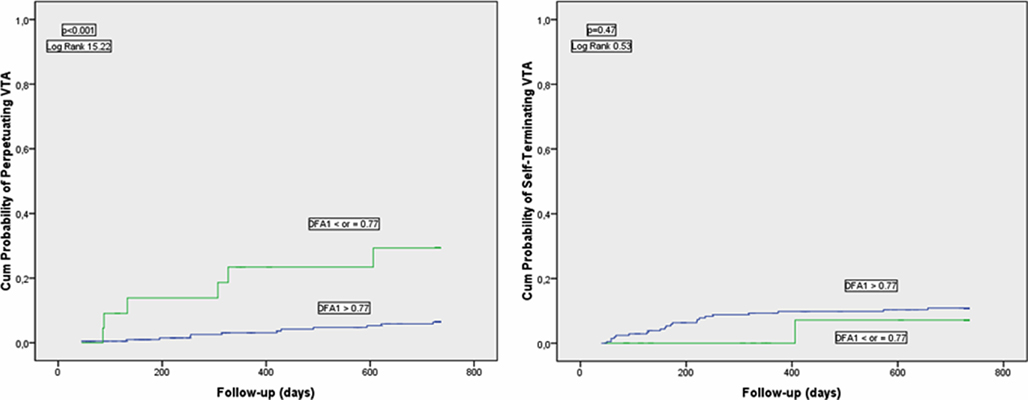
Figure 1. Cumulative proportional probability of perpetuating (left chart) and self-terminating (right chart) ventricular tachyarrhythmias (VTA) for patients with the short-term scaling exponent obtained by the detrended fluctuation analysis (DFA1) ≤ or >0.77. Reproduced with a permission from Perkiömäki et al. (2011).
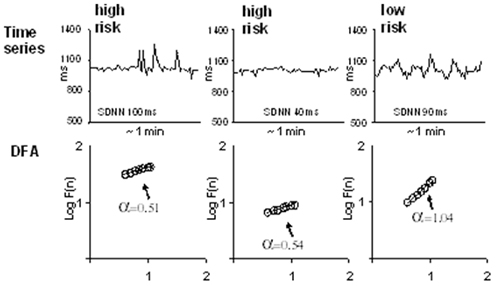
Figure 2. RR interval tachograms in two patients at high-risk for cardiac mortality who have low short-term fractal scaling exponent (α; left and middle) and one patient with low risk and normal short-term fractal scaling exponent. Reproduced with a permission from Huikuri et al. (2009b).
Heart failure patients show loss of fractal organization in heart rate dynamics (Peng et al., 1995), and this is associated with the risk of death (Ho et al., 1997). Reduced short-term scaling exponent is more closely related to the risk of mortality in patients with less severe than in those with more advanced heart failure (Mäkikallio et al., 2001a). The short-term scaling exponent has also been shown to predict long-term risk for heart failure hospitalization after AMI (Perkiömäki et al., 2010).
The spontaneous onset of atrial fibrillation in patients without a structural heart disease is preceded by altered short-term fractal-like scaling properties of HRV (Vikman et al., 1999), and the short-term scaling exponent changes toward more random direction in ectopic tachycardia reflecting disturbances in autonomic regulation or in ectopic atrial pacemakers (Huikuri et al., 1999b).
Some of the non-linear measures of HRV, such as the short-term scaling exponent, have some advantages over the conventional measures of HRV considering risk stratification purposes. These advantages include: less dependency on heart rate, less inter-individual and intra-individual variation (Perkiömäki et al., 2001c; Pikkujämsä et al., 2001; Maestri et al., 2007), smaller relative changes of individual values over time after AMI (Perkiömäki et al., 2001c), and relatively good comparability of individual values between long-term and short-term electrocardiographic recordings (Perkiömäki et al., 2001b).
The cardiac arrhythmias and risk stratification after acute myocardial infarction (CARISMA) study included patients, who had left ventricular ejection fraction ≤40% measured from 3 to 21 days after AMI. Several HRV measures, when analyzed from 24-h ECGs recorded at 6 weeks after the AMI, predicted the primary endpoint of ventricular fibrillation or symptomatic sustained ventricular tachycardia. However, the short-term scaling exponent was the only HRV measure, when analyzed from 24-h ECGs recorded at 1 week after the AMI, which significantly predicted the primary endpoint. It is noteworthy that the arrhythmic endpoint events were objectively detected using implantable loop recorders in the CARISMA study (Huikuri et al., 2009a), whereas in most of the previous studies arrhythmic events/sudden arrhythmic cardiac death have been evaluated on clinical basis. In the recent non-invasive risk assessment early after a myocardial infarction (REFINE) study (Exner et al., 2007), which included post-AMI patients with somewhat better preserved left ventricular function than the CARISMA study, HRV measured from 10 to 14 weeks after the AMI tended to predict the primary endpoint of cardiac death or resuscitated cardiac arrest, but had no association with the primary endpoint when measured from 2 to 4 weeks after the AMI. These notions underline the importance of timing for measurement of HRV after AMI.
The data on the prognostic significance of the complexity measures of HRV are limited (Huikuri et al., 2009b). Decreased multiscale entropy of heart rate has been observed to predict mortality in trauma patients (Norris et al., 2008a,b). There has been shown to be association with reduced complexity in heart rate dynamics and postoperative complications after vascular surgery (Fleisher et al., 1993). Decreased complexity in heart rate behavior measured by approximate entropy has been found to precede spontaneous episodes of atrial fibrillation in patients without structural heart disease (Vikman et al., 1999) and in patients after coronary artery bypass surgery (Hogue et al., 1998). Furthermore, the complexity of heart rate dynamics has been observed to reduce during 1 year follow-up after coronary artery bypass operation (Laitio et al., 2006).
Usefulness of Heart Rate Variability in Clinical Decision Making and Future Perspectives
Despite a large body of evidence documenting the predictive value of various HRV indices, none of these methods are in widespread clinical use at the moment. If patients would be risk stratified to a therapy based on a HRV measurement, the therapy should improve the patients’ outcome. Therefore it should be evaluated in well-designed prospective randomized studies, whether the selection of the patients to the therapy based on potentially useful HRV measurements improves the prognosis. An ideal risk stratifier should specifically predict sudden arrhythmic death as an implantable cardioverter-defibrillator (ICD) therapy is effective in preventing such events. The patients with severely depressed left ventricular function are at highest risk for life-threatening ventricular arrhythmias (Huikuri et al., 2001), however, it has been found that HRV measurements work prognostically better in patients with more preserved left ventricular function (Mäkikallio et al., 2005) than in those with more severe left ventricular dysfunction. Additionally, there is evidence to support the concept that disturbed HRV predicts cardiac death in general and not specifically sudden arrhythmic death in patients with severe left ventricular dysfunction (Perkiömäki et al., 2001a; Zareba et al., 2003). On the other hand, there are numerically more sudden deaths among lower risk post-AMI patients with better preserved left ventricular function (Huikuri et al., 2001). Furthermore, as described above the results of the CARISMA study show that measures of HRV analyzed from electrocardiographic recordings obtained several weeks after AMI predict life-threatening ventricular tachyarrhythmias in post-AMI patients with moderately depressed left ventricular function (Huikuri et al., 2009a). Taken together, the post-AMI patients with moderate/mild left ventricular dysfunction would be the best target population for future prospective studies aiming to assess whether an intervention based on the assessment of HRV improves the outcome. In the CARISMA study, according to the receiver operator characteristics curve analysis, e.g., the short-term scaling exponent analyzed from 24-h ECGs recorded at 6 weeks after the AMI had the area under the curve of order of 0.75 for predicting life-threatening ventricular tachyarrhythmias. This can be considered potentially useful accuracy for risk stratification purposes. However, the accuracy of this level does not simultaneously allow both an excellent specificity and sensitivity for any selected cutpoint. Therefore it would be important to try to find additional good risk markers, which could in combination with HRV measurements increase the accuracy in predicting sudden arrhythmic death. This is particularly important, if ICD therapy is considered based on this risk stratification strategy as ICD implantation and therapy suffer from potential complications and discomfort and are relatively expensive. Severely depressed left ventricular function is considered to be a sufficient risk indicator for prophylactic ICD implantation in post-AMI patients with acceptable numbers needed to treat values for getting a benefit (Moss et al., 2002; Bardy et al., 2005). However, most of the post-AMI patients with better preserved left ventricular function at high-risk for sudden arrhythmic death do not get a primary prophylactic ICD therapy. Taken together, it would therefore be justified to consider to apply a cutpoint with compromised sensitivity of a HRV measurement to get high specificity, high positive predictive accuracy, and a low number needed to treat value in future prospective randomized prophylactic ICD studies in post-AMI patients with moderate/mild left ventricular dysfunction.
Despite many advancements, the analysis of HRV is still far from routine clinical use. Before it can be a useful tool for clinicians, at least the following questions need clarification: what would be the optimal timing for HRV analysis after AMI? What would be the optimal target population for the use of HRV analysis as a risk stratifier? What method(s) should be used for HRV analysis in clinical settings? What would be the optimal preprocessing method for editing premature depolarizations for different HRV parameters in different clinical settings? What would be the recommendable cutpoints of selected HRV measurements for risk stratification purposes in different cardiac conditions for different endpoints, in a same cardiac condition with different degree of left ventricular dysfunction and heart failure, and in different age and gender groups? What would be a recommendable length of ECG recording? Under what kind of conditions should the ECG recordings be done? What is the accuracy of selected HRV measures in predicting different adverse events in different cardiac conditions? What would be the expected benefits of HRV analysis to patients’ further evaluation and treatment? What would be the number needed to treat value, e.g., to get benefit from ICD therapy? Could the HRV analysis be used in the patients’ follow-up, etc.? Hopefully, after further developments and standardization the HRV analysis alone or with other risk indicators will soon serve as a useful tool for risk stratification.
Conflict of Interest Statement
The author declares that the research was conducted in the absence of any commercial or financial relationships that could be construed as a potential conflict of interest.
References
Airaksinen, K. E., Ikäheimo, M. J., Linnaluoto, M. K., Niemelä, M., and Takkunen, J. T. (1987). Impaired vagal heart rate control in coronary artery disease. Br. Heart J. 58, 592–597.
Axelrod, S., Lishner, M., Oz, O., Bernheim, J., and Ravid, M. (1987). Spectral analysis of fluctuations in heart rate: an objective evaluation of autonomic nervous control in chronic renal failure. Nephron 45, 202–206.
Bardy, G. H., Lee, K. L., Mark, D. B., Poole, J. E., Packer, D. L., Boineau, R., Domanski, M., Troutman, C., Anderson, J., Johnson, G., McNulty, S. E., Clapp-Channing, N., Davidson-Ray, L. D., Fraulo, E. S., Fishbein, D. P., Luceri, R. M., Ip, J. H., and Sudden Cardiac Death in Heart Failure Trial (SCD-HeFT) Investigators. (2005). Amiodarone or an implantable cardioverter-defibrillator for congestive heart failure. N. Engl. J. Med. 352, 225–237. [Erratum in: N. Engl. J. Med. 352, 2146].
Bernardi, L., Lumina, C., Ferrari, M. R., Ricordi, L., Vandea, I., Fratino, P., Piva, M., and Finardi, G. (1988). Relationship between fluctuations in heart rate and asymptomatic nocturnal ischemia. Int. J. Cardiol. 20, 39–51.
Bigger, J. T., Fleiss, J., Steinman, R. C., Rolnitzky, L. M., Kleiger, R. E., and Rottman, J. N. (1992). Frequency domain measures of heart period variability and mortality after myocardial infarction. Circulation 85, 164–171.
Bigger, J. T., Fleiss, J. L., Rolnitzky, L. M., and Steinman, R. C. (1993). The ability of several short-term measures of RR variability to predict mortality after myocardial infarction. Circulation 88, 927–934.
Bigger, J. T. Jr., Steinman, R. C., Rolnitzky, L. M., Fleiss, J. L., Albrecht, P., and Cohen, R. J. (1996). Power law behavior of RR-interval variability in healthy middle-aged persons, patients with recent acute myocardial infarction, and patients with heart transplants. Circulation 93, 2142–2151.
Brouwer, J., van Veldhuisen, D. J., Manint Veld, A. J., Haaksma, J., Dijk, W. A., Visser, K. R., Boomsma, F., Dunselman, P. H. J. M., Lie, K. I., and For the Dutch Ibopamine Multicenter Trial Study Group. (1996). Prognostic value of heart rate variability during long-term follow-up in patients with mild to moderate heart failure. J. Am. Coll. Cardiol. 28, 1183–1189.
Casolo, G., Balli, E., Fazi, A., Gori, C., Freni, A., and Gensini, G. (1991). Twenty-four-hour spectral analysis of heart rate variability in congestive heart failure secondary to coronary artery disease. Am. J. Cardiol. 67, 1154–1158.
Casolo, G., Balli, E., Taddei, T., Amuhasi, J., and Gori, C. (1989). Decreased spontaneous heart rate variability on congestive heart rate failure. Am. J. Cardiol. 64, 1162–1167.
Cloarec-Blanchard, L., Girard, A., Houhou, S., Grunveld, J. P., and Elghozi, J. L. (1992). Spectral analysis of short-term blood pressure and heart rate variability in uremic patients. Kidney Int. 41(Suppl. 37), S14–S18.
Copie, X., Hnatkova, K., Staunton, A., Fei, L., Camm, A. J., and Malik, M. (1996). Predictive power of increased heart rate versus depressed left ventricular ejection fraction and heart rate variability for risk stratification after myocardial infarction. Results of a two-year follow-up study. J. Am. Coll. Cardiol. 27, 270–276.
Cripps, T. R., Malik, M., Farrell, T. G., and Camm, A. J. (1991). Prognostic value of reduced heart rate variability after myocardial infarction: clinical evaluation of a new analysis method. Br. Heart J. 65, 14–19.
Denton, T. A., Diamond, G. A., Helfant, R. H., Khan, S., and Karagueuzian, H. (1990). Fascinating rhythm: a prime on chaos theory and its application to cardiology. Am. Heart J. 120, 1419–1440.
Exner, D. V., Kavanagh, K. M., Slawnych, M. P., Mitchell, L. B., Ramadan, D., Aggarwal, S. G., Noullett, C., Van Schaik, A., Mitchell, R. T., Shibata, M. A., Gulamhussein, S., McMeekin, J., Tymchak, W., Schnell, G., Gillis, A. M., Sheldon, R. S., Fick, G. H., Duff, H. J., and Refine Investigators. (2007). Noninvasive risk assessment early after a myocardial infarction the REFINE study. J. Am. Coll. Cardiol. 50, 2275–2284.
Farrell, T. G., Bashir, Y., Cripps, T., Malik, M., Poloniecki, J., Bennett, E. D., Ward, D. E., and Camm, A. J. (1991). Risk stratification for arrhythmic events in postinfarction patients based on heart rate variability, ambulatory electrocardiographic variables and the signal-averaged electrocardiogram. J. Am. Coll. Cardiol. 18, 687–697.
Fei, L., Copie, X., Malik, M., and Camm, A. J. (1996). Short- and long-term assessment of heart rate variability for risk stratification after acute myocardial infarction. Am. J. Cardiol. 77, 681–684.
Fleisher, L. A., Pincus, S. M., and Rosenbaum, S. H. (1993). Approximate entropy of heart rate as a correlate of postoperative ventricular dysfunction. Anesthesiology 78, 683–692.
Goldberger, A. L. (1990a). Fractal electrodynamics of the heartbeat. Ann. N. Y. Acad. Sci. 591, 402–409.
Goldberger, A. L. (1990b). Nonlinear dynamics, fractals and chaos: applications to cardiac electrophysiology. Ann. Biomed. Eng. 18, 195–198.
Goldberger, A. L. (1996). Non-linear dynamics for clinicians: chaos theory, fractals, and complexity at the bedside. Lancet 347, 1312–1314.
Guzetti, S., Piccaluga, E., Casati, R., Cerutti, S., Lombardi, F., Pagani, M., and Malliani, A. (1988). Sympathetic predominance in essential hypertension: a study employing spectral analysis of heart rate variability. J. Hypertens. 6, 711–717.
Hartikainen, J., Malik, M., Staunton, A., Poloniecki, J., and Camm, A. J. (1996). Distinction between arrhythmic and nonarrhythmic death after acute myocardial infarction based on heart rate variability, signal-averaged electrocardiogram, ventricular arrhythmias and left ventricular ejection fraction. J. Am. Coll. Cardiol. 28, 296–304.
Hayano, J., Sakakibara, Y., Yamada, M., Ohte, N., Fujinami, T., Yokoyama, K., Watanabe, Y., and Takata, K. (1990). Decreased magnitude of heart spectral components in coronary artery disease. Its relation to angiographic severity. Circulation 81, 1217–1224.
Ho, K. K., Moody, G. B., Peng, C. K., Mietus, J. E., Larson, M. G., Levy, D., and Goldberger, A. L. (1997). Predicting survival in heart failure case and control subjects by use of fully automated methods for deriving nonlinear and conventional indices of heart rate dynamics. Circulation 96, 842–848.
Hogue, C. W. Jr., Domitrovich, P. P., Stein, P. K., Despotis, G. D., Re, L., Schuessler, R. B., Kleiger, R. E., and Rottman, J. N. (1998). RR interval dynamics before atrial fibrillation in patients after coronary artery bypass graft surgery. Circulation 98, 429–434.
Huikuri, H. V., Castellanos, A., and Myerburg, R. J. (2001). Sudden death due to cardiac arrhythmias. N. Engl. J. Med. 345, 1473–1482.
Huikuri, H. V., Jokinen, V., Syvänne, M., Nieminen, M. S., Airaksinen, K. E., Ikäheimo, M. J., Koistinen, J. M., Kauma, H., Kesäniemi, A. Y., Majahalme, S., Niemelä, K. O., and Frick, M. H. (1999a). Heart rate variability and progression of coronary atherosclerosis. Arterioscler. Thromb. Vasc. Biol. 19, 1979–1985.
Huikuri, H. V., Poutiainen, A. M., Mäkikallio, T. H., Koistinen, M. J., Airaksinen, K. E., Mitrani, R. D., Myerburg, R. J., and Castellanos, A. (1999b). Dynamic behaviour and autonomic regulation of ectopic atrial pacemakers. Circulation 100, 1416–1422.
Huikuri, H. V., Mäkikallio, T. H., Airaksinen, K. E., Seppänen, T., Puukka, P., Räiha, I. J., and Sourander, L. B. (1998). Power-law relationship of heart rate variability as a predictor of mortality in the elderly. Circulation 97, 2031–2036.
Huikuri, H. V., Mäkikallio, T. H., Peng, C. K., Goldberger, A. L., Hintze, U., and Moller, M. (2000). Fractal correlation properties of R-R interval dynamics and mortality in patients with depressed left ventricular function after an acute myocardial infarction. Circulation 101, 47–53.
Huikuri, H. V., Niemelä, M. J., Ojala, S., Rantala, A., Ikäheimo, M. J., and Airaksinen, K. E. J. (1994). Circadian rhythms of frequency domain measures of heart rate variability in healthy subjects and patients with coronary artery disease. Effects of arousal and upright posture. Circulation 90, 121–126.
Huikuri, H. V., Raatikainen, M. J., Moerch-Joergensen, R., Hartikainen, J., Virtanen, V., Boland, J., Anttonen, O., Hoest, N., Boersma, L. V., Platou, E. S., Messier, M. D., and Bloch-Thomsen, P. E. (2009a). Prediction of fatal or near-fatal cardiac arrhythmia events in patients with depressed left ventricular function after an acute myocardial infarction. Eur. Heart J. 30, 689–698.
Huikuri, H. V., Perkiömäki, J. S., Maestri, R., and Pinna, G. D. (2009b). Clinical impact of evaluation of cardiovascular control by novel methods of heart rate dynamics. Philos. Transact. A Math. Phys. Eng. Sci. 367, 1223–1238.
Inoue, K., Miyake, S., Kumashiro, M., Ogata, H., and Yoshimura, O. (1990). Power spectral analysis of heart rate variability in traumatic quadriplegic humans. Am. J. Physiol. 258, H1722–H1726.
Iyengar, N., Peng, C. K., Morin, R., Goldberger, A. L., and Lipsitz, L. A. (1996). Age-related alterations in the fractal scaling of cardiac interbeat interval dynamics. Am. J. Physiol. 271, R1078–R1084.
Jokinen, V., Tapanainen, J. M., Seppänen, T., and Huikuri, H. V. (2003). Temporal changes and prognostic significance of measures of heart rate dynamics after acute myocardial infarction in the beta-blocking era. Am. J. Cardiol. 92, 907–912.
Kienzle, M. G., Ferguson, D. W., Birkett, C. L., Myers, G. A., Berg, W. J., and Mariano, D. J. (1992). Clinical, hemodynamic and sympathetic neural correlates of heart rate variability in congestive heart failure. Am. J. Cardiol. 69, 761–767.
Kleiger, R. E., Miller, J. P., Bigger, J. T. Jr., Moss, A. J., and The Multicenter Post-Infarction Research Group. (1987). Decreased heart rate variability and its association with increased mortality after acute myocardial infarction. Am. J. Cardiol. 59, 256–262.
Kuroiwa, Y., Shimada, Y., and Toyokura, Y. (1983). Postural hypotension and low R-R interval variability in parkinsonism, spino-cerebellar degeneration, and Shy-Drager syndrome. Neurology 33, 463–467.
Laitio, T. T., Huikuri, H. V., Koskenvuo, J., Jalonen, J., Mäkikallio, T. H., Helenius, H., Kentala, E. S., Hartiala, J., and Scheinin, H. (2006). Long-term alterations of heart rate dynamics after coronary artery bypass graft surgery. Anesth. Analg. 102, 1026–1031.
Maestri, R., Pinna, G. D., Porta, A., Balocchi, R., Sassi, R., Signorini, M. G., Dudziak, M., and Raczak, G. (2007). Assessing nonlinear properties of heart rate variability from short-term recordings: are these measurements reliable? Physiol. Meas. 28, 1067–1077.
Mäkikallio, T. H., Barthel, P., Schneider, R., Bauer, A., Tapanainen, J. M., Tulppo, M. P., Schmidt, G., and Huikuri, H. V. (2005). Prediction of sudden cardiac death after acute myocardial infarction: role of Holter monitoring in the modern treatment era. Eur. Heart J. 26, 762–769.
Mäkikallio, T. H., Hoiber, S., Kober, L., Torp-Pedersen, C., Peng, C. K., Goldberger, A. L., and Huikuri, H. V. (1999a). Fractal analysis of heart rate dynamics as a predictor of mortality in patients with depressed left ventricular function after acute myocardial infarction. TRACE Investigators. TRAndolapril cardiac evaluation. Am. J. Cardiol. 83, 836–839.
Mäkikallio, T. H., Koistinen, J., Jordaens, L., Tulppo, M. P., Wood, N., Golosarsky, B., Peng, C. K., Goldberger, A. L., and Huikuri, H. V. (1999b). Heart rate dynamics before spontaneous onset of ventricular fibrillation in patients with healed myocardial infarcts. Am. J. Cardiol. 83, 880–884.
Mäkikallio, T. H., Huikuri, H. V., Hintze, U., Videbaek, J., Mitrani, R. D., Castellanos, A., Myerburg, R. J., and Moller, M. (2001a). Fractal analysis and time- and frequency-domain measures of heart rate variability as predictors of mortality in patients with heart failure. Am. J. Cardiol. 87, 178–182.
Mäkikallio, T. H., Huikuri, H. V., Mäkikallio, A., Sourander, L. B., Mitrani, R. D., Castellanos, A., and Myerburg, R. J. (2001b). Prediction of sudden cardiac death by fractal analysis of heart rate variability in elderly subjects. J. Am. Coll. Cardiol. 37, 1395–1402.
Mäkikallio, T. H., Seppänen, T., Airaksinen, K. E., Koistinen, J., Tulppo, M. P., Peng, C. K., Goldberger, A. L., and Huikuri, H. V. (1997). Dynamic analysis of heart rate may predict subsequent ventricular tachycardia after myocardial infarction. Am. J. Cardiol. 80, 779–783.
Mandawat, M. K., Wallbridge, D. R., Pringle, S. D., Riyami, A. A. S., Latif, S., Macfarlane, P. W., Lorimer, A. R., and Cobbe, S. M. (1994). Impaired heart rate variability and increased ventricular ectopic activity in patients with left ventricular hypertrophy. J. Electrocardiol. 27(Suppl.), 179–181.
Mandawat, M. K., Wallbridge, D. R., Pringle, S. D., Riyami, A. A. S., Latif, S., Macfarlane, P. W., Lorimer, A. R., and Cobbe, S. M. (1995). Heart rate variability in left ventricular hypertrophy. Br. Heart J. 73, 139–144.
Mortara, A., La Rovere, M. T., Signorini, M. G., Pantaleo, P., Pinna, G., Martinelli, L., Ceconi, C., Cerutti, S., and Tavazzi, L. (1994). Can power spectral analysis of heart rate variability identify a high risk subgroup of congestive heart failure patients with excessive sympathetic activation? A pilot study before and after heart transplantation. Br. Heart J. 71, 422–430.
Moss, A. J., Zareba, W., Hall, W. J., Klein, H., Wilber, D. J., Cannom, D. S., Daubert, J. P., Higgins, S. L., Brown, M. W., Andrews, M. L., and Multicenter Automatic Defibrillator Implantation Trial II Investigators. (2002). Prophylactic implantation of a defibrillator in patients with myocardial infarction and reduced ejection fraction. N. Engl. J. Med. 346, 877–883.
Neubauer, B., and Gundersen, H. J. (1978). Analysis of heart rate variations in patients with multiple sclerosis. A simple measure of autonomic nervous disturbances using an ordinary ECG. J. Neurol. Neurosurg. Psychiatr. 41, 417–419.
Nolan, J., Batin, P. D., Andrews, R., Lindsay, S. J., Brooksby, P., Mullen, M., Baig, W., Flapan, A. D., Cowley, A., Prescott, R. J., Neilson, J. M., and Fox, K. A. (1998). Propective study of heart rate variability and mortality in chronic heart failure. Results of the United Kingdom heart failure evaluation and assessment of risk trial (UK-Heart). Circulation 98, 1510–1516.
Nolan, J., Flapan, A. D., Capewell, S., MacDonald, T. M., Neilson, J. M. M., and Ewing, D. J. (1992). Decreased cardiac parasympathetic activity in chronic heart failure and its relation to left ventricular function. Br. Heart J. 67, 482–485.
Norris, P. R., Anderson, S. M., Jenkins, J. M., Williams, A. E., and Morris, J. A. Jr. (2008a). Heart rate multiscale entropy at three hours predicts hospital mortality in 3,154 trauma patients. Shock 30, 17–22.
Norris, P. R., Stein, P. K., and Morris, J. A. Jr. (2008b). Reduced heart rate multiscale entropy predicts death in critical illness: a study of physiologic complexity in 285 trauma patients. J. Crit. Care 23, 399–405.
Odemuyiwa, O., Poloniecki, J., Malik, M., Farrell, T., Xia, R., Staunton, A., Kulakowski, P., Ward, D., and Camm, J. (1994). Temporal influences on the prediction of postinfarction mortality by heart rate variability: a comparison with the left ventricular ejection fraction. Br. Heart J. 71, 521–527.
Peng, C. K., Buldyrev, S. V., Havlin, S., Simons, M., Stanley, H. E., and Goldberger, A. L. (1994). Mosaic organization of DNA nucleotides. Phys. Rev. E49, 1685–1689.
Peng, C. K., Havlin, S., Stanley, H. E., and Goldberger, A. L. (1995). Quantification of scaling exponents and crossover phenomena in nonstationary heartbeat time series. Chaos 5, 82–87.
Perkiömäki, J. S., Bloch Thomsen, P. E., Kiviniemi, A. M., Messier, M. D., Huikuri, H. V., and For the CARISMA Study Investigators. (2011). Risk factors of self-terminating and perpetuating ventricular tachyarrhythmias in post-infarction patients with moderately depressed left ventricular function, a CARISMA sub-analysis. Europace 13, 1604–1611.
Perkiömäki, J. S., Hämekoski, S., Junttila, M. J., Jokinen, V., Tapanainen, J., and Huikuri, H. V. (2010). Predictors of long-term risk for heart failure hospitalization after acute myocardial infarction. Ann. Noninvasive Electrocardiol. 15, 250–258.
Perkiömäki, J. S., Huikuri, H. V., Koistinen, J. M., Mäkikallio, T., Castellanos, A., and Myerburg, R. J. (1997). Heart rate variability and dispersion of QT interval in patients with vulnerability to ventricular tachycardia and ventricular fibrillation after previous myocardial infarction. J. Am. Coll. Cardiol. 30, 1331–1338.
Perkiömäki, J. S., Ikäheimo, M. J., Pikkujämsä, S. M., Rantala, A., Lilja, M., Kesäniemi, Y. A., and Huikuri, H. V. (1996). Dispersion of the QT interval and autonomic modulation of heart rate in hypertensive men with and without left ventricular hypertrophy. Hypertension 28, 16–21.
Perkiömäki, J. S., Jokinen, V., Tapanainen, J., Airaksinen, K. E., and Huikuri, H. V. (2008). Autonomic markers as predictors of nonfatal acute coronary events after myocardial infarction. Ann. Noninvasive Electrocardiol. 13, 120–129.
Perkiömäki, J. S., Zareba, W., Daubert, J. P., Couderc, J. P., Corsello, A., and Kremer, K. (2001a). Fractal correlation properties of heart rate dynamics and adverse events in patients with implantable cardioverter-defibrillators. Am. J. Cardiol. 88, 17–22.
Perkiömäki, J. S., Zareba, W., Kalaria, V. G., Couderc, J., Huikuri, H. V., and Moss, A. J. (2001b). Comparability of nonlinear measures of heart rate variability between long- and short-term electrocardiographic recordings. Am. J. Cardiol. 87, 905–908.
Perkiömäki, J. S., Zareba, W., Ruta, J., Dubner, S., Madoery, C., Deedwania, P., Karcz, M., Bayes de Luna, A., and Ideal Investigators. (2001c). Fractal and complexity measures of heart rate dynamics after acute myocardial infarction. Am. J. Cardiol. 88, 777–781.
Petretta, M., Marciano, F., Bianchi, V., Migaux, M. L., Valva, G., De Luca, N., Salemme, L., Berardino, S., and Bonaduce, D. (1995). Power spectral analysis fo heart period variability in hypertensive patients with left ventricular hypertrophy. Am. J. Hypertens. 8, 1206–1213.
Pfeifer, M. A., Cook, D., Brodsky, J., Tice, D., Reenan, A., Swedine, S., Halter, J. B., and Porte, D. Jr. (1982). Quantitative evaluation of cardiac parasympathetic activity in normal and diabetic man. Diabetes 31, 339–345.
Pikkujämsä, S. M., Mäkikallio, T. H., Airaksinen, K. E., and Huikuri, H. V. (2001). Determinants and interindividual variation of R-R interval dynamics in healthy middle-aged subjects. Am. J. Physiol. Heart Circ. Physiol. 280, H1400–H1406.
Pikkujämsä, S. M., Mäkikallio, T. H., Sourander, L. B., Räiha, I. J., Puukka, P., Skytta, J., Peng, C. K., Goldberger, A. L., and Huikuri, H. V. (1999). Cardiac interbeat interval dynamics from childhood to senescence: comparison of conventional and new measures based on fractals and chaos theory. Circulation 100, 393–399.
Pincus, S. M., and Goldberger, A. L. (1994). Physiologic time-series analysis: what does regularity quantify? Am. J. Physiol. 226, H1643–H1656.
Sands, K. E., Appel, M. L., Lilly, L. S., Schoen, F. J., Mudge, G. H. Jr., and Cohen, R. J. (1989). Power spectrum analysis of heart rate variability in human cardiac transplant recipients. Circulation 79, 76–82.
Saul, J. P., Albrecht, P., Berger, R. D., and Cohen, R. J. (1987). “Analysis of long-term heart rate variability: methods, 1/f scaling and implications,” in Computers in Cardiology (Silver Spring, MD: IEEE Computer Society Press), 419–:422.
Saul, J. P., Arai, Y., Berger, R. D., Lilly, L. S., Colucci, W. S., and Cohen, R. J. (1988). Assessment of autonomic regulation in chronic congestive heart failure by heart rate spectral analysis. Am. J. Cardiol. 61, 1292–1299.
Schmidt, G., Malik, M., Barthel, P., Schneider, R., Ulm, K., Rolnitzky, L., Camm, A. J., Bigger, J. T. Jr., and Schömig, A. (1999). Heart-rate turbulence after ventricular premature beats as a predictor of mortality after acute myocardial infarction. Lancet 353, 1390–1396.
Schneider, R. A., and Costiloe, J. P. (1965). Relationship of sinus arrhythmia to age and its prognostic significance in ischemic heart disease. Clin. Res. 13, 219.
Skinner, J. E., Pratt, C. M., and Vybiral, T. (1993). A reduction in the correlation dimension of heartbeat intervals precedes imminent ventricular fibrillation in human subjects. Am. Heart J. 125, 731–743.
Smith, S. A. (1982). Reduced sinus arrhythmia in diabetic autonomic neuropathy: diagnostic value of an age-related normal range. Br. Med. J. 285, 1599–1601.
Stein, P. K., Domitrovich, P. P., Kleiger, R. E., and Cast Investigators. (2004). Including patients with diabetes mellitus or coronary artery bypass grafting decreases the association between heart rate variability and mortality after myocardial infarction. Am. Heart J. 147, 309–316.
Tapanainen, J. M., Thomsen, P. E., Kober, L., Torp-Pedersen, C., Mäkikallio, T. H., Still, A. M., Lindgren, K. S., and Huikuri, H. V. (2002). Fractal analysis of heart rate variability and mortality after an acute myocardial infarction. Am. J. Cardiol. 90, 347–352.
Task Force of the European Society of Cardiology and the North American Society of Pacing and Electrophysiology. (1996). Heat rate variability. Standards of measurement, physiological interpretation, and clinical use. Circulation 93, 1043–1065.
Tsuji, H., Venditti, F. J. Jr., Manders, E. S., Evans, J. C., Larson, M. G., Feldman, C. L., and Levy, D. (1994). Reduced heart rate variability and mortality risk in an elderly cohort. The Framingham Heart Study. Circulation 90, 878–883.
Tulppo, M. P., Kiviniemi, A. M., Hautala, A. J., Kallio, M., Seppänen, T., Mäkikallio, T. H., and Huikuri, H. V. (2005). Physiological background of the loss of fractal heart rate dynamics. Circulation 112, 314–319.
Tuzcu, V., Nas, S., Börklü, T., and Ugur, A. (2006). Decrease in the heart rate complexity prior to the onset of atrial fibrillation. Europace 8, 398–402.
Vaishnav, S., Stevenson, R., Marchant, B., Lagi, K., Ranjadayalan, K., and Timmis, A. D. (1994). Relation between heart rate variability early after acute myocardial infarction and long-term mortality. Am. J. Cardiol. 73, 653–657.
van de Borne, P., Montano, N., Pagani, M., Oren, R., and Somers, V. K. (1997). Absence of low-frequency variability of sympathetic nerve activity in severe heart failure. Circulation 95, 1449–1454.
Vikman, S., Mäkikallio, T. H., Yli-Mäyry, S., Pikkujämsä, S., Koivisto, A. M., Reinikainen, P., Airaksinen, K. E., and Huikuri, H. V. (1999). Altered complexity and correlation properties of R-R interval dynamics before the spontaneous onset of paroxysmal atrial fibrillation. Circulation 100, 2079–2084.
Voss, A., Hnatkova, K., Wessel, N., Kurths, J., Sander, A., Schirdewan, A., Camm, A. J., and Malik, M. (1998). Multiparametric analysis of heart rate variability used for risk stratification among survivors of acute myocardial infarction. Pacing Clin. Electrophysiol. 21, 186–192.
Whang, W., and Bigger, J. T. Jr. (2003). Comparison of the prognostic value of RR-interval variability after acute myocardial infarction in patients with versus those without diabetes mellitus. Am. J. Cardiol. 92, 247–251.
Wheeler, S. G., Ahroni, J. H., and Boyko, E. J. (2002). Prospective study of autonomic neuropathy as a predictor of mortality in patients with diabetes. Diabetes Res. Clin. Pract. 58, 131–138.
Wolf, M. M., Varigos, G. A., Hunt, D., and Sloman, J. G. (1978). Sinus arrhythmia in acute myocardial infarction. Med. J. Aust. 2, 52–53.
Zareba, W., Couderc, J. P., Perkiömäki, J. S., Berkowitzch, A., and Moss, A. J. (2003). Heart rate variability and outcome in postinfarction patients with severe left ventricular dysfunction. Circulation 108(Suppl.), :3209.
Keywords: heart rate, heart rate variability, non-linear methods, mortality, sudden death
Citation: Perkiömäki JS (2011) Heart rate variability and non-linear dynamics in risk stratification. Front. Physio. 2:81. doi: 10.3389/fphys.2011.00081
Received: 24 August 2011;
Paper pending published: 13 September 2011;
Accepted: 21 October 2011;
Published online: 09 November 2011.
Edited by:
Heikki Veli Huikuri, University of Oulu, FinlandReviewed by:
Juha Koskenvuo, University of Turku, FinlandTomi Laitinen, University of Eastern Finland and Kuopio University Hospital, Finland
Copyright: © 2011 Perkiömäki. This is an open-access article subject to a non-exclusive license between the authors and Frontiers Media SA, which permits use, distribution and reproduction in other forums, provided the original authors and source are credited and other Frontiers conditions are complied with.
*Correspondence: Juha S. Perkiömäki, Division of Cardiology, Department of Medicine, University of Oulu, P.O. Box 5000 (Kajaanintie 50), Fin-90014, Oulu, Finland. e-mail: juha.perkiomaki@oulu.fi