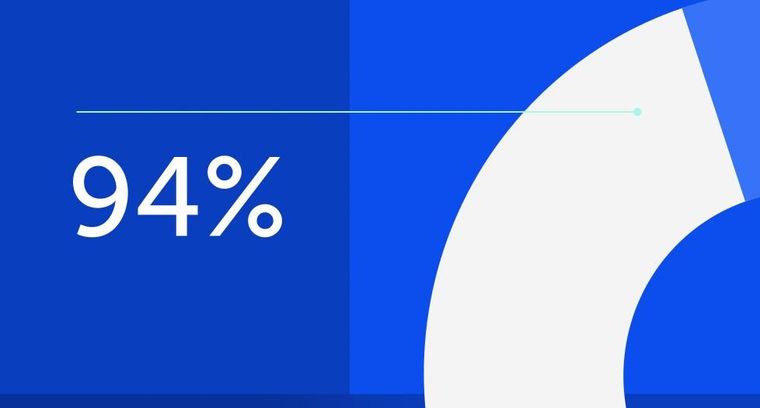
94% of researchers rate our articles as excellent or good
Learn more about the work of our research integrity team to safeguard the quality of each article we publish.
Find out more
ORIGINAL RESEARCH article
Front. Phys., 24 March 2025
Sec. Statistical and Computational Physics
Volume 13 - 2025 | https://doi.org/10.3389/fphy.2025.1572999
This study investigates the modification of coal pore structures using composite biomass surfactants and explores its implications for methane adsorption and desorption characteristics. Coal samples from the 13–1 coal seam in Liuzhuang Mine, Huainan, China, are analyzed using low-temperature nitrogen adsorption experiments. The box-counting-based multifractal theory is used to assess coal pore heterogeneity and connectivity before and after surfactant treatment. The results reveal significant improvements in pore structure uniformity and connectivity, providing insights into the relationship between pore characteristics and methane adsorption behavior. This research offers a foundational understanding for optimizing coalbed methane extraction and enhancing environmental sustainability in coal mining operations.
The extraction of coalbed methane (CBM) plays a critical role in addressing global energy demands while concurrently reducing greenhouse gas emissions. Nevertheless, the efficiency of CBM recovery is significantly constrained by the complex pore systems in coal, which are characterized by heterogeneous pore size distributions, low connectivity, and limited permeability [1]. These structural attributes directly impact key processes such as methane adsorption and desorption, as well as diffusion and migration, thereby posing substantial challenges for effective gas extraction [2–4]. While traditional fractal models have been employed to describe the self-similarity inherent in porous materials, they often fail to fully capture the complex and multifactorial heterogeneity native to coal pores, particularly under chemical modification. This gap underscores the necessity for advanced analytical frameworks capable of quantifying structural intricacies and informing targeted enhancements.
Multifractal theory has arisen as a robust analytical approach for examining the hierarchical and non-uniform characteristics of coal pore networks. Unlike conventional monofractal models, multifractal analysis decomposes complex systems into a spectrum of singular metrics, enabling precise quantification of spatial heterogeneity and connectivity [5–7]. By analyzing scaling behaviors across diverse probability distributions, multifractal methods offer critical insights into the evolution of pore structures under external modifications, such as those resulting from the application of surfactants [8–11]. Recent investigations highlight the efficacy of multifractal theory in correlating pore geometry with gas storage and transport capacities [12–14]. However, its application to assess structural changes induced by biomass-based surfactants remains limited, leaving critical knowledge gaps in the development of environmentally sustainable CBM extraction techniques.
Chemical surfactants, predominantly synthetic variants, have been extensively studied for their ability to improve coal wettability and enhance pore connectivity [15, 16]. Nonetheless, their persistence in the environment and associated toxicity raise concerns that conflict with global sustainability objectives. Biomass-derived surfactants, made from renewable sources such as soybean protein isolates, offer a promising alternative due to their amphiphilic properties and high biodegradability [17–20]. For example, soybean protein isolates (SPIs) modified with glucose have demonstrated improved adsorption capabilities on coal surfaces, leading to altered surface hydrophobicity and more uniform pore networks [21, 22]. Despite these advancements, the multifractal response of coal pores to such biomass-based surfactants remains underexplored, impeding the systematic design of green chemical modification strategies. Expanding our understanding of this area is vital for achieving sustainable CBM recovery solutions.
This study addresses these gaps by integrating multifractal theory with low-temperature nitrogen adsorption experiments to evaluate pore structure modifications in anthracite coal treated with composite biomass surfactants. Coal samples from the Liuzhuang Mine were analyzed before and after surfactant treatment, focusing on multifractal spectra (e.g., f(α)∼α, Dq∼q) and connectivity parameters such as the Hurst index. The objectives are threefold: 1) to establish quantitative links between surfactant-induced pore modifications and methane adsorption-desorption behavior, 2) to assess the efficacy of biomass surfactants in reducing structural heterogeneity, and 3) to provide a theoretical foundation for optimizing CBM recovery through sustainable chemical interventions. By bridging multifractal analysis with green chemistry, this work advances both fundamental understanding and practical applications in energy resource management.
The subsequent sections are organized as follows: Section 2 details coal sample preparation and surfactant modification protocols and introduces experimental methods for pore structure characterization. Section 3 presents multifractal analyses and connectivity assessments, while Section 4 discusses implications for CBM extraction and environmental sustainability.
Coal samples were extracted from the 13-1 coal seam situated in Liuzhuang Mine, Huainan, China. This seam exhibits structural variability, ranging from simple to complex formations. Positioned within the central portion of the fourth coal-bearing stratum, the seam’s thickness spans from 0.98 m to 11.07 m, averaging 4.34 m. The primary coal seams exhibit gas content values between 0.21 and 1.47 m3/t, with a mean of 0.65 m3/t. The gas saturation is very low, and the maximum value is only 18%, which belongs to reservoirs with poor permeability.
According to the industrial analysis method of coal (GB/T 212-2008), the collected coal samples are tested for industrial analysis. The coal quality characteristics are shown in Table 1.
In Table 1, Mad (Moisture Content as Determined by Air-Drying, %), refers to the percentage of moisture present in a coal sample after it has been subjected to air drying at ambient conditions. This parameter is crucial for understanding the water content that affects the coal’s heating value and combustion efficiency; Aad (Ash Content as Determined in Air-Dried Condition, %), denotes the percentage of inorganic residue or ash remaining after the combustion of a coal sample that has been air-dried. The ash content is significant as it impacts the coal’s combustibility, ash disposal, and overall energy yield; Vdaf (Volatile Matter Content as Determined in Dry and Ash-Free Condition, %), represents the percentage of volatile matter in the coal, expressed on a dry and ash-free basis. This parameter is essential for assessing the coal’s behavior during combustion and gasification, as volatile matter influences ignition, flame characteristics, and overall energy release; Fcd (Fixed Carbon Density, %), indicates the density of fixed carbon within a coal sample, which is a key indicator of the coal’s carbon content remaining after the volatile matter has been released. The fixed carbon density is vital for determining the coal’s heating capacity and its suitability for various industrial applications.
In this paper, soybean protein isolate was used as the main raw material, and based on alkaline protease hydrolysis reaction, soybean protein isolate hydrolysate was grafted with glucose to obtain modified soybean protein isolate based surfactant (modified SPI). The structure is shown in Figure 1. Using the modified SPI as the main body, the modified solution was prepared with 1wt%KCl base solution and rhamnolipidemic biomass surfactant.
Figure 1 illustrates the basic unit structure of the modified soybean protein isolate (SPI), which serves as the primary component of the biomass surfactant used in this study. This structural representation is crucial for understanding the chemical modifications made to the SPI, such as the grafting of glucose through alkaline protease hydrolysis. These modifications enhance the surfactant’s functionality, enabling it to improve the pore structure of coal by optimizing uniformity and connectivity.
Following the crushing of the original coal sample, coal powder with a particle size range of 60–80 mesh was carefully screened and subsequently immersed in six different composite modification solutions with varying ratios (1:9 to 3:2). The samples were thoroughly soaked, rinsed, and filtered, then dried to achieve a constant weight. Finally, the treated samples were bagged, sealed, and stored for further use.
In compliance with the Chinese national standard for determining the specific surface area of solid materials via the gas adsorption BET method (GB/T 19587-2017), a specific surface area and micropore adsorption analyzer was employed for the low-temperature nitrogen adsorption experiments (refer to Figure 2). The experimental process was conducted at a temperature of 77 K, utilizing approximately 5 g of the sample, with an equilibrium time interval of 10 s.
The Barrett–Joyner–Halenda (BJH) method, which is based on Kelvin capillary condensation theory and assumes cylindrical pore geometry, is a widely used approach for pore size analysis (see [23, 24]). Nonetheless, traditional methods remain inadequate for accurately characterizing micropores or narrow mesopores, particularly when analyzing pore size distributions below 10 nm. These techniques often underestimate pore diameters by approximately 20%–30%. In comparison, Nonlocal Density Functional Theory (NLDFT) has emerged as a more advanced and dependable framework for investigating the pore structures and adsorption properties of porous materials. Unlike conventional approaches, NLDFT integrates both statistical and spatial correlations between adsorbate molecules as well as their interactions with pore walls, achieving molecular-level precision in representing adsorption phenomena. This methodology has been widely employed in the analysis of microporous and mesoporous materials, such as activated carbons, zeolites, and coal matrices, due to its ability to accurately model intricate adsorption isotherms and pore size distributions. By addressing the shortcomings of classical models like Brunauer–Emmett–Teller (BET) and Barrett–Joyner–Halenda (BJH)—which often rely on simplified geometric assumptions—NLDFT offers a more nuanced depiction of pore connectivity, heterogeneity, and molecular-scale interactions. Specifically, NLDFT enables the prediction of adsorption energies and the spatial distribution of adsorbed molecules within porous networks, rendering it an effective tool for evaluating both microporous and mesoporous structures. In this study, the NLDFT methodology is leveraged for the quantitative characterization of mesopore size distributions, establishing a robust framework for elucidating the pore structures of coal and other porous systems.
The multifractal characteristics of pores are studied by the box-counting method. Considering that N2 (77K) adsorption is suitable for mesoporous, that is, the pore size range is 2nm–50 nm. In this section, the aperture range of 2–50 nm is taken as an example. According to the Kelvin equation (see [23–26]),
where
which is called partition function or statistical moment function. Define
characterizes the local singular intensity of the object, and the larger the value of α(q), the higher the regularity of the aperture distribution. The multifractal spectral function of the aperture distribution with respect to α(q) can be expressed as:
The multifractal spectrum, denoted as f (α(q)), characterizes the fractal dimension of subsets sharing an equivalent singularity index. If the aperture distribution exhibits multifractal properties, f (α(q)) will display a unimodal convex function profile. Within this analysis, the q value spans from −10 to 10 with an increment of 1 for each step.
Define the generalized fractal dimension as
Specifically,
Multifractal theory is a theoretical framework for describing systems with complex distributions or structures. It describes the inhomogeneity and complexity of systems through a series of fractal dimensions (see Equations 1-7). In multifractal theory, the generalized fractal dimension D(q) is a key parameter used to characterize the distribution characteristics of the system at different scales, where q is a real number and can take any value. Different values of generalized fractal dimensions correspond to different types of dimensions, of which D0, D1, and D2 are the three most commonly used dimensions, each of which has a specific meaning.
Capacity dimension D0: Also known as the box dimension, it is the most basic fractal dimension used to describe the rough structure of a set. D0 reflects the global distribution characteristics of the entire coal sample pore diameter, regardless of the internal details of the coal sample pore diameter or the uneven distribution. The larger the result, the wider the distribution range of the pore diameter. Information dimension D1: Also known as information dimension or Shannon dimension, is defined by the concept of Shannon entropy. D1 reveals the detail structure and non-uniformity of coal sample pore size distribution, which is a measure of the complexity of coal sample pore size. The larger the result, the more concentrated the pore size distribution. Correlation dimension D2: Also known as correlation dimension or correlation dimension, it is defined by considering the correlation between the points within the pore diameter of the coal sample. D2 can be regarded as a measure to describe the correlation between the internal structure of coal sample pore size, reflecting the density of some areas in coal sample pore size and the uniformity of distribution. The larger the result, the more uniform the spacing of pore size distribution. D0, D1 and D2 describe the fractal characteristics of coal sample pore size from different perspectives, including its global structure, information complexity and internal correlation, which together form the basis of multifractal analysis, and can be used to comprehensively reveal the distribution characteristics and internal laws of complex systems. D (-q)-D(q) describes the difference in fractal dimensions between sparse and dense regions of the system. This difference reflects the heterogeneity and complexity of the internal structure of the system. When D (-q)-D(q) > 0, it indicates that the fractal dimension of the sparse region in the system is higher than that of the dense region, which means that the non-uniformity of the system is strong, and the sparse region contributes more to the fractal characteristics of the system.
Taking mixed 3:7 as an example, let the total interval I = [0.38, 0.94]. Using the same dichotomy as [12, 27], the interval I of length L is divided into
In the multifractal analysis of aperture distribution, the first step is to verify whether the object of study exhibits multifractal properties at the spatial scale under investigation. The existence of a linear relationship between U (q,ε) and lgε is a key prerequisite to confirm that an object has multifractal characteristics. The relationship between the generalized fractal dimension U (q,ε) and log-aperture lgε (see Figure 3) of the coal sample modified with the mixed biomass surfactant shows a significant linear correlation. The results confirm the multifractal properties revealed by the pore size distribution data of N2 (77K) adsorption experiments at low temperature.
Figure 3. Linear fitting relationship between partition function and scale (taking LZ coal sample 3:7 as example).
The generalized dimension spectrum D(q)∼q curves of coal samples treated with biomass surfactants are shown in Figure 4. Combined with the inverse “S” shape of the curve, the pore size distribution of coal samples is characterized by non-uniform distribution. The larger the range of D(q), the greater the heterogeneity of the fractal structure. It can also be seen from the figure that after the coal sample is modified, the generalized dimension spectrum curve becomes more and more gentle, that is, the volatility of the curve is weakened, and the range of the curve (that is, the numerical range covered by the curve) is also gradually shrinking, which indicates that the non-uniformity of high-rank coal is smaller. In other words, the internal structural complexity of the modified coal is reduced, and it has weaker inhomogeneity.
Figure 4. Generalized dimensional spectral D (q)∼q curve of pore size distribution of coal samples (taking LZ coal sample 3:7 as example).
The results of D0, D1, D2 and D-10-D10 in the generalized fractal dimensions of coal samples are shown in Table 2. For raw coal and composite (3:7) coal samples, their D0 values are the same (0.7925), which indicates that the degree of pore space filling of the two is consistent in fractal dimension. However, on D1 and D2 values, the coal treated with the mixed biomass surfactant is 0.7645 and 0.7382, respectively, which are higher than that of raw coal (0.7584 and 0.7264). This means that compared with raw coal, the uniformity of pore distribution and the degree of correlation between pores are improved. The values of D-10-D10 reflect the diversity and complexity of pore structure. The D-10-D10 value of raw coal is 0.4084, while that of coal treated with mixed biomass surfactant is 0.3746, indicating that the pore structure of raw coal has higher diversity and complexity than that of coal treated with mixed biomass surfactant. Although there is no difference in the degree of pore space filling between raw coal and the coal treated with mixed biomass surfactant, the coal treated with mixed biomass surfactant performs better in the uniformity of pore distribution and the degree of pore correlation. These differences may have an impact on the physical and chemical properties of coal samples, which in turn affect their performance in practical applications.
In multifractal analysis, △α and △f are two important parameters, which are used to describe the heterogeneity and complexity of coal sample pores (see [12]). These two parameters are derived from the analysis of multifractal spectrum, which is extracted from coal sample pores through a series of operations to represent the fractal dimension of coal sample pores at different scales. The following are the meanings of these two parameters.
In the multifractal spectrum, the minimum and maximum values of α(q) represent the local shape characteristics of the sparsest and densest parts of the coal sample pores, respectively. Thus, △α = αmax-αmin describes the difference in fractal properties between the densest and the sparsest parts of the coal sample pores. The larger △α value indicates that the pore space distribution of coal sample is non-uniform.
In the multifractal spectrum, f(α) describes the fractal dimension of coal sample pores with a specific local Holder index. It can be understood as the richness or complexity of coal sample pores under this fractal property. Something like △f = f (αmax) - f (αmin) describes the difference in fractal dimension between the densest and the sparsest parts. However, because of the way f(α) is defined and calculated, △f is often used to represent the width of the multifractal spectrum, that is, the shape and range of the spectrum, and is not directly used to compare the fractal dimension of the densest and sparsest parts.
The multifractal spectrum curve illustrating the pore distribution of the coal sample treated with the mixed biomass surfactant, compared to that of raw coal, is presented in Figure 5. This confirms the presence of multifractal characteristics in the pore size distribution. Correspondingly, the multifractal singular spectrum parameters of the coal samples were computed, with the results summarized in Table 3.
Figure 5. Multifractal spectral curve of pore size distribution of coal samples (taking LZ coal sample 3:7 as example).
As depicted in Figure 5, the spectrum curve of pore distribution, comparing treated coal samples to raw coal following surfactant modification, exhibits a unimodal convex profile. This finding demonstrates that the pore size distribution, determined via the low-temperature N2 adsorption method at 77 K, possesses multifractal features. Table 3 provides the detailed results of the calculated multifractal singular spectrum parameters for the coal samples.
From the data in Table 3, it is evident that the treated coal exhibits a smaller △α value and a larger △f value compared to raw coal. These observations suggest that biomass surfactant modification enhances the local density of the pore size distribution, leading to a more uniform fractal behavior across different scales relative to untreated coal. Such changes in pore structure likely exert a favorable influence on the physical and chemical properties of the coal samples, with potential implications for energy applications and environmental sustainability.
In the field of coal science, the Hurst index plays a significant role in characterizing the pore structure of materials such as coal seams, with a particular emphasis on their connectivity (see [28–30]). Ranging from 0 to 1, the Hurst index reflects the degree of long-range dependence and structural self-similarity, with values closer to 1 indicating stronger self-similarity and persistence in the material’s structural patterns. As a fundamental tool in fractal and multifractal analysis, the Hurst index not only aids in assessing pore connectivity but also provides insights into the anisotropic and heterogeneous properties of coal seams. Building on the multifractal framework, the generalized Hurst index extends this concept to better capture the complex, scale-dependent nature of pore connectivity in coal. Represented as H(q), the generalized Hurst index is determined using the scale index τ(q) and the multifractal spectrum f(α). It is expressed as follows:
In Equation 8, H(q) signifies the generalized Hurst index, which describes the connectivity of apertures, while τ(q) denotes the scale index. Notably, when q = 2, H(q) reduces to the classical Hurst index, typically ranging from 0.5 to 1. A higher Hurst index indicates stronger aperture connectivity, whereas a lower value reflects weaker connectivity. Refer to Table 4 below for further details.
It can be found from the table that the Hurst index of the coal treated with the mixed biomass surfactant is higher than that of the raw coal, indicating that the pore connectivity is improved after the mixed modification.
The study systematically investigates the multifractal characteristics of coal pore structures under the influence of mixed biomass surfactants, providing significant insights into the optimization of coal pore connectivity and uniformity. The findings reveal that the pore size distribution of coal samples, as determined by low-temperature N2 adsorption experiments, exhibits distinct multifractal properties. The application of mixed biomass surfactants leads to a notable enhancement in the uniformity of the pore structure, reducing internal heterogeneity and improving the overall connectivity of the pore network. This is evidenced by the increase in the Hurst index of treated coal samples compared to raw coal, indicating improved self-similarity and a more consistent distribution pattern across larger spatial scales.
The generalized dimension spectrum (Dq∼q) and multifractal singular spectrum (f(α)∼α) analyses further demonstrate the reduction in structural complexity and heterogeneity of coal pores after surfactant treatment. A reduced slope and a narrower range in the generalized dimension spectrum suggest that the treated coal exhibits a more homogeneous pore distribution. Similarly, the decrease in Δα and Δf values in the multifractal singular spectrum analysis confirms the reduction in pore heterogeneity and complexity. These structural improvements are critical for enhancing methane desorption, diffusion, and migration, thereby addressing challenges in coalbed methane (CBM) recovery.
However, the study has certain limitations. The scalability of biomass surfactant applications in industrial settings remains uncertain, as the study was conducted under controlled laboratory conditions. Future research should evaluate the economic feasibility and operational challenges of large-scale implementation. Additionally, the study focuses on a specific biomass surfactant formulation (such as subdivide the mixing ratios) and coal seam. Exploring alternative biomass surfactant formulations and testing their effects under varying coal seam conditions could provide broader insights into their applicability and effectiveness. These directions will further enhance the understanding of biomass surfactants as sustainable solutions for optimizing coalbed methane recovery and improving energy resource management.
The raw data supporting the conclusions of this article will be made available by the authors, without undue reservation.
LY: Conceptualization, Methodology, Resources, Software, Validation, Visualization, Writing–original draft, Writing–review and editing. YY: Writing–original draft.
The author(s) declare that financial support was received for the research and/or publication of this article. This paper was supported by the Key Project of the Natural Science Foundation of Universities in Anhui Province (KJ2021A1389), Anhui Province Outstanding Young Talents Support Program (No. gxyg2021270), Anhui University Collaborative Innovation Project GXXT-2020-057, and Key Project of University Humanities and Social Science in Anhui Province (No. SK2019A0102).
The authors declare that the research was conducted in the absence of any commercial or financial relationships that could be construed as a potential conflict of interest.
The author(s) declare that no Generative AI was used in the creation of this manuscript.
All claims expressed in this article are solely those of the authors and do not necessarily represent those of their affiliated organizations, or those of the publisher, the editors and the reviewers. Any product that may be evaluated in this article, or claim that may be made by its manufacturer, is not guaranteed or endorsed by the publisher.
1. Xu C, Nie W, Liu Z, Peng H, Yang S, Liu Q. Multi-factor numerical simulation study on spray dust suppression device in coal mining process. Energy (2019) 182:544–58. doi:10.1016/j.energy.2019.05.201
2. Bardestani R, Patience GS, Kaliaguine S. Experimental methods in chemical engineering: specific surface area and pore size distribution measurements—BET, BJH, and DFT. Can J Chem Eng (2019) 97(11):2781–91. doi:10.1002/cjce.23632
3. Ji B, Jiang B, Yuan L, Yu C, Zhou G, Zhao Y, et al. Experimental and molecular dynamics simulation study on the influence of SDS and JFC composite ratios on bituminous coal wettability. Process Saf Environ Prot (2023) 174:473–84. doi:10.1016/j.psep.2023.03.081
4. Nikolova C, Gutierrez T. Biosurfactants and their applications in the oil and gas industry: current state of knowledge and future perspectives. Front Bioeng Biotechnol (2021) 9:626639. doi:10.3389/fbioe.2021.626639
5. Cai F, Zhang Q, Yang L. Fractal characteristics and microstructure of coal with impact of starch-polymerized aluminum sulfate fracturing fluids. Fractal and Fractional (2024) 8(4):228. doi:10.3390/fractalfract8040228
6. Yang L, Cai F, Yuan Y. Fractal dimension and nuclear magnetic resonance characteristics of surfactants for coal gas desorption. Fractal and Fractional (2023) 7(3):217. doi:10.3390/fractalfract7030217
7. Zhao Y, Lin B, Liu T, Zheng Y, Sun Y, Zhang G, et al. Multifractal analysis of coal pore structure based on NMR experiment: a new method for predicting T2 cutoff value. Fuel (2021) 283:119338. doi:10.1016/j.fuel.2020.119338
8. Miao Z, Qiu Z, Guofu L, Xuehai F, Chaochao D, Huihu L, et al. Fluid distribution and pore structure multifractal characteristics analysis of coal measure mudstone. J Nat Gas Sci Eng (2021) 88:103810. doi:10.1016/j.jngse.2021.103810
9. Sun Y, Zhai C, Zhao Y, Xu J, Cong Y, Zheng Y, et al. Multifractal analysis and neural network prediction of pore structures in coal reservoirs based on NMR T2 spectra. Energy and Fuels (2021) 35(14):11306–18. doi:10.1021/acs.energyfuels.1c01409
10. Li T, Li HL, Zhang L, Zheng S. Complete synchronization of discrete-time variable-order fractional neural networks with time delays. Chin J Phys (2024) 91:883–94. doi:10.1016/j.cjph.2024.08.022
11. Johansyah MD, Sambas A, Farman M, Vaidyanathan S, Zheng S, Foster B, et al. Global mittag-leffler attractive sets, boundedness, and finite-time stabilization in novel chaotic 4d supply chain models with fractional order form. Fractal and Fractional (2024) 8(8):462. doi:10.3390/fractalfract8080462
12. Zheng S, Yao Y, Liu D, Cai Y, Liu Y, Li X. Nuclear magnetic resonance T2 cutoffs of coals: a novel method by multifractal analysis theory. Fuel (2019) 241:715–24. doi:10.1016/j.fuel.2018.12.044
13. Wang D, Hu H, Wang T, Tang T, Li W, Zhu G, et al. Difference between of coal and shale pore structural characters based on gas adsorption experiment and multifractal analysis. Fuel (2024) 371:132044. doi:10.1016/j.fuel.2024.132044
14. Zhang S, Liu H, Wu C, Jin Z. Influence of particle size on pore structure and multifractal characteristics in coal using low-pressure gas adsorption. J Pet Sci Eng (2022) 212:110273. doi:10.1016/j.petrol.2022.110273
15. Gan J, Wang D, Xiao Z, Wang YN, Zhang K, Zhu X, et al. Experimental and molecular dynamics investigations of the effects of ionic surfactants on the wettability of low-rank coal. Energy (2023) 271:127012. doi:10.1016/j.energy.2023.127012
16. Niu W, Nie W, Yuan M, Bao Q, Zhou W, Yan J, et al. Study of the microscopic mechanism of lauryl glucoside wetting coal dust: environmental pollution prevention and control. J Hazard Mater (2021) 412:125223. doi:10.1016/j.jhazmat.2021.125223
17. Chen Y, Xu G, Albijanic B. Evaluation of SDBS surfactant on coal wetting performance with static methods: preliminary laboratory tests. Energy Sourc A: Recovery, Utilization Environ Effects (2017) 39(23):2140–50. doi:10.1080/15567036.2017.1403503
18. Dou J, Liu J, Wang Y, Zhi L, Shen J, Wang G. Surface activity, wetting, and aggregation of a perfluoropolyether quaternary ammonium salt surfactant with a hydroxyethyl group. Molecules (2023) 28(20):7151. doi:10.3390/molecules28207151
19. Ni G, Sun Q, Xun M, Wang H, Xu Y, Cheng W, et al. Effect of NaCl-sds compound solution on the wettability and functional groups of coal. Fuel (2019) 257:116077. doi:10.1016/j.fuel.2019.116077
20. Tian Q, Nie W, Bao Q, Niu W, Li R, Zhang X, et al. Research on coal dust pollution prevention and control based on co-solvent association: macro-micro experiments and molecular dynamics simulations. Appl Surf Sci (2024) 651:159289. doi:10.1016/j.apsusc.2024.159289
21. Niu W, Nie W, Bao Q, Tian Q, Liu F, Li R, et al. Interactions between fluorocarbon surfactant and coal dust at macro, meso and molecular scales and their applications in coal dust control. Chem Eng J (2023) 475:146059. doi:10.1016/j.cej.2023.146059
22. Xu F, Wang S, Kong R, Wang C. Synergistic effects of dodecane-castor oil acid mixture on the flotation responses of low-rank coal: a combined simulation and experimental study. Int J Mining Sci Technology (2023) 33(5):649–58. doi:10.1016/j.ijmst.2022.11.010
23. Wang Y, Shardt N, Lu C, Li H, Elliott JA, Jin Z. Validity of the Kelvin equation and the equation-of-state-with-capillary-pressure model for the phase behavior of a pure component under nanoconfinement. Chem Eng Sci (2020) 226:115839. doi:10.1016/j.ces.2020.115839
24. Stahlfeld KW, Belmont EL. BET and Kelvin analyses by thermogravimetric desorption. Langmuir (2023) 39(25):8814–23. doi:10.1021/acs.langmuir.3c00854
25. Shi K, Santiso EE, Gubbins KE. Current advances in characterization of nano-porous materials: pore size distribution and surface area. In: JC Moreno-Piraján, L Giraldo-Gutierrez, and F Gómez-Granados, editors. Porous materials. Engineering materials. Cham: Springer (2021).
26. Ullah R, Siraj M, Zarshan F, Ahsan Abbasi B, Yaseen T, Abdul W, et al. A comprehensive overview of fabrication of biogenic multifunctional metal/metal oxide nanoparticles and applications. Rev Inorg Chem (2024). doi:10.1515/revic-2024-0031
27. Zhang M, Liu Q, Wang X, Dong Z. Multiple fractal characterization of medium-high rank coal integrating mercury intrusion porosimetry, N2 and CO2 adsorption experiments. J China coal Soc (2024) 49(5):2394–404. doi:10.13225/j.cnki.jccs.2023.0433
28. Prajapati R, Kumar R, Singh UK. Assessment of reservoir heterogeneities and hydrocarbon potential zones using wavelet-based fractal and multifractal analysis of geophysical logs of Cambay basin, India. Mar Pet Geology (2024) 160:106633. doi:10.1016/j.marpetgeo.2023.106633
29. Chen S, Liu D, Wang Z, Xu L, Li Q, Ren L, et al. Predicting T2 cutoffs from nmr logging based on multifractal theory: a case study of a shale reservoir in the songliao basin, China. Energy and Fuels (2025) 39:4292–303. doi:10.1021/acs.energyfuels.5c00022
30. Kumar C, Shrivastav M, Escrig J, Ţălu Ş, Yadav RP, Guzman F. Unravelling the impact of surface roughness and cationic Ni2+ vacancies to Ni3+ ions ratio on optical properties of NiOx thin film: insights fractal and multifractal dimension. Ceramics Int Online (2025). doi:10.1016/j.ceramint.2025.01.362
Keywords: multifractal, coal, hurst index, biomass surfactant, aperture distribution
Citation: Yang L and Yuan Y (2025) Multifractal analysis and modification of coal pore structures with impact of clean compound biomass surfactants. Front. Phys. 13:1572999. doi: 10.3389/fphy.2025.1572999
Received: 08 February 2025; Accepted: 05 March 2025;
Published: 24 March 2025.
Edited by:
Song Zheng, Zhejiang University of Finance and Economics, ChinaCopyright © 2025 Yang and Yuan. This is an open-access article distributed under the terms of the Creative Commons Attribution License (CC BY). The use, distribution or reproduction in other forums is permitted, provided the original author(s) and the copyright owner(s) are credited and that the original publication in this journal is cited, in accordance with accepted academic practice. No use, distribution or reproduction is permitted which does not comply with these terms.
*Correspondence: Yuan Yuan, eXVhbnk3N0B1c3RjLmVkdS5jbg==
Disclaimer: All claims expressed in this article are solely those of the authors and do not necessarily represent those of their affiliated organizations, or those of the publisher, the editors and the reviewers. Any product that may be evaluated in this article or claim that may be made by its manufacturer is not guaranteed or endorsed by the publisher.
Research integrity at Frontiers
Learn more about the work of our research integrity team to safeguard the quality of each article we publish.