- 1Institute of Imaging and Computer Vision (LfB), RWTH Aachen University, Aachen, Germany
- 2Department of Physics of Molecular Imaging Systems (PMI), Institute for Experimental Molecular Imaging, RWTH Aachen University, Aachen, Germany
- 3Institute of High Energy Physics (HEPHY), Austrian Academy of Sciences, Vienna, Austria
- 4Hyperion Hybrid Imaging Systems GmbH, Aachen, Germany
- 5Fraunhofer Institute for Digital Medicine MEVIS, Aachen, Germany
- 6Physics Institute III B, RWTH Aachen University, Aachen, Germany
PET is a functional imaging method that can visualize metabolic processes and relies on the coincidence detection of emitted annihilation quanta. From the signals recorded by coincident detectors, TOF information can be derived, usually represented as the difference in detection timestamps. Incorporating the TOF information into the reconstruction can enhance the image’s SNR. Typically, PET detectors are assessed based on the coincidence time resolution (CTR) they can achieve. However, the detection process is affected by factors that degrade the timing performance of PET detectors. Research on timing calibrations develops and evaluates concepts aimed at mitigating these degradations to restore the unaffected timing information. While many calibration methods rely on analytical approaches, machine learning techniques have recently gained interest due to their flexibility. We developed a residual physics-based calibration approach, which combines prior domain knowledge with the flexibility and power of machine learning models. This concept revolves around an initial analytical calibration step addressing first-order skews. In the subsequent step, any deviation from a defined expectation is regarded as a residual effect, which we leverage to train machine learning models to eliminate higher-order skews. The main advantage of this idea is that the experimenter can guide the learning process through the definition of the timing residuals. In earlier studies, we developed models that directly predicted the expected time difference, which offered corrections only implicitly (implicit correction models). In this study, we introduce a new definition for timing residuals, enabling us to train models that directly predict correction values (explicit correction models). We demonstrate that the explicit correction approach allows for a massive simplification of the data acquisition procedure, offers exceptionally high linearity, and provides corrections able to improve the timing performance from (371
1 Introduction
The introduction of precise time-of-flight (TOF) information in positron emission tomography (PET) leads to a significant improvement in the signal-to-noise ratio (SNR) of the reconstructed images, which could aid physicians in diagnosis [1, 2]. For this reason, in recent years, there has been increased research into various approaches that have the potential to further improve TOF-PET, with the ultimate goal of eventually achieving a timing resolution in the order of 10 ps [3].
The PET data acquisition begins with the detection of the emitted
Besides previously mentioned research lines, a considerable number of studies are conducted on the development and improvement of calibration techniques aiming to minimize any deteriorating effects impacting the TOF measurement by estimating unaffected timestamps or providing corrections to them. Mathematically (see Equation 1), one can describe a timestamp
with the offset value being a composition of different deteriorating sub-offset
Here,
While in former times, primarily analytical calibration approaches were studied, artificial intelligence (AI) methods gained interest in recent years, so that machine learning approaches are also an active area of research. Classical PET timing calibration methods in the literature rely on solving matrix equations [25–27] or use timing alignment probes [28, 29]. Also, data-driven approaches without requiring specialized data acquisition setups have been investigated, e.g., using consistency conditions [30–33] or detector-intrinsic characteristics [34, 35]. Other approaches use statistical modeling under the premise of a maximum-likelihood approach [36, 37].
Many machine learning-based timing calibrations utilize supervised learning and, therefore, generate labeled data by measuring radiation sources at multiple positions [38–42] or at a single position [43]. Nearly all of the existing approaches work with the signal waveforms. Although this data represents a rich source of information, it often requires dedicated measurement hardware capable of high sampling rates, e.g., oscilloscopes, that might be challenging to implement in a full scanner setting.
In recent studies, we developed and demonstrated the functionality of a machine learning-based timing calibration for clinically relevant detectors (coupled to digital silicon photomultipliers (SiPMs) [44, 45] and analog SiPMs [46]) without the need of waveform information. Instead, we utilized detector blocks coupled to matrices of SiPMs, as is done in pre-clinical and clinical settings. Furthermore, we proposed to follow a residual physics-based calibration concept, which separates the calibration effort into two parts.
In the first part, an analytical calibration procedure is conducted, which addresses so-called skews of first order that do not change during measurement and are similar for each event. After correction for those skews, each deviation from an expectation can be interpreted as a residual effect caused by higher-order skews that might change on an event basis. To address these higher-order skews in the second part, we endeavor to use machine learning techniques since they offer high flexibility and are suitable for finding characteristic patterns in the calibration data. The strength of the residual physics concept is the underlying idea that the experimenter can incorporate prior domain knowledge in the way the residual effects are defined.
When applying machine learning techniques in physical problems, one should ensure that the trained models produce results that are in alignment with physical laws. Therefore, we proposed in prior works, to use a three-fold evaluation scheme. In the first instance, models are assessed regarding their mean absolute error (MAE) performance. How well the models are with physics is tested in the second instance. Finally, models that passed the first two evaluation levels are tested in the third instance regarding the improvement in coincidence time resolution (CTR).
In our proof-of-concept study [44], we used a similar label generation like Berg and Cherry [38], which demands the collection of multiple source positions between two detectors in order to label the data for supervised training of the model. Successfully trained regression models are able to predict the expected time difference for a given input sample, e.g., consisting of information about the measured time difference, the detected timestamps, the detected energy signals, and the estimated
Although this procedure demonstrated that significant improvements in CTR are possible, the acquisition of the labeled data was time-consuming considering future applications in a fully equipped PET scanner. In a follow-up work [46], we demonstrated that the acquisition time can be significantly reduced without losing the calibration quality making the approach more practical. However, the study also revealed a strong dependence of the trained regression models on the used training step width given as the distance between subsequent source positions. As soon as this stepping distance becomes too large, the regression model showed on finer sampled test data a form of collapse towards a classification model for positions present in the training data set. This resulted in a worse prediction quality for radiation sources located at positions being not present in the training data. Even though the overall prediction quality of the collapsing models was in an acceptable range regarding the MAE, it also led to a substantial loss of linearity. The linearity property of a calibration model, namely, that a source shift in the spatial domain translates linearly to shift of the time difference distribution in the time domain, is especially for TOF-PET one of the most important attributes, since it ensures a correct interpretation of the TOF information. The precise value of the maximum training step width at which the model remains functional might depend on the spatial sampling of the training dataset and on the timing resolution that can be achieved with the detectors being calibrated. While the precise derivation of the dependence on the measurement parameters is out of the scope of this work, one can safely assume that a training step width being large compared to either the testing step width or/and the timing resolution will likely result in a model collapse. The implication of this observation leads to the conclusion that the smaller the CTR value and/or testing step width is, the smaller the training step width used has to be, resulting potentially in an elaborate data acquisition.
In this work, we provide a new definition of the timing residuals (oriented on the work of Onishi et al. [43]), which allows us to train TOF-correction models explicitly providing correction values instead of expected time differences.
In the first part, we compare the novel residual formulation (explicit corrections) with the already established one (implicit corrections) regarding a three-fold evaluation scheme. The analyses use real measurement data and demonstrate that by using the explicit correction approach, the strong dependence on the step width is removed, the biased prediction distributions are suppressed, and the linearity property is preserved. Considering a typical PET scanner geometry, we will refer to this study as the transaxial performance study.
In the second part, we analyze how the in-plane distribution of sources for a given location between the detectors affects the calibration quality, minding a later in-system application with, e.g., dedicated phantoms or a point source located at a single position between the detectors. In the following, this study is called the in-plane distribution study.
2 Materials
2.1 PET detectors
Two clinically relevant PET detectors of identical design are used for the experiments. The scintillator topology is given by 4 × 4 LYSO:Ce,Ca crystals manufactured by Taiwan Applied Crystals (TAC), which have shown promising performance in previous studies [5]. ESR-OCA-ESR sheets with a thickness of 155 μm cover the lateral sides of the outer crystals. Each of the 4 × 4 crystal elements encloses a volume of 3.8 mm × 3.8 mm x 20 mm, features polished top and bottom faces with depolished lateral faces [47, 48], and allows for light-sharing. The crystals are coupled to a 4 × 4 array of Broadcom NUV-MT SiPMs (AFBR-S4N44P164M) having a pitch of 3.8 mm and 40 μm SPAD size. Each SiPM is coupled to one channel of the TOFPET2 ASIC (version 2c) [49, 50]. A timestamp in picoseconds and an energy value in arbitrary units is reported if an SiPM is triggered and defines a so-called hit. The trigger and validation settings can be found in Table A4. The overvoltage is set to 7 V to balance good timing performance [5] with a reasonable noise floor [5, 21].
2.2 Experimental setup and settings
The measurement setup is located in a light-protected climate chamber, which is controlled at a temperature of 16°C. The sensor tiles are mounted at a distance of 565 mm. In between the detectors, a 22Na point source with an active diameter of 0.5 mm is used. The source holder is connected to a custom-manufactured translation stage system, allowing movement along all three spatial axes with a precision of <1 μm. The activity of the source was given to 1.4 MBq. The ASIC board temperatures are kept constant while operation and are measured to be (33.9
3 Methods
3.1 Defining timing residuals: implicit and explicit corrections
Labeling the acquired measurement data is essential to make it applicable for supervised learning algorithms. The labels represent a type of ground truth since they guide the training procedure of machine learning models. However, for PET timing calibrations, the underlying ground truth is not accessible since the time skews can vary on an event basis, and the only information available to the experimenter is the measured time difference given as the difference between the timestamps reported by two coincident detectors. Nevertheless, it is possible to obtain a certain level of ground truth by connecting the spatial location of a radiation source with the expected time difference, assuming that no systematic skews are present. By shifting the radiator to a different spatial location
with
Models trained with this definition of the timing residuals correct the measured timestamps
To remove the need for measuring multiple source positions along the
with
Furthermore, this explicit correction formulation (see Equations 6, 7) introduces a translational symmetry into the labeling process. Therefore, it does not pose any restriction on the number of sources along the
When comparing both label definitions (see Figure 1), one can see that the label distribution is discrete for the implicit approach. Precisely, the number of unique labels (unique expected time differences) is equal to the number of used source positions, which might result in a sparse coverage of the label domain. Contrary to this, the explicit correction approach generates a continuous spectrum of labels, which is independent from the number of measured source positions due to translational symmetry of the formulation resulting in a high coverage of the label domain. The various label approaches also lead to differences in terms of label balance or imbalance. In the implicit approach, it is very easy to generate a high balance of labels by using the same measurement time per source position. This promotes that all labels can be trained equally well. In comparison, the explicit approach leads to a label imbalance independent of the selected measurement time. This is because the labels are based, among other things, on the measured time difference spectrum, which is Gaussian-like distributed. As a result, corrections with a large magnitude are less often represented in the training data set than corrections with a smaller magnitude.
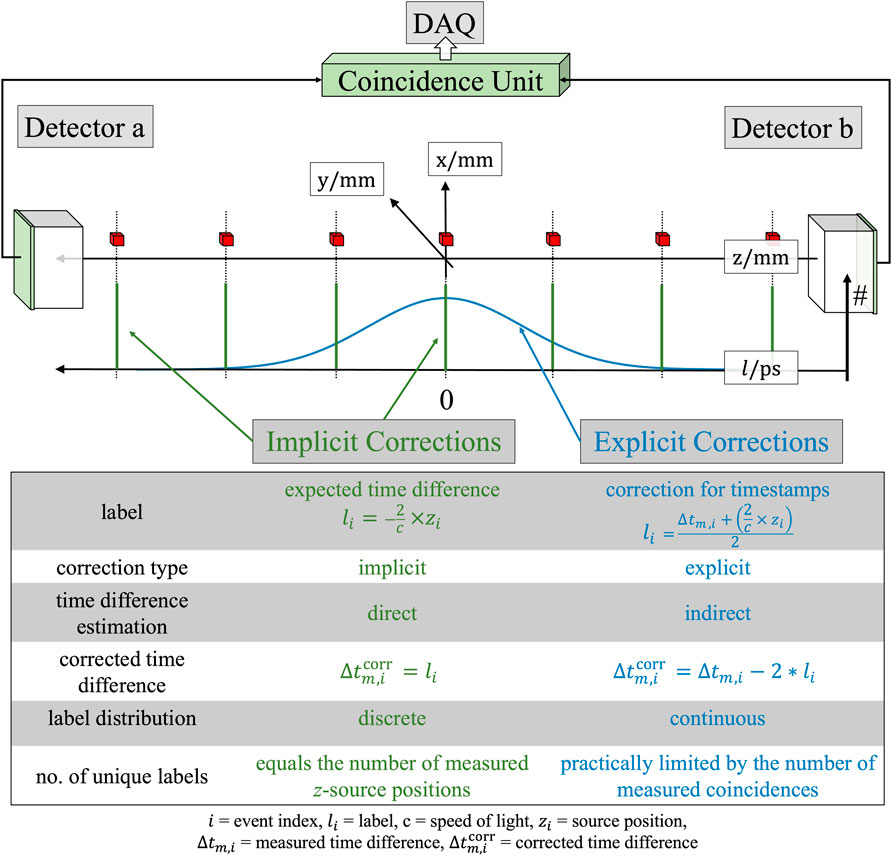
Figure 1. Visualization of the label distributions of the implicit (green) and explicit (blue) correction approach. The radiation source positions are displayed as red cubes.
3.2 Evaluation metrics
In recent works, we proposed to use a three-folded evaluation scheme [45, 46, 51] for any kind of machine learning-based TOF correction or calibration. The scheme consists of a data scientific, physics-based and PET-based evaluation approach. Only models that pass the first two evaluation criteria are evaluated in a subsequent step regarding their application in PET.
3.2.1 Data scientific evaluation
The data scientific evaluation approach assesses the models based on typical metrics known from machine learning. In this work, we calculate the MAE for all distinct
with
The weighted mean MAE (see Equation 10) takes the label distribution into account by weighting the MAE with the number of occurrences
We perform the data scientific evaluation without posing any restrictions on the estimated energy of the input data.
3.2.2 Physics-based evaluation
The next evaluation part considers aspects from physics, which are the linearity and a so-called
where we assume that the mean
Equation 11 closely resembles the fundamental Equation 3, with both describing a linear dependence between the expected time difference and the source position. We evaluate qualitatively if this assumption is fulfilled by the predictions of our models using reduced
with
As a quantitative measure, we use the number of
In order to suppress any deteriorating effects coming from time walk, events are selected to be in an energy window from 430 keV to 590 keV for the physics-based evaluation. A model passes the physics-based evaluation, if the
3.2.3 PET-based evaluation
If the data scientific and physics-based evaluations are positive, finally, the performance of the model is tested assessing the achievable CTR. For this, data is fed into the models, and the resulting predictions are filled into a histogram. The full width at half maximum (FWHM) of this distribution is estimated by fitting a Gaussian function assuming Poissonian uncertainties on the time differences. We perform this analysis for test data from the iso-center (describing the center of the setup
3.3 Model architecture
We use gradient-boosted decision trees (GBDTs) as model architecture for the second part of the residual physics-based timing calibration. GBDTs are a classical machine learning approach with the advantage that they can handle missing values and allow the implementation in a field programmable gate array (FPGA) [53]. This makes it especially suitable for the application in PET scanners minding edge-AI approaches [54, 55]. The model is based on an ensemble of binary decision trees, which uses boosting as a learning procedure. Each tree that is added to the ensemble during the training process attempts to minimize the errors of the predecessor model. In this study, we worked with the implementation of XGBoost [56], which has proven it’s predictive power [57] in our prior studies [44, 46].
Like in many other machine learning algorithms, several hyperparameters can be set before training a model. The maximum tree depth
3.4 Study design
Several datasets were recorded in order to realize the different study designs for evaluating the implicit and explicit correction approach. In the following text, we will often refer to the coordinate system defined in Figure 1.
3.4.1 Transaxial performance study
This study aims to compare both calibration approaches regarding their performance along the axis connecting both detector stacks. To acquire data for training, validation and testing 35 positions along the
From the acquired dataset, 2.64
In addition to the experiment, where each
For both kinds of training approaches (implicit and explicit), the same amount of training and validation samples are used, and a big parameter space of the maximum tree depth
All models are evaluated regarding their MAE performance and linearity behavior. In prior studies [44, 46], we demonstrated that this results in edge effects leading to non-Gaussian distribution (see Figure 3). For this reason, the linearity analysis of the implicit correction models is performed for a large central region (−100 mm to 100 mm), while the explicit correction approach allows an analysis over the complete
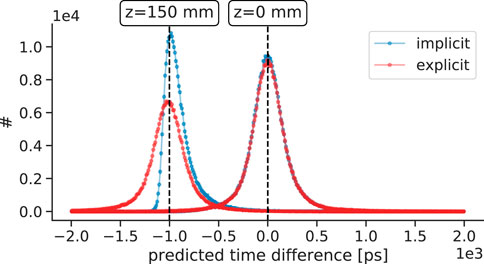
Figure 3. Examples of the prediction distribution of implicit and explicit correction models. While the explicit correction model preserves the Gaussian time difference shape, the implicit correction model is strongly affected by edge effects leading to non-Gaussian distributions. In this example, the implicit model
3.4.2 In-plane distribution study
This study aims to evaluate how the in-plane source distribution affects the performance of explicit correction models. Data is acquired at only one
which also can be seen Figure 4. Identical to the transaxial performance study, five explicit correction models were trained for each dataset with the maximum tree depth
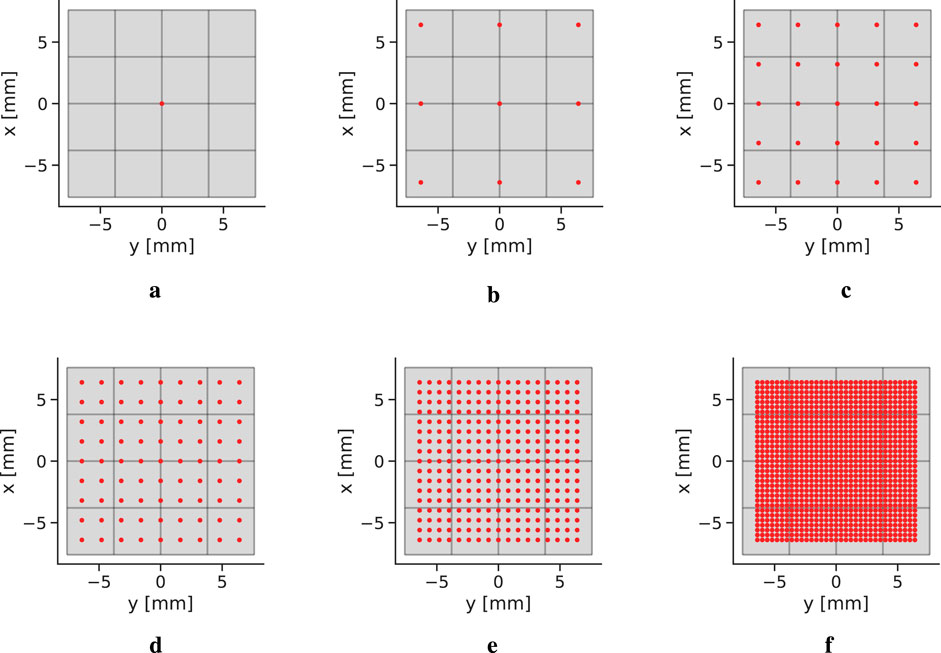
Figure 4. In-plane source distributions (see Equation 15). The red dots represent the position of a source, while the gray background displays the detector. (a) 1 × 1 grid. (b) 3 × 3 grid. (c) 5 × 5 grid. (d) 9 × 9 grid. (e) 17 × 17 grid. (f) 33 × 33 grid.
3.5 Data pre-processing
Hits corresponding to a single
To each cluster, a
Following our proposed residual physics-based calibration scheme, a conventional time skew calibration [59] is conducted in order to remove the major skews of the first order.
3.6 Input features
The input features for both models are derived from the detection information of coincident clusters and can be separated into a set with information from detector
The
While these quantities represent the input for the explicit correction models, the implicit correction models use one additional feature: the measured time difference. Based on a prior statistical analysis on how many triggered SiPMs are contained in a cluster,
4 Results
4.1 Transaxial performance study
4.1.1 Data scientific evaluation
Prior to assessing the models with the three-fold evaluation scheme, we checked that the models were trained successfully by analyzing the training and validation curves. All models showed a smooth training progression and were fully trained out.
The MAE progression of the explicit and implicit correction models for different training step width is displayed in Figures 5–7. For the models trained on data with a step width of 10 mm, both correction models show a smooth MAE progression without any outliers. While most of the MAE values of the implicit correction models are located in a region between 100 ps and 200 ps, the MAE rises towards the edges of the testing data. The labels of the training dataset for implicit correction models are, in good approximation, uniformly distributed. The MAE progression of the explicit correction models resembles a U-form, with the lowest MAE of sub-100ps being achieved at labels of small magnitude. When moving to higher label magnitudes, and therefore, also to higher correction magnitudes, the MAE strongly rises. The explicit label distribution follows, in good approximation, a Gaussian shape with non-Gaussian tails.
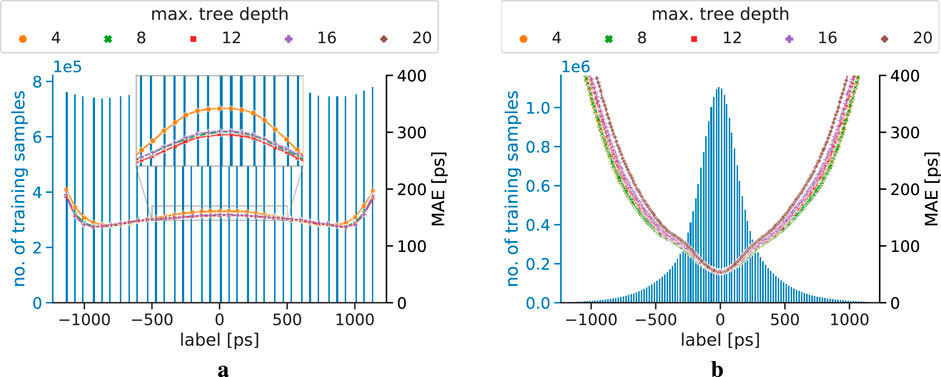
Figure 5. MAE progression of the correction models trained on a dataset using a step width of 10 mm, and tested on unseen data with a step width of 10 mm. The blue axis and histogram display the label distribution of the training dataset. The MAE progression
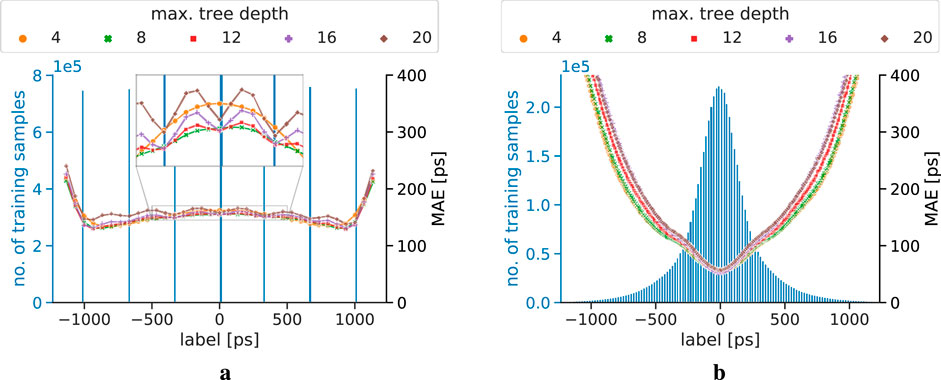
Figure 6. MAE progression of the correction models trained on a dataset using a step width of 50 mm, and tested on unseen data with a step width of 10 mm. The blue axis and histogram displays the label distribution of the training dataset. (a) Implicit correction models. (b) Explicit correction models.
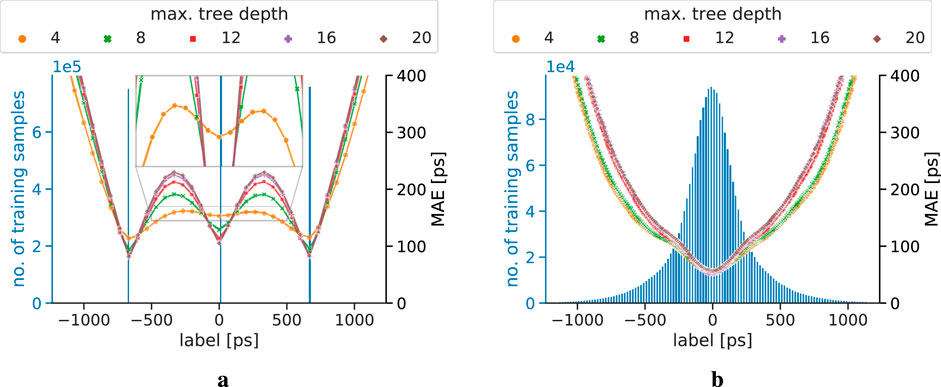
Figure 7. MAE progression of the correction models trained on a dataset using a step width of 100 mm, and tested on unseen data with a step width of 10 mm. The blue axis and histogram display the label distribution of the training dataset. (a) Implicit correction models. (b) Explicit correction models.
For both correction approaches trained on 10 mm step width data, no significant performance differences for different maximum depths of the trees can be observed, except for the implicit correction model with maximum depth 20 providing by far the worst MAE performance (MAEs
The MAE performances of models trained on data with fewer source positions on the
The implicit correction models are strongly affected by spatial undersampling, which can also be seen in the corresponding label distribution of the training data. Oscillation effects in the MAE progression can be seen, and become more dominant the bigger the spatial undersampling is, which was first observed and studied in [46]. In addition, implicit correction models with low maximum tree depth show a more robust behavior against the spatial undersampling than models with higher complexity. This can especially be seen in Figure 6a, where the zoom-in window clearly reveals oscillation effects for the implicit models starting from a maximum tree depth of 12. Furthermore, the figure also suggests that the model with a maximum tree depth of 8 shows very minor oscillation behavior, being in a transition zone between non-oscillation
The observations show that all explicit correction models meet the data scientific quality check. All implicit correction models pass the quality check for a training step width of 10 mm, except for
4.1.2 Physics-based evaluation
The estimated
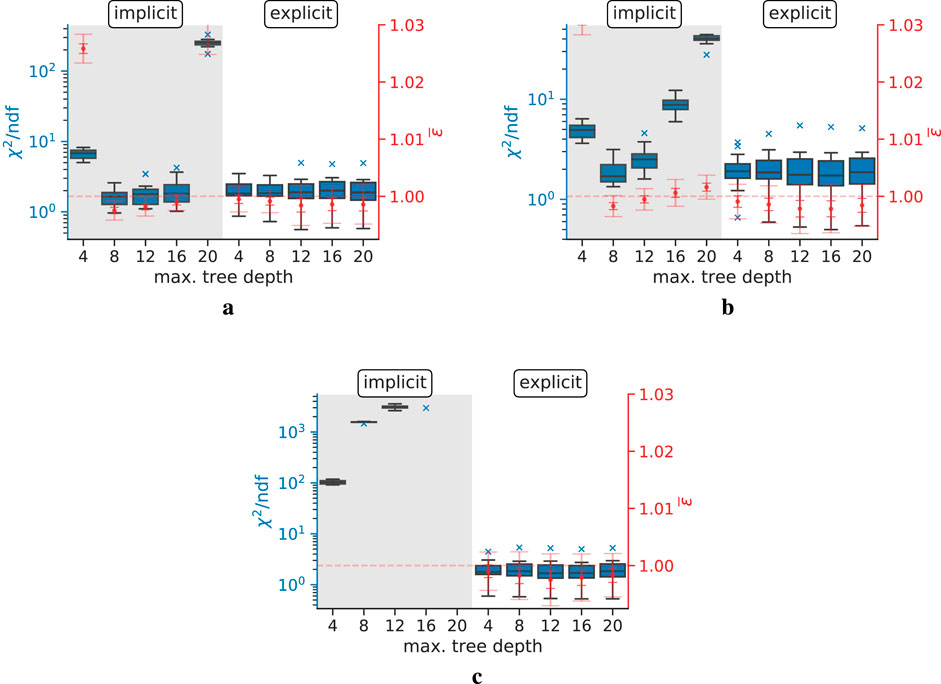
Figure 8. Linearity evaluation for the implicit and explicit models trained on training data with different step widths. The blue box plots correspond to the blue axis on the left and represent the reduced
4.1.3 PET-based evaluation
All correction models that passed the quality control are able to improve the CTR. The achieved timing resolutions estimated from a point source in the isocenter are listed in Table 1; Tables A1, A2. Furthermore, the results of the two models
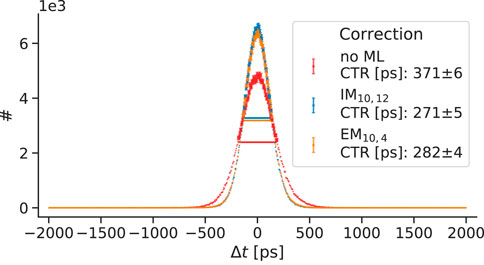
Figure 9. Obtained timing resolutions for different implicit and explicit correction models. The values are based on coincidences being in an energy window from 430 keV to 590 keV. The correction model ‘no ML’ refers to performing only an analytical time skew calibration (first part of the residual physics calibration scheme), without subsequent use of machine learning.
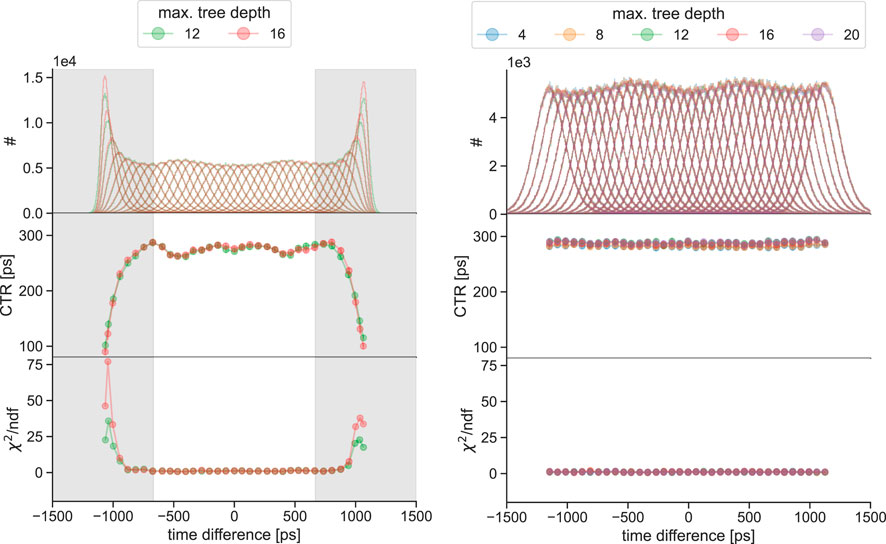
Figure 10. CTR progression along the
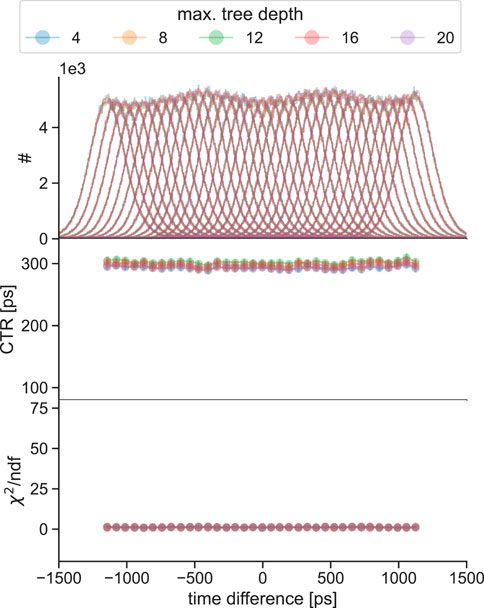
Figure 11. CTR progression along the
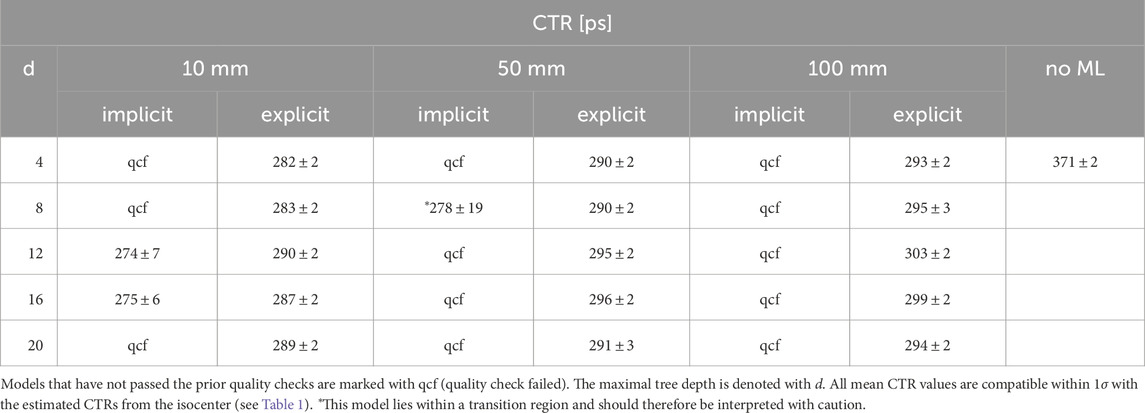
Table 2. Mean CTR values along the
4.2 In-plane distribution study
4.2.1 Data scientific evaluation
The data scientific evaluations of the trained explicit correction models are depicted in Figures 12, 13. It can be seen that the MAE progression is smooth, and no outliers or oscillation effects are visible. The shape of the MAE progression resembles the U-form, which is already known from the previous study. There are slight differences visible for different in-plane source distributions, namely, that models trained on extended source distribution show a flatter MAE progression than compressed distributions when moving towards label values with a large magnitude. Apparently, this trend occurs for all the different source distributions, except for the case of 3 × 3, which performs the worst. This trend is reversed for small label magnitudes, where models trained on compressed source distributions show lower MAE values. For convenience, Figure 12 also displays the MAE progression of a dummy model predicting a correction value of 0 ps for every input.
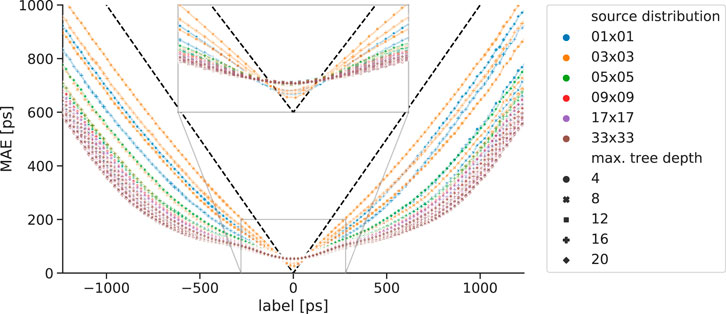
Figure 12. MAE progression of explicit correction models trained with different maximum tree depth and in-plane source distributions. The dashed black line displays the MAE progression of a dummy model predicting for every input a correction value of 0 ps.
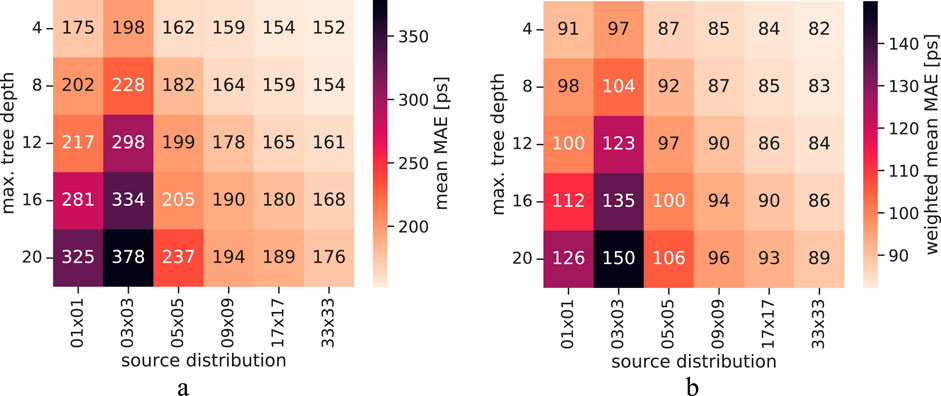
Figure 13. Visualizations of the mean MAE and weighted mean MAE dependent on the maximum tree depth and the source distribution. While the mean MAE is given as mean of the points displayed as curve in Figure 12, the weighted mean is calculated by weighting the MAE value of a specific label with the label’s occurrence frequency. (a) Mean MAE. (b) Weighted mean MAE.
For better visualization and interpretability, the mean MAE values and weighted mean MAE values are displayed as heatmaps in Figure 13. The mean MAE values are calculated as the mean of the MAE progression curve of Figure 12, while the weighted mean MAE values are given by considering the relative label occurrence (see Figure 5b). Both plots reveal the trend of improved MAE performance when models with small maximum tree depth are trained on the extended source distribution. Again, the case of 3 × 3 sources serves as an exception.
4.2.2 Physics-based evaluation
All trained models were evaluated regarding their linearity property and agreement with physics using the methods explained in Section 3.2.2. For the sake of better visualization and comparability, the results are displayed as heatmaps showing the
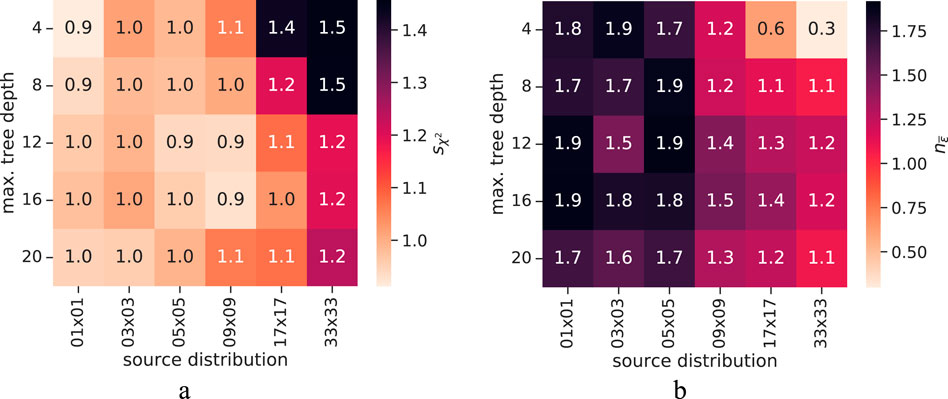
Figure 14. Visualization of the metrics used during the physics-based evaluation dependent on the maximum tree depth and the source distribution. (a) Calculated sχ2 values. (b) Calculated
The heatmap displaying the
Overall, the plots demonstrate that all explicit correction models show a high agreement with our physics-based expectations with only minor differences between differently trained models.
4.2.3 PET-based evaluation
The timing resolutions achieved when using the trained explicit correction models are listed in Tables 3, 4; Table A3. Some of the resulting time difference distributions are depicted in Figure 15. Models that have been trained on extensive in-plane source distributions show the best CTR values. Furthermore, the values indicate the trend that training on more in-plane sources leads to models with a higher ability to correct deteriorating effects, resulting in better CTRs. An exception is given by models trained on 3 × 3 in-plane sources, which return the worst CTR performance. Although no general trend regarding the maximum tree depth is visible, in the two extreme cases (1 × 1 and 33 × 33) explicit models with a maximum depth of 4 perform the best.
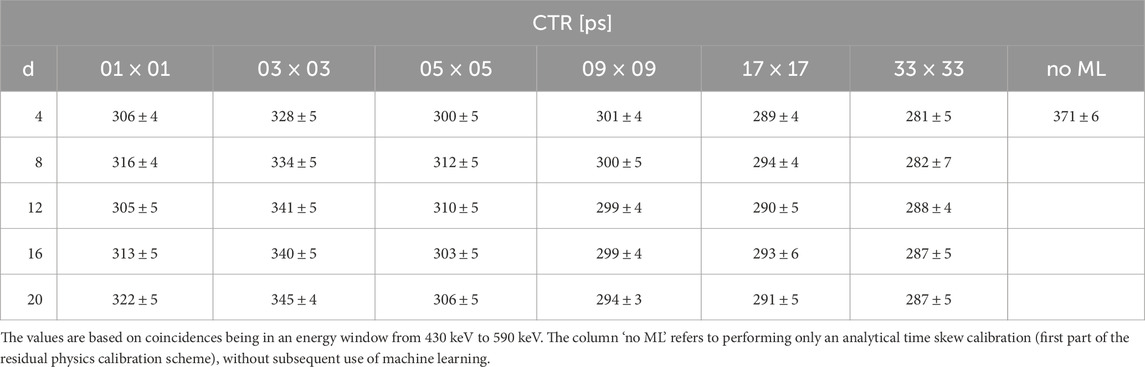
Table 3. Obtained timing resolutions after usage of explicit correction models trained on data with different in-plane source distributions.
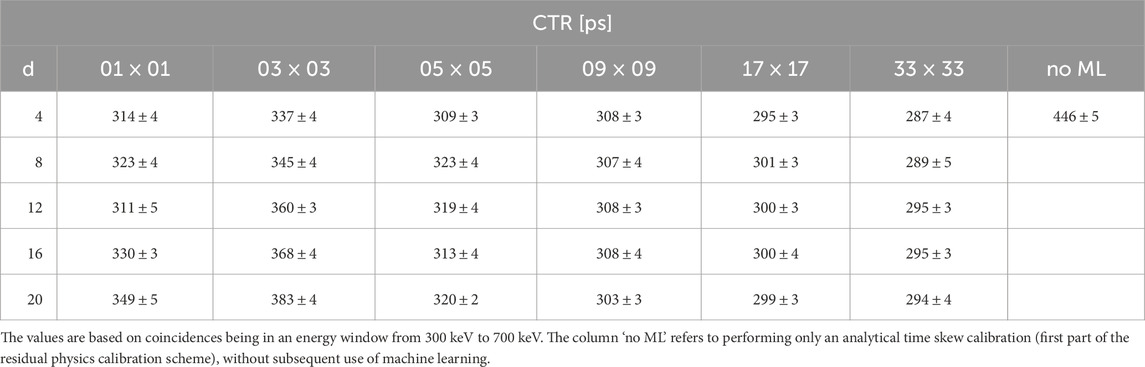
Table 4. Obtained timing resolutions after usage of explicit correction models trained on data with different in-plane source distributions.
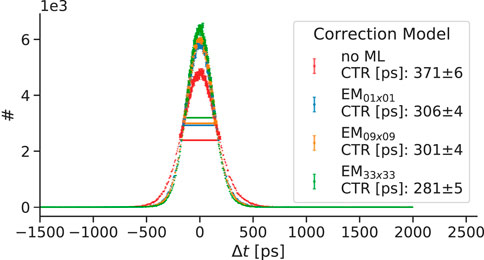
Figure 15. Obtained timing resolutions for explicit correction models trained on data with different in-plane source distributions. The values are based on coincidences being in an energy window from 430 keV to 590 keV. The correction model ‘no ML’ refers to performing only an analytical time skew calibration (first part of the residual physics calibration scheme), without subsequent use of machine learning.
When comparing the CTRs from coincidences of a small and large energy window, one sees only a minor degradation
All trained explicit correction models are able to significantly improve the achievable timing resolution compared to performing only an analytical time skew correction without subsequent use of machine learning. For the best case, the CTR can be improved by nearly 25% for data with an energy from 430 keV to 590 keV, and 36% for data with an energy from 300 keV to 700 keV.
5 Discussion
In this work a novel formulation of a residual physics-based timing calibration was introduced, capable of providing explicit timestamp corrections values. Two distinguished aspects of the calibration were investigated. In the transaxial performance study the effect of reduced source positions along the
The data scientific evaluation of the transaxial performance study revealed both approaches are capable of significantly improving the achievable CTR when training and testing data have the same spatial sampling. All explicit and implicit correction models showed a smooth MAE progression, except for the implicit correction model with a maximum tree depth of 20. Although the learning curves of this model showed no abnormal course and converged to a minimum, the validation error was clearly higher than for the other implicit models. We assume that the model might be too complex for the given problem, suppressing effective learning. As soon as the spatial sampling of the training data became more sparse than the spatial sampling of the test data, implicit correction models showed the tendency to oscillation effects. Those effects led to a very good performance at positions that are known to the model, and worse performance at unknown positions. It can be stated that implicit models with a high maximum tree depth tended to be more sensitive to undersampling than models with less complexity. Although the MAE curves of the explicit correction models seemed steeper, indicating a higher MAE, one has to consider the underlying label distribution. While there are many training samples with low label magnitudes, the number of high magnitude label samples is low making it hard for the models to learn characteristic patterns. The results suggested that explicit correction models are more robust against a spatially undersampled training dataset. This can be reasoned by the fact, that the label distribution is independent from the location and number of source positions along the
In the in-plane distribution study, we demonstrated that the explicit correction formulation is capable of providing good results, even if the training data does not have multiple source positions along the
6 Summary and outlook
In this work, we presented a novel way of defining timing residuals using a residual physics-based timing calibration approach, allowing explicit access to TOF corrections. We demonstrated that the explicit correction approach offers many benefits compared to the implicit correction models: independence from the used spatial sampling along the axis in transaxial direction, high linearity across the full test data range, and only minor degradation regarding the achievable CTR. Since the novel formulation does not rely on measuring source positions between facing detectors, we removed the demand for a dedicated motorized setup, making the method more practical for an in-system application. Compared to our proof-of-concept study, where the best implicit correction model had a maximum tree depth of 18 [44], the novel explicit approach offers a significant reduction in the memory requirements of a model by a factor of approximately
For the future, and with the perspective of an in-system application, we want to test how stable the correction models perform when applied to different detector stacks of the same design and material. Through the design of our features, we expect some degree of robustness. However, we are also investigating foundational modeling approaches [60, 61] that will hopefully allow us to train generalistic models suitable for many detector stacks of the same kind. Additionally, we plan to investigate how various in-plane source distributions affect the training of correction models, with the goal of designing a suitable calibration phantom. Furthermore, we want to explore in future studies how the explicit correction models perform concerning the reported feature importance when applied repeatedly to data corrected with the predicted corrections.
Data availability statement
The raw data supporting the conclusions of this article will be made available by the authors, without undue reservation.
Author contributions
SN: Conceptualization, Data curation, Formal Analysis, Investigation, Methodology, Project administration, Resources, Software, Supervision, Validation, Visualization, Writing – original draft, Writing – review and editing. LL: Data curation, Investigation, Software, Writing – review and editing. VN: Supervision, Writing – review and editing, Resources. YK: Resources, Writing – review and editing. SG: Writing – review and editing, Resources. FM: Resources, Writing – review and editing. VS: Funding acquisition, Writing – review and editing, Supervision.
Funding
The author(s) declare that financial support was received for the research and/or publication of this article. Open access funding provided by the Open Access Publishing Fund of RWTH Aachen University.
Acknowledgments
We thank the RWTH Aachen University Hospital workshop, Harald Radermacher, and Oswaldo Nicolas Martinez Arriaga for the help when setting up the translation stage system.
Conflict of interest
Author VS was employed by Hyperion Hybrid Imaging Systems GmbH.
The remaining authors declare that the research was conducted in the absence of any commercial or financial relationships that could be construed as a potential conflict of interest.
The author(s) declared that they were an editorial board member of Frontiers, at the time of submission. This had no impact on the peer review process and the final decision.
Generative AI statement
The author(s) declare that no Generative AI was used in the creation of this manuscript.
Publisher’s note
All claims expressed in this article are solely those of the authors and do not necessarily represent those of their affiliated organizations, or those of the publisher, the editors and the reviewers. Any product that may be evaluated in this article, or claim that may be made by its manufacturer, is not guaranteed or endorsed by the publisher.
References
1. Karp JS, Surti S, Daube-Witherspoon ME, Muehllehner G. Benefit of time-of-flight in PET: experimental and clinical results. J Nucl Med (2008) 49:462–70. doi:10.2967/jnumed.107.044834
2. Kadrmas DJ, Casey ME, Conti M, Jakoby BW, Lois C, Townsend DW. Impact of time-of-flight on PET tumor detection. J Nucl Med (2009) 50:1315–23. doi:10.2967/jnumed.109.063016
3. Lecoq P, Morel C, Prior JO, Visvikis D, Gundacker S, Auffray E, et al. Roadmap toward the 10 ps time-of-flight PET challenge. Phys Med and Biol (2020) 65:21RM01. doi:10.1088/1361-6560/ab9500
4. Nemallapudi MV, Gundacker S, Lecoq P, Auffray E, Ferri A, Gola A, et al. Sub-100 ps coincidence time resolution for positron emission tomography with LSO:Ce codoped with Ca. Phys Med and Biol (2015) 60:4635–49. doi:10.1088/0031-9155/60/12/4635
5. Nadig V, Herweg K, Chou MMC, Lin JWC, Chin E, Li CA, et al. Timing advances of commercial divalent-ion co-doped LYSO:Ce and SiPMs in sub-100 ps time-of-flight positron emission tomography. Phys Med and Biol (2023) 68:075002. doi:10.1088/1361-6560/acbde4
6. Brunner SE, Gruber L, Marton J, Suzuki K, Hirtl A. Studies on the cherenkov effect for improved time resolution of TOF-PET. IEEE Trans Nucl Sci (2014) 61:443–7. doi:10.1109/TNS.2013.2281667
7. Terragni G, Pizzichemi M, Roncali E, Cherry SR, Glodo J, Shah K, et al. Time resolution studies of thallium based cherenkov semiconductors. Front Phys (2022) 10. doi:10.3389/fphy.2022.785627
8. Brunner SE, Schaart DR. BGO as a hybrid scintillator/Cherenkov radiator for cost-effective time-of-flight PET. Phys Med and Biol (2017) 62:4421–39. doi:10.1088/1361-6560/aa6a49
9. Kratochwil N, Auffray E, Gundacker S. Exploring cherenkov emission of BGO for TOF-PET. IEEE Trans Radiat Plasma Med Sci (2021) 5:619–29. doi:10.1109/TRPMS.2020.3030483
10. Gonzalez-Montoro A, Pourashraf S, Cates JW, Levin CS. Cherenkov radiation–based coincidence time resolution measurements in BGO scintillators. Front Phys (2022) 10. doi:10.3389/fphy.2022.816384
11. Cates JW, Choong WS, Brubaker E. Scintillation and cherenkov photon counting detectors with analog silicon photomultipliers for TOF-PET. Phys Med and Biol (2024) 69:045025. doi:10.1088/1361-6560/ad2125
12. Pourashraf S, Cates JW, Levin CS. A magnetic field compatible readout circuit for enhanced coincidence time resolution in BGO Cherenkov radiation–based TOF-PET detectors. Med Phys (2025). doi:10.1002/mp.17643
13. Konstantinou G, Lecoq P, Benlloch JM, Gonzalez AJ. Metascintillators for ultrafast gamma detectors: a review of current state and future perspectives. IEEE Trans Radiat Plasma Med Sci (2022) 6:5–15. doi:10.1109/TRPMS.2021.3069624
14. Konstantinou G, Latella R, Moliner L, Zhang L, Benlloch JM, Gonzalez AJ, et al. A proof-of-concept of cross-luminescent metascintillators: testing results on a BGO:BaF2 metapixel. Phys Med and Biol (2023) 68:025018. doi:10.1088/1361-6560/acac5f
15. Seifert S, Schaart DR. Improving the time resolution of TOF-PET detectors by double-sided readout. IEEE Trans Nucl Sci (2015) 62:3–11. doi:10.1109/TNS.2014.2368932
16. Borghi G, Peet BJ, Tabacchini V, Schaart DR. A 32 mm × 32 mm × 22 mm monolithic LYSO:Ce detector with dual-sided digital photon counter readout for ultrahigh-performance TOF-PET and TOF-PET/MRI. Phys Med and Biol (2016) 61:4929–49. doi:10.1088/0031-9155/61/13/4929
17. Tabacchini V, Surti S, Borghi G, Karp JS, Schaart DR. Improved image quality using monolithic scintillator detectors with dual-sided readout in a whole-body TOF-PET ring: a simulation study. Phys Med and Biol (2017) 62:2018–32. doi:10.1088/1361-6560/aa56e1
18. Kratochwil N, Roncali E, Cates JW, Arino-Estrada G. Timing perspective with dual-ended high-frequency SiPM readout for TOF-PET. In: 2024 IEEE nuclear science symposium (NSS), medical imaging conference (MIC) and room temperature semiconductor detector conference (RTSD) (2024). p. 1. doi:10.1109/NSS/MIC/RTSD57108.2024.10655496
19. Gundacker S, Turtos RM, Auffray E, Paganoni M, Lecoq P. High-frequency SiPM readout advances measured coincidence time resolution limits in TOF-PET. Phys Med and Biol (2019) 64:055012. doi:10.1088/1361-6560/aafd52
20. Cates JW, Choong WS. Low power implementation of high frequency SiPM readout for Cherenkov and scintillation detectors in TOF-PET. Phys Med and Biol (2022) 67:195009. doi:10.1088/1361-6560/ac8963
21. Nadig V, Hornisch M, Oehm J, Herweg K, Schulz V, Gundacker S. 16-channel SiPM high-frequency readout with time-over-threshold discrimination for ultrafast time-of-flight applications. EJNMMI Phys (2023) 10:76. doi:10.1186/s40658-023-00594-z
22. Weindel K, Nadig V, Herweg K, Schulz V, Gundacker S. A time-based double-sided readout concept of 100 mm LYSO:Ce,Ca fibres for future axial TOF-PET. EJNMMI Phys (2023) 10:43. doi:10.1186/s40658-023-00563-6
23. Piemonte C, Gola A. Overview on the main parameters and technology of modern Silicon Photomultipliers. Nucl Instr Methods Phys Res Section A: Acc Spectrometers, Detectors Associated Equipment (2019) 926:2–15. doi:10.1016/j.nima.2018.11.119
24. Acerbi F, Gundacker S. Understanding and simulating SiPMs. Nucl Instr Methods Phys Res Section A: Acc Spectrometers, Detectors Associated Equipment (2019) 926:16–35. doi:10.1016/j.nima.2018.11.118
25. Mann AB, Paul S, Tapfer A, Spanoudaki VC, Ziegler SI. A computing efficient PET time calibration method based on pseudoinverse matrices. In: 2009 IEEE nuclear science symposium conference record. Orlando, FL: IEEE (2009). p. 3889–92. doi:10.1109/NSSMIC.2009.5401925
26. Reynolds PD, Olcott PD, Pratx G, Lau FWY, Levin CS. Convex optimization of coincidence time resolution for a high-resolution PET system. IEEE Trans Med Imaging (2011) 30:391–400. doi:10.1109/TMI.2010.2080282
27. Freese DL, Hsu DFC, Innes D, Levin CS. Robust timing calibration for PET using L1-norm minimization. IEEE Trans Med Imaging (2017) 36:1418–26. doi:10.1109/TMI.2017.2681939
28. Thompson C, Camborde M, Casey M. A central positron source to perform the timing alignment of detectors in a PET scanner. IEEE Trans Nucl Sci (2005) 4:2361–5. doi:10.1109/NSSMIC.2004.1462731
29. Bergeron M, Pepin CM, Arpin L, Leroux JD, Tétrault MA, Viscogliosi N, et al. A handy time alignment probe for timing calibration of PET scanners. Nucl Instr Methods Phys Res Section A: Acc Spectrometers, Detectors Associated Equipment (2009) 599:113–7. doi:10.1016/j.nima.2008.10.024
30. Werner ME, Karp JS. TOF PET offset calibration from clinical data. Phys Med Biol (2013) 58:4031–46. doi:10.1088/0031-9155/58/12/4031
31. Defrise M, Rezaei A, Nuyts J. Time-of-flight PET time calibration using data consistency. Phys Med and Biol (2018) 63:105006. doi:10.1088/1361-6560/aabeda
32. Li Y. Consistency equations in native detector coordinates and timing calibration for time-of-flight PET. Biomed Phys & Eng Express (2019) 5:025010. doi:10.1088/2057-1976/aaf756
33. Li Y. Autonomous timing calibration for time-of-flight PET. In: 2021 IEEE nuclear science symposium and medical imaging conference (NSS/MIC) (2021). p. 1–3. doi:10.1109/NSS/MIC44867.2021.9875654
34. Rothfuss H, Moor A, Young J, Panin V, Hayden C. Time alignment of time of flight positron emission tomography using the background activity of LSO. In: 2013 IEEE nuclear science symposium and medical imaging conference. Seoul, South Korea: IEEE (2013). p. 1–3. doi:10.1109/NSSMIC.2013.6829400
35. Panin VY, Aykac M, Teimoorisichani M, Rothfuss H. LSO background radiation time properties investigation: toward data driven LSO time alignment. In: 2022 IEEE nuclear science symposium and medical imaging conference (NSS/MIC) (2022). p. 1–3. doi:10.1109/NSS/MIC44845.2022.10399107
36. Dam HT, Borghi G, Seifert S, Schaart DR. Sub-200 ps CRT in monolithic scintillator PET detectors using digital SiPM arrays and maximum likelihood interaction time estimation. Phys Med and Biol (2013) 58:3243. doi:10.1088/0031-9155/58/10/3243
37. Borghi G, Tabacchini V, Schaart DR. Towards monolithic scintillator based TOF-PET systems: practical methods for detector calibration and operation. Phys Med and Biol (2016) 61:4904–28. doi:10.1088/0031-9155/61/13/4904
38. Berg E, Cherry SR. Using convolutional neural networks to estimate time-of-flight from PET detector waveforms. Phys Med and Biol (2018) 63:02LT01. doi:10.1088/1361-6560/aa9dc5
39. LaBella A, Tavernier S, Woody C, Purschke M, Zhao W, Goldan AH. Toward 100 ps coincidence time resolution using multiple timestamps in depth-encoding PET modules: a Monte Carlo simulation study. IEEE Trans Radiat Plasma Med Sci (2021) 5:679–86. doi:10.1109/TRPMS.2020.3043691
40. Feng X, Muhashi A, Onishi Y, Ota R, Liu H. Transformer-CNN hybrid network for improving PET time of flight prediction. Phys Med and Biol (2024) 69:115047. doi:10.1088/1361-6560/ad4c4d
41. Muhashi A, Feng X, Onishi Y, Ota R, Liu H. Enhancing Coincidence Time Resolution of PET detectors using short-time Fourier transform and residual neural network. Nucl Instr Methods Phys Res Section A: Acc Spectrometers, Detectors Associated Equipment (2024) 1065:169540. doi:10.1016/j.nima.2024.169540
42. Feng X, Ran H, Liu H. Predicting time-of-flight with Cerenkov light in BGO: a three-stage network approach with multiple timing kernels prior. Phys Med and Biol (2024) 69:175013. doi:10.1088/1361-6560/ad6ed8
43. Onishi Y, Hashimoto F, Ote K, Ota R. Unbiased TOF estimation using leading-edge discriminator and convolutional neural network trained by single-source-position waveforms. Phys Med and Biol (2022) 67:04NT01. doi:10.1088/1361-6560/ac508f
44. Naunheim S, Kuhl Y, Schug D, Schulz V, Mueller F. Improving the timing resolution of positron emission tomography detectors using boosted learning—a residual physics approach. IEEE Trans Neural Networks Learn Syst (2023) 36:582–94. doi:10.1109/TNNLS.2023.3323131
45. Naunheim S, Mueller F, Kuhl Y, de Paiva LL, Schulz V, Schug D. First steps towards in-system applicability of a novel PET timing calibration method reaching sub-200 ps CTR. In: 2023 IEEE nuclear science symposium, medical imaging conference and international symposium on room-temperature semiconductor detectors (NSS MIC RTSD) (2023). p. 1. doi:10.1109/NSSMICRTSD49126.2023.10338073
46. Naunheim S, Mueller F, Nadig V, Kuhl Y, Breuer J, Zhang N, et al. Holistic evaluation of a machine learning-based timing calibration for PET detectors under varying data sparsity. Phys Med and Biol (2024) 69:155026. doi:10.1088/1361-6560/ad63ec
47. Trummer J, Auffray E, Lecoq P. Depth of interaction resolution of LuAP and LYSO crystals. Nucl Instr Methods Phys Res Section A: Acc Spectrometers, Detectors Associated Equipment (2009) 599:264–9. doi:10.1016/j.nima.2008.10.033
48. Pizzichemi M, Polesel A, Stringhini G, Gundacker S, Lecoq P, Tavernier S, et al. On light sharing TOF-PET modules with depth of interaction and 157 ps FWHM coincidence time resolution. Phys Med and Biol (2019) 64:155008. doi:10.1088/1361-6560/ab2cb0
49. Bugalho R, Francesco AD, Ferramacho L, Leong C, Niknejad T, Oliveira L, et al. Experimental characterization of the TOFPET2 ASIC. J Instrumentation (2019) 14:P03029. doi:10.1088/1748-0221/14/03/P03029
50. Nadig V, Gundacker S, Herweg K, Naunheim S, Schug D, Weissler B, et al. ASICs in PET: what we have and what we need. EJNMMI Phys (2025) 12:16. doi:10.1186/s40658-025-00717-8
51. Naunheim S, Mueller F, Nadig V, Kuhl Y, Breuer J, Zhang N, et al. Using residual physics to reach near-200 ps CTR with TOFPET2 ASIC readout and clinical detector blocks. In: 2024 IEEE nuclear science symposium (NSS), medical imaging conference (MIC) and room temperature semiconductor detector conference (RTSD) (2024). p. 1–2. doi:10.1109/NSS/MIC/RTSD57108.2024.10655453ISSN: 2577-0829
53. Krueger K, Mueller F, Gebhardt P, Weissler B, Schug D, Schulz V. High-throughput FPGA-based inference of gradient tree boosting models for position estimation in PET detectors. IEEE Trans Radiat Plasma Med Sci (2023) 7:253–62. doi:10.1109/TRPMS.2023.3238904
54. Zhou Z, Chen X, Li E, Zeng L, Luo K, Zhang J. Edge intelligence: paving the last mile of artificial intelligence with edge computing. Proc IEEE (2019) 107:1738–62. doi:10.1109/JPROC.2019.2918951
55. Deng S, Zhao H, Fang W, Yin J, Dustdar S, Zomaya AY. Edge intelligence: the confluence of edge computing and artificial intelligence. IEEE Internet Things J (2020) 7:7457–69. doi:10.1109/JIOT.2020.2984887
56. Chen T, Guestrin C. XGBoost: a scalable tree boosting system. In: Proceedings of the 22nd ACM SIGKDD International Conference on Knowledge Discovery and Data Mining, 16. New York, NY, USA: Association for Computing Machinery (2016). p. 785–94. doi:10.1145/2939672.2939785
57. Grinsztajn L, Oyallon E, Varoquaux G. Why do tree-based models still outperform deep learning on typical tabular data? In: Proceedings of the 36th International Conference on Neural Information Processing Systems, 22. Red Hook, NY, USA: Curran Associates Inc. (2024). p. 507–20.
58. Müller F, Schug D, Hallen P, Grahe J, Schulz V. Gradient tree boosting-based positioning method for monolithic scintillator crystals in positron emission tomography. IEEE Trans Radiat Plasma Med Sci (2018) 2:411–21. doi:10.1109/TRPMS.2018.2837738
59. Naunheim S, Kuhl Y, Solf T, Schug D, Schulz V, Mueller F. Analysis of a convex time skew calibration for light sharing-based PET detectors. Phys Med and Biol (2022) 68:025013. doi:10.1088/1361-6560/aca872
60. Masbaum T, Paiva LL, Mueller F, Schulz V, Naunheim S. First steps towards a foundation model for positioning in positron emission tomography detectors. In: 2024 IEEE nuclear science symposium (NSS), medical imaging conference (MIC) and room temperature semiconductor detector conference (RTSD) (2024). p. 1. doi:10.1109/NSS/MIC/RTSD57108.2024.10655512
61. Lavronenko K, Paiva LL, Mueller F, Schulz V, Naunheim S. Towards artificial data generation for accelerated PET detector ML-based timing calibration using GANs. In: 2024 IEEE nuclear science symposium (NSS), medical imaging conference (MIC) and room temperature semiconductor detector conference (RTSD) (2024). p. 1–2. doi:10.1109/NSS/MIC/RTSD57108.2024.10657766
Appendix
Consideration of the linearity of explicit correction models
Although, we will not provide a formal proof why the explicit correction models show a strong linearity robustness, in this section we analyze the behavior more theoretically. We will follow the notation used in the previous sections. Let
and the corrected time difference
The linearity analysis relies on a linear regression using
with the variables on the right side of the equation sign being defined in Equation 11. For our consideration we want to take a closer look on left side of the equation sign, since it is the part affected by the explicit correction model. Formally the expected time difference is given as
which translates in the case of explicitly corrected timestamps to
If we assume that the explicit correction model was successfully trained, we can approximate the predictions by the labels (see Equation 6),
Inserting Equation A10 into Equation A9, yields
which is the expected time difference for the non-corrected timestamps. Although, we assumed that the predictions can be approximated by the labels, the presented considerations provide some basic understanding of the robustness of the explicit correction models. Furthermore, the requirements and assumptions can probably a bit softened since we approximate the expectation value by a Gaussian fit.
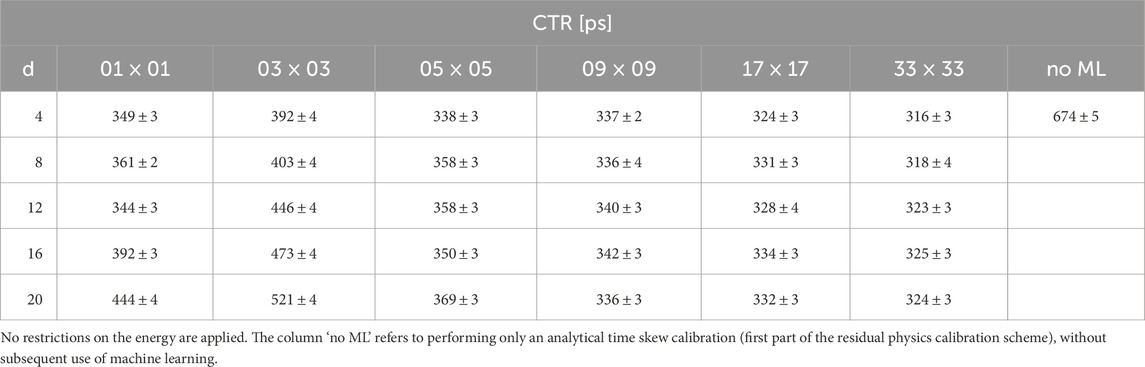
TABLE A3. Obtained timing resolutions after usage of explicit correction models trained on data with different in-plane source distributions.
Keywords: TOF, PET, CTR, machine learning, TOFPET2, light-sharing
Citation: Naunheim S, Lopes de Paiva L, Nadig V, Kuhl Y, Gundacker S, Mueller F and Schulz V (2025) Rethinking timing residuals: advancing PET detectors with explicit TOF corrections. Front. Phys. 13:1570925. doi: 10.3389/fphy.2025.1570925
Received: 04 February 2025; Accepted: 11 March 2025;
Published: 17 April 2025.
Edited by:
Woon-Seng Choong, Berkeley Lab (DOE), United StatesReviewed by:
Dominique Thers, IMT Atlantique Bretagne-Pays de la Loire, FranceSeungeun Lee, Berkeley Lab (DOE), United States
Copyright © 2025 Naunheim, Lopes de Paiva, Nadig, Kuhl, Gundacker, Mueller and Schulz. This is an open-access article distributed under the terms of the Creative Commons Attribution License (CC BY). The use, distribution or reproduction in other forums is permitted, provided the original author(s) and the copyright owner(s) are credited and that the original publication in this journal is cited, in accordance with accepted academic practice. No use, distribution or reproduction is permitted which does not comply with these terms.
*Correspondence: Stephan Naunheim, c3RlcGhhbi5uYXVuaGVpbUBwbWkucnd0aC1hYWNoZW4uZGU=; Volkmar Schulz, dm9sa21hci5zY2h1bHpAbGZiLnJ3dGgtYWFjaGVuLmRl
†ORCID: Stephan Naunheim, orcid.org/0000-0003-0306-7641; Luis Lopes de Paiva, orcid.org/0009-0004-4944-2720; Vanessa Nadig, orcid.org/0000-0002-1566-0568; Yannick Kuhl, orcid.org/0000-0002-4548-0111; Stefan Gundacker, orcid.org/0000-0003-2087-3266; Florian Mueller, orcid.org/0000-0002-9496-4710; Volkmar Schulz, orcid.org/0000-0003-1341-9356