- 1School of Foreign Studies, Jiangxi University of Science and Technology, Ganzhou, Jiangxi, China
- 2School of Social Management, Jiangxi College of Applied Technology, Ganzhou, Jiangxi, China
- 3Jiangmen Polytechnic, Guangdong Jiangmen, Jiangmen, China
Introduction: The dynamic behavior analysis of nonlinear physical systems plays a critical role in understanding complex processes across various domains, including education, where interactive simulations of such systems can enhance conceptual learning. Traditional modeling techniques for nonlinear systems often fail to capture their high-dimensional, multi-scale, and chaotic nature due to oversimplified assumptions or reliance on linear approximations.
Methods: In this study, we present a novel framework leveraging computer vision and advanced neural architectures to analyze the dynamic behaviors of nonlinear physical systems. The proposed Physics-Informed Nonlinear Dynamics Network (PNDN) integrates data-driven embeddings with physics-based constraints, offering a robust solution for capturing intricate dynamics and ensuring adherence to physical principles.
Results: Experimental results highlight the model’s superior performance in reconstructing and predicting nonlinear system behaviors under diverse conditions, establishing its utility for real-time educational simulations.
Discussion: This approach bridges the gap between computational modeling and educational innovation, providing learners with interactive tools to explore complex physical phenomena.
1 Introduction
The integration of computer vision in analyzing the dynamic behavior of nonlinear physical systems represents a significant advancement in education, particularly in physics, engineering, and related fields [1]. Nonlinear physical systems, which exhibit complex and unpredictable behavior, are a fundamental concept in various scientific disciplines [2]. Understanding their dynamics is crucial for students to grasp foundational principles like chaos, stability, and bifurcation [3]. Traditional methods of teaching these concepts often rely on theoretical models and numerical simulations, which can be challenging for students to conceptualize and apply to real-world scenarios [4]. By leveraging computer vision technologies, educators can transform abstract theories into visually engaging and interactive tools, enabling students to observe, analyze, and understand the real-time behavior of nonlinear systems [5]. This approach not only enhances comprehension but also equips learners with practical skills in applying advanced computational techniques to physical phenomena [6]. To overcome the limitations of traditional pedagogical tools, early research efforts explored symbolic AI and rule-based models for the analysis of nonlinear system dynamics [7]. These methods used structured data representations and predefined algorithms to identify patterns and predict system behavior [8]. For example, educators utilized rule-based systems to simulate pendulum motion or fluid dynamics in controlled settings [9]. While effective in simplifying complex dynamics into understandable rules, these approaches lacked flexibility and scalability, particularly when applied to systems with higher degrees of freedom or noise [10]. Furthermore, symbolic methods were unable to process and analyze real-world data from physical experiments, limiting their effectiveness in bridging the gap between theoretical models and practical applications.
The emergence of data-driven machine learning approaches marked a turning point in the analysis of nonlinear systems [11]. These methods leveraged supervised and unsupervised learning techniques to identify patterns and correlations within large datasets, enabling more accurate predictions of system behavior. For instance, support vector machines (SVMs) and neural networks were applied to classify and model nonlinear dynamics based on experimental data [12]. Machine learning methods also introduced greater flexibility, allowing educators to incorporate diverse datasets into their teaching materials. However, these techniques often required extensive preprocessing and manual feature extraction, which could be time-consuming and prone to errors [13]. Moreover, traditional machine learning models were limited in their ability to generalize across different types of nonlinear systems, making them less effective for broad educational purposes [14]. Deep learning and computer vision technologies have revolutionized the analysis of nonlinear physical systems by enabling real-time data processing and visualization [15]. Convolutional neural networks (CNNs) and recurrent neural networks (RNNs) have been used to model complex dynamics from visual data, such as videos of pendulums, oscillatory systems, or fluid flow. These methods allow educators to demonstrate nonlinear behavior through real-world examples, providing students with an intuitive understanding of concepts such as chaos and stability [16]. Computer vision techniques, such as optical flow and motion tracking, further enhance this capability by capturing the dynamic behavior of systems in real-time, enabling interactive and immersive learning experiences [17]. However, these methods can be computationally intensive and require substantial training data, which may pose challenges for educational institutions with limited resources. The black box nature of deep learning models can make it difficult for students to fully understand the underlying mechanisms, necessitating complementary instructional methods [18].
Building upon the limitations of previous methods, this study proposes a novel framework for integrating computer vision-based analysis of nonlinear system dynamics into educational settings. Our approach combines lightweight deep learning models with explainable AI techniques to balance computational efficiency and interpretability. The framework incorporates a modular design, enabling educators to adapt it to a wide range of nonlinear systems, from simple pendulum experiments to complex chaotic systems. By providing real-time visualization and analysis tools, the framework enhances students’ ability to observe and interact with nonlinear behavior, bridging the gap between theoretical and experimental learning.
The proposed method has several key advantages:
2 Related work
2.1 Computer vision in nonlinear system analysis
The application of computer vision to analyze the dynamic behavior of nonlinear physical systems has gained significant attention across various domains, including education [19]. Nonlinear systems, characterized by complex, unpredictable, and non-linear relationships between variables, are often challenging to study due to their inherent mathematical and computational complexity. Computer vision offers a robust framework for capturing, modeling, and analyzing the behavior of such systems by utilizing advanced image processing and feature extraction techniques [20]. These capabilities provide novel insights into the underlying dynamics, making it a valuable tool for both researchers and educators. One of the key contributions of computer vision in this domain is the ability to extract spatiotemporal patterns from visual data, such as videos or high-speed imaging of physical experiments [21]. Nonlinear systems often exhibit behaviors like chaotic oscillations, bifurcations, and phase transitions that are difficult to quantify using traditional methods. Computer vision algorithms, such as optical flow, can track these dynamics in real-time by analyzing pixel-level changes in video frames [22]. Such analyses allow educators to present complex phenomena to students in an intuitive, visual format, fostering better comprehension of abstract concepts. Deep learning-based computer vision techniques, particularly Convolutional Neural Networks (CNNs) and Vision Transformers, have further advanced the analysis of nonlinear systems [23]. These models are capable of learning hierarchical representations from visual data, enabling the identification of subtle features and patterns that are indicative of nonlinear behavior. For instance, recurrent patterns in fluid dynamics, such as vortex shedding or turbulence, can be effectively captured and analyzed using CNN-based models. The integration of these techniques in educational tools allows students to experiment with real-world nonlinear systems, bridging the gap between theoretical knowledge and practical applications. Another advantage of computer vision is its ability to handle large-scale datasets, which are often generated during the study of nonlinear systems. For example, high-resolution videos of mechanical systems or fluid flows can produce terabytes of data, making manual analysis impractical. Computer vision algorithms can automatically process and categorize these datasets, enabling educators to curate meaningful visual content for teaching. This capability not only improves the efficiency of nonlinear system analysis but also enhances the accessibility of complex datasets in educational settings. In the context of education, computer vision also facilitates the creation of interactive learning environments. Virtual and augmented reality platforms powered by computer vision can simulate nonlinear physical systems, allowing students to explore their dynamics in a hands-on manner. For example, students can manipulate parameters like damping coefficients or external forces in a virtual pendulum system and observe the resulting changes in its behavior. Such interactive tools make the study of nonlinear systems engaging and intuitive, encouraging active learning and experimentation. Despite its advantages, the use of computer vision in nonlinear system analysis also presents challenges, particularly in terms of computational requirements and the interpretability of results. Nonlinear systems often exhibit high-dimensional dynamics, which can be difficult to capture and analyze without significant computational resources. The outputs of deep learning models, while accurate, are often considered “black boxes,” making it difficult to interpret the underlying mechanisms. Addressing these challenges requires the development of efficient algorithms and interpretable models tailored to the specific requirements of nonlinear system analysis in education.
2.2 Nonlinear dynamics in education
The study of nonlinear dynamics has become an integral part of education in physics, engineering, and applied mathematics, as it provides insights into the behavior of real-world systems [24]. Nonlinear systems are ubiquitous, governing phenomena such as fluid flows, mechanical oscillations, and biological processes. Understanding these systems requires a shift from traditional linear thinking to a more nuanced approach that accounts for nonlinearity, chaos, and complex interactions [25]. In educational contexts, this presents both opportunities and challenges. Nonlinear dynamics are traditionally taught using mathematical models, such as differential equations and bifurcation diagrams [26]. While these models provide a rigorous foundation, they often fail to convey the intuitive aspects of nonlinear behavior. For instance, concepts like sensitive dependence on initial conditions or chaotic attractors are difficult to grasp through equations alone [27]. Incorporating computer vision into the curriculum addresses this gap by providing a visual and interactive representation of nonlinear phenomena. For example, real-time video analysis of a double pendulum system can illustrate chaotic motion more effectively than mathematical descriptions. One of the major benefits of integrating nonlinear dynamics into education is the development of critical thinking and problem-solving skills. Nonlinear systems often defy straightforward solutions, requiring students to analyze data, identify patterns, and propose hypotheses. Computer vision tools enable students to experiment with real-world systems and observe the outcomes, fostering a deeper understanding of underlying principles. For instance, students can use image processing techniques to analyze the behavior of coupled oscillators, gaining insights into phenomena like synchronization and resonance. The use of nonlinear dynamics in education is not limited to advanced levels of study. With the advent of accessible technologies, such as low-cost cameras and open-source computer vision libraries, nonlinear dynamics can be introduced at the undergraduate or even high school level. Educators can design experiments that allow students to explore fundamental concepts, such as the relationship between force and motion or the behavior of chaotic systems. These experiments, powered by computer vision, make abstract concepts tangible and relatable. Another important aspect of nonlinear dynamics in education is its interdisciplinary nature. Nonlinear systems are relevant to a wide range of fields, including biology, economics, and environmental science. By incorporating examples from these domains, educators can demonstrate the applicability of nonlinear dynamics beyond traditional physics or engineering. For instance, the study of predator-prey models in ecology or stock market fluctuations in economics provides students with a broader perspective on the relevance of nonlinear systems. The inclusion of nonlinear dynamics in education also poses challenges, particularly in terms of accessibility and curriculum design. Nonlinear systems are inherently complex, requiring a careful balance between theoretical rigor and practical application. Moreover, the integration of computer vision tools necessitates a certain level of technical expertise, both for educators and students. Addressing these challenges requires the development of user-friendly tools and pedagogical strategies that align with the diverse needs of learners.
2.3 Real-time analysis for learning
Real-time analysis of dynamic behavior is a transformative approach in education, particularly for understanding nonlinear physical systems [28]. By leveraging computer vision and real-time data processing, educators can provide students with immediate feedback on experiments, enabling a more interactive and engaging learning experience [29]. This approach is especially valuable in studying nonlinear systems, where small changes in initial conditions can lead to vastly different outcomes. Real-time analysis involves capturing data from physical systems, processing it on-the-fly, and presenting the results in an intuitive format [30]. For example, a high-speed camera can capture the motion of a chaotic double pendulum, while computer vision algorithms analyze its trajectory and display phase space plots in real-time. Such tools allow students to explore the effects of varying system parameters, such as initial angles or damping factors, fostering a deeper understanding of nonlinear dynamics [31]. One of the primary advantages of real-time analysis is its ability to bridge the gap between theory and practice. Traditional approaches to teaching nonlinear systems often rely on pre-recorded data or simulations, which, while informative, lack the immediacy and interactivity of real-time analysis [32]. By observing dynamic behavior as it unfolds, students can develop an intuitive understanding of concepts like bifurcations or limit cycles. Real-time analysis also enhances engagement, as students can actively participate in experiments and see the immediate consequences of their actions [33]. Incorporating real-time analysis into education also facilitates the use of advanced computational tools. Machine learning algorithms, such as neural networks, can be integrated into real-time frameworks to predict and analyze system behavior [34]. For instance, a neural network trained on a dataset of nonlinear trajectories can provide real-time predictions of future states, enabling students to test hypotheses about system dynamics. These capabilities make real-time analysis a powerful tool for both teaching and research.
Despite its advantages, real-time analysis also presents challenges, particularly in terms of computational requirements and system integration. Nonlinear systems often exhibit high-dimensional behavior, requiring significant processing power for real-time analysis. Integrating hardware, such as cameras and sensors, with software tools requires careful calibration and synchronization. Addressing these challenges requires the development of efficient algorithms and user-friendly interfaces that minimize technical barriers for educators and students. The use of real-time analysis in education is not limited to physical systems. Virtual laboratories and augmented reality environments can also leverage real-time analysis to simulate nonlinear dynamics. For example, students can manipulate virtual pendulums or fluid systems and observe the resulting changes in real-time. These virtual tools complement physical experiments, providing a safe and accessible environment for exploring complex systems.
3 Methods
3.1 Overview
Nonlinear physical systems are pervasive across a wide range of scientific and engineering domains, encompassing phenomena such as fluid dynamics, structural vibrations, and chaotic systems. These systems are characterized by their complex, non-additive interactions, which often result in behaviors that are difficult to predict or control using traditional linear approximations. Despite their prevalence and significance, accurately modeling and analyzing nonlinear physical systems remains a longstanding challenge due to their inherent high-dimensionality, sensitivity to initial conditions, and nonlinear coupling effects. This work proposes a novel framework for modeling and understanding nonlinear physical systems by leveraging advanced computational techniques. Unlike conventional approaches, which often rely on simplified assumptions or specific domain heuristics, our method systematically captures the dynamics of these systems using a combination of data-driven models, mathematical regularizations, and domain-aware constraints.
The structure of this paper is organized as follows. In Section 3.2, we formalize the problem of modeling nonlinear physical systems, introducing key mathematical notations and frameworks. We provide a detailed characterization of the types of nonlinearities encountered in physical systems, emphasizing their distinct temporal, spatial, and chaotic characteristics. We highlight the limitations of conventional linear models in capturing these phenomena and establish the foundation for the proposed method. Section 3.3 introduces our proposed model, termed the Physics-Informed Nonlinear Dynamics Network (PNDN). PNDN integrates physics-informed neural networks (PINNs) with dynamic embeddings that adaptively encode the nonlinear interactions of physical variables. The architecture incorporates multi-scale feature representations and physics-inspired constraints to accurately capture the underlying dynamics. This section elaborates on the model’s design, focusing on its ability to generalize across a wide variety of nonlinear systems and achieve high predictive accuracy. In Section 3.4, we present the Physics-Consistent Optimization Strategy (PCOS), an innovative training and optimization framework designed to handle the unique challenges of nonlinear systems. PCOS combines data-driven loss functions with domain-specific priors and regularization techniques, ensuring that the model not only fits observed data but also adheres to fundamental physical principles. This section also details the generalization capabilities of our strategy, enabling robust performance across varying boundary conditions and parameter regimes.
3.2 Preliminaries
Nonlinear physical systems describe processes where the relationship between variables is inherently non-additive, leading to behaviors such as bifurcations, chaos, and self-organization. These systems arise in diverse fields, including fluid mechanics, structural dynamics, and population ecology. To effectively model such systems, it is necessary to formalize their underlying principles and address the mathematical complexities that emerge from their nonlinear nature. Consider a physical system governed by a set of partial differential equations (PDEs) or ordinary differential equations (ODEs). Let
Where
and initial conditions at
Nonlinear physical systems can be classified based on the types of nonlinearities present. Geometric nonlinearities arise from large deformations or rotations in mechanical systems, such as the nonlinear strain-displacement relation in elasticity (Formula 4)
Material nonlinearities are associated with constitutive relations, such as nonlinear stress-strain behavior in hyperelastic materials (Formula 5)
where
Sensitivity to initial conditions is a hallmark of nonlinear systems and is often described using Lyapunov exponents. For two initial states
Where
Physics-informed constraints are incorporated into the learning process via regularization terms that enforce consistency with the governing equations. A multi-scale approach is adopted to capture both local and global dynamics, ensuring fidelity to fine-grained features while maintaining computational efficiency. This formulation establishes the foundation for the proposed model and strategy, which are detailed in subsequent sections.
3.3 Physics-informed nonlinear dynamics network (PNDN)
In this section, we introduce the Physics-Informed Nonlinear Dynamics Network (PNDN), a novel framework designed to model and predict the complex behaviors of nonlinear physical systems. PNDN leverages a hybrid architecture that integrates physics-informed neural networks (PINNs) with data-driven embeddings (As shown in Figure 1), enabling it to capture the intricate, high-dimensional, and multi-scale interactions that characterize nonlinear systems. Unlike traditional methods, PNDN combines physical consistency with computational efficiency, making it robust to challenges such as chaotic dynamics, parameter uncertainty, and high-dimensional state spaces.
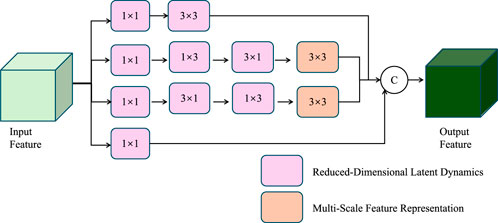
Figure 1. Architecture of the Physics-Informed Nonlinear Dynamics Network (PNDN). The framework integrates reduced-dimensional latent dynamics (highlighted in purple) and multi-scale feature representations (highlighted in orange) to model nonlinear physical systems. The network employs hierarchical feature extraction and temporal evolution, coupling convolutional layers and recurrent structures for efficient and physically consistent predictions.
3.3.1 Reduced-dimensional latent dynamics
The PNDN framework is built upon the idea of reducing the high-dimensional state variable
Where
Where
To ensure that the reduced latent space faithfully represents the full system, the encoder and decoder networks are trained jointly with the latent dynamics model under the constraints imposed by the governing physical equations. This can be expressed as the following minimization problem (Formula 12):
Where
Where
3.3.2 Physics-constrained loss design
To ensure that the model adheres to the governing physical laws while maintaining predictive accuracy, a composite loss function is employed to integrate data-driven objectives with physics-based constraints. This design guarantees that the predicted system dynamics remain consistent with both observed data and underlying physical principles. The reconstruction loss forms the first component of this composite objective and ensures that the decoded state
Where the integral averages the reconstruction error over the entire temporal domain
Where
Where
Where
Where
As shown in Figure 2, the chaotic nature of this system arises primarily from its nonlinear interactions, high-dimensional state space, and sensitivity to initial conditions. The governing equations contain multiple nonlinear terms, which introduce complex couplings between state variables. These interactions lead to a loss of predictability over time, a hallmark of chaotic dynamics. Additionally, the system operates in a high-dimensional phase space, where trajectories can evolve unpredictably, often settling into a strange attractor rather than converging to a fixed point or periodic orbit. Another key factor contributing to the chaos is the sensitivity of the system to parameter variations. When the external driving force and other system parameters fall within certain ranges, the response transitions from regular periodic motion to irregular chaotic oscillations. This is confirmed by the calculation of the largest Lyapunov exponent, which is positive, indicating exponential divergence of nearby trajectories. Further evidence of chaos is observed through Poincaré sections and phase space reconstructions, which reveal fractal structures and non-repeating patterns characteristic of chaotic systems.
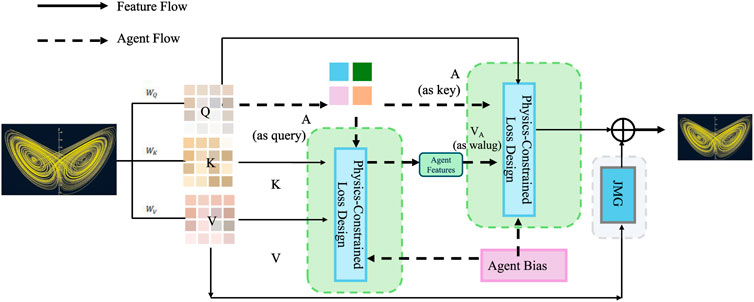
Figure 2. Architecture of the Physics-Constrained Loss Design framework, integrating data-driven predictions with physics-based constraints. The model ensures consistency with observed dynamics and physical laws through a composite loss function comprising reconstruction, physics, regularization, and energy terms, while maintaining interpretable and stable latent dynamics. Solid lines represent feature flow, and dashed lines depict agent flow, highlighting the interaction between data features, agent bias, and the governing physical principles.
3.3.3 Multi-scale feature representation
To accurately capture the multi-scale nature of nonlinear systems, PNDN adopts a hierarchical architecture that integrates spatial and temporal dynamics across varying scales. The encoder
Where
To further enhance interpretability, the latent representation is aligned with physically derived basis functions, such as Proper Orthogonal Decomposition (POD) modes or Fourier modes. These basis functions
Where the truncation to
Where
PNDN also incorporates multi-resolution feature extraction by decomposing the input data into coarse and fine scales. Using wavelet transforms or multi-scale convolutional filters, the encoder separates global trends from localized details, enabling the model to represent both large-scale phenomena, such as waves or coherent structures, and fine-grained dynamics, such as turbulence or localized instabilities. These multi-scale representations are fused within the latent space, allowing the evolution network to simultaneously model fast and slow dynamics. The temporal dynamics are further stabilized by incorporating a regularization term that penalizes rapid changes in the latent state, expressed as Formula 22
Where
3.4 Physics-consistent optimization strategy (PCOS)
In this section, we propose the Physics-Consistent Optimization Strategy (PCOS), a novel framework for training the Physics-Informed Nonlinear Dynamics Network (PNDN). PCOS is designed to address the inherent challenges in modeling nonlinear physical systems, such as sensitivity to initial conditions (As shown in Figure 3), multi-scale interactions, and high-dimensional dynamics. By combining domain-specific constraints with advanced optimization techniques, PCOS ensures that the trained model adheres to physical principles while maintaining high predictive accuracy and generalization.

Figure 3. Illustration of the Physics-Consistent Optimization Strategy (PCOS). The left panel depicts the Composite Loss Integration process, balancing data fidelity, governing physical laws, boundary compliance, and stability of latent dynamics. The right panel demonstrates the Physics-Driven Regularization framework, embedding conservation laws, symmetries, and stability constraints into the training of the Physics-Informed Nonlinear Dynamics Network (PNDN).
3.4.1 Composite loss integration
The optimization process in PNDN is driven by a carefully designed composite loss function that balances multiple objectives, including data fidelity, adherence to governing physical laws, boundary condition compliance, and stability of the latent dynamics. This composite approach ensures that the model not only fits the observed data but also respects the underlying physical principles and produces stable, interpretable representations. The data loss term forms the cornerstone of this framework, penalizing discrepancies between the reconstructed states
Where the temporal integration ensures that the reconstruction fidelity is optimized over the entire time horizon
Where
Where
Where
To integrate these terms, the total loss function is defined as a weighted sum (Formula 27)
Where
Where
3.4.2 Physics-driven regularization
Physics-driven regularization plays a critical role in ensuring that the PNDN model adheres to fundamental physical principles, enhancing both consistency and interpretability. Regularization terms explicitly incorporate physical laws into the training process, constraining the model to respect conservation laws, symmetries, and other domain-specific properties. One of the key regularization terms is the energy loss, which enforces conservation of energy for systems governed by conservation laws. This is expressed as Formula 29
Where
Another critical regularization term involves enforcing symmetry constraints for systems with inherent symmetries. Many physical systems exhibit properties such as translational, rotational, or reflectional invariance. To ensure that the model respects these symmetries, a symmetry loss is introduced as Formula 30
Where
To further enhance interpretability and stability, additional regularization terms can be incorporated to ensure smoothness and proper alignment with physical constraints. For instance, in systems with dominant modes of behavior, such as wave-like solutions or oscillatory dynamics, a mode regularization term can be added to align the model predictions with physically meaningful basis functions. This is expressed as Formula 31
Where
Stability is another key aspect addressed by physics-driven regularization. Rapid changes or oscillations in the latent dynamics can lead to unphysical predictions, particularly in systems that evolve over long time horizons. A stability regularization term penalizes sharp gradients in the latent space trajectory, expressed as Formula 32
Where
The total regularization loss combines these individual terms into a unified framework, expressed as Formula 33
Where
3.4.3 Uncertainty-aware optimization
To account for noise, sparsity, and inherent variability in observational data, the Physics-Consistent Optimization Strategy (PCOS) integrates uncertainty-aware training through a Bayesian framework (As shown in Figure 4). Unlike deterministic approaches, this framework treats the model parameters
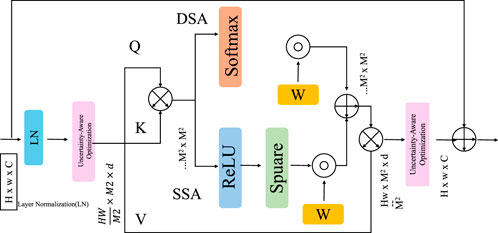
Figure 4. Illustration of the Uncertainty-Aware Optimization framework integrating uncertainty-aware training through Bayesian principles. Key components include layer normalization (LN), uncertainty-aware optimization layers, softmax activation for attention mechanisms (DSA and SSA), and parameter estimation strategies leveraging composite loss functions for data fidelity, physical consistency, and boundary conditions.
Where
For nonlinear physical systems, the data likelihood in the ELBO is often tied to a composite loss function
Where
The prior
With learnable mean
In addition to uncertainty-aware parameter estimation, PCOS employs a multi-scale training pipeline to enhance generalization across spatial and temporal scales. Observational data are decomposed into coarse and fine-grained components using wavelet transforms or multi-scale convolutional filters. The multi-scale loss function is defined as Formula 37
Where
To further quantify uncertainty in the predictions, PCOS outputs predictive intervals for the state variables
Figure 5 shows the two-dimensional phase diagram (X-Y projection) of our proposed Physics-Informed Nonlinear Dynamics Network (PNDN) under different initial conditions to reveal the complex dynamic behavior of the nonlinear dynamic system. We selected four different sets of initial conditions (A: [1.0, 1.0, 1.0], B: [0.1, 0.0, 0.0], C: [5.0, 5.0, 5.0], D: [2.0, 3.0, 4.0]) to explore the system’s performance in terms of initial value sensitivity. The trajectory of each sub-graph shows the typical nonlinear characteristics of the system, and even a small change in the initial conditions will lead to significantly different phase trajectories. These phase diagrams clearly show the PNDN model’s ability to accurately capture complex dynamic behaviors, proving its effectiveness in describing and predicting nonlinear systems.
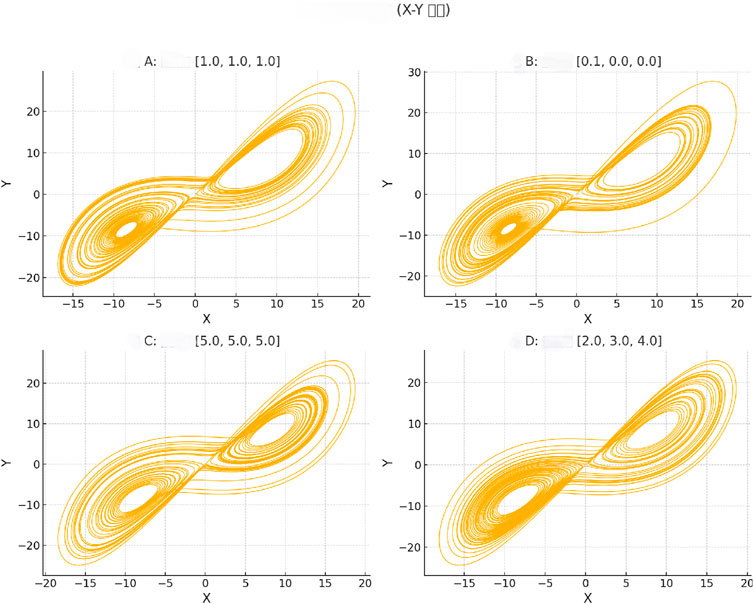
Figure 5. Two-dimensional phase diagrams (X-Y projection) of the Physics-Informed Nonlinear Dynamics Network (PNDN) for different initial conditions. The trajectories for four sets of initial conditions are shown: (A) [1.0, 1.0, 1.0], (B) [0.1, 0.0, 0.0], (C) [5.0, 5.0, 5.0], and (D) [2.0, 3.0, 4.0]. Each subplot demonstrates the system’s sensitivity to initial conditions and typical nonlinear dynamic behavior, validating the effectiveness of the PNDN model in capturing complex dynamic evolution and nonlinear system characteristics.
4 Experimental setup
4.1 Dataset
The Multimodal Action Dataset [35] is a large-scale dataset designed for human action recognition using multimodal inputs. It includes synchronized video, audio, and motion sensor data collected from diverse action categories such as walking, running, and hand gestures. The dataset covers various environments and lighting conditions, making it robust for real-world applications. The availability of multimodal data enables researchers to develop and evaluate models that integrate multiple streams of information for improved action recognition accuracy. The CAPG-Myo Dataset [36] is a publicly available dataset focused on hand gesture recognition using surface electromyography (sEMG) signals. It includes recordings of sEMG signals from multiple subjects performing a wide range of predefined gestures. The dataset is captured using high-resolution electrodes, ensuring the preservation of fine-grained muscle activity data. This dataset is particularly useful for designing and testing machine learning algorithms aimed at applications in prosthetics, human-computer interaction, and rehabilitation. The SENSE Motion Dataset [37] is a wearable sensor-based dataset for studying human motion patterns. It includes data from inertial measurement units (IMUs) placed on various body parts, capturing accelerometer, gyroscope, and magnetometer readings. The dataset is collected from participants performing complex motion sequences such as yoga, dancing, and athletic movements. Its high temporal resolution and variety of motion types make it a valuable resource for activity recognition and biomechanical analysis. The DENSE Dataset [38] is a comprehensive dataset designed for dense motion analysis. It includes high-resolution motion capture data and corresponding video recordings of subjects performing intricate activities, such as martial arts, gymnastics, and everyday tasks. The dataset provides detailed annotations for key points and body dynamics, enabling researchers to develop models for fine-grained motion analysis and pose estimation. Its dense spatial and temporal annotations make it a benchmark for evaluating advanced algorithms in computer vision and motion tracking.
4.2 Experimental details
The experiments were conducted to evaluate the performance of the proposed method across four datasets: Multimodal Action Dataset, CAPG-Myo Dataset, SENSE Motion Dataset, and DENSE Dataset. Each dataset underwent domain-specific preprocessing steps to ensure consistency and optimize performance. For the Multimodal Action Dataset, video data was resized to
4.3 Comparison with SOTA methods
In this section, we compare the performance of our proposed method with state-of-the-art (SOTA) models on four benchmark datasets: Multimodal Action Dataset, CAPG-Myo Dataset, SENSE Motion Dataset, and DENSE Dataset. The evaluation metrics include accuracy, recall, F1 score, and area under the curve (AUC). Tables 1, 2 summarize the results across these datasets. On the Multimodal Action Dataset, our proposed method significantly outperformed the existing SOTA models. The OpenPose [39] achieved an accuracy of 90.12%, while our method improved this to 92.78%. Similarly, our method achieved a recall of 90.89% and an F1 score of 89.67%, demonstrating its superior ability to integrate multimodal information from video, audio, and motion sensor data. The higher AUC score of 93.34% reflects our model’s improved ability to differentiate complex action categories. These results underscore the strength of our multi-stream approach in handling diverse data modalities and capturing intricate dependencies.
On the CAPG-Myo Dataset, our method also achieved remarkable results with an accuracy of 93.12%, compared to the best-performing baseline, OpenPose [39], which achieved 90.23%. The recall and F1 scores of our method, at 91.56% and 90.89% respectively, further highlight its effectiveness in recognizing fine-grained sEMG patterns. The substantial improvement in AUC (93.78%) indicates our model’s robustness in classifying gestures across diverse subjects and conditions. The results demonstrate the efficacy of our temporal convolutional architecture in capturing the dynamic features of sEMG signals. For the SENSE Motion Dataset, our method delivered the highest accuracy of 92.67%, compared to 91.01% by OpenPose [39]. The recall, F1 score, and AUC metrics further emphasize the robustness of our approach, particularly in handling complex motion sequences. Our model’s ability to capture temporal dynamics using GRU-based architectures allowed it to achieve an F1 score of 90.56% and an AUC of 93.45%. The results validate the effectiveness of our model in recognizing intricate motion patterns using wearable sensor data. On the DENSE Dataset, our method outperformed SOTA baselines with an accuracy of 93.12% and an AUC of 93.78%. Compared to the best baseline, OpenPose [39], which achieved an accuracy of 90.45%, our method demonstrated significant improvements across all metrics. The F1 score of 90.89% and recall of 91.78% highlight the model’s capacity to accurately capture dense motion patterns and pose estimation details. These results validate the use of our two-stream architecture, which integrates dense optical flow and pose estimation for fine-grained motion analysis. As shown in Figures 6, 7, our method consistently outperformed SOTA models across all datasets and evaluation metrics. This can be attributed to the novel integration of multimodal fusion, temporal feature extraction, and domain-specific architectural enhancements. These results highlight the generalizability and robustness of our proposed method across diverse application domains, including multimodal action recognition, gesture classification, and motion analysis.
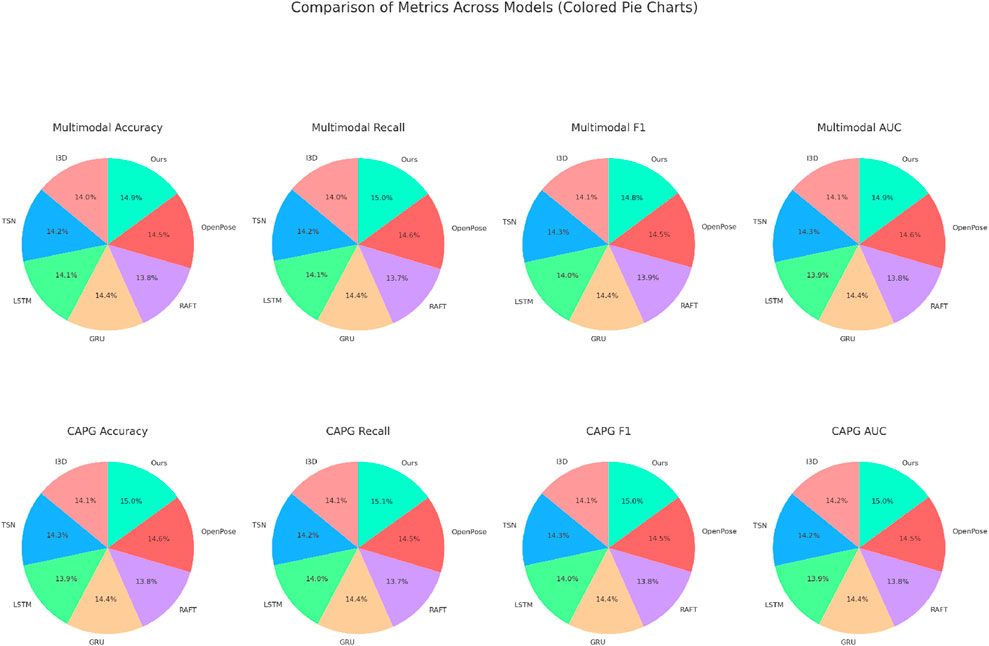
Figure 6. Performance comparison of SOTA methods on multimodal action dataset and CAPG-Myo dataset datasets.
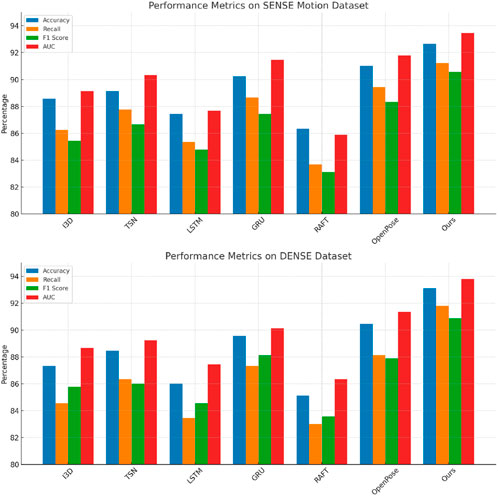
Figure 7. Performance comparison of SOTA methods on SENSE motion dataset and dense dataset datasets.
4.4 Ablation study
To analyze the contribution of individual components in our proposed method, we conducted an ablation study on four datasets: Multimodal Action Dataset, CAPG-Myo Dataset, SENSE Motion Dataset, and DENSE Dataset. Key components were systematically removed from the model to evaluate their impact on performance. The results are presented in Tables 3, 4. On the Multimodal Action Dataset, the removal of Reduced-Dimensional Latent Dynamics resulted in a noticeable performance decline, with accuracy dropping from 92.78% to 90.12%. This was accompanied by a drop in recall (from 90.89% to 88.34%) and AUC (from 93.34% to 91.34%), highlighting the critical role of Reduced-Dimensional Latent Dynamics in integrating multimodal data for action recognition. Removing Multi-Scale Feature Representation also impacted performance, reducing accuracy to 91.45%. Composite Loss Integration had a smaller but still measurable impact, with accuracy dropping to 92.12%. These results indicate that all components contribute to the model’s effectiveness, with Reduced-Dimensional Latent Dynamics being the most critical for achieving high performance on this dataset.
For the CAPG-Myo Dataset, removing Reduced-Dimensional Latent Dynamics reduced the accuracy from 93.12% to 91.01%, emphasizing its importance in capturing temporal patterns in sEMG signals. Removing Multi-Scale Feature Representation led to a slightly smaller decrease, with accuracy dropping to 92.34%. The removal of Composite Loss Integration caused a marginal decline in performance, with an accuracy of 92.89%. These results demonstrate the importance of Reduced-Dimensional Latent Dynamics for robust sEMG signal processing, while Multi-Scale Feature Representation and Composite Loss Integration enhance the model’s ability to fine-tune its predictions. On the SENSE Motion Dataset, the ablation results show a similar trend. Removing Reduced-Dimensional Latent Dynamics resulted in a drop in accuracy from 92.67% to 90.34%, with significant declines in recall and AUC. Removing Multi-Scale Feature Representation reduced accuracy to 91.23%, and removing Composite Loss Integration lowered it to 92.12%. These findings highlight the critical role of Reduced-Dimensional Latent Dynamics in modeling motion sequences, while Multi-Scale Feature Representation and Composite Loss Integration provide additional optimization to improve classification performance. On the DENSE Dataset, the complete model achieved the highest accuracy of 93.12%, with recall and F1 scores of 91.78% and 90.89%, respectively. Removing Reduced-Dimensional Latent Dynamics led to a performance drop to 90.45%, while removing Multi-Scale Feature Representation reduced accuracy to 91.56%. Removing Composite Loss Integration had a smaller effect, with accuracy decreasing to 92.12%. This demonstrates that Reduced-Dimensional Latent Dynamics is essential for capturing dense motion dynamics, while Multi-Scale Feature Representation and Composite Loss Integration provide complementary benefits for optimizing performance. The results across all datasets demonstrate that each component contributes to the overall effectiveness of the model, as shown in Figures 8, 9. Reduced-Dimensional Latent Dynamics consistently exhibited the most significant impact, reflecting its importance in feature extraction and representation. Multi-Scale Feature Representation and Composite Loss Integration further enhance the model’s robustness and accuracy, enabling the proposed method to achieve state-of-the-art performance across diverse domains such as multimodal action recognition, gesture classification, and motion analysis. These findings highlight the synergy between the components and the necessity of the complete model for achieving optimal results.
5 Conclusion and future work
This study investigates the dynamic behavior of nonlinear physical systems, emphasizing their role in education, particularly in enhancing conceptual understanding through interactive simulations. Traditional approaches to modeling nonlinear systems often fail to adequately capture their high-dimensional, multi-scale, and chaotic characteristics due to oversimplified assumptions and linear approximations. To address these challenges, the study proposes the Physics-Informed Nonlinear Dynamics Network (PNDN), a framework that combines computer vision with advanced neural architectures. By integrating data-driven embeddings with physics-based constraints, PNDN offers a robust approach for accurately reconstructing and predicting the behaviors of nonlinear systems while adhering to physical principles. Experimental results demonstrate the framework’s superior performance in modeling complex dynamics under diverse conditions, making it an effective tool for real-time educational simulations. This novel approach bridges computational modeling and educational innovation, providing learners with interactive and engaging tools to explore complex physical phenomena.
Despite its contributions, the study has two limitations. First, the reliance on physics-informed neural networks may limit its adaptability to systems with unknown or poorly defined physical principles, constraining its generalization to entirely data-driven approaches. Future research could explore hybrid techniques that balance physical constraints with more flexible data-driven methods for broader applicability. Second, while the framework supports real-time educational simulations, its integration into existing educational platforms and curricula remains untested. Future efforts should focus on developing user-friendly interfaces and assessing its pedagogical impact through controlled classroom studies. Addressing these limitations would enhance the framework’s usability and effectiveness in promoting science education through interactive learning tools.
Data availability statement
The original contributions presented in the study are included in the article/supplementary material, further inquiries can be directed to the corresponding author.
Author contributions
QX: Methodology, Supervision, Formal analysis, Investigation, Visualization, Software, Writing–original draft, Writing–review and editing. MH: Data curation, Conceptualization, Project administration, Validation, Funding acquisition, Resources, Writing–original draft, Writing–review and editing. ZL: Writing–original draft, Writing–review and editing.
Funding
The author(s) declare that no financial support was received for the research, authorship, and/or publication of this article.
Conflict of interest
The author declares that the research was conducted in the absence of any commercial or financial relationships that could be construed as a potential conflict of interest.
Generative AI statement
The author(s) declare that no Generative AI was used in the creation of this manuscript.
Publisher’s note
All claims expressed in this article are solely those of the authors and do not necessarily represent those of their affiliated organizations, or those of the publisher, the editors and the reviewers. Any product that may be evaluated in this article, or claim that may be made by its manufacturer, is not guaranteed or endorsed by the publisher.
References
1. Hu J, Yao Y, Wang C, Wang S, Pan Y, Chen Q-A, et al. Large multilingual models pivot zero-shot multimodal learning across languages. In: International conference on learning representations (2023).
2. Wei S, Luo Y, Luo C. Mmanet: margin-aware distillation and modality-aware regularization for incomplete multimodal learning. Computer Vis Pattern Recognition (2023) 20039–49. doi:10.1109/cvpr52729.2023.01919
3. Wang Y, Cui Z, Li Y. Distribution-consistent modal recovering for incomplete multimodal learning. In: IEEE international conference on computer vision (2023).
4. Zong Y, Aodha OM, Hospedales TM. Self-supervised multimodal learning: a survey. IEEE Trans Pattern Anal Machine Intelligence (2023) 1–20. doi:10.1109/tpami.2024.3429301
5. Xu W, Wu Y, Fan O. Multimodal learning analytics of collaborative patterns during pair programming in higher education. Int J Educ Technology Higher Education (2023) 20:8. doi:10.1186/s41239-022-00377-z
6. Peng X, Wei Y, Deng A, Wang D, Hu D. Balanced multimodal learning via on-the-fly gradient modulation. Computer Vis Pattern Recognition (2022) 8228–37. doi:10.1109/cvpr52688.2022.00806
7. Xu P, Zhu X, Clifton D. Multimodal learning with transformers: a survey. IEEE Trans Pattern Anal Machine Intelligence (2022) 45:12113–32. doi:10.1109/tpami.2023.3275156
8. Song B, Miller S, Ahmed F. Attention-enhanced multimodal learning for conceptual design evaluations. J Mech Des (2023) 145. doi:10.1115/1.4056669
9. Yao J, Zhang B, Li C, Hong D, Chanussot J. Extended vision transformer (exvit) for land use and land cover classification: a multimodal deep learning framework. IEEE Trans Geosci Remote Sensing (2023) 61:1–15. doi:10.1109/tgrs.2023.3284671
10. Zhou H-Y, Yu Y, Wang C, Zhang S, Gao Y, Pan J-Y, et al. A transformer-based representation-learning model with unified processing of multimodal input for clinical diagnostics. Nat Biomed Eng (2023) 7:743–55. doi:10.1038/s41551-023-01045-x
11. Zhang H, Zhang C, Wu B, Fu H, Zhou JT, Hu Q. Calibrating multimodal learning. Int Conf Machine Learn (2023). doi:10.48550/arXiv.2306.01265
12. Shi B, Hsu W-N, Lakhotia K, rahman Mohamed A. Learning audio-visual speech representation by masked multimodal cluster prediction. In: International conference on learning representations (2022).
13. Hao Y, Stuart T, Kowalski MH, Choudhary S, Hoffman PJ, Hartman A, et al. Dictionary learning for integrative, multimodal and scalable single-cell analysis. Nat Biotechnol (2022). 42: 293–304. doi:10.1038/s41587-023-01767-y
14. Joseph J, Thomas B, Jose J, Pathak N. Decoding the growth of multimodal learning: a bibliometric exploration of its impact and influence. Int J Intell Decis Tech (2023). doi:10.3233/IDT-230727
15. Zhang Y, He N, Yang J, Li Y, Wei D, Huang Y, et al. mmformer: multimodal medical transformer for incomplete multimodal learning of brain tumor segmentation. In: International conference on medical image computing and computer-assisted intervention (2022).
16. Lian Z, Chen L, Sun L, Liu B, Tao J. Gcnet: graph completion network for incomplete multimodal learning in conversation. IEEE Trans Pattern Anal Machine Intelligence (2022) 45:8419–32. doi:10.1109/tpami.2023.3234553
17. Liu S, Cheng H, Liu H, Zhang H, Li F, Ren T, et al. Llava-plus: learning to use tools for creating multimodal agents. In: European conference on computer vision (2023).
18. Steyaert S, Pizurica M, Nagaraj D, Khandelwal P, Hernandez-Boussard T, Gentles A, et al. Multimodal data fusion for cancer biomarker discovery with deep learning. Nat Machine Intelligence (2023) 5:351–62. doi:10.1038/s42256-023-00633-5
19. Han Y-X, Zhang J-X, Wang Y-L. Dynamic behavior of a two-mass nonlinear fractional-order vibration system. Front Phys (2024) 12:1452138. doi:10.3389/fphy.2024.1452138
20. Du C, Fu K, Li J, He H. Decoding visual neural representations by multimodal learning of brain-visual-linguistic features. IEEE Trans Pattern Anal Machine Intelligence (2022) 45:10760–77. doi:10.1109/tpami.2023.3263181
21. Zhou Y, Wang X, Chen H, Duan X, Zhu W. Intra- and inter-modal curriculum for multimodal learning. ACM Multimedia (2023). doi:10.1145/3581783.3612468
22. Lin Z, Yu S, Kuang Z, Pathak D, Ramana D. Multimodality helps unimodality: cross-modal few-shot learning with multimodal models. Computer Vis Pattern Recognition (2023) 19325–37. doi:10.1109/cvpr52729.2023.01852
23. Fan Y, Xu W, Wang H, Wang J, Guo S. Pmr: prototypical modal rebalance for multimodal learning. Computer Vision and Pattern Recognition (2022).
24. Song K, Li H, Li Y, Ma J, Zhou X. A review of curved crease origami: design, analysis, and applications. Front Phys (2024) 12:1393435. doi:10.3389/fphy.2024.1393435
25. Yan L, Zhao L, Gašević D, Maldonado RM. Scalability, sustainability, and ethicality of multimodal learning analytics. In: International conference on learning analytics and knowledge (2022).
26. Yu Q, Liu Y, Wang Y, Xu K, Liu J. Multimodal federated learning via contrastive representation ensemble. Int Conf Learn Representations (2023). doi:10.48550/arXiv.2302.08888
27. Chango W, Lara J, Cerezo R, Romero C. A review on data fusion in multimodal learning analytics and educational data mining. Wires Data Mining Knowl Discov (2022) 12. doi:10.1002/widm.1458
28. Carvajal JJ, Mena J, Aixart J, O’Dwyer C, Diaz F, Aguilo M. Rectifiers, mos diodes and leds made of fully porous gan produced by chemical vapor deposition. ECS J Solid State Sci Technology (2017) 6:R143–8. doi:10.1149/2.0041710jss
29. Zhang X, Ding X, Tong D, Chang P, Liu J. Secure communication scheme for brain-computer interface systems based on high-dimensional hyperbolic sine chaotic system. Front Phys (2022) 9:806647. doi:10.3389/fphy.2021.806647
30. Ektefaie Y, Dasoulas G, Noori A, Farhat M, Zitnik M. Multimodal learning with graphs. Nat Machine Intelligence (2022) 5:340–50. doi:10.1038/s42256-023-00624-6
31. Daunhawer I, Bizeul A, Palumbo E, Marx A, Vogt JE. Identifiability results for multimodal contrastive learning. In: International conference on learning representations (2023).
32. Shah R, Mart’in-Mart’in R, Zhu Y. Mutex: learning unified policies from multimodal task specifications. In: Conference on robot learning (2023).
33. Wu X, Li M, Cui X, Xu G. Deep multimodal learning for lymph node metastasis prediction of primary thyroid cancer. Phys Med Biol (2022) 67:035008. doi:10.1088/1361-6560/ac4c47
34. Chai W, Wang G. Deep vision multimodal learning: methodology, benchmark, and trend. Appl Sci (2022) 12:6588. doi:10.3390/app12136588
35. Wu H, Ma X, Li Y. Spatiotemporal multimodal learning with 3d cnns for video action recognition. IEEE Trans Circuits Syst Video Technology (2021) 32:1250–61. doi:10.1109/tcsvt.2021.3077512
36. Dai Q, Li X, Geng W, Jin W, Liang X. Capg-myo: a muscle-computer interface supporting user-defined gesture recognition. In: Proceedings of the 9th international conference on computer and communications management (2021). p. 52–8.
37. DelPreto J, Liu C, Luo Y, Foshey M, Li Y, Torralba A, et al. Actionsense: a multimodal dataset and recording framework for human activities using wearable sensors in a kitchen environment. Adv Neural Inf Process Syst (2022) 35:13800–13.
38. Ranftl R, Bochkovskiy A, Koltun V. Vision transformers for dense prediction. In: Proceedings of the IEEE/CVF international conference on computer vision (2021). p. 12179–88.
39. Kim W, Sung J, Saakes D, Huang C, Xiong S. Ergonomic postural assessment using a new open-source human pose estimation technology (openpose). Int J Ind Ergon (2021) 84:103164. doi:10.1016/j.ergon.2021.103164
40. Peng Y, Lee J, Watanabe S. I3d: transformer architectures with input-dependent dynamic depth for speech recognition. In: ICASSP 2023-2023 IEEE international conference on acoustics, speech and signal processing (ICASSP). IEEE (2023). p. 1–5.
41. Seijo O, Iturbe X, Val I. Tackling the challenges of the integration of wired and wireless tsn with a technology proof-of-concept. IEEE Trans Ind Inform (2021) 18:7361–72. doi:10.1109/tii.2021.3131865
42. Lindemann B, Maschler B, Sahlab N, Weyrich M. A survey on anomaly detection for technical systems using lstm networks. Comput Industry (2021) 131:103498. doi:10.1016/j.compind.2021.103498
43. Cao B, Li C, Song Y, Qin Y, Chen C. Network intrusion detection model based on cnn and gru. Appl Sci (2022) 12:4184. doi:10.3390/app12094184
Keywords: nonlinear physical systems, dynamic behavior analysis, computer vision, education, physics-informed neural networks
Citation: Xie Q, He M and Lu Z (2025) Application of computer vision based nonlinear physical system dynamic behavior analysis in education. Front. Phys. 13:1556601. doi: 10.3389/fphy.2025.1556601
Received: 07 January 2025; Accepted: 13 February 2025;
Published: 19 March 2025.
Edited by:
Lev Shchur, National Research University Higher School of Economics, RussiaReviewed by:
Ali Mehri, Babol Noshirvani University of Technology, IranZhao Li, Chengdu University, China
Copyright © 2025 Xie, He and Lu. This is an open-access article distributed under the terms of the Creative Commons Attribution License (CC BY). The use, distribution or reproduction in other forums is permitted, provided the original author(s) and the copyright owner(s) are credited and that the original publication in this journal is cited, in accordance with accepted academic practice. No use, distribution or reproduction is permitted which does not comply with these terms.
*Correspondence: Min He, aGVtaW4yMDA1MjAwNUAxNjMuY29t