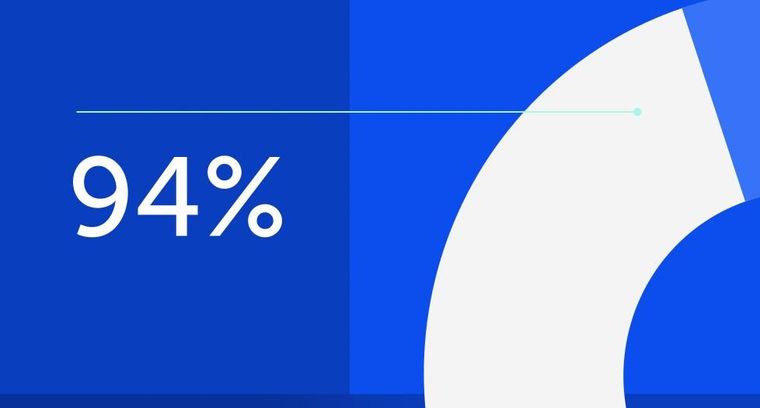
94% of researchers rate our articles as excellent or good
Learn more about the work of our research integrity team to safeguard the quality of each article we publish.
Find out more
ORIGINAL RESEARCH article
Front. Phys., 22 April 2025
Sec. Social Physics
Volume 13 - 2025 | https://doi.org/10.3389/fphy.2025.1553224
Introduction: In the aviation field, drone search and rescue is a highly urgent task involving small target detection. In such a resource-constrained scenario, there are challenges of low accuracy and high computational requirements.
Methods: This paper proposes IYFVMNet, an improved lightweight detection network based on YOLOv8. The key challenges include feature extraction for small objects and the trade-off between detection accuracy and speed. To address these, four major innovations are introduced: (1) Fasternet is used to improve the bottleneck structure in the cross-stage feature fusion backbone network. This approach fully utilizes all feature map information while minimizing the computational and memory requirements. (2) the neck network structure is optimized using the Vovnet Gsconv Cross Stage Partial module. This operation also reduces the computational cost by decreasing the amount of required feature map channels, while maintaining the effectiveness of the feature representation. (3) he Minimum Point Distance Intersection over Union loss function is employed to optimize bounding box detection during model training. (4) to construct the overall network structure, the Layer-wise Adaptive Momentum Pruning algorithm is used for thinning.
Results: Experiments on the TinyPerson dataset demonstrate that IYFVMNet achieves a 46.3% precision, 30% recall, 29.3% mAP50, and 11.8% mAP50-95.
Discussion: The model exhibits higher performance in terms of accuracy and efficiency when compared to other benchmark models, which demonstrates the effectiveness of the improved algorithm (e.g., YOLO-SGF, Guo-Net, TRC-YOLO) in small-object detection and provides a reference for future research.
In the field of precision search-and-rescue and life detection, the use of unmanned aerial vehicles is a crucial solution [1]. Whether in a boundless sea or in a complex urban environment, drones are able to detect and provide precise search and rescue locations. In these scenarios, the searched person appears as small object in a large scene [2]. Small-object detection is a long-term challenge in deep learning convolutional neural network modelling, where such models are designed to identify and ascertain the positions of small objects in low-resolution images. Such detection is used in a variety of scenarios, such as autonomous driving, intelligent monitoring, and medical image analysis.
Due to the inconspicuous features of small objects and high requirements for positioning accuracy, achieving more accurate small-object detection still faces the following challenges [3].
(1) Difficulty in feature extraction. Due to lower resolution and limited actionable feature information of small objects, it is difficult for traditional feature extraction methods to extract discriminative features effectively, which increases the difficulty of object detection.
(2) Data imbalance. In many practical applications, the number of samples of small objects is much smaller than that for large objects, which affects the efficiency of model training.
(3) Balance between detection accuracy and speed. In general, improving detection accuracy requires developing more complex network structures and using more computing resources. However, this may come at the expense of reducing detection speed. However, this could lead to a decrease in detection speed, making it challenging to strike an optimal balance.
Although there has been extensive research on small object detection in recent years, most studies have focused on improving accuracy or making specific improvements for particular scenarios, often addressing only one of the aforementioned issues. This paper, however, approaches the problem from two perspectives: feature extraction and balancing accuracy and speed under limited computational resources. YOLOv8, as the most advanced model in object detection tasks, has been widely adopted due to its high accuracy and speed. YOLOv8n, the most lightweight model in the YOLOv8 series, features fewer parameters and lower computational complexity, achieving the fastest convergence speed. Although its accuracy is slightly lower than that of larger models, it has become the preferred choice for addressing the aforementioned challenges in resource-constrained scenarios such as drone search and rescue. Therefore, based on the YOLOv8n model, this paper proposes a lightweight small object detection network, IYFVMNet. By incorporating a P2 detection head to enrich small object features and utilizing the FasterNet and VoV-GSCSP structures to balance efficiency and accuracy, the model achieves significant improvements. The main contributions of this paper are as follows.
(1) The cross-stage feature fusion (C2f) backbone network is improved using the FasterNet module to reduce channel sparsity. The structure is able to reduce both computational and memory requirements by adopting partial convolution (PConv), which performs convolution operations on only a subset of the input channels. This improvement maximizes the use of the feature map information while further lowering the computational complexity of the model.
(2) The vovnet gsconv cross stage partial (VoV-GSCSP) structure is adopted with the goal of creating a lightweight model. By using group shuffle convolution (GSConv), the number of parameters does not increase significantly while maintaining high model accuracy.
(3) The minimum point distance intersection over union (MPDIoU) loss function is used to minimize the distances between the upper-left and lower-right corner of the predicted bounding box and the ground truth bounding box, which effectively solves the limitations of the existing loss function in specific cases.
Through the aforementioned innovative designs, the proposed IYFVMNet in this paper demonstrates significant advancements over existing technologies in the following aspects. First, it achieves higher detection accuracy while maintaining lower computational complexity. Second, by incorporating lightweight design and pruning strategies, it effectively addresses the challenge of real-time detection in resource-constrained environments. Finally, the optimized loss function enables more precise localization of small objects, resulting in notable improvements in both accuracy and efficiency, while also providing a more practical solution for real-world applications. To validate the effectiveness of the improved model, experiments were conducted on the TinyPerson dataset, and comparisons were made with the baseline model YOLOv8n as well as other lightweight detection networks. The experimental results show that, compared to YOLOv8n, IYFVMNet reduces the number of model parameters by half while improving detection accuracy by 1.2%, increasing recall by 1.1%, and boosting mAP50 by 0.7%. This demonstrates clear advantages in both computational efficiency and detection accuracy.
The remainder of the paper is structured as follows. Section 2 describes some relevant work on YOLOv8 in recent years. Section 3 provides a detailed description of the baseline model. Section 4 describes the process of improving the model. Section 5 comprehensively verifies the validity of the model improvement method by performing comparison and ablation experiments. Section 6 gives the conclusions and future research directions.
With the continuous improvement of computer hardware, deep learning technologies have made significant progress in small-object detection, such as in the fields of remote sensing and medical imaging [4]. At present, deep learning object detection models are mainly divided into two major categories: one stage (SSD, YOLO series, etc.) and two stage (FastRCNN, etc.). Two-stage methods use region proposal networks to generate candidate regions, and then apply classification and localization networks to these regions to perform further classification and bounding box regression. However, when using these methods it possible that small objects are overlooked in the candidate regions generated by the region proposal network, and it difficult for them to identify small objects in the subsequent classification and localization process. Consequently, these methods suffer from performance loss when applied to small targets. One-stage approaches predict the bounding box and categories directly on an image for end-to-end object detection, which are more suitable for real-time detection of small objects and they have higher computational efficiency. As an example, the YOLO series has received extensive research attention in this field [5].
In recent years, YOLOv8 has been widely applied in various fields and continuously driven breakthroughs in innovative technologies [6]. Gunawan et al. [7] compiled a self-built long-distance face recognition dataset and achieved good results. Zhao et al. [8] proposed the Z-YOLOv8s network, which improved the YOLOv8 model by integrating the RepViT and C2f modules to enhance spatial feature extraction, and introduced the LSKNet and SPD Conv modules to improve small-object detection accuracy. Zhong et al. [9] proposed the SPD-YOLOv8 algorithm for small-object detection in complex scenes. In this algorithm, traditional convolution and pooling layers are replaced with the SPD-Conv module, and the MPDIoU loss function is used to optimize box fitting, thereby improving small-object detection accuracy. Xu et al. [10] proposed an improved YOLOv8 object detection algorithm, aiming to enhance performance in complex environments for drones through multi-scale feature fusion, conditional convolution, and Wise-IoU loss function optimization. In addition, YOLOv8 has been extensively applied in various fields such as rocket detection, circuit board defect detection, cell instance segmentation, reflective vest detection, and drone detection. Recently, Jovanovix et al. [11] utilized YOLOv8 to handle the morphological changes of rockets at different distances and angles, combining multi-scale feature fusion technology, demonstrating high robustness and real-time performance in complex backgrounds. Jovanovix et al. [12] employed a lightweight YOLOv8 model for circuit board defect detection, ensuring accuracy while reducing computational resources. Kang et al. [13] proposed the ASF-YOLO model for cell instance segmentation in overlapping cells and complex backgrounds, using multi-scale features and attention mechanisms to improve precision. Milanovic et al. [14] utilized a highly generalized pre-trained YOLOv8 model to achieve real-time detection of reflective vests under complex lighting conditions. Petrovic et al. [15] leveraged YOLOv8’s multi-scale detection module to identify small targets in complex backgrounds for drone detection, showing significant advantages. These studies demonstrate the powerful object detection capabilities of YOLOv8 and its potential for future applications in more fields.
Current research has made significant contributions to improving accuracy. However, achieving real-time monitoring remains a critical issue due to limited resources and large model sizes in small-object detection tasks. A significant amount of research has been dedicated to lightweight network models. Cao et al. [16] proposed the lightweight GCL-YOLO network, which requires fewer parameters, has less computational complexity, and accurately predicts the position and localization of high-density small targets by using the GhostConv module, a new prediction head, and the Focal EIOU loss function. Wu et al. [12] introduced SEConv, a lightweight convolution that replaces standard convolution (SC), reducing the network’s parameter count and accelerating the detection process in remote sensing images. Guo et al. [17] proposed the lightweight YOLO-SGF algorithm, which uses the GCFVoV lightweight fusion network as the neck and combines ShuffleNetV2-block1 with C2f for feature extraction, providing new ideas for lightweight networks. Du et al. [18] proposed the STD-YOLOv8 flame detection algorithm, which fuses the BiFPN bidirectional feature pyramid network and uses the NWD loss function to enhance model robustness. Guo et al. [19] proposed an underwater object detection network that uses the FasterNet module, GSConv, and PConv to ensure accuracy while being lightweight. The methods mentioned above have enhanced the original YOLO model, contributing to advancements in the domain of object detection. However, there is limited research on improving networks from both the perspectives of small target features and lightweight design. Based on this, this paper proposes the IYFVMNet model, which leverages the powerful feature representation and computational complexity reduction capabilities of the FasterNet network structure and the VoV-GSCSP module. This approach enhances detection efficiency with relatively less sacrificing the accuracy of small target detection. Additionally, the use of the P2 detection head further enriches the feature information of small targets, demonstrating excellent performance in small target detection.
It is worth noting that the hybrid approach combining metaheuristic algorithms with machine learning has recently garnered significant attention in the research community and has achieved remarkable results across various fields. For instance, Antonijevic et al. [20] employed metaheuristic algorithms such as genetic algorithms to automatically adjust key parameters of models, providing effective technical support for the security of metaverse IoT systems. Nandal et al. [21] utilized the Whale Optimization Algorithm to optimize the YOLOv8 algorithm for detecting driving behavior. Elgamily et al. [22] applied six metaheuristic optimization algorithms in combination with YOLOv7 and YOLOv8 for remote sensing object detection. This novel research field successfully integrates machine learning with swarm intelligence methods. In future research, the aim is to introduce metaheuristic algorithms to fine-tune model parameters, further enhancing the model’s performance and adaptability.
Compared with previous versions, YOLOv8 represents a new breakthrough in network architecture due to its improved accuracy and speed. To meet the needs of different scenarios, different network widths and depths have been designed. Based on different scaling factors, the network model structures, ranging from small to large, include five versions: YOLOv8n, YOLOv8s, YOLOv8m, YOLOv8l, and YOLOv8x. In particular, YOLOv8n is widely used due to its low parameter and calculation requirements. The overall structure of YOLOv8 is composed of three main components: the backbone network, the neck network, and the head network. These three parts are respectively used for feature extraction, feature fusion, and detection. Each part has its own responsibilities in terms of specific functions and design optimization. The network structure is illustrated in Figure 1.
The left side of Figure 1 illustrates YOLOv8’s backbone network, which is responsible for extracting features from the input image. The backbone network replaces YOLOv5’s C3 module with the C2f module, improving gradient flow and reducing the network’s weight. The cross stage partial (CSP) mechanism is used to divide the feature map into two parts for processing. One part is used for residual connections, while the other is employed for feature fusion, enhancing feature expression and reducing computational load. The structure consists of multiple stacked Conv modules and C2f modules. The SPPF module is preserved in the backbone network, mapping the input feature map to multiple fixed-size feature spaces through multi-scale pooling, allowing the network to process images of varying sizes.
The middle section of the network structure diagram represents the neck network, which fuses features of different scales extracted by the backbone network. This part is composed of Path Aggregation Network (PANet) [23], which includes Feature Pyramid Network (FPN) [24]. First, features are passed upwards layer by layer through an upsampling operation, facilitating the fusion of low-level and high-level feature maps. Second, features are passed down layer by layer using downsampling operations, merging high-level and low-level feature maps. Finally, horizontal connections between paths are established to boost performance. The fusion of features at different scales through these three paths enhances small-object detection.
The right section of the network structure diagram represents the head network, responsible for the final detection stage. The head has multiple convolutional layer branches. The first classification branch is responsible for predicting the object category probability; the second bounding box regression branch is responsible for predicting the bounding box position and the third is a confidence branch, used to predict the confidence. By combining these three parts, an anchor-free design is used to simplify the detection process. In addition, by predicting the center point, height, and width, high-precision object detection is achieved.
YOLOv8 utilizes multiple loss functions to optimize accuracy, including classification loss, bounding box regression loss, and distribution focal loss (DFL).
In the classification loss component, YOLOv8 employs binary cross-entropy (BCE) loss, calculated as Equation 1:
where y denotes the true label and p denotes the predicted probability.
For bounding box regression, YOLOv8 employs the complete intersection over union (CIoU) as the loss function. Compared to traditional IoU, CIoU considers the overlapping region, the distance between box centers, and the aspect ratio, aiming to enhance the convergence speed and accuracy of bounding box predictions. The CIoU loss function, LCIoU is calculated as Equation 2:
where Bgt denotes the center point of ground truth box, Bprd denotes the center point of the prediction box, ρ(Bgt, Bprd) represents the Euclidean distance between the center point of ground truth box and prediction box, and C is the diagonal length of the concatenated region formed by the two rectangular boxes. V is used to compute the difference in aspect ratio between the two rectangular boxes calculated as Equation 3, while α is a weighting factor, calculated as follows Equation 4:
DFL is introduced to further enhance the precision of bounding box regression. DFL directs the model’s focus towards more challenging samples by adjusting the loss based on the prediction distribution and the ground truth box distribution. DFL is calculated as follows Equation 5:
where pt is the predicted probability value and γ is a moderation factor.
The final loss function in YOLOv8 is the weighted sum of the CIoU, BCE, and DFL components, expressed as Equation 6:
To balancing detection accuracy and speed in small-object detection, an improved network structure, IYFVMNet, is introduced as an enhancement of YOLOv8. The C2f-faster architecture of the model uses FasterNet to improve the C2f bottleneck structure and PConv to improve the conventional convolution, which enhance the diversity of feature representation while maintaining efficient computation and utilizing the information of all channels. Second, The slim-neck design of the model incorporates the VoV-GSCSP module, built on GSConv, to refine the neck network by integrating SC and depthwise separable convolution (DSC). The design has strong feature extraction and feature fusion capabilities while significantly minimizing parameters and computation. Additionally, MPDIoU is used for model traing. This addressed the problem of prediction boxes and ground truth boxes having the same aspect ratio but differing in width and height. Finally, the LAMP algorithm is employed to achieve network slimming by incorporating a scaling factor within the batch normalization layer. The network design is illustrated in Figure 2.
FasterNet [25], a new network structure based on PConv, replaces the C2f bottleneck structure, and is displayed in Figure 3. The network structure consists of one PConv layer and two pointwise convolutions (PWConv), which together form the residual structure. The structural features of the PConv layer enable FasterNet to maintain high accuracy while being much faster than other networks, being crucial for boosting the performance of various visual tasks.
PConv is an efficient feature extraction technique, which selectively processes input channels, thus decreasing computational cost and memory consumption. The PConv module also enhances feature representation, making it richer and more robust, as illustrated in Figure 4. Compared to conventional convolution, PConv exhibits selectivity in channel volume, performing calculations only on a subset of input channels, which results in reduced computational complexity.
VoV-GSCSP [26], a multi-scale partial network module, is used to optimize the neck network structure in YOLOv8n by incorporating VoVNet and GSConv, which enhance learning capability through multi-scale feature fusion and cross-scale connections. The VoV-GSCSP module is composed of multiple GSConv layers and feature aggregation operations, enriching and strengthening feature robustness through feature fusion at different scales. A structural diagram of VoV-GSCSP is illustrated in Figure 5.
The GS bottleneck module includes GSConv, which reduces the computational cost by decreasing the amount of feature map channels and maintaining effective feature representation. GSConv is a new lightweight convolution technique that combines the benefits of SC and DSC, achieved with a “shuffle” operation in the network. GSConv first generates channel-dense feature maps using SC, and then evenly mixes this feature information into each section of the sparse feature maps created by DSC through the shuffle operation. This mixing strategy allows the output feature maps of DSC to be as close as possible to the feature maps of SC, significantly improving the accuracy of the model while keeping the computational cost low. The GS includes a GSConv layer for feature extraction and channel expansion, followed by a convolution layer for channel compression. This design reduces network parameters and computation, illustrated in Figure 6.
To optimize bounding box detection, a loss function is essential. YOLOv8 uses a CIoU-based loss function. This function takes into account both the center point distance and aspect ratio when aligning the predicted box with the ground truth box, which has been shown to have strong performance [27]. However, it measures the aspect ratio in relative terms. When the height and width values are inconsistent, the CIoU loss function will lose its effectiveness. To address this issue, Intersection over Union with Minimum Points Distance (MDPIoU) [28] was designed to address this limitation, which is calculated as Equation 7:
where w and h denote the width and height of the input image, respectively,
where
where
MDPIoU, as a bounding box loss function, is defined as follows Equation 10:
Compared to CIoU, MDPIoU introduces a new method that uses the distance between two points to measure similarity, which simplifies the computational process regardless if the bounding boxes overlap.
The Layer-wise Adaptive Momentum Pruning (LAMP) [29] method is a pruning approach for neural networks, which considers the differences in weight magnitudes across layers and reduces the model’s parameters and computational complexity, while simultaneously maintaining or enhancing its accuracy. Compared to other compression methods, LAMP is more effective at reducing redundancy and preserving key feature information, which makes it very useful in the field of network compression. The essence of the LAMP approach is the adaptive strategy to pruning, which is based on customizing the pruning rate to the weight magnitudes within each layer instead of the entire network and sorting from high to low. LAMP uses the strength of each weight in all layers to decide which weights to keep and which to remove. This is a major improvement over traditional pruning techniques, in that LAMP focuses on preserving weights that are important for the model’s predictions and reducing the drop in performance that usually happens during pruning. This can be summarized into two main steps.
Step 1. Calculate the pruning threshold. For each layer l, first calculate the absolute value of all weights and determine the pruning threshold Γl based on the set pruning ratio pl calculated as Equation 11:
where
Step 2. Perform the pruning. Set all weights with an absolute value less than the threshold Γl in layer l to zero.
In this way, LAMP can effectively identify weights that have a minimal impact on model performance and prune. The pruned network is then fine-tuned to restore or improve the model’s accuracy.
The experimental environment was set up with the an Intel(R) Xeon(R) Platinum 8358P CPU, and an RTX 3090 (24 GB) GPU. All experiments were conducted under consistent conditions using Python 3.8 and PyTorch 1.10.0 to ensure fairness. The experimental parameters were set based on literature [10]. The specific experimental parameters are provided in Table 1.
TinyPerson is an open image dataset focused on small-object detection. The dataset includes annotated images from various natural scenes and lighting conditions, designed to test an algorithm’s small-object detection and model generalization under occlusion and varying object densities. Images were extracted manually every 50 frames, for two specific categories: people in ocean and earth-based scenes, denoted “sea_persons” and “earth_persons,” respectively [30]. The images contain small objects, all smaller than 20 pixels. The dataset comprises 794 training images and 816 validation images, totaling 1,610. The example of dataset is shown in Figure 7.
To access the model comprehensively, the precision (P), recall (R), and mean average precision (mAP) among other metrics are used.
Precision quantifies the ratio of true positives to total positive predictions calculated as Equation 12:
Recall represents the ratio of true positives to all actual positive instances calculated as Equation 13:
mAP represents the average of AP values across all categories, with AP defined as the area under the precision-recall (P-R) curve. A higher AP score reflects superior model performance.
Firstly, to determine which YOLOv8 model structure to adopt as the baseline model, experiments were conducted on the TinyPerson dataset using five structures: YOLOv8n, YOLOv8s, YOLOv8m, YOLOv8l, and YOLOv8x. The BoxLoss, ClaLoss, DflLoss, and TotalLoss were calculated for each model, and the convergence process was visually represented, as shown in Figure 8. YOLOv8n is represented in blue, YOLOv8s in orange, YOLOv8m in green, YOLOv8l in red, and YOLOv8x in purple. Figure 8a shows the comparison of BoxLoss, Figure 8b shows the comparison of ClaLoss, Figure 8c shows the comparison of DflLoss, and Figure 8d shows the comparison of TotalLoss. The results of each module concerning parameter quantity, computational complexity (GFLOPs), and detection performance metrics are shown in Table 2.
Figure 8. Comparison of Training Loss of the YOLOv8 series models. (a) BoxLoss, (b) ClsLoss, (c) DflLoss, (d) Total Loss.
From the results shown in Figure 8 and Table 2, it can be observed that YOLOv8n, as the most lightweight model, achieves relative convergence around 50 epochs in various loss calculations, particularly in BoxLoss, with the fastest convergence speed. However, this comes at the cost of reduced accuracy. Since this study primarily focuses on lightweight design for model selection, YOLOv8n was chosen as the baseline model for experiments to meet the requirements of high efficiency in real-time devices.
The backbone network’s performance greatly influences the algorithm’s overall effectiveness. To illustrate the effectiveness of C2f-faster, five different backbone network modules were selected for comparative experiments: C2f-faster, Adaptive Convolution (AKConv) [31], Receptive Field Attention Convolution (RFAConv) [32], Spatial and Channel Reconstruction Convolution (SCcConv) [33], and All-dimensional Dynamic Convolution (ODConv) [34]. The results for each module in term of parameter quantity, computational complexity (GFLOPs), and detection performance metrics are shown in Table 2. The convergence process is visually represented in Figure 9. C2f is shown in blue, C2f-faster in orange, AKConv in green, RFAConv in red, SCcConv in purple, and ODConv in brown.
Figure 9. Comparison of Training Loss of backbone network. (a) BoxLoss, (b) ClsLoss, (c) DflLoss, (d) Total Loss.
As shown in Table 3, the C2f-faster module offers notable benefits in terms of parameter count and computational complexity, featuring 2,300,838 parameters and 6.3 GFLOPs. The accuracy and recall of C2f-faster are slightly lower than those of the best-performing RFAConv and SCcConv modules, but it uses 82,000 and 33,000 fewer parameters, respectively. Moreover, its computational complexity is cut down by one to two GFLOPs. In comparison to the AKConv and ODConv modules, the amounts of GFLOPs are similar, but the C2f-faster module uses 23,000 and 77,000 fewer parameters, and has an improved accuracy of 2.0% and 1.5%, respectively. From Figure 9, it can be observed that the improved model exhibits a similar trend in Loss across different modules. However, the BoxLoss converges the fastest at around 50 epochs, albeit at the cost of some accuracy. These results indicate that the structure can leverage global information to capture detailed features. From the perspective of balancing precision and efficiency, C2f-faster is the more suitable choice as it demonstrates outstanding computational efficiency.
Building upon the backbone network improvements, this section further validates the performance enhancement of slim-neck. This structure is used in object detection tasks and is referred to as C2f-faster-lightweight. This structure was compared with four other neck network structures: Global Feature Pyramid Network (GFPN) [35], High-level Screening-feature Fusion Pyramid Networks (HS-FPN) [36], Asymptotic Feature Pyramid Network (AFPN-P345) [37], and Bidirectional Feature Pyramid Network (BiFPN) [38]. Table 4 presents the results of the neck network modules, including parameter count, computational complexity (GFLOPs), and detection performance metrics. The convergence process is visually represented in Figure 10. C2f-faster-lightweight is shown in blue, C2f-faster-GFPN in orange, C2f-faster-HSFPN in green, C2f-faster-AFPN-P345 in red, and C2f-faster-BiFPN in purple.
Figure 10. Comparison of Training Loss of neck network. (a) BoxLoss, (b) ClsLoss, (c) DflLoss, (d) Total Loss.
According to the data displayed in Table 4, the C2f-faster-lightweight module requires 2,512,310 parameters and 6.4 GFLOPs. Both metrics are at moderate levels but demonstrate good computational efficiency. Compared to C2f-faster-GFPN, C2f-faster-HSFPN, C2f-faster-AFPN-P345, and C2f-faster-BiFPN, the C2f-faster-lightweight module achieved the highest accuracy, being higher than the other modules by 3.2%, 3.7%, 1.8%, and 3.3%, respectively. Compared to C2f-faster-HSFPN, which has the lowest computational requirements, YOLOv8-lightweight, which requires slightly more computations, has significantly better detection performance. Compared to GFPN (8.3 GFLOPs) and BiFPN (7.1 GFLOPs), YOLOv8-lightweight exhibits higher detection precision while having a similar computational complexity. From Figure 10, it can be observed that the C2f-faster-lightweight model exhibits faster convergence in BoxLoss, demonstrating its strong capability in predicting bounding boxes. Overall, the C2f-faster-lightweight module performs excellently across the various metrics.
The traditional IoU loss function has some shortcomings when dealing with detection box regression tasks. This section aims to analyze the performance of the MDPIoU3 loss function in YOLOv8, by comparing it with the commonly used DIoU [39], EIoU [40], and SIoU [41] loss functions. Table 5 presents the results of different loss functions across various object detection tasks.
As shown in Table 5, the MDPIoU3 loss function demonstrates advantages in precision (P), recall (R), and mean average precision at an IoU threshold of 0.50 (mAP50) and across thresholds of 0.5–0.95 (mAP50-95). Due to the further optimization in scale and position regression, the MDPIoU3 loss function is better at balancing precision and recall. MDPIoU3 outperforms the other loss functions, enhancing model performance and demonstrating greater practicality.
The LAMP method was used for network pruning. Table 6 shows the model’s performance variations at different pruning rates, including metrics such as the number of parameters, GFLOPs, precision (P), recall (R), mAP50, and mAP50-95.
From Table 6, it can be observed that when the pruning rate exceeds 1.7, the model’s performance declines significantly. At a pruning rate of 1.6, the model achieves the highest precision (0.463) and recall (0.3), while mAP50 and mAP50-95 are 0.293 and 0.118, respectively. The number of model parameters reduced to 1,563,194, and the amount of GFLOPs decreased to 5.8. These results demonstrate optimal overall performance while maintaining a lower computational load. Compared to the other pruning rates, the 1.6 pruning rate is the most effective to reduce the complexity of the model significantly without sacrificing performance.
IThis section presents an ablation study of the four proposed improvements, assessing the contribution of each to the model’s performance. The improvements—C2f-faster, slim-neck-P2, MDPIoU, and LAMP pruning strategy—were incorporated sequentially and are labeled as A, B, C, and D, respectively. The baseline model is YOLOv8n. All ablation experiments were conducted based on this baseline model, and these improvements were gradually introduced. Table 7 presents the results of the ablation experiments.
As shown in Table 7, incorporating C2f-faster (A) significantly reduces the model’s parameters and computational cost, but leads to a decrease in accuracy and recall. On the other hand, slim-neck-P2 (B) enhances the model’s performance overall, particularly in recall (increasing to 0.317) and mAP50 (improving from 0.286 to 0.307). These results show that slim-neck-P2 reduces the model size while capturing multi-scale features more effectively. Using C2f-faster with slim-neck-P2 (A + B) makes the model lighter and faster. Adding MDPIoU (C) does not change the outcome much, but when combining it with A + B, the recall improves to 0.307 and mAP50 improves to 0.298, meaning the model performs better overall. The addition of the LAMP pruning strategy (D) slightly reduces the mAP50 and mAP50-95 scores, but it also lowers the accuracy and recall rates. This suggests that LAMP pruning can make the model lighter without significantly harming the entire performance. Overall, each enhancement module optimizes the model’s efficiency while allowing it to maintain good performance, which demonstrates the viability and practicality of these improvements.
To further validate the effectiveness of the proposed network, the Guo Net [19], YOLO-SGF [17], YOLO-SE [42], and TRC-YOLO [43] models were selected for comparison. The results are provided in Table 8.
As shown in Table 8, the improved IYFVMNet, model presented in this paper outperforms the other models across various indicators. Despite the reduction in the number of parameters, the accuracy remains high, demonstrating the network’s strong feature representation capability. Overall, the improved model presented in this paper outperforms the other algorithms across various performance indicators.
The heat map shows the attention distribution of the model during target detection. Select two maps to display the heat map, as shown in Figure 11. It can be seen that the key areas of the heat map display are concentrated on small target people, indicating that the model can effectively capture image features.
To assess the performance of the enhanced YOLOv8 model in small-object detection, two images from the test set were chosen for visual analysis, as shown in Figure 12.
In Figure 12, the original YOLOv8 model detected only seven earth_persons and mistakenly identified one sea_person in the first image, whereas the improved IYFVMNet model successfully detected 12 earth_persons with no false sea_person detections. This indicates that the enhanced model achieves a higher recall rate, making it more effective at detecting small objects within a scene. Moreover, the refined model demonstrates superior precision, with more accurate object localization and a decrease in the false detection rate. For the second image, there are numerous small objects. The baseline model identified 10 earth_persons and 62 sea_persons, while the improved model detected 11 earth_persons and 63 sea_persons. The total counts are similar, but the enhanced model shows more consistent performance in this complex scenario, with fewer misidentifications and a slight improvement in recall rate. Overall, the improved model has bolstered overall detection capabilities relative to the other models.
The utilization of drones for detecting people in myriad scenes has become an essential component of contemporary search-and-rescue frameworks. In this context, studies of the real-time detection of small-person objects can greatly boost the efficiency and success rate of rescue missions. This paper presents IYFVMNet, an enhanced and lightweight detection network based on the YOLOv8 model. It achieved an accuracy of 46.3% on the TinyPerson dataset, with a recall rate of 30%, mAP50 of 29.3%, and mAP50-95 of 11.8%. The model achieves higher accuracy while using only half the number of parameters compared to the original model. Regarding the balance between computational complexity and precision, the model is more appropriate for environments with constrained resources than other improved models.
The proposed IYFVMNet model has significant policy implications, particularly in the context of search-and-rescue operations and disaster management. By enabling accurate and efficient detection of small objects, such as individuals in distress, the model can significantly enhance the effectiveness of rescue missions, potentially saving lives in critical situations.
Despite the promising results achieved by the proposed model, there are still several limitations that need to be addressed in future research. From a theoretical perspective, the model’s performance remains constrained by the inherent challenges of small-object detection. For instance, the limited feature extraction capabilities make it difficult to fully capture and represent object information. From a practical standpoint, deploying the model on resource-constrained devices continues to pose significant challenges. Additionally, the diversity and quality of the dataset remain crucial factors influencing the model’s performance. The TinyPerson dataset used in this study cannot fully represent real-world scenarios, such as extreme weather conditions affected by climate changes.
Future research will be aimed at refining the feature extraction and detection mechanisms to enhance the model’s efficiency. Additionally, the goal is to enable faster detection on machines with limited resources, providing rescue teams with precise locations of individuals in need. Another promising direction is the integration of metaheuristic optimization algorithms to fine-tune the model’s hyperparameters, thereby improving both accuracy and computational efficiency. Future research will focus on these aspects and explore new methods to enhance the model’s performance and applicability. This would significantly enhance the safety and effectiveness of rescue missions.
The original contributions presented in the study are included in the article/supplementary material, further inquiries can be directed to the corresponding author.
FY: Conceptualization, Formal Analysis, Investigation, Methodology, Project administration, Resources, Validation, Visualization, Writing – original draft. LP: Conceptualization, Data curation, Methodology, Resources, Software, Validation, Visualization, Writing – review and editing. HC: Funding acquisition, Methodology, Software, Supervision, Validation, Writing – review and editing. LZ: Data curation, Investigation, Methodology, Project administration, Visualization, Writing – review and editing.
The author(s) declare that financial support was received for the research and/or publication of this article. The authors acknowledge funding received from the following science foundations: Research on Multi Agent Parallel Simulation Model for Large Scale Complex Systems Based on Mesoscale Theory (No. 202203021221145).
Author LZ was employed by Shanxi Intelligent Transportation Institute Co., Ltd.
The remaining author declares that the research was conducted in the absence of any commercial or financial relationships that could be construed as a potential conflict of interest.
The author(s) declare that no Generative AI was used in the creation of this manuscript.
All claims expressed in this article are solely those of the authors and do not necessarily represent those of their affiliated organizations, or those of the publisher, the editors and the reviewers. Any product that may be evaluated in this article, or claim that may be made by its manufacturer, is not guaranteed or endorsed by the publisher.
1. Yang YH, Miao ZL, Zhang H, Wang B, Wu L. Lightweight attention-guided YOLO with level set layer for landslide detection from optical satellite images. IEEE J Selected Top Appl Earth Observations Remote Sensing (2024) 17:3543–59. doi:10.1109/jstars.2024.3351277
2. Cheng G, Yuan X, Yao X, Yan K, Zeng Q, Xie X, et al. Towards large-scale small object detection: survey and benchmarks. IEEE Trans Pattern Anal Machine Intelligence (2023) 45(11):13467–88. doi:10.1109/tpami.2023.3290594
3. Zhao C, Liu RW, Qu J, Gao R. Deep learning-based object detection in maritime unmanned aerial vehicle imagery: review and experimental comparisons. Eng Appl Artif Intelligence (2024) 128:107513. doi:10.1016/j.engappai.2023.107513
4. Hussain M. Yolov1 to v8: unveiling each variant–a comprehensive review of yolo. IEEE Access (2024) 12:42816–33. doi:10.1109/access.2024.3378568
5. Vijayakumar A, Vairavasundaram S. Yolo-based object detection models: a review and its applications. Multimedia Tools Appl (2024) 83(35):83535–74. doi:10.1007/s11042-024-18872-y
6. Varghese REJIN, Sambath M. YOLOv8: a novel object detection algorithm with enhanced performance and robustness. In: 2024 international conference on advances in data engineering and intelligent computing Systems (ADICS), 18–19 April 2024; Chennai, India. IEEE. (2024). p. 1–6.
7. Gunawan F, Hwang CL, Cheng ZE. ROI-YOLOv8-Based far-distance face-recognition. In 2023 international conference on advanced robotics and intelligent Systems (ARIS). IEEE (2023). p. 1–6.
8. Zhao R, Tang SH, Supeni EEB, Rahim SA, Fan L. Z-YOLOv8s-based approach for road object recognition in complex traffic scenarios. Alexandria Eng J (2024) 106:298–311. doi:10.1016/j.aej.2024.07.011
9. Zhong R, Peng E, Li Z, Ai Q, Han T, Tang Y. SPD-YOLOv8: an small-size object detection model of UAV imagery in complex scene. The J Supercomputing (2024) 80(12):17021–41. doi:10.1007/s11227-024-06121-w
10. Xu LY, Zhao YF, Zhai YH, Huang L, Ruan C. Small object detection in UAV images based on YOLOv8n. Int J Comput Intelligence Syst (2024) 17(1):223–35. doi:10.1007/s44196-024-00632-3
11. Jovanovic L, Antonijevic M, Perisic J, Milovanovic M, Miodrag Z, Budimirovic N, et al. Computer vision based areal photographic rocket detection using yolov8 models. Int J Robotics Automation Technology (2024) 11:37–49. doi:10.31875/2409-9694.2024.11.03
12. Jovanovic L, Jokic A, Milovanovic M, Antonijevic M, Zivkovic M, Bacanin N, et al. Exploring lightweight YOLOv8 architectures for circuit board defect detection. In: 2024 international conference on intelligent computing and emerging communication technologies (ICEC). IEEE (2024). p. 1–6.
13. Kang M, Ting CM, Ting FF, Phan RCW. ASF-YOLO: a novel YOLO model with attentional scale sequence fusion for cell instance segmentation. Image Vis Comput (2024) 147:105057. doi:10.1016/j.imavis.2024.105057
14. Milanovic A, Jovanovic L, Zivkovic M, Bacanin N, Cajic M, Antonijevic M, et al. Exploring pre-trained model potential for reflective vest real time detection with yolov8 models. In: 2024 3rd international conference on applied artificial intelligence and computing (ICAAIC). 05-07 June 2024; Salem, India. IEEE. (2024). p. 1210–6.
15. Petrovic A, Bacanin N, Jovanovic L, Cadjenovic J, Kaljevic J, Zivkovic M, et al. Computer-vision unmanned aerial vehicle detection system using yolov8 architectures. Int J Robotics Automation Technology (2024) 11:1–12. doi:10.31875/2409-9694.2024.11.01
16. Cao J, Bao W, Shang H, Yuan M, Cheng Q. GCL-YOLO: a GhostConv-based lightweight YOLO network for uav small object detection. Remote Sens. (2023) 15(20):4932. doi:10.3390/rs15204932
17. Guo C, Ren K, Chen Q. YOLO-SGF: lightweight network for object detection in complex infrared images based on improved YOLOv8. Infrared Phys and Technology (2024) 142:105539. doi:10.1016/j.infrared.2024.105539
18. Du H, Li Q, Guan Z, Zhang H, Liu Y. An improved lightweight YOLOv8 network for early small flame target detection. Processes (2024) 12(9):1978. doi:10.3390/pr12091978
19. Guo A, Sun K, Zhang Z. A lightweight YOLOv8 integrating FasterNet for real-time underwater object detection. J Real-Time Image Process (2024) 21(2):49–62. doi:10.1007/s11554-024-01431-x
20. Antonijevic M, Zivkovic M, Jovicic MD, Nikolic B, Perisic J, Milovanovic M, et al. Intrusion detection in metaverse environment internet of things systems by metaheuristics tuned two level framework. Scientific Rep (2025) 15(1):3555. doi:10.1038/s41598-025-88135-9
21. Nandal P, Pahal S, Sharma T, Omesh O. Real-time driver drowsiness detection using YOLOv8 with Whale optimization algorithm. SAE Int J Transportation Saf (2025) 13(09-13-01-0001). doi:10.4271/09-13-01-0001
22. Elgamily KM, Mohamed MA, Abou-Taleb AM, Ata MM. Enhanced object detection in remote sensing images by applying metaheuristic and hybrid metaheuristic optimizers to YOLOv7 and YOLOv8. Scientific Rep (2025) 15(1):7226. doi:10.1038/s41598-025-89124-8
23. Liu S, Qi L, Qin H, Shi J, Jia J. Path aggregation network for instance segmentation. In: Proceedings of the IEEE conference on computer vision and pattern recognition. 18-23 June 2018; Salt Lake City, UT, USA. IEEE. (2018). p. 8759–68.
24. Lin TY, Dollár P, Girshick R, He K, Hariharan B, Bolongie S. Feature pyramid networks for object detection. In: Proceedings of the IEEE conference on computer vision and pattern recognition (2017). p. 2117–25.
25. Chen J, Kao S, He H, Zhuo W, Wen S, Lee C, et al. Run, don't walk: chasing higher FLOPS for faster neural networks. In: Proceedings of the IEEE/CVF conference on computer vision and pattern recognition (2023). p. 12021–31.
26. Li HL, Li J, Wei HB, Liu Z, Zhan ZF, Ren QL, et al. Slim-neck by GSConv: a better design paradigm of detector architectures for autonomous vehicles. arXiv preprint arXiv:2206.02424 (2022) 10. doi:10.48550/arXiv.2206.02424
27. Zheng ZH, Wang P, Ren DW, Liu W, Ye R, Hu Q, et al. Enhancing geometric factors in model learning and inference for object detection and instance segmentation. IEEE Trans Cybernetics (2022) 52(8):8574–86. doi:10.1109/tcyb.2021.3095305
28. Ma S, Xu Y. MPDIoU: a loss for efficient and accurate bounding box regression. arXiv preprint arXiv:2307.07662 (2023). doi:10.48550/arXiv.2307.07662
29. Lee J, Park S, Mo S, Ahn S, Shin J. Layer-adaptive sparsity for the magnitude-based pruning. (2020). Available online at: https://arxiv.org/abs/2010.07611. (Acceseed April, 02, 2025).
30. Yu XH, Gong YQ, Jiang N, Ye Q, Han Z. Scale match for tiny person detection. In: 2020 IEEE winter conference on applications of computer vision (WACV). Piscataway: IEEE (2020). p. 1257–65.
31. Zhang X, Song YZ, Song TT, Yang DG, Ye YC, Zhou J, et al. AKConv: convolutional kernel with arbitrary sampled shapes and arbitrary number of parameters. arXiv preprint arXiv:2311.11587 (2023). doi:10.48550/arXiv.2311.11587
32. Zhang X, Liu C, Yang D, Son T, Ye T, Li K, et al. RFAConv: innovating spatial attention and standard convolutional operation. arXiv preprint arXiv:2304.03198 (2023). doi:10.48550/arXiv.2304.03198
33. Li J, Wen Y, He L. Scconv: spatial and channel reconstruction convolution for feature redundancy. In: Proceedings of the IEEE/CVF conference on computer vision and pattern recognition (2023). p. 6153–62.
34. Li C, Zhou A, Yao A. Omni-dimensional dynamic convolution. arXiv preprint arXiv:2209.07947 (2022). doi:10.48550/arXiv.2209.07947
35. Cocias T, Razvant A, Grigorescu S. GFPNet: a deep network for learning shape completion in generic fitted primitives. IEEE Robotics Automation Lett (2020) 5(3):4493–500. doi:10.1109/lra.2020.3000851
36. Chen Y, Zhang C, Chen B, Huang Y, Sun Y, Wang C, et al. Accurate leukocyte detection based on deformable-DETR and multi-level feature fusion for aiding diagnosis of blood diseases. Comput Biol Med (2024) 170:107917. doi:10.1016/j.compbiomed.2024.107917
37. Yang G, Lei J, Zhu Z, Cheng S, Feng Z, Liang R. AFPN: Asymptotic feature pyramid network for object detection. In: 2023 IEEE international conference on Systems, man, and cybernetics (SMC). IEEE (2023). p. 2184–9.
38. Zhu L, Deng ZJ, Hu XW, Fu CW, Xu XW, Qin J, et al. Bidirectional feature pyramid network with recurrent attention residual modules for shadow detection. In: Proceedings of the European conference on computer vision. Springer, Cham: ECCV (2018). p. 121–36.
39. Zheng Z, Wang P, Liu W, Li J, Ye R, Ren D. Distance-IoU loss: faster and better learning for bounding box regression. Proc AAAI Conf Artif intelligence (2020) 34(07):12993–3000. doi:10.1609/aaai.v34i07.6999
40. Zhang YF, Ren W, Zhang Z, Jia Z, Wang L, Tan T. Focal and efficient IOU loss for accurate bounding box regression. Neurocomputing (2022) 506:146–57. doi:10.1016/j.neucom.2022.07.042
41. Gevorgyan Z. SIoU loss: more powerful learning for bounding box regression. arXiv preprint arXiv:2205.12740 (2022). doi:10.48550/arXiv.2205.12740
42. Wu T, Dong Y. YOLO-SE: improved YOLOv8 for remote sensing object detection and recognition. Appl Sci (2023) 13(24):12977. doi:10.3390/app132412977
Keywords: small object detection, YOLOv8 algorithm, FasterNet, vovnet gsconv, LAMP
Citation: Yang F, Pan L, Cui H and Zhang L (2025) An improved lightweight tiny-person detection network based on YOLOv8: IYFVMNet. Front. Phys. 13:1553224. doi: 10.3389/fphy.2025.1553224
Received: 30 December 2024; Accepted: 27 March 2025;
Published: 22 April 2025.
Edited by:
Milos Antonijevic, Singidunum University, SerbiaReviewed by:
Nebojsa Bacanin, Singidunum University, SerbiaCopyright © 2025 Yang, Pan, Cui and Zhang. This is an open-access article distributed under the terms of the Creative Commons Attribution License (CC BY). The use, distribution or reproduction in other forums is permitted, provided the original author(s) and the copyright owner(s) are credited and that the original publication in this journal is cited, in accordance with accepted academic practice. No use, distribution or reproduction is permitted which does not comply with these terms.
*Correspondence: Fan Yang, eWFuZ2ZhbkB0eXVzdC5lZHUuY24=
Disclaimer: All claims expressed in this article are solely those of the authors and do not necessarily represent those of their affiliated organizations, or those of the publisher, the editors and the reviewers. Any product that may be evaluated in this article or claim that may be made by its manufacturer is not guaranteed or endorsed by the publisher.
Research integrity at Frontiers
Learn more about the work of our research integrity team to safeguard the quality of each article we publish.