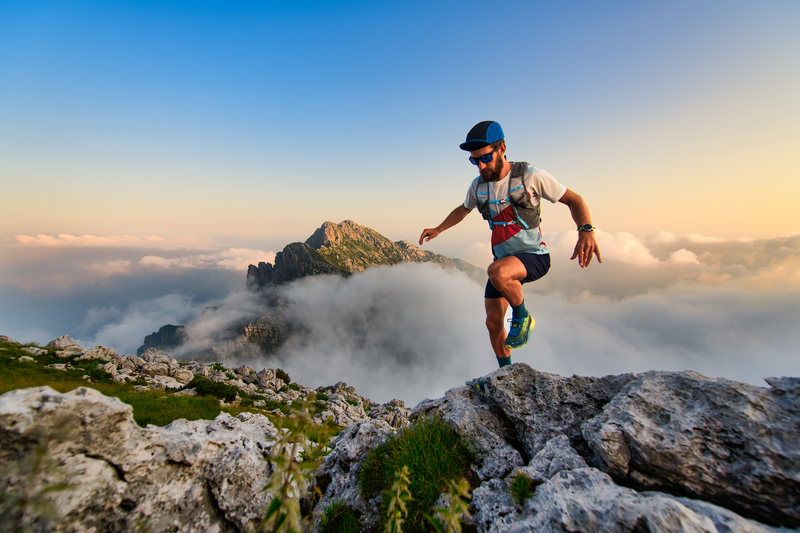
94% of researchers rate our articles as excellent or good
Learn more about the work of our research integrity team to safeguard the quality of each article we publish.
Find out more
ORIGINAL RESEARCH article
Front. Phys.
Sec. Social Physics
Volume 13 - 2025 | doi: 10.3389/fphy.2025.1551215
The final, formatted version of the article will be published soon.
You have multiple emails registered with Frontiers:
Please enter your email address:
If you already have an account, please login
You don't have a Frontiers account ? You can register here
With the advent of modern media platforms, the dissemination of information has become faster and more far-reaching than ever before. These platforms amplify susceptibility to societal influences, as individuals respond to widely circulating information while being shaped by the perspectives of those around them. By aligning with others' opinions, individuals contribute to establishing shared norms through both macroscopic and microscopic influences. This paper explores the roles of these influences -social conformity at the population-wide level and peer pressure at the localized level -in shaping opinion dynamics within today's informationrich environment. Building on the Hegselmann-Krause opinion dynamics model, we introduce modifications to incorporate peer pressure through three modes of dynamic social circles.While conformity and peer pressure have been studied previously, we focus specifically on how properties and behaviors evolve in an opinion-dependent manner from both individual and social circle perspectives. Furthermore, we incorporate radical agents, characterized by resistance to influence. However, unlike radicals as referred to the stubborn agents commonly discussed in previous literature, our radical agents adjust their behavior based on their position in the opinion spectrum rather than remaining entirely fixed in their views. Simulations reveal that removing agents with distant opinions from social circles facilitates population convergence more than adding agents with similar opinions. Our model suggests that increasing the number of agents within social circles accelerates opinion shifts. Similarly, broadening confidence bounds fosters cluster formation. In addition, a higher initial number of radical agents makes it more likely for non-radical agents to adopt radical views. Likewise, when agents are influenced more by macroscopic rather than microscopic forces, radicalization becomes more likely. Together, one or more of these dynamics drive convergence between radical and non-radical agents.
Keywords: opinion dynamics, Social Conformity, peer pressure, Bounded Confidence Model, agent-based simulation
Received: 25 Dec 2024; Accepted: 10 Mar 2025.
Copyright: © 2025 Shibata and Okada. This is an open-access article distributed under the terms of the Creative Commons Attribution License (CC BY). The use, distribution or reproduction in other forums is permitted, provided the original author(s) or licensor are credited and that the original publication in this journal is cited, in accordance with accepted academic practice. No use, distribution or reproduction is permitted which does not comply with these terms.
* Correspondence:
Kanako Shibata, Meiji University, Suginami, Japan
Disclaimer: All claims expressed in this article are solely those of the authors and do not necessarily represent those of their affiliated organizations, or those of the publisher, the editors and the reviewers. Any product that may be evaluated in this article or claim that may be made by its manufacturer is not guaranteed or endorsed by the publisher.
Research integrity at Frontiers
Learn more about the work of our research integrity team to safeguard the quality of each article we publish.