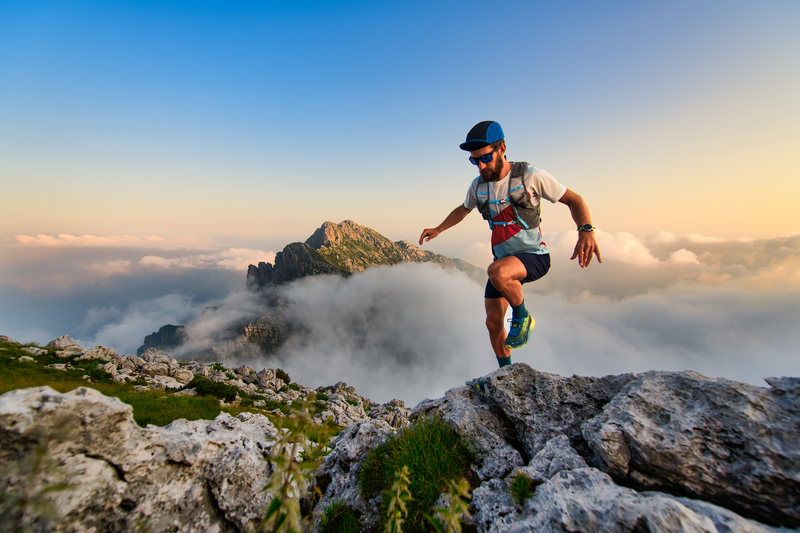
94% of researchers rate our articles as excellent or good
Learn more about the work of our research integrity team to safeguard the quality of each article we publish.
Find out more
REVIEW article
Front. Phys.
Sec. Quantum Engineering and Technology
Volume 13 - 2025 | doi: 10.3389/fphy.2025.1551209
The final, formatted version of the article will be published soon.
You have multiple emails registered with Frontiers:
Please enter your email address:
If you already have an account, please login
You don't have a Frontiers account ? You can register here
Its complexity constrains advancements in fusion energy and high energy applications driven by plasma physics, multiscale phenomena beyond classical computing limits. These transformative solutions, especially in plasma simulations, for which exponential speedup is possible, represent significant promise toward breakthroughs in sustainable energy and extreme state studies. In this review, Quantum Computing (QC) is explored as a means to drive plasma physics simulations forward by providing applications such as fusion energy and high-energy systems. This includes computational methods for simulating turbulence, wave-particle interactions, and Magnetohydrodynamic (MHD) instabilities that have near-quantum efficiency. We show that by integrating QC into plasma research, one can solve large-scale linear equations, compute eigenvalues, and optimize complex systems, performing better than classical methods. This discussion examines the potential of quantum computing for plasma physics, highlighting its current limitations, including hardware constraints and the need for specialized algorithms tailored to model complex plasma phenomena accurately. These challenges notwithstanding, QC has the potential to dramatically change plasma modeling and expedite the development of fusion reactors. QC represents a new approach to engineer away computational bottlenecks, providing unprecedented views of plasma behavior needed for sustainable energy breakthroughs. The results from this work underscore the continued importance of looking outside of plasma physics to realize QC's full potential in advancing high-energy science.
Keywords: Quantum computing, Plasma Physics, fusion energy, High-energy applications, quantum algorithms
Received: 24 Dec 2024; Accepted: 11 Feb 2025.
Copyright: © 2025 Yang. This is an open-access article distributed under the terms of the Creative Commons Attribution License (CC BY). The use, distribution or reproduction in other forums is permitted, provided the original author(s) or licensor are credited and that the original publication in this journal is cited, in accordance with accepted academic practice. No use, distribution or reproduction is permitted which does not comply with these terms.
* Correspondence:
Yifei Yang, Chongqing University, Chongqing, China
Disclaimer: All claims expressed in this article are solely those of the authors and do not necessarily represent those of their affiliated organizations, or those of the publisher, the editors and the reviewers. Any product that may be evaluated in this article or claim that may be made by its manufacturer is not guaranteed or endorsed by the publisher.
Research integrity at Frontiers
Learn more about the work of our research integrity team to safeguard the quality of each article we publish.