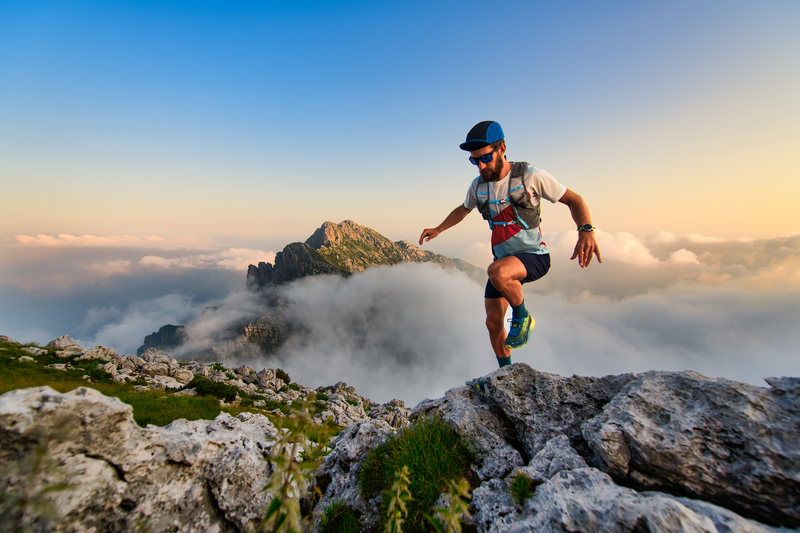
95% of researchers rate our articles as excellent or good
Learn more about the work of our research integrity team to safeguard the quality of each article we publish.
Find out more
ORIGINAL RESEARCH article
Front. Phys. , 31 March 2025
Sec. Social Physics
Volume 13 - 2025 | https://doi.org/10.3389/fphy.2025.1550471
This article is part of the Research Topic Finance and Production Complex Systems View all 10 articles
Climate risk has a negative impact on the stable operation of social, economic, and financial systems. Local commercial banks operate in a single geographical area and are unable to effectively diversify risk. This article uses data from local commercial banks from 2009 to 2021 to explore the impact of climate risk on the soundness of local commercial banks. The research results show that climate risk, represented by extreme rainfall and transition risk, affects the soundness of local commercial banks by increasing direct losses to credit entities, reducing corporate profitability, and decreasing corporate credit demand. Further analysis shows that for rural commercial banks, extreme rainfall and extreme high temperatures diminish their soundness by affecting agricultural production. In regions with high temperatures, extreme heat reduces workers’ labor income and the productivity of enterprises’ labor, thereby affecting the soundness of local commercial banks. The larger the scale, the higher the degree of digital transformation, and the higher the level of property insurance of local commercial banks, the smaller the impact of climate risk on their soundness. Therefore, it is suggested that local commercial banks should raise awareness of climate risk, expand their business scope, increase insurance coverage for credit entities, and accelerate digital transformation to maintain stable operations in the context of increasing climate risk.
Climate change is a major challenge facing all mankind, seriously affecting the human living environment and socio-economic stability. As shown in Figure 1, the global warming trend is still ongoing, with frequent extreme high temperatures and an increase in extreme precipitation events in China. The frequency of extreme high-temperature events in China in 2022 was the highest since 1961 (Figure 2), and the climate risk index shows an upward trend. In order to reduce the impact of climate change risks in China, China proposed the “dual carbon target” set in September 2020, which aims to peak carbon dioxide emissions before 2030 and achieve carbon neutrality before 2060. In the 1990s, Schelling (1992), Ausubel (1991) [1, 2], and others began to discuss the impact of climate change on agriculture, economy, technological change, and other aspects. Carney (2015) [3] believes that climate change poses a threat to financial stability and long-term prosperity. With the implementation of the Paris Agreement, central banks and financial regulatory authorities of various countries have begun to study the possibility of financial systemic risks brought about by climate change. Climate change can trigger structural changes in the financial system, which has become a global consensus, but central banks around the world are divided over whether climate change factors should be included in the macro monetary policy and prudential supervision framework. The 20th National Congress of the Communist Party of China proposed actively participating in global governance to address climate change, strengthening the financial stability guarantee system, and safeguarding the bottom line of preventing systemic risks. The 2024 government work report also pointed out the vigorous development of the green and low-carbon economy, active and steady promotion of carbon peak neutrality, and classified disposal of financial risks. Climate change is increasingly being recognized as a contributing factor to financial risks.
Figure 1. Global average temperature anomaly from 1850 to 2022 (relative to the average from 1850 to 1900). Reprinted with permission from Blue Book on Climate Change in China (2023) [90] by CMA Climate Change Centre, Copyright: Beijing, Science Press.
Figure 2. Frequency of Extreme High Temperature Events in China from 1961 to 2022. Reprinted with permission from Blue Book on Climate Change in China (2023) [90] by CMA Climate Change Centre, Copyright: Beijing, Science Press.
The Bank of England’s 2018 report found that most banks are starting to consider climate change risks like other risks, rather than simply a social responsibility. The People’s Bank of China will carry out climate risk stress tests in 2021, organize 19 systemically important banks to conduct sensitivity stress tests on high-carbon industries, and improve financial institutions’ risk management awareness. The European Central Bank stated that the losses incurred by European banks due to the rise in carbon asset prices and the impact of natural disasters exceeded €70 billion; The Bank of England found that banks and insurance companies that do not incorporate climate risk into their daily management may face profit losses of more than 10%. Climate risk has not yet been clearly recognized and effectively addressed by most financial institutions, and only a small number of large financial institutions adopt some environmental assessments (NGFS). Because small-scale banks are mostly local banks, their service recipients’ information disclosure is not standardized and the quality of disclosure information is low. Therefore, small banks may face more severe climate risks (Liu et al., 2024) [4]. Local commercial banks include urban commercial banks, rural commercial banks, rural credit cooperatives, and village banks that operate within their residential areas. Compared to national banks, they cannot effectively diversify risks or avoid regional economic fluctuations (Wang et al., 2012) [5]. The business scope of national commercial banks and joint-stock commercial banks is more dispersed across regions and industries, and the impact of climate change on their operations is limited; Local commercial banks mainly serve counties with concentrated service areas. When extreme weather occurs, it has a significant impact on their operations (Liu et al., 2021; Pan et al., 2022) [6, 7]. Currently, China remains in an indirect financing system dominated by commercial banks. Local commercial banks undertake the mission of economic transformation, upgrading and development. Climate change increases the operational risks of banks, which are then transmitted throughout the financial system, triggering systemic risks. Therefore, discussing the impact of climate risks on the soundness of local commercial banks in the context of “dual carbon” is crucial for establishing a climate risk prevention framework for small- and medium-sized banks, as well as providing a basis for building a prudent regulatory system for climate risks, which holds practical significance.
The main marginal contributions of this article are as follows: (1) Existing literature focuses on the impact of climate risk on the credit risk of local commercial banks, but fails to reveal the negative effects of climate risk on the overall operation of local commercial banks. This article expands the single impact of climate risk on the credit risk of local commercial banks by examining the impact on their soundness. (2) This article makes a significant contribution to the literature by introducing a soundness indicator for commercial banks, which provides a more comprehensive measure of bank stability compared to the commonly used credit risk indicators in existing research. While prior studies have largely focused on the impact of climate risks on banks’ risk-taking behavior using credit risk measures (Song et al., 2024) [8], this study extends the analysis by assessing the soundness of local commercial banks in China, incorporating both physical and transition risks. (3) By analyzing the heterogeneity of samples from multiple perspectives, this study explores the differences in the impact of different categories of physical risks on the heterogeneity of samples, and provides different climate risk compensation mechanisms for local commercial banks based on their own characteristics.
This study provides insights into the relationship between climate risks and bank soundness. However, we acknowledge that climate risks and financial regulations have continued to evolve beyond 2021. With the intensification of climate change, the frequency and intensity of extreme climate events are increasing, and financial institutions are continuously improving their risk management measures. Consequently, future research should incorporate more recent data to assess the dynamic nature of climate risks and the effectiveness of emerging regulatory policies (Campiglio et al., 2018) [9]. Additionally, new methodologies, such as stress testing and scenario analysis, can help model the impact of future climate risks on the financial sector more accurately (NGFS, 2020) [10]. The economic and financial aspects, as well as climate risk exposures, are closely interconnected among local commercial banks across different regions. While this study focuses on the impact of local climate risks on the soundness of individual local commercial banks, it does not fully account for the inter-regional synergistic effects. Future research could incorporate these cross-regional spillover effects to provide a more comprehensive understanding of how climate risks affect the banking sector across regions.
The remainder of this article is organized as follows: The second part reviews relevant literature on climate finance and banking operational risks, summarizes the transmission channels of climate risk to local commercial banks, and presents hypotheses. The third part outlines a research plan based on assumptions, presents the data, and constructs a regression model. The fourth part empirically examines the impact of regional climate risks on the soundness of local commercial banks and analyzes the results. The fifth part conducts a robustness test and further analysis to ensure the validity of the results. The sixth part summarizes the article and offers policy recommendations based on the experimental results.
Climate finance is an emerging research area in finance in recent years, such as the impact of climate risks on asset pricing, financial instruments, financial regulation, and public policy. The signing of the Paris Agreement in 2015 significantly advanced climate finance research. This chapter reviews the existing literature on climate finance and analyzes the mechanisms through which climate risk affects local commercial banks, proposing hypotheses.
The European Central Bank’s 2019 Financial Stability Assessment Report noted that if climate risks are not reasonably priced by the market, they may pose systemic risks across the eurozone. Climate finance risks are characterized by the loss of underlying financial asset value due to climate change, with features of ‘low probability and high loss’. Climate risk encompasses the risks from natural disasters, extreme weather, resource crises, and other negative impacts on human survival and economic growth due to climate change (Guo et al., 2023b) [11]. NGFS (2020) [10] also includes the risks associated with actions taken to address climate change within the scope of climate risk. Most researchers classify climate risk into physical and transition risks (Carney, 2015; NGFS, 2020; Cepni et al, 2023) [3, 10, 12]. Physical risks refer to direct economic losses from extreme weather events such as rainstorms, droughts, high temperatures, and floods, as well as indirect impacts caused by the ongoing effects of subsequent events; Transition risk refers to a series of policies introduced by various countries to address climate change, which lead to the accumulation of uncertain risks in the low-carbon transition market, such as the effects of policies, investor preferences, and market dynamics on economic and financial activities (Yang et al., 2024) [13]. Transition risk is long-term and typically occurs in industries with high levels of asset stranding, such as fossil energy (Zhang et al., 2022) [14].
Finance is the core and lifeblood of the modern economy. A financial crisis can have a profound impact on economic development. In China, banks occupy a central role in the financial system. Initially, China established a highly centralized financial system dominated by large banks (Lin and Li, 2001) [15]. Yao and Dong (2014) [16] believe that China should establish a financial system centered around small and medium-sized banks. By the end of 2023, the total assets of China’s banking industry are expected to account for over 90% of the total assets in financial institutions. This shows that China’s financial system remains dominated by banks. Maintaining the stability of the banking sector is key to ensuring financial stability. Effective banking governance strengthens risk management, promotes stability, and is crucial to financial stability and economic growth (Wang, 2013) [17]. Systemic risks in banking can lead to economic crises and severe socio-economic consequences (Milne, 2009) [18]. A flawed banking system can reduce resource allocation efficiency and increase uncertainty in economic growth (Jokipii and Monnin, 2013) [19]. Stable operations are the fundamental principle of commercial banking, essential across different contexts and institutional conditions, and widely accepted internationally. Gong (2004) [20] suggests that sound banking operations should adapt to economic conditions and market changes, balance safety and efficiency, evolve steadily, and pursue development on a stable foundation. A bank’s soundness reflects its ability to withstand market shocks. The lower the soundness, the more likely a bank is to face a run or collapse during systemic risk, which is linked to its fragility (Wang and Zhu, 2022) [21]. Chen and Wang (2011) [22] argue that existing research fails to fully capture the micro-level risks and robustness of bank operations, and propose a bank robustness indicator system based on five factors: capital adequacy, asset quality, profitability, liquidity, and risk. Most existing research examines the impact of climate change on commercial banks primarily from a risk perspective, often overlooking profitability and efficiency.
The primary source of commercial bank profits is the interest margin between deposits and loans, known as “interest income”. Additionally, “non-interest income” comes from intermediary business activities (Wang and Liu, 2013) [23]. In the fourth quarter of 2023, non-interest income accounted for 19.93% of total income, while interest income exceeded 80%. This indicates that China’s commercial banks still rely primarily on the traditional deposit-loan model for profits. Extreme weather events, such as high and low temperatures and heavy precipitation, can damage assets and reduce profitability for commercial bank borrowers, leading to direct or indirect losses for households and businesses, increasing the likelihood of defaults, and disrupting the stability of banks (Pan et al., 2022) [7]. Specifically, physical risks may lead to a variety of adverse financial outcomes for banks. For instance, extreme weather events such as floods, droughts, and storms can damage physical assets, disrupt supply chains, and reduce the productivity of key industries (e.g., agriculture, energy, manufacturing), all of which have significant financial implications for banks. When such risks are not adequately accounted for, they can lead to higher default rates on loans, especially in sectors most vulnerable to these events (e.g., agriculture, infrastructure, real estate) (Stern, 2007) [24]. Climate risks can cause corporate assets to become stranded or damaged, leading to fluctuations and depreciation in asset values, increasing uncertainty, and causing depreciation of assets mortgaged or held by commercial banks, thus increasing their operational risks (Ploeg and Rezai, 2020) [25]. Wu et al. (2024) [26] argue that the increase in climate risk will lead to a deterioration in the credit quality of commercial banks, resulting in heightened systemic risk, but with a limited impact on the depreciation of their investment portfolios. The intensification of climate risks has resulted in lower efficiency, business damage, supply chain disruptions, asset stranding, unstable income and profits, reduced repayment ability and willingness, rising default rates, and exacerbated bank credit risks, ultimately impacting their soundness. Climate risk not only reduces the profitability of enterprises but also intensifies financing constraints, lowers financing needs, reduces research and development investment, and ultimately decreases total factor productivity (Liu et al., 2023) [27]. Climate change significantly affects the operational efficiency of enterprises, leading to fixed asset losses, reduced expected returns, and decreased financial performance, including revenue, expenses, assets, liabilities, capital, and financing (Qing et al., 2024) [28]. In the context of banking regulation, the Basel III framework recognizes the importance of assessing and mitigating risk factors that could impair a bank’s financial health. Several studies have emphasized the importance of integrating physical climate risks into stress testing and financial disclosure practices to improve the resilience of financial institutions (Stern, 2007) [24]. These insights suggest that integrating physical risks into the assessment of banking risk is essential to ensure the long-term stability and soundness of financial systems. In short, climate risks have increased financing constraints for businesses, reduced their financing capacity and demand, and led to a contraction in the credit scale of local banks. When bank deposit size remains unchanged, a decrease in loan size lowers the loan-to-deposit ratio. The loan-to-deposit ratio reflects a bank’s asset allocation and is a key indicator of its operational efficiency. A higher loan-to-deposit ratio indicates greater efficiency in asset allocation (Zhen and Cao, 2005) [29]. Climate risk reduces corporate financing demand, decreasing the loan scale of local commercial banks, lowering their asset allocation efficiency, and further weakening their soundness.
In addition to the impact of physical risk, transition risk can also be transmitted through enterprises to banks. In order to alleviate the effects of climate change and extreme weather, the international community has begun advocating for low-carbon transformation and limiting carbon emissions, and has signed agreements such as the Paris Agreement to restrict member countries’ carbon emissions. In the process of low-carbon transformation, financial risks have rapidly increased along with the accumulation of climate risks. Climate risks and natural disasters have led to instability in the banking industry, exacerbating financial risks (Hansen, 2022) [30]. In 2020, China proposed the “dual carbon” goal, striving to achieve carbon peak before 2030 and carbon neutrality before 2060. According to data from the International Energy Agency, China’s carbon emissions in 2021 were 10.648 billion tons, accounting for 31.7%, ranking first in the world. China is facing enormous pressure to reduce emissions. Yu et al. (2022) [31] face severe challenges in emissions reduction, requiring significant changes in socio-economic development models and technologies, rapid development of low-carbon energy, decarbonization of energy transformation, and accelerated industrial restructuring. Thus, Chinese enterprises are facing enormous transformation challenges and regulatory pressures. Traditional environmental economic theory holds that environmental regulations affect the production conditions for optimal costs of enterprises. When the internalization of environmental costs intensifies, it will squeeze out the funds invested by enterprises in other places, resulting in reduced efficiency, business damage, and decreased competitiveness in domestic and international markets (Yu and Sun, 2017) [32]. At the same time, strict environmental regulations have increased the financial risks of enterprises, which are more evident in terms of profitability and debt increase (Wang and Wang, 2024) [33]. In the process of transformation, high carbon emitting industries such as fossil steel face the risk of non clean energy assets being stranded due to emission restrictions (Sun et al., 2024) [34], which further leads to a decrease in the value of assets pledged to banks and increases their operational risks. Transition risks arise from significant changes in policy, technology, and market dynamics as economies shift towards sustainability. The transition to a low-carbon economy is driven by policies such as carbon pricing, regulations on emissions, and incentives for renewable energy. These policies directly impact financial markets, influencing capital flows and the cost of financing, particularly for carbon-intensive industries. According to Green Finance Theory, policies aimed at promoting green investments and sustainable practices redirect capital flows towards low-carbon technologies, which can influence the financial stability of banks with large exposures to high-carbon sectors (Lamperti et al., 2021) [35]. Furthermore, the financial transmission mechanisms explain how these policies affect banks through various channels. For instance, carbon taxes increase the cost of financing for carbon-intensive sectors, elevating credit risk for banks that are heavily invested in these industries. At the same time, the introduction of stricter environmental regulations can lead to asset devaluation in high-carbon industries, impacting the collateral value for loans (Carney, 2015; Battiston et al., 2017) [3, 36]. Moreover, the transition can also cause shifts in market expectations, creating both risks and opportunities for banks as they adjust their portfolios and risk management strategies (Nguyen et al., 2023) [37]. In addition, the interaction between policy uncertainty and financial markets is a critical factor in the financial transmission of climate transition risks. The introduction of new policies or changes in existing ones can cause uncertainty in the financial markets, leading to volatility in asset prices and shifting investment patterns (Pastor and Veronesi, 2012) [38]. Banks must navigate this uncertainty while adapting their risk assessment frameworks to mitigate the impact of potential policy changes on their financial soundness.
The above analysis indicates that both ‘physical risks’ and ‘transition risks’ affect the soundness of commercial banks through credit entities, potentially triggering systemic risks. Extreme weather events cause both direct and indirect economic losses to households and businesses. Over the past 50 years, extreme heat, low temperatures, precipitation, and other events have occurred frequently, reaching 11,778 cases, causing more than 2 million deaths and direct economic losses of up to 4.3 trillion US dollars. Given the increasing frequency of extreme weather events, direct and indirect losses caused by climate risks will be transmitted to the banking sector through credit entities, leading to higher default rates, lower collateral value, and profit losses, ultimately decreasing bank soundness. Based on this, the following hypotheses are proposed.
H1. Climate risks have negative impacts on the soundness of local commercial banks.
H2. Climate risk can cause direct economic losses to credit entities, reduce corporate profitability, increase corporate financing constraints, and reduce corporate financing demand, thereby reducing the soundness of local commercial banks.
Risk refers to the uncertainty surrounding future losses, including both the size and likelihood of such losses occurring (Guo et al., 2004) [39]. Huang et al. (2010) [40] defined meteorological disaster risk as a future scenario in which meteorological factors cause economic and social losses, based on the definitions of risk and natural disasters. The risk of natural disasters primarily affects the social economy in two ways: direct losses to the real economy and the anticipated impact of disasters on economic entities (Chen et al., 2014) [41]. Insurance has been highly successful in risk management, as it converts losses from uncertain or low-probability natural disasters into deterministic costs through premiums (Wang, 2006) [42]. Shao et al. (2017) [43] argue that the existence of insurance can reduce macroeconomic losses from risks and help mitigate economic and social fluctuations. For extreme weather, both pre- and post-disaster insurance measures can reduce losses to economic entities. Natural disasters are characterized by low probability and high loss, making the demand for natural disaster insurance by economic entities distinct from other forms of insurance. People tend to choose insurance for risks with a higher probability of occurrence. In China, natural disaster insurance has yet to play its full role, primarily due to insufficient demand for disaster insurance from economic entities, which remains far below global levels (Tian et al., 2015) [44]. Therefore, as climate risks intensify, insurance coverage becomes an effective means of mitigating losses for economic entities, thereby reducing the negative impact of credit entities on bank operations.
Digital technology disrupts enterprise production and operation by reducing costs, eliminating intermediaries, and transforming existing production models and organizational structures. Digital transformation has become a strategic goal for most banks. The digital transformation of commercial banks can significantly improve management, reduce operating costs, enhance operational efficiency, alleviate information asymmetry, and reduce risk-taking. The impact on small and medium-sized banks is more pronounced (Jiang et al., 2023; Zhai et al., 2023) [45, 46]. Improving the digitalization of local commercial banks strengthens risk management awareness, enhances understanding of climate risks, and reduces information asymmetry with high climate-risk credit entities. Digital transformation also helps commercial banks accurately identify enterprise credit needs, direct credit to physical businesses, prevent excessive corporate financialization, improve credit efficiency, and optimize credit structures (Ding and Zhou, 2024) [47]. Improving the digitalization of local commercial banks alleviates financing constraints, boosts financing needs, expands credit volume, and enhances operational performance. Based on this, the following research hypotheses are proposed.
H3. The higher the level of insurance coverage and digitalization, the less impact climate risk has on the soundness of local commercial banks.
This article uses data from local commercial banks in China from 2009 to 2021 as the research sample. Bank data are obtained from Wuhan University’s China Banking Database (CBD), while climate data, including rainfall and temperature in China, is sourced from the publicly available FTP server of the National Climate Data Center (NCDC) in the United States. Enterprise and macroeconomic data are sourced from the WIND and CSMAR databases. The reason for choosing 2009 as the starting point is that, prior to 2009, the number of local commercial banks and the available data samples were relatively small and not representative. However, as of this study, data for 2022 and beyond have not yet been fully processed and standardized within the CBD. Therefore, the dataset currently extends only up to 2021, which remains highly representative of key trends in banking soundness and climate risk exposure. Although climate risks and financial policies continue to evolve, this period remains relevant for several reasons. First, it captures significant regulatory changes in climate-related financial risks, including the early implementation of green finance initiatives in China (Ma and Zadek, 2019) [48]. Second, it covers multiple extreme climate events, such as severe floods and heatwaves, allowing for an analysis of banks’ responses to different climate risks (Song et al., 2024) [8]. Third, financial institutions have historically adapted their risk management strategies gradually, meaning that insights drawn from this period can still provide valuable guidance for understanding long-term trends in banking stability under climate risks (Bolton et al., 2020) [49]. To mitigate the influence of extreme values, a 1% truncation has been applied to bank financial variables. After data processing, the sample includes 293 local commercial banks, comprising 104 urban commercial banks, 185 rural commercial banks, and 4 rural credit cooperatives, with a total of 2,294 observations. The sample spans 31 provinces and autonomous regions, 131 prefecture-level cities, and 251 county-level administrative regions, demonstrating strong representativeness.
The study of climate risk typically requires controlling for time-invariant factors such as geographical characteristics. Therefore, using a fixed-effects model to analyze climate risk is more appropriate (Ding and Sun, 2022) [50]. To verify the above hypothesis, this article constructs the following baseline regression model for empirical analysis:
Among them, i represents a certain bank, t represents the year, and γ represents the prefecture level city where the bank is located. The dependent variable
Li and Li (2024) [51] believe that the risk impact of climate change on the insurance industry has a significant time lag. Fan et al. (2024) [52] found through a sample study of Chinese listed banks that climate risk has a delayed impact on banks. Similarly, there is a certain lag and persistence in the transmission of climate risks to banks, which means that previous climate risks may have an impact on current bank operations. Therefore, this article adds a first-order lag term of climate risk
Most scholars primarily consider bank stability—namely non-performing loans or risk-taking—when studying climate risks. However, the concept of bank soundness is distinct from other common bank risk indicators, as it focuses on a bank’s ability to withstand various financial and environmental shocks. Unlike market, liquidity, or credit risk, which typically assess specific types of risks, soundness is a broader measure that integrates capital adequacy, liquidity management, and overall resilience to external pressures. Soundness is particularly valuable because it provides a comprehensive assessment of a bank’s capacity to navigate financial turbulence or economic crises (Laeven and Levine, 2009; Coyle, 2000; Diamond and Dybvig, 1983) [53–55]. Soundness indicators offer a holistic evaluation, encompassing both short-term and long-term risks, making them ideal for assessing a bank’s ability to endure diverse financial and market conditions. Additionally, soundness emphasizes a bank’s preparedness for economic crises, ensuring that it is not only effectively managing risks but also structurally resilient over time (Basel Committee on Banking Supervision, 2011; Blundell-Wignall and Atkinson, 2010) [56, 57]. This makes soundness measures particularly relevant for evaluating both regulatory compliance and operational robustness across the banking sector. This article builds on Gong Feng’s research (Gong, 2004) [20] and classifies bank soundness into three categories: stability indicators, development indicators, and efficiency indicators. Stability indicators include the capital adequacy ratio (Car), liquidity ratio (Liq), non-performing loan ratio (Npl), and provision coverage ratio (Pc). Development indicators comprise the asset growth rate (Dasset), profit growth rate (Dnrofit), and deposit growth rate (Ddeposit). Efficiency indicators consist of return on capital (Pcaptial), return on assets (Passet), income profit margin (Pincome), per capita profit (Pemp), and cost income rate (Cincome). This article employs the entropy method to synthesize 12 tertiary indicators into 3 secondary indicators, which are then consolidated into a single primary indicator. Since all indicators are standardized and normalized before applying the entropy method, a higher bank soundness score indicates better overall bank performance.
Climate risk is typically difficult to measure directly. This article draws on the research method of Pan et al. (2022) [7] and uses extreme weather events to quantify climate risk. Given the availability of extreme weather data, this article conducts empirical tests using extreme high temperatures (Htd), extreme low temperatures (Ltd.), and extreme rainfall (Ipd). Due to significant regional climate variations in China, this article employs the relative threshold method to determine extreme weather thresholds for each region. Temperature and rainfall data from 1981 to 2010 are used as the baseline for climate averages, and the extreme thresholds for local commercial banks are defined using daily meteorological observations from stations in the cities where banks are located. The percentile relative threshold method is applied to determine these thresholds, and the frequency of extreme events occurring each year during the sample period is calculated. Specifically, the methodology involves the following steps: Downloading data from the National Climate Data Center in the United States, extracting it, and selecting data from Chinese stations based on their country IDs. For temperature data, within each station, arranging the daily highest and lowest temperatures during the standard period from lowest to highest, and setting the 90th and 10th percentiles as the thresholds for extreme high and low temperatures, respectively. If, during the sample period, a station records a highest temperature exceeding the extreme high-temperature threshold or a lowest temperature falling below the extreme low-temperature threshold, an extreme high-temperature or low-temperature event is identified in the corresponding city. Daily data are then aggregated into annual reports to determine the number of extreme temperature days per year in each city. For rainfall data, sorting annual rainfall data (greater than 0 mm) within the standard period from lowest to highest, and setting the extreme rainfall threshold as the average of the 90th percentile of each year’s rainfall data. Similarly, if a station records total annual rainfall exceeding this threshold during the sample period, an extreme rainfall event is identified in the city where the station is located. Daily data are then aggregated into annual reports to determine the number of extreme rainfall days per year in each city.
To effectively identify the impact of climate risks, this article draws on the methodologies of Pan et al. (2022) [7], Liu et al. (2021) [6], and Xu (2022) [58] and selects the following control variables: Bank asset size (Lsize): The logarithm of total bank assets. Equity asset ratio (Ea): The proportion of equity assets to total assets. Deposit ratio (Dep): The proportion of total deposits to total assets. Net interest margin (Nim): The ratio of a bank’s net interest income to its total interest-bearing assets. Macroeconomic variables are selected based on regional economic development and environmental conditions. Due to data availability, nighttime light brightness (Lights) is used as a proxy for economic development, while the average urban PM2.5 concentration is used as a proxy for environmental quality. The detailed definitions of the variables are presented in Table 1, and the descriptive statistics of the sample are shown in Table 2.
To test the impact of climate risk on bank soundness, this paper employs the regression model specified in Equation 1. The regression results are presented in Table 3. Columns (1)–(6) report the effects of extreme rainfall, extreme high temperatures, and extreme low temperatures on the soundness of local commercial banks. The results indicate a lagged effect of extreme rainfall on the soundness of local commercial banks. Current extreme rainfall does not significantly affect bank soundness, whereas one-period lagged extreme rainfall exhibit a significant negative impact. This negative effect remains statistically significant even after the inclusion of control variables. However, the effects of extreme high and extreme low temperatures remain insignificant, even after controlling for additional variables, suggesting that these temperature extremes do not have a meaningful impact on the soundness of local commercial banks. Ray et al. (2021) [59] found that the frequency of extreme events in India has increased over the past 50 years, with fatalities from floods far exceeding those caused by heatwaves and cold waves. Similarly, Hu et al. (2024) [60] assert that in China, extreme rainfall has the greatest impact and longest duration, persisting for up to 7 months. From an enterprise perspective, extreme rainfall increases environmental uncertainty and operational risks, exacerbating financing constraints, which hinders production activities and promotes financialization. However, extreme high and low temperatures do not exhibit significant effects (Deng et al., 2024) [61]. Extreme precipitation and extreme heat events are intensifying globally, with a rising likelihood of compound extreme events and low-probability, high-impact disasters (Zhai et al., 2021) [62]. In terms of disaster impact, economic losses, and post-disaster recovery, extreme rainfall tends to cause greater direct economic losses, affect a larger population, and require more complex and prolonged recovery efforts compared to extreme temperature events. According to an investigation by the Ministry of Emergency Management of the People’s Republic of China, the consequences of extreme rainfall significantly outweigh those of extreme high temperatures. This suggests that compared to extreme high temperatures, extreme rainfall occurs more frequently, results in greater losses, affects a wider range, and presents greater challenges for disaster response and recovery. Therefore, the negative impact of extreme rainfall on the soundness of local commercial banks is significant, whereas the impact of extreme temperatures remains insignificant.
Climate risk can be categorized into physical risk and transition risk. The previous discussion focused on physical risks, represented by extreme temperatures and extreme rainfall. Transition risk refers to the uncertainty enterprises face in implementing green transformation under increasingly stringent climate policies. These risks include the stranding of high-carbon assets, energy substitution, technological innovation, and environmental regulations, all of which negatively affect business operations. Transition risk undoubtedly impacts the day-to-day operations of enterprises by raising production costs, such as expenses related to clean energy adoption and low-carbon innovation. These factors erode profitability and diminish asset values, which in turn transmit to the banking sector, ultimately reducing the soundness of local commercial banks. Due to the lack of detailed data on commercial bank loan recipients, this article follows the methodology of Li and Pan (2022) [63] to measure transition risk at the prefecture-level city by using the proportion of GDP from high-carbon-emission industries relative to the city’s total GDP. Specifically, we measure regional transition risk using the proportion of GDP from agriculture, forestry, animal husbandry and fishery, construction, real estate, industry, transportation, warehousing, and postal services. The empirical results are presented in Table 4. As shown in Table 4, transition risk also exerts a negative impact on the soundness of local commercial banks. This finding supports Hypothesis 1, confirming that climate risks have negative impacts on the soundness of local commercial banks.
To further verify the impact of extreme rainfall and extreme temperatures, we adjusted the thresholds for extreme rainfall, extreme high temperature, and extreme low temperature by both increasing and decreasing them. Specifically, the threshold for extreme rainfall was set at the 95th and 99th percentiles, the threshold for extreme high temperature at the 95th and 99th percentiles, and the threshold for extreme low temperature at the fifth and first percentiles. These stricter thresholds for extreme rainfall and temperature allow us to assess whether the regression results remain robust under different threshold specifications. The results are presented in Table 4.
Secondly, China has a vast geographic expanse and significant regional climate variations, leading to differences in both the probability and impact of extreme weather events. As this article employs the relative threshold method to measure extreme weather, the occurrence of extreme high temperatures in traditionally cooler regions and extreme low temperatures in warmer regions may not significantly affect social production and economic activities. This could introduce potential regression bias. Extreme temperature events in central and northern China exhibit strong long-range correlations, suggesting that extreme high temperature events are more likely to occur in these regions. Conversely, regions such as Yunnan-Guizhou, Northeast China, and the Qinghai-Tibet Plateau are less affected by extreme high temperatures (Ren et al., 2010) [64]. Similarly, extreme low temperatures have minimal impact on areas south of the Nanling Mountains. This paper follows the threshold criteria proposed by Cai (2016) [65] for extreme temperature events across different provinces. Specifically, provinces with an extreme high temperature threshold below 30°C and an extreme low temperature threshold above 0°C were excluded from the sample. This includes Guangdong, Guangxi, and Hainan, which are less affected by extreme low temperatures, as well as Qinghai and the Tibet Autonomous Region, which are less affected by extreme high temperatures. The results are presented in Table 5. In Table 5, Columns (1)–(2) present the results after excluding samples from Qinghai and Tibet, while Columns (3)–(4) show the results after excluding samples from Guangdong, Guangxi, and Hainan. The findings in Tables 5, 6 demonstrate that after adjusting the threshold criteria and removing specific samples, the results remain consistent with the baseline regression.
In the main regression, this article adopts the commonly used international practice of using the past 30 years as the standard period to calculate the extreme event threshold. Since the data in this article starts from 2009, the closest standard period is 1981–2010. In order to avoid the impact of incorrect selection of standard periods on empirical results, this article uses two standard periods, 1971–2000 and 1971–2010, to calculate extreme event thresholds and re conduct empirical tests. The regression results are shown in Table 7. According to the results in Table 7, after replacing the standard period, extreme rainfall still has a negative impact on the operational risk of local commercial banks, while extreme temperature does not have a significant impact, which is consistent with the main regression. The greater the fluctuation of temperature and rainfall, the greater the unexpected impact on the social economy. Therefore, this article draws on the approach of Liu et al. (2021) to measure climate risk using the fluctuation of annual average temperature and annual average rainfall, and conducts empirical testing again (We use temperature and rainfall fluctuations calculated based on the base period of 1991–2021). The specific results are shown in Table 8. The results in Table 8 show that rainfall fluctuations have a negative impact on the soundness of local commercial banks, and the impact lags behind; Temperature fluctuations have no significant impact on the soundness of local commercial banks, consistent with the main regression, demonstrating the robustness of the results.
Since the reform and opening-up, China’s rural credit cooperatives have undergone four stages of reform, with the period from 2003 to 2010 marking the stage of provincial-level government management and comprehensive reform exploration (Sun and Wang, 2022) [66]. By the end of 2010, most of China’s rural credit cooperatives had completed the transition to a shareholding system. To avoid the potential impact of these reforms on the analysis, we excluded samples prior to 2011. At the end of 2020, the outbreak of COVID-19 had a profound impact on China’s economy and society. He et al. (2020) [67] argued that in the aftermath of COVID-19, the probability of enterprise defaults increased, significantly affecting urban and rural commercial banks. To eliminate potential biases caused by the COVID-19 shock, we also excluded samples after 2020. The results are presented in Table 9, which shows that the regression findings remain consistent with the main regression, demonstrating the robustness of the results.
Soundness primarily emphasizes stability, which refers to a bank’s ability and condition to maintain financial stability. Stability is the most critical factor in assessing bank soundness. According to Gong Feng’s research (Gong, 2004) [20], the stability index score is the highest-weighted and most influential indicator of bank soundness. Therefore, we replaced the soundness indicator with the stability indicator for robustness testing and selected the default rate as a key variable for further analysis. The regression results, presented in Table 10, show that extreme rainfall has a negative impact on the stability of local commercial banks with a lag effect, while extreme high and low temperatures remain insignificant. Similarly, extreme rainfall significantly increases the non-performing loan ratio of local commercial banks without any lag, whereas extreme high and low temperatures have no significant impact on the non-performing loan ratio. Furthermore, transition risk significantly reduces the stability of local commercial banks, but does not have a significant impact on the non-performing loan ratio.
To address the potential endogeneity issue between climate risk and the soundness of local commercial banks, we employed an instrumental variable (IV) approach. Specifically, we followed the methodology of Guo et al. (2023a) [68] and used the latitude of local commercial banks as an instrument for extreme rainfall. Latitude is an inherent geographical factor specific to each locality, and it satisfies the strict exogeneity condition required for instrumental variables. This choice is based on the fact that latitude is highly correlated with precipitation patterns, as regions closer to the equator typically receive more rainfall due to the higher levels of solar radiation and atmospheric convection that occur in these areas (Sauter et al., 2023) [69]. Latitude, as a geographical variable, is independent of the financial conditions of banks, making it an appropriate instrument for climate risk in the context of our study.
Table 11 presents findings from the instrumental variables’ two-stage estimation. All the statistical figures derived from the Cragg-Donald Wald test surpass the critical threshold of 16.38, as determined at the 10% significance level by Stock-Yogo (2005), indicating that the problem of weak instrumental variables does not exist. The coefficients before Ipd in Columns (2) were negative and significant at the 10% significance level, which was consistent with the results of the main regression.
The main credit targets of commercial banks are individuals, households, and enterprises. Climate risk affects the soundness of local commercial banks through three credit entities: individuals, households, and businesses. Extreme weather causes asset impairment and losses for bank credit entities, leading to a decrease in corporate profitability and increasing household income uncertainty. This affects individual, household, and corporate income, resulting in a decrease in the value of bank collateral assets, reduced repayment ability and willingness for individuals and businesses, a decline in the quality of commercial bank credit assets, an increase in default rates, a further increase in non-performing loans, and a decrease in profits. At the same time, from the perspective of profit and risk, banks need to raise interest rates to compensate for the increased operational risks of enterprises, which increases the financing costs of enterprises, increases financing constraints, reduces financing demand, and leads to a reduction in bank credit supply. Therefore, the impact of climate risk on the soundness of local commercial banks is mainly through the direct losses caused by extreme weather to credit subjects, the indirect effects on the profitability of enterprises, and the reduction of credit supply.
This article uses the logarithm of the direct economic losses caused by natural disasters at the provincial level published by the National Bureau of Statistics as an intermediary variable to measure the direct economic losses of commercial bank service providers. Referring to the mediation effect model proposed by Wen and Ye (2014) [70], a stepwise test method was used to construct a model to examine whether climate risk affects the soundness of local commercial banks by affecting the direct economic losses of service providers in Equation 2 and in Equation 3.
The regression results are shown in Supplementary Table S1. Columns (2) and (3) in Supplementary Table S1 report the regression results of models (2) and (3). The Ipd coefficient in column (2) is positive and significant at the 1% significance level, indicating that extreme rainfall can cause direct economic losses to bank service recipients. In column (3), L The loss coefficient is negative and significant, with Ipd and L Ipd is not significant, indicating that the direct economic losses of bank service recipients have a negative impact on the soundness of local commercial banks, and this impact has a lag effect. The results of columns (1)–(3) indicate that the direct economic losses of service providers are the mediating variable for the impact of climate risk on the soundness of local commercial banks.
Climate risk worsens the profitability of enterprises, affects their production status, increases the probability of default, and thus affects the soundness of local commercial banks. Due to the inability to obtain bank level enterprise data, it is not possible to conduct bank enterprise matching in a simple way. Therefore, this article refers to the approach of Bertrand and Mullainathan (2001) [71] and Ge et al. (2021) [72], selects a sample of A-share listed companies, and establishes the following two-stage model to explore the impact mechanism of climate risk on the soundness of local commercial banks - whether the profitability of enterprises is established:
The estimated coefficients in Equation 5 multiplied by
Climate risk increases the financing constraints for enterprises, reduces their financing needs, leads to a decrease in operational efficiency, increases financial difficulties, and reduces the credit scale of local commercial banks, thereby affecting soundness. This article selects the logarithm of the total loan amount of local commercial banks and the enterprise SA index as intermediary variables to measure the scale of bank loans and financing constraints of enterprises, referring to the mediation effect model of Wen and Ye (2014) [70] and the two-stage model in the previous section.
The heterogeneity analysis is crucial in understanding the varying impacts of climate risks on local commercial banks. Different types of banks (such as state-owned or private banks) may respond differently to these risks due to varying levels of government support and risk management capacities (Yeung, 2021) [73]. Moreover, larger banks, with their more extensive resources, are better equipped to handle climate risks compared to smaller institutions (Battiston et al., 2017) [36]. Finally, geographical differences play a key role as regions with higher exposure to physical climate risks, such as coastal areas or arid regions, experience more severe impacts, which can affect their financial stability (Jia et al., 2022) [74].
In order to capture the impact of transition risks on local commercial banks, we acknowledge that enterprises’ technological innovation capabilities and policy adaptation capabilities play a crucial role. However, due to data limitations, particularly the absence of detailed reports on technological innovation and policy adaptation capabilities for many banks in our sample, we were unable to directly incorporate these factors into our analysis. As an alternative, we examined bank size and bank nature (rural commercial banks vs urban commercial banks), as they are often linked to the technological innovation and policy adaptation capacities of the enterprises within the banks’ portfolios. Research has shown that larger banks tend to have more diversified portfolios and better access to technological innovation, which enables them to better manage transition risks (Zhu, 2013) [75]. Similarly, rural commercial banks tend to be more affected by policies, and their policy adaptability is weak, thus weakening their capacity to navigate transition risks (Hao, 2022) [76]. By analyzing these characteristics, we indirectly assess the potential influence of technological innovation and policy adaptation capabilities on the climate risk exposure of the banks.
Agricultural production is one of the most sensitive sectors to climate change, and climate is the primary factor affecting agriculture. Climate change has led to significant changes in China’s crop planting system and production layout, and the increase in climate risks will directly affect the agricultural economy, resulting in a decrease in crop yields (Ding and Sun, 2022) [50]. Meanwhile, there is an inverted U-shaped relationship between precipitation and crop production. Extreme precipitation leads to waterlogging in farmland, loss of soil fertility, and affects crop yield, which is a factor contributing to the vulnerability of agricultural production (Zhou et al., 2010) [77]. Liu et al. (2012) [78] studied the impact of extreme weather on agricultural economic output in China. The research results showed that climate risk is the Granger cause of reduced agricultural economic output, and extreme rainfall has the greatest impact on agricultural economy. Due to the different nature of local commercial banks, there are significant differences in their service providers and business scope. Rural commercial banks mainly serve agricultural production oriented objects such as agriculture, rural areas, and farmers, and their business scope is mostly related to agriculture, rural areas, and farmers, with geographical locations concentrated in rural areas such as counties. We divided the sample into rural commercial banks (RCB) and urban commercial banks (UCB) for grouped regression to test whether the nature of banks affects the operational robustness of local commercial banks under climate risk. The regression results are shown in Supplementary Table S4, which indicates that neither extreme rainfall nor extreme temperature have a significant impact on the soundness of urban commercial banks. Extreme rainfall has no significant impact on the soundness of rural commercial banks at the 90% threshold, but when the threshold is raised, the impact of extreme rainfall on their operations significantly increases, indicating that the higher the degree of extreme rainfall, the greater the impact on the soundness of rural commercial banks. The impact of extreme high temperatures on the soundness of rural commercial banks increases with the increase of the high temperature threshold, and there is no lag effect. In the basic regression, extreme high temperatures have no impact on the operation of local commercial banks, but due to the significant negative impact of high temperatures on agricultural production, extreme high temperatures have a significant negative impact on rural commercial banks serving agriculture, rural areas, and farmers. There is no significant impact of extreme low temperature on the soundness of rural commercial banks under the three thresholds. The possible reason is that the average annual temperature in China has been increasing year by year, with a significant warming in winter and a relatively small impact of low temperatures on crop yields (Pan et al., 2011) [79]. Yu (2019) [80] studied the impact of climate change on crop production in Zhejiang Province. The study showed that extreme low temperatures had limited effects on crop yield reduction, with the highest impact on rapeseed yield reduction. For every day of increase in extreme low temperatures, rapeseed yield decreased by only 0.53%–0.79%, while extreme high temperatures had a greater impact on crop yield. For every day of increase in extreme high temperatures, early rice yield decreased by 3.91%–5.08%. Therefore, under the background of climate change, the impact of extreme low temperatures on agricultural production and economic and social development is limited, and rural commercial banks are not significantly affected by it. This article also conducts heterogeneity analysis based on the nature of commercial banks on transition risk. The results are shown in Supplementary Table S5. According to Supplementary Table S5, transition risk also have a greater impact on the soundness of rural commercial banks. Rural commercial banks tend to have weaker resistance to policy risks compared to larger banks. This is primarily due to their limited capacity to adapt to policy changes and lower human resource investments. These banks rely heavily on government support and are more vulnerable to policy shifts, especially those related to agricultural financing. Additionally, their lack of advanced risk management and technological resources limits their ability to handle transition risks effectively. Consequently, rural commercial banks face greater challenges and more significant impacts on their financial stability when dealing with transition risks, as they have fewer resources and less capacity to manage such risks compared to larger, more well-equipped banks.
The larger the scale of a bank, the wider its business scope, the more service objects it serves, and the more branches it has. Its assets are relatively diversified, and risks are more easily spread across various industries. Jiang and Chen (2012) [81] believe that the scale of commercial banks has the function of risk diversification. The larger the scale, the more diversified the operating assets, which can effectively diversify certain risks and reduce their risk-taking. This is more conducive to maintaining soundness and preventing systemic risks from occurring. This article starts from the perspective of local commercial bank assets, and divides the sample of local commercial banks into large and small banks through asset grouping regression to determine the impact of climate risk on the soundness of local commercial banks of different sizes. The results are shown in Supplementary Table S6. According to Supplementary Table S6, extreme rainfall has a negative impact on the soundness of smaller local commercial banks, and the impact increases with the increase of the threshold, while the impact on larger local commercial banks is not significant. The impact of extreme high and low temperatures on the soundness of new commercial banks in both large and small areas is not significant at different thresholds. The results in Supplementary Table S7 indicate that transition risk also has a negative impact on the soundness of smaller local commercial banks. Larger banks generally possess a significant advantage in technological innovation and human capital compared to smaller institutions like rural commercial banks. Their ability to invest in advanced technologies and specialized talent enhances their capacity to manage transition risks effectively. This enables them to adapt more efficiently to policy shifts and market changes. As a result, larger banks experience a lower impact from transition risks, with their financial stability and resilience being less affected compared to smaller banks. Both physical and transformational risks have a greater impact on smaller local commercial banks, making it difficult to effectively diversify risks.
With the intensification of global climate change, high temperatures have caused a series of impacts on human health. Temperature can increase negative emotions in humans, leading to psychological disorders. Yu et al. (2020) [82] believe that meteorology has an emotional effect, and teenagers, the elderly, and workers are susceptible to high temperatures and high temperature stress. KO et al. (2024) [83] analyzed data from 140 countries and found that climate change significantly reduces the happiness of people in the region. The impact of extreme high temperatures on labor productivity shows an inverted U-shaped relationship. When the temperature is too high, it will reduce the labor productivity of workers. Due to the decrease in labor productivity of workers, enterprises will increase mechanized production to replace labor (Huang et al., 2023; Wang and Lin, 2021) [84, 85]. Therefore, based on Cai’s research [65], this article retains samples from provinces with high temperature thresholds above 36 °C to empirically test the impact of extreme high temperatures on the soundness of local commercial banks. The regression results are shown in Supplementary Table S8. Extreme high temperatures only reduce the soundness of local commercial banks at the 99% threshold, indicating that in high-temperature areas, the operational robustness of local commercial banks will only be affected when extreme temperatures further intensify.
Based on the above analysis, climate risk can cause direct economic losses to individuals, households, and businesses, resulting in fixed asset losses, reduced income, decreased repayment ability and willingness, and a decline in the soundness of local commercial banks. Insurance can convert uncertain losses and risks into fixed premiums, and convert uncertain losses faced by individuals, households, and businesses into fixed costs, greatly enhancing their ability to resist risks. Therefore, purchasing property insurance can enhance one’s ability to resist risks and prevent low-risk, high loss risk shocks at a lower cost. This article uses property insurance income data from various provinces to divide the samples into high and low levels of property insurance, and conducts empirical tests by grouping them. Meanwhile, using the interaction term approach, the moderating effect of property insurance was verified, and the results are shown in Supplementary Table S8. According to Supplementary Table S8, climate risk has a negative impact on the soundness of local commercial banks in samples with low insurance levels, while the impact is not significant in samples with high insurance levels. At the same time, under the interaction term model, the coefficient before the interaction term between extreme rainfall and property insurance income is positive, indicating that property insurance has a positive moderating effect on the impact of climate risk on the operational robustness of local commercial banks, which is consistent with hypothesis 3.
Climate has a significant impact on agricultural production, and rural commercial banks are greatly affected by climate risks. But insurance can mitigate the negative impact of climate risks on the operation of local commercial banks. Agricultural insurance can supplement farmers’ risk diversification ability in large-scale natural disasters and help restore production (Zhang et al., 2007) [86]. Agricultural insurance can alleviate the impact of climate risks on agricultural production and the risks transmitted to local commercial banks. This article uses agricultural insurance income data from various provinces to empirically test whether the level of agricultural insurance protection can alleviate the impact of climate risks on the soundness of local commercial banks. As shown in Supplementary Table S9, agricultural insurance is beneficial in mitigating the negative impact of climate risks on local commercial banks. But the coefficient is not significant under the interaction term model, which may be due to the relatively backward and narrow coverage of China’s agricultural insurance system in the early days. In 2013, China implemented the “Regulations on Agricultural Insurance”, and the development of agricultural insurance entered a new stage (We excluded samples before 2014, and the coefficient before the interaction term was significantly positive, proving the impact of agricultural insurance on mitigating climate risks).
Regarding the transition risk, this article also divided the samples into two groups based on property insurance and agricultural insurance, with high and low insurance levels, for grouped regression. The regression results are shown in Supplementary Table S10. The results in Supplementary Table S10 show that the levels of agricultural insurance and property insurance have no mitigating effect on transition risks. The possible reason is that transition risk is the risk of increased costs and decreased profits in the production process due to environmental regulations, carbon emission restrictions, and other factors. Therefore, purchasing property insurance and agricultural insurance cannot have a positive effect on environmental penalties, asset obsolescence, and green technology innovation faced by enterprises during the transformation process.
The digital transformation of local commercial banks has the effect of reducing costs and increasing efficiency, effectively alleviating the degree of information asymmetry between banks and enterprises, improving operational efficiency, and expanding credit scale. According to the above analysis, improving the digitalization level of local commercial banks is beneficial for mitigating the negative impact of climate risks on their soundness. This article uses an interaction term model to verify the effect of digital level mitigation of climate risk on the operational robustness of local commercial banks. The measurement of digitalization level is generally obtained by using Python text analysis to aggregate the frequency of keywords appearing in the “digitalization” dictionary in the annual reports of listed companies (Wu et al., 2021) [87]. Local commercial banks are different from listed companies in that it is difficult to collect annual reports and disclose information irregularly, making it difficult to obtain effective digital transformation keywords. This article draws on the approach of Qi et al. (2024) [88] and uses a “Python text analysis + information retrieval” method to construct a digitalization level index for local commercial banks, measuring the level of digitalization. Python text analysis are used to statistically analyze keyword search results, which are summarized by bank year. This index has obvious right bias, and we perform logarithmic processing on it. The regression results are shown in Supplementary Table S11. The significant improvement in digitalization has alleviated the impact of extreme rainfall on the soundness of local commercial banks. Meanwhile, the risk of transformation does not have a significant impact on the soundness of local commercial banks with high levels of digital transformation, but still has a significant negative impact on the soundness of local commercial banks with low levels of digital transformation.
This article uses a sample of 293 local commercial banks from 2009 to 2021 to categorize climate risk into physical risk (extreme high temperatures, extreme low temperatures, and extreme rainfall) and transition risk. It quantitatively analyzes the impact of climate risk on the soundness of local commercial banks and tests the heterogeneity of this impact across bank size, type, and property insurance levels. The main conclusions of the empirical test are as follows: (1) Climate risk, represented by extreme rainfall, significantly reduces the soundness of local commercial banks, while extreme high and low temperatures have no significant impact across the entire sample. (2) Climate risk affects the soundness of local commercial banks by impacting the direct losses and profitability of individuals, households, and businesses, reducing repayment capacity and loan willingness. (3) Agriculture is an industry highly impacted by climate risks. Extreme high temperatures and extreme rainfall negatively affect the soundness of rural commercial banks, with the impact intensifying as climate risks increase, but no significant effect on urban commercial banks. (4) Large-scale local commercial banks have stronger risk diversification capabilities, while extreme rainfall has a greater impact on smaller-scale local commercial banks, with the effect increasing as climate risk intensifies. (5) Property insurance preserves assets at low cost and converts uncertain climate risks into premiums. (6) Digital transformation reduces information asymmetry among local commercial banks, improves capital utilization, and mitigates the impact of climate risks on their soundness. (7) Both transition and physical risks negatively impact the soundness of local commercial banks. Due to differences in impact channels, transition risk has a greater effect on rural and smaller banks, while the alleviating effect of agricultural insurance is not significant.
Based on the above analysis, this article proposes the following recommendations:
First, raise awareness of climate risks among local commercial banks and integrate them into their daily management practices. Different types of climate risks should be assigned appropriate weights. For risks such as extreme rainfall, which can lead to significant losses, preventive measures and contingency plans should be established in advance.
Second, rural commercial banks with a high proportion of agricultural credit should carefully consider the impact of local climate on agricultural production, including whether specific agricultural products are affected by extreme temperatures and rainfall. In daily operations, when collateral is involved, it is crucial to assess the potential for climate risks to cause damage, and for collateral vulnerable to climate risks, its value should be appropriately adjusted.
Third, local commercial banks should pursue diversified business operations to broaden their scope and mitigate climate risks. A concentrated business scope makes operations highly vulnerable to climate risks, which can have a significant impact. Diversifying into other industries less affected by climate risks can help mitigate these risks and enhance operational stability. Additionally, risk hedging products can be developed to manage potential climate risks. Certain agricultural products are less impacted by extreme temperatures and rainfall, with some crops even benefiting from these conditions. Credit supply can be adjusted based on the type of operation to hedge against climate risks.
Fourth, local commercial banks should encourage or even mandate property insurance for individuals facing higher climate risks, incorporating insurance purchase decisions into credit assessments to determine credit limits and interest rates. When granting credit to individual and family customers, banks should consider their insurance coverage to ensure sufficient risk protection. Banks can collaborate with insurance companies to offer policy incentives for high-quality customers, improving bank soundness and boosting insurance companies’ revenues. Simultaneously, local commercial banks should accelerate their digital transformation, aiming to reach higher levels of digitalization, reduce information asymmetry between banks and enterprises, and enhance fund utilization efficiency.
Finally, the feasibility of digital transformation varies across banks due to differences in financial resources, technological investment capabilities, and talent availability (Cuesta et al., 2015) [89]. Large commercial banks have the resources to invest in advanced AI-driven risk prediction models, while small and medium-sized banks may face challenges due to limited technical expertise and funding constraints. To effectively integrate digital transformation into climate risk management, banks can adopt a phased implementation strategy based on their size and technological readiness: Large banks: Can establish dedicated climate risk management departments, develop AI-driven climate risk models, and integrate blockchain for secure green finance transactions. Medium-sized banks: Can focus on partnerships with fintech firms, adopting cloud-based climate risk analysis tools, and enhancing data-sharing platforms for climate finance. Small banks: Should prioritize cost-effective solutions, such as utilizing government-provided climate risk databases, outsourcing data analysis to specialized firms, and leveraging public-private collaborations for technology adoption.
The original contributions presented in the study are included in the article/Supplementary Material, further inquiries can be directed to the corresponding authors.
YX: Data curation, Methodology, Software, Writing–original draft, Writing–review and editing. YW: Conceptualization, Funding acquisition, Methodology, Writing–original draft. MX: Data curation, Writing–review and editing. CG: Investigation, Visualization, Writing–review and editing.
The author(s) declare that financial support was received for the research, authorship, and/or publication of this article. Major Project of Philosophy and Social Science Research of Guizhou Province (No. 22GZZB07) and Humanities and Social Science Fundamental Research Funds for Central Universities of the Nanjing Agricultural University (No. SKCX2023013).
We sincerely thank Professor Yan Liu from Wuhan University for providing the data. All errors are authors’ own.
The authors declare that the research was conducted in the absence of any commercial or financial relationships that could be construed as a potential conflict of interest.
The author(s) declare that no Generative AI was used in the creation of this manuscript.
All claims expressed in this article are solely those of the authors and do not necessarily represent those of their affiliated organizations, or those of the publisher, the editors and the reviewers. Any product that may be evaluated in this article, or claim that may be made by its manufacturer, is not guaranteed or endorsed by the publisher.
The Supplementary Material for this article can be found online at: https://www.frontiersin.org/articles/10.3389/fphy.2025.1550471/full#supplementary-material
3. Carney M. Breaking the tragedy of the horizon – climate change and financial stability. London: Speech at Lloyd’s of London (2015). Available from: https://www.bankofengland.co.uk/speech/2015/breaking-the-tragedy-of-the-horizon-climate-change-and-financial-stability.
4. Liu Z, Li J, Sun H. Climate transition risk and bank risk-taking: the role of digital transformation. Financ Res Lett (2024) 61:105028. doi:10.1016/j.frl.2024.105028
5. Wang Q, Wu W, Huang J. Geographic diversification of city commercial banks: lending expansion, risk level and bank performance. J Financial Res (2012) 01:141–53.
6. Liu B, Wang XH, Li MX. Climate change and the credit risk of rural financial institutions. J Financial Res (2021) 12:96–115.
7. Pan M, Liu HY, Cheng ZS. The impact of extreme climate on commercial banks' risk-taking: evidence from local commercial banks in China. J Financial Res (2022) 10:39–57.
8. Song Y, Chen W, Zhang K. The impact of climate change on the risk-taking level of Chinese commercial banks: empirical evidence from Chinese local commercial banks. Clim Change Econ (2024) 15:2440002. doi:10.1142/s2010007824400025
9. Campiglio E, Dafermos Y, Monnin P, Ryan-Collins J, Schotten G, Tanaka M. Climate change challenges for central banks and financial regulators. Nat Clim Chang (2018) 8:462–8. doi:10.1038/s41558-018-0175-0
10. NGFS (Network for Greening the Financial System). Guide to climate scenario analysis for central banks and supervisors (2020). Available from: https://www.ngfs.net.
11. Guo K, Li Y, Zhang Y, Ji Q, Zhao W. How are climate risk shocks connected to agricultural markets? J Commod Mark (2023) 32:100367. doi:10.1016/j.jcomm.2023.100367
12. Cepni O, Demirer R, Pham L, Rognone L. Climate uncertainty and information transmissions across the conventional and esg assets. J Int Financial Markets, Institutions and Money (2023) 83:101730. doi:10.1016/j.intfin.2022.101730
13. Yang ZH, Li DC, Chen YT. Research on the “green swan” risk in financial markets: based on the dual perspectives of physical risk and transition risk. J Manage World (2024) 40(02):47–67. doi:10.19744/j.cnki.11-1235/f.2024.0016
14. Zhang S, Lu LP, Zhang XM, Wang H. Climate risk in financial system: assessment, pricing, and management. Chin Rev Financial Stud (2022) 14(01):99–120.
15. Lin YF, Li YJ. Promoting the growth of medium and small-sized enterprises through the development of medium and small-sized financial institutions. Econ Res J (2001) 1:11–8.
16. Yao YJ, Dong GF. The development of small and medium-sized banks and the financing constraints of SMEs: empirical research from the perspective of optima financial structure theory in new structural economics. J Finance Econ (2014) 40(01):105–15. doi:10.16538/j.cnki.jfe.2014.01.010
17. Wang M. A research on the impact of shocks between China's banking soundness and macro-economic. Jilin: Jilin University (2013).
18. Milne A. “The fall of the house of credit: borrowing short and lending long: the illusion of liquidity in structured credit,” in The Fall of the House of Credit. Cambridge: Cambridge University Press. (2009). doi:10.1017/CBO9780511596643.007
19. Jokipii T, Monnin P. The impact of banking sector stability on the real economy. J Int Money Finance (2013) 32:1–16. doi:10.1016/j.jimonfin.2012.02.008
20. Gong F. Research on the soundness operation of China's banking industry under the background of financial globalization. Shanghai: Shanghai Acad Soc Sci (2004).
21. Wang H, Zhu JY. Bank soundness and liquid asset allocation from the perspective of financial regulation. Econ Res J (2022) 57(12):104–23.
22. Chen SD, Wang M. Study on stability of China's banking system. J Quantitative and Technol Econ (2011) 28(10):64–77. doi:10.13653/j.cnki.jqte.2011.10.008
23. Wang MS, Liu XF. Research on the impact of income structure of commercial banks on profitability: analysis based on panel data of 14 listed banks in China. Nankai Business Rev (2013) 16(02):143–9. doi:10.3969/j.issn.1008-3448.2013.02.015
25. Ploeg RVD, Rezai A. Stranded assets in the transition to a carbon-free economy. Annual Review of Resource Economics (2020) 12 (1). doi:10.1146/annurev-resource-110519-040938
26. Wu B, Wen F, Zhang Y, Huang ZJ. Climate risk and the systemic risk of banks: a global perspective. J Int Financial Markets, Institutions and Money (2024) 95:102030. doi:10.1016/j.intfin.2024.102030
27. Liu B, Li YQ, Jiang YJ. Climate changes and the total factor productivity of manufacturing enterprises: mechanism analysis and empirical tests. J Hunan Univ (Social Sciences) (2023) 37(01):78–87. doi:10.16339/j.cnki.hdxbskb.2023.01.011
28. Qing L, Li P, Dagestani AA, Woo C, Zhong K. Does climate change exposure impact on corporate finance and energy performance? Unraveling the moderating role of ceos’ green experience. J Clean Prod (2024) 461:142653. doi:10.1016/j.jclepro.2024.142653
29. Zheng LJ, Cao TQ. Empirical analysis of efficiency and influencing factors of commercial banks in China. J Financial Res (2005) 1:91–101.
30. Hansen LP. Central banking challenges posed by uncertain climate change and natural disasters. J Monet Econ (2022) 125:1–15. doi:10.1016/j.jmoneco.2021.09.010
31. Yu GR, Hao TX, Zhu JX. Discussion on action strategies of China's carbon peak and carbon neutrality. Bull Chin Acad Sci (2022) 37(04):423–34. doi:10.16418/j.issn.1000-3045.20220121001
32. Yu DH, Sun T. Environmental regulation, skill premium and international competitiveness of manufacturing industry. China Ind Econ (2017) 05:35–53. doi:10.19581/j.cnki.ciejournal.2017.05.004
33. Wang J, Wang H. Environmental regulation and corporate financial risk: the role of credit guarantees. Financ Res Lett (2024) 63:105357. doi:10.1016/j.frl.2024.105357
34. Sun J, Yang Y, Zhou P. Low-carbon transition risks in the energy sector: a systematic review. Sustain Prod Consum (2024) 50:115–27. doi:10.1016/j.spc.2024.07.025
35. Lamperti F, Bosetti V, Roventini A, Tavoni M, Treibich T. Three green financial policies to address climate risks. J Financial Stab (2021) 54:100875. doi:10.1016/j.jfs.2021.100875
36. Battiston S, Mandel A, Monasterolo I, Schütze F, Visentin G. A climate stress-test of the financial system. Nat Clim Chang (2017) 7:283–8. doi:10.1038/nclimate3255
37. Nguyen Q, Diaz-Rainey I, Kuruppuarachchi D, McCarten M, Tan EK. Climate transition risk in US loan portfolios: are all banks the same? Int Rev Financial Anal (2023) 85:102401. doi:10.1016/j.irfa.2022.102401
38. Pastor L, Veronesi P. Uncertainty about government policy and stock prices. J Finance (2012) 67(4):1219–64. doi:10.1111/j.1540-6261.2012.01746.x
39. Guo XT, Pu YJ, Lin L. Risk concept and its quantitative characterization. J Quantitative and Technol Econ (2004) 21(2):111–5. doi:10.3969/j.issn.1000-3894.2004.02.018
40. Huang CF, Liu AN, Wang Y. A discussion on basic definition of disaster risk. J Nat Disasters (2010) 19(6):8–16. doi:10.13577/j.jnd.2010.0602
41. Chen GJ, Zhao JF, Wu XL, Zhao XQ. Rare disaster risks and macroeconomic fluctuations in China. Econ Res J (2014) 49(08):54–66.
43. Shao QQ, Wang B, Bai LF. Risk shocks, insurance elements and economic fluctuations in China. J Financial Res (2017) 06:1–16.
44. Tian L, Yao P, Wang HB. The relationship among government behavior, risk perception and catastrophe insurance demand. China Soft Sci (2015) 09:70–81. doi:10.3969/j.issn.1002-9753.2015.09.007
45. Jiang H, Tang SF, Wu WY. Research on the lmpact of digital transformation on commercial banks risk-taking-theoretical logic and empirical evidence. Stud Int Finance (2023) 01:62–73. doi:10.16475/j.cnki.1006-1029.2023.01.006
46. Zhai SB, Cheng YT, Xie L. Impact of commercial banks' digital transformation on their risk-taking level. J Beijing Technol Business Univ (Social Sciences) (2023) 38(02):75–86. doi:10.12085/j.issn.1009-6116.2023.02.007
47. Ding X, Zhou Y. Digital transformation and bank credit allocation: based on the perspective of bank lending to the real economy. J Quantitative and Technol Econ (2024) 41(03):193–216. doi:10.13653/j.cnki.jqte.20240004.001
48. Ma J, Zadek S. Decarbonizing the belt and road: a green finance roadmap. Beijing: Tsinghua Univ. and UNEP Rep (2019).
49. Bolton P, Despres M, Pereira da Silva LA, Samama F, Svartzman R. The green swan. BIS Books, Bank for International Settlements, number 31 (2020).
50. Ding YG, Sun QX. Impact of climate risks on China's agricultural economic development: heterogeneity and mechanism analyses. J Financial Res (2022) 09:111–31.
51. Li ZH, Li KH. The theoretical analysis and empirical testing of the risk impact of climate change on the insurance industry. INSURANCE STUDIES (2024) 07:18–35. doi:10.13497/j.cnki.is.2024.07.002
52. Fan WN, Wang F, Zhang H, Ling R, Jiang HF. Heterogeneous responses of commercial banks to various climate risks: evidence from 42 a-share listed banks in China. Clim Chang Econ (Singap) (2024) 15. doi:10.1142/S2010007824500064
53. Laeven L, Levine R. Bank governance, regulation, and risk-taking. J Financ Econ (2009) 93:259–75. doi:10.1016/j.jfineco.2008.09.003
55. Diamond DW, Dybvig PH. Bank runs, deposit insurance, and liquidity. J Polit Econ (1983) 91:401–19. doi:10.1086/261155
56. Basel Committee on Banking Supervision. Basel III: a global regulatory framework for more resilient banks and banking systems. Bank Int Settl Rep (2011). Available from: https://www.bis.org/publ/bcbs189.htm (Accessed October 15, 2024).
57. Blundell-Wignall A, Atkinson P. The current financial crisis: causes and policy issues. OECD J Financ Mark Trends (2010) 1:87–121. doi:10.1787/fmt-v2010-1-en
58. Xu HX. Research on the impact of climate change on bank credit risk. Wuhan: Zhongnan University of Economics and Law (2022). doi:10.27660/d.cnki.gzczu.2022.001770
59. Ray K, Giri RK, Ray SS, Dimri AP, Rajeevan M. An assessment of long-term changes in mortalities due to extreme weather events in India: a study of 50 years’ data, 1970–2019. Weather Clim Extrem (2021) 32:100315. doi:10.1016/j.wace.2021.100315
60. Hu L, Meng J, Xiong C, Fang W, Yang J, Liu M, et al. City-level resilience to extreme weather shocks revealed by satellite nighttime lights in China. Sustain Cities Soc (2024) 101:105167. doi:10.1016/j.scs.2023.105167
61. Deng C, Su Z, Feng Y. Extreme climate and corporate financialization: evidence from China. Econ Anal Pol (2024) 81:306–21. doi:10.1016/j.eap.2023.12.001
62. Zhai PM, Zhou BQ, Chen Y, Yu R. Several new understandings in the climate change science. Clim Change Res (2021) 17(6):629–35. doi:10.12006/j.issn.1673-1719.2021.201
63. Li S, Pan Z. Climate transition risk and bank performance: evidence from China. J Environ Manage (2022) 323:116275. doi:10.1016/j.jenvman.2022.116275
64. Ren GY, Feng GL, Yan ZW. Progresses in observation studies of climate extremes and changes in mainland China. Climatic Environ Res (2010) 15(04):337–53.
65. Cai WX. Temporal trends features and spatial distributions of extreme climate events in China. Beijing: University of International Business and Economics (2016).
66. Sun XF, Wang CC. Research on the impact of shareholding reform of rural credit cooperatives on county economic growth in China. J Finance Econ (2022) 48(4):154–68. doi:10.16538/j.cnki.jfe.20211218.402
67. He CY, Wen YC, Chang YL, Gen XX. Measurement and analysis of the COVID-19 epidemic impact on China's economy. J Quantitative and Technol Econ (2020) 37(5):3–22. doi:10.13653/j.cnki.jqte.2020.05.001
68. Guo K, Bian Y, Zhang DY, Ji Q. The impact of climate-related risk on local government financing costs. J Environ Econ (2023) 8(03):108–31. doi:10.19511/j.cnki.jee.2023.03.006
69. Sauter C, Fowler H, Westra S, Ali A, Peleg N, White C. Compound extreme hourly rainfall preconditioned by heatwaves most likely in the mid-latitudes. Weather Clim Extremes (2023) 40:100563. doi:10.1016/j.wace.2023.100563
70. Wen ZL, Ye BJ. Analyses of mediating effects: the development of methods and models. Adv Psychol Sci (2014) 22(05):731–45. doi:10.3724/SP.J.1042.2014.00731
71. Bertrand M, Mullainathan S. Are CEOs rewarded for luck? The ones without principals are. Q J Econ (2001) 116(3):901–32. doi:10.1162/00335530152466269
72. Ge XY, Zhuang JL, Liu Y. How does trade policy uncertainty affect bank risk—an examination of the firm performance channel. China Ind Econ (2021) 8:133–51. doi:10.3969/j.issn.1006-480X.2021.08.008
73. Yeung G. Chinese state-owned commercial banks in reform: inefficient and yet credible and functional? J Chin Governance (2021) 6(2):198–231. doi:10.1080/23812346.2020.1772537
74. Jia S, Yang C, Wang M, Failler P. Heterogeneous impact of land-use on climate change: study from a spatial perspective. Front Environ Sci (2022) 10:840603. doi:10.3389/fenvs.2022.840603
75. Zhu MX. Research on the formation mechanism of China's listed commercial banks’ innovative capability and its influence on bank performance. Shandong: Shandong University (2013).
76. Hao Z. Risk sources of small and medium sized commercial banks in China. Doctoral dissertation, ISCTE-Instituto Universitario de Lisboa Portugal (2022).
77. Zhou SD, Zhou WK, Zhu GH, Wang CX, Wang Y. Impact of climate change on agriculture and its countermeasures. J Nanjing Agric Univ (Social Sci Edition) (2010) 10(01):34–9. doi:10.3969/j.issn.1671-7465.2010.01.006
78. Liu J, Xu XF, Luo H. Empirical study on the impact of extreme weather and climate events on agricultural economic output in China. Scientia Sinica (Terrae) (2012) 42(7):1076–82.
79. Pan GX, Gao M, Hu GH, et al. Issues and challenges on mitigation of climate change impacts on China's future agriculture. J Agro-Environment Sci (2011) 30(9):1707–12.
80. Yu SA. The impact of climate change on crop production. An empirical study in Zhejiang. Zhejiang: Zhejiang University (2019). doi:10.27461/d.cnki.gzjdx.2019.000671
81. Jiang SX, Chen YC. Monetary policy, bank capital and risk-taking. J Financial Res (2012)(04) 1–16.
82. Yu GL, Chen TT, Zhao FQ. The influence of air temperature and temperature variability on menta health. Adv Psychol Sci (2020) 28(8):1282–92. doi:10.3724/SP.J.1042.2020.01282
83. Ko J, Leung CK, Chen X, Palmer DA. From emissions to emotions: exploring the impact of climate change on happiness across 140 countries. Glob Transitions (2024) 6:231–40. doi:10.1016/j.glt.2024.10.005
84. Huang QH. The impact of extreme temperature to labor income: evidence from Chinese manufacturing firms Emission-Water saving-Employment Impact in China. Shandong: Shandong University (2023).
85. Wang CC, Lin QQ. How does bad weather affect labor productivity? — Evidence from the adaptation behavior of couriers. China Econ Q (2021) 21(03):797–818. doi:10.13821/j.cnki.ceq.2021.03.03
86. Zhang YH, Shi QH, Gu HY. A theoretical and positive study on the demand of crop insurance. J Quantitative and Technol Econ (2007) 24(4):65–75. doi:10.3969/j.issn.1000-3894.2007.04.007
87. Wu F, Hu HZ, Lin HY, Ren XY. Enterprise digital transformation and capital market performance: empirical evidence from stock liquidity. J Manage World (2021) 37(07):130–44. doi:10.19744/j.cnki.11-1235/f.2021.0097
88. Qi H, Zhou YS, He LF. Digital transformation and the improvement of the dual performance of rural financial institutions. China Rural Surv (2024) 1:108–26. doi:10.20074/j.cnki.11-3586/f.2024.01.006
89. Cuesta C, Ruesta M, Tuesta D, Urbiola P. The digital transformation of the banking industry. BBVA Res (2015) 1(4):1–10. doi:10.1109/TMAG.2013.2243424
Keywords: climate risk, local commercial banks, soundness, insurance, digitalization
Citation: Xu Y, Wang Y, Xie M and Guo C (2025) The impact of climate risk on the soundness of local commercial banks in China—based on physical risk and transition risk. Front. Phys. 13:1550471. doi: 10.3389/fphy.2025.1550471
Received: 23 December 2024; Accepted: 13 February 2025;
Published: 31 March 2025.
Edited by:
Ze Wang, Capital Normal University, ChinaReviewed by:
Xiaoyun Xing, Beijing Forestry University, ChinaCopyright © 2025 Xu, Wang, Xie and Guo. This is an open-access article distributed under the terms of the Creative Commons Attribution License (CC BY). The use, distribution or reproduction in other forums is permitted, provided the original author(s) and the copyright owner(s) are credited and that the original publication in this journal is cited, in accordance with accepted academic practice. No use, distribution or reproduction is permitted which does not comply with these terms.
*Correspondence: Yiqiu Wang, d2FuZ3lpcWl1QG5qYXUuZWR1LmNu; Yang Xu, eHV5YW5nQHN0dS5uamF1LmVkdS5jbg==
Disclaimer: All claims expressed in this article are solely those of the authors and do not necessarily represent those of their affiliated organizations, or those of the publisher, the editors and the reviewers. Any product that may be evaluated in this article or claim that may be made by its manufacturer is not guaranteed or endorsed by the publisher.
Research integrity at Frontiers
Learn more about the work of our research integrity team to safeguard the quality of each article we publish.