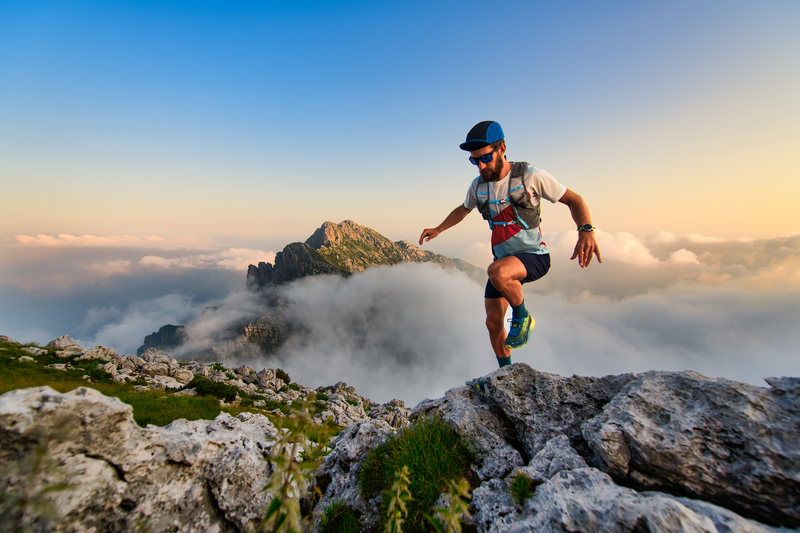
94% of researchers rate our articles as excellent or good
Learn more about the work of our research integrity team to safeguard the quality of each article we publish.
Find out more
ORIGINAL RESEARCH article
Front. Phys.
Sec. Social Physics
Volume 13 - 2025 | doi: 10.3389/fphy.2025.1548204
The final, formatted version of the article will be published soon.
You have multiple emails registered with Frontiers:
Please enter your email address:
If you already have an account, please login
You don't have a Frontiers account ? You can register here
The structure of the bank-firm loan network is crucial for understanding the transmission of systemic risk within the banking system. Drawing on complex network theory, this study analyzes loan data from 370 Chinese commercial banks spanning January 2013 to December 2023 to construct a syndicated loan network, wherein different banks lend to the same enterprise. This analysis reveals how the structure of this network influences systemic risk in the banking sector across various periods. Our findings indicate that, in the long term, network density and centralization significantly mitigate systemic risk, whereas transitivity and average clustering coefficients have a positive effect on systemic risk. In the short term, the network demonstrates strong mean-reverting properties. Additionally, we observe a noteworthy phenomenon: the bankfirm loan relationships exhibit a 'core-periphery' hierarchical structure, characterized by a network that is both robust and fragile. These insights offer a novel perspective on the relationship between bank network structures and systemic risk, contributing to the interdisciplinary application of physics in economic and financial studies.
Keywords: bank-firm loan network, Systemic Risk, Topological Structure, network formation, ECM
Received: 19 Dec 2024; Accepted: 24 Feb 2025.
Copyright: © 2025 Li, Fu and Li. This is an open-access article distributed under the terms of the Creative Commons Attribution License (CC BY). The use, distribution or reproduction in other forums is permitted, provided the original author(s) or licensor are credited and that the original publication in this journal is cited, in accordance with accepted academic practice. No use, distribution or reproduction is permitted which does not comply with these terms.
* Correspondence:
Zhengyong Li, Lanzhou University of Finance and Economics, Lanzhou, China
Disclaimer: All claims expressed in this article are solely those of the authors and do not necessarily represent those of their affiliated organizations, or those of the publisher, the editors and the reviewers. Any product that may be evaluated in this article or claim that may be made by its manufacturer is not guaranteed or endorsed by the publisher.
Research integrity at Frontiers
Learn more about the work of our research integrity team to safeguard the quality of each article we publish.