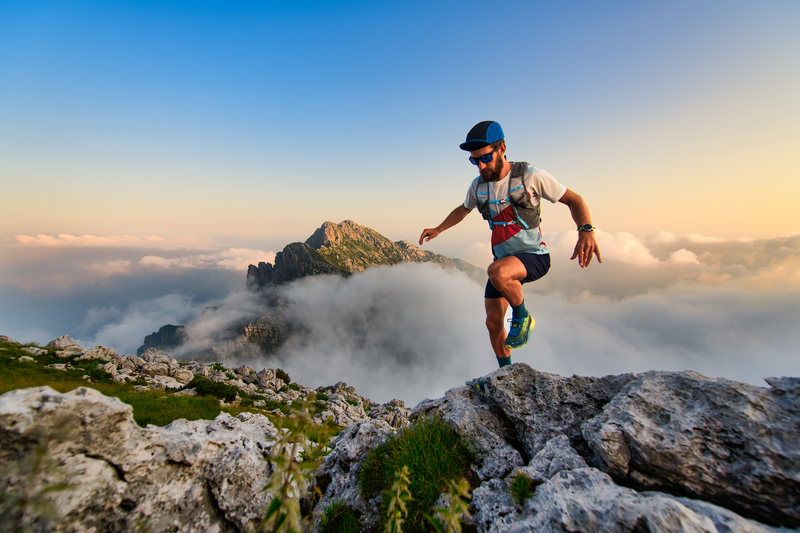
94% of researchers rate our articles as excellent or good
Learn more about the work of our research integrity team to safeguard the quality of each article we publish.
Find out more
ORIGINAL RESEARCH article
Front. Phys.
Sec. Interdisciplinary Physics
Volume 13 - 2025 | doi: 10.3389/fphy.2025.1546620
The final, formatted version of the article will be published soon.
You have multiple emails registered with Frontiers:
Please enter your email address:
If you already have an account, please login
You don't have a Frontiers account ? You can register here
Advances in deep learning methods have demonstrated remarkable development in wheelset fault diagnosis. The currently popular deep neural networks suffer from design flaws in their network structure, including low accuracy, large computational complexity, limitations in frequency domain analysis and long time series feature encoding. To tackle the aforementioned issues, a Transformer network model based on dual convolutional neural network and cross-attention enhancement (Trans-DCC) is proposed for wheelset bearing fault diagnosis. Firstly, a dual feature fusion mechanism is designed in the first layer of the Transformer encoder. The dual feature fusion mechanism consists of dual convolutional neural networks(CNNs), which fully extracts low-level features from the timefrequency signals and reduces the complexity of the subsequent attention calculations. Secondly, a cross-attention model is established to the last layer in the Transformer encoder, which integrates the multihead attention mechanism, the time-domain feature and frequency-domain feature extracted by feedforward connection layer. By calculating the attention weights, the model focuses more on important features before performing feature enhancement fusion; Finally, fully connected layers and softmax functions are used as classifier to realize the fualt diagnosis of the wheelset bearings. By analyzing the train wheelset bearing dataset, the examination demonstrates the accuracy and effectiveness of the Trans-DCC.
Keywords: transformer network, dual convolutional neural network, cross-attention, Wheelset bearing, Fault diagnosis
Received: 17 Dec 2024; Accepted: 26 Mar 2025.
Copyright: © 2025 Zhao, Li and Ma. This is an open-access article distributed under the terms of the Creative Commons Attribution License (CC BY). The use, distribution or reproduction in other forums is permitted, provided the original author(s) or licensor are credited and that the original publication in this journal is cited, in accordance with accepted academic practice. No use, distribution or reproduction is permitted which does not comply with these terms.
* Correspondence:
Zengqiang Ma, Shijiazhuang Tiedao University, Shijiazhuang, China
Disclaimer: All claims expressed in this article are solely those of the authors and do not necessarily represent those of their affiliated organizations, or those of the publisher, the editors and the reviewers. Any product that may be evaluated in this article or claim that may be made by its manufacturer is not guaranteed or endorsed by the publisher.
Research integrity at Frontiers
Learn more about the work of our research integrity team to safeguard the quality of each article we publish.