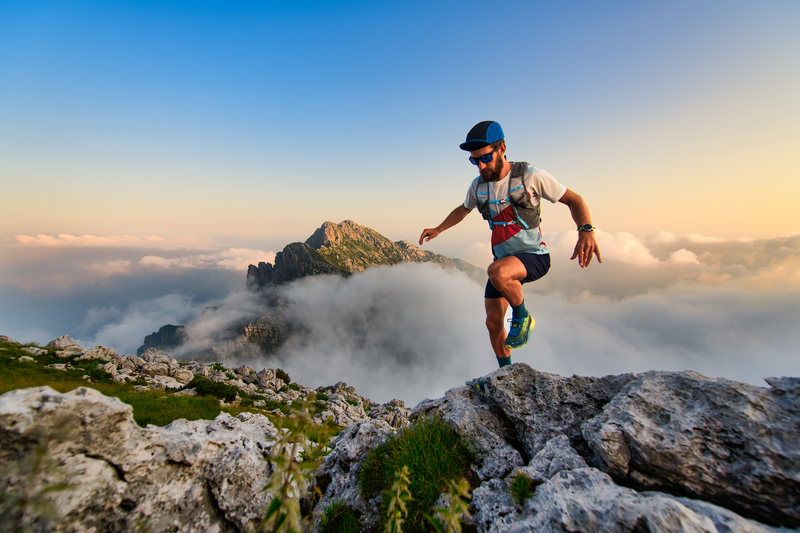
94% of researchers rate our articles as excellent or good
Learn more about the work of our research integrity team to safeguard the quality of each article we publish.
Find out more
TECHNOLOGY AND CODE article
Front. Phys.
Sec. Social Physics
Volume 13 - 2025 | doi: 10.3389/fphy.2025.1542666
This article is part of the Research Topic Real-World Applications of Game Theory and Optimization, Volume II View all 8 articles
The final, formatted version of the article will be published soon.
You have multiple emails registered with Frontiers:
Please enter your email address:
If you already have an account, please login
You don't have a Frontiers account ? You can register here
The Internet of Vehicles, as a new generation of information infrastructure that integrates multiple industries such as automotive, information communication, and transportation, is currently in a rapid development stage. However, its data supply chain involves numerous stakeholders and faces severe challenges in terms of data sharing, security, and regulation. To address this issue, this paper utilizes evolutionary game theory, setting key variables such as the strategy set, probability combination, and game behavior of each stakeholder to construct a tripartite evolutionary game model and its replicator dynamic equations, involving the Internet of Vehicles data sharing platform, vehicle manufacturers, and sellers. We studied the equilibrium solutions of this model and conducted an in-depth analysis of the local stability of the equilibrium state. Through simulation analysis, we explored the interference factors and their mechanisms of action in the interaction and dynamic changes during the evolutionary process and analyzed the impact of different parameters on the system's evolution. The experimental results show that compensation mechanisms and the risk of information leakage have a significant impact on decision-making behavior; enhancing the security technology of the data-sharing platform and the construction of the data governance system, as well as implementing corresponding incentive and punitive measures, can promote the system to reach a stable state. The results of this study provide a scientific and reasonable decision-making basis for core enterprises in the Internet of Vehicles data supply chain, helping them to more effectively supervise and coordinate the data sharing behavior of downstream enterprises, thereby enhancing the collaborative effect of the entire supply chain system and improving the overall competitiveness and stability of the supply chain.
Keywords: Internet of vehicles, data sharing, Stakeholders, evolutionary game, Simulation analysis
Received: 10 Dec 2024; Accepted: 24 Feb 2025.
Copyright: © 2025 Wu. This is an open-access article distributed under the terms of the Creative Commons Attribution License (CC BY). The use, distribution or reproduction in other forums is permitted, provided the original author(s) or licensor are credited and that the original publication in this journal is cited, in accordance with accepted academic practice. No use, distribution or reproduction is permitted which does not comply with these terms.
* Correspondence:
Ruihan Wu, School of Software, Taiyuan University of Technology, Taiyuan, China
Disclaimer: All claims expressed in this article are solely those of the authors and do not necessarily represent those of their affiliated organizations, or those of the publisher, the editors and the reviewers. Any product that may be evaluated in this article or claim that may be made by its manufacturer is not guaranteed or endorsed by the publisher.
Research integrity at Frontiers
Learn more about the work of our research integrity team to safeguard the quality of each article we publish.