- 1College of Big Data, Yunnan Agricultural University, Kunming, China
- 2Yunnan Engineering Technology Research Center of Agricultural Big Data, Yunnan Agricultural University, Kunming, China
- 3Yunnan Engineering Research Center for Big Data Intelligent Information Processing of Green Agricultural Products, Yunnan Agricultural University, Kunming, China
Introduction: With the booming development of e-commerce, agricultural product recommendation plays an increasingly important role in helping consumers discover and select products. However, the following three problems still exist in the traditional agricultural product recommendation domain: (1) the problem of missing modalities made it difficult for consumers to intuitively and comprehensively understood the product information; (2) most of them relied on shallow information about the basic attributes of agricultural products and ignored the deeper associations among the products; (3) they ignored the deeper connections among individual users and the intrinsic associations between the user embedding and the localized user representation in different modalities, which affected the accuracy of user modeling and hindered the final recommendation effect.
Methods: To address these problems, this paper innovatively proposed an agricultural product recommendation algorithm based on LLAVA and user behavioral characteristics, MP-LLaVRec(Modal Preference - Large Language and Vision Recommendation). It consisted of three main components: (1) LLAVA data enhancement, which introduced a multimodal macromodel to improve the understanding of node attributes; (2) agricultural product association relationship fusion, which constructed and improved the complex association network structure among products to ensure that the system can better understand the substitution relationship, complementary relationship, and implied consumption logic among products; (3) user modal preference feature extraction block, which deeply mined the interaction data between consumers and products, and advanced the effective user feature information from the correspondence between global user representations and local modal user representations.
Results and Discussion: We conduct experiments on a real dataset from Amazon's large-scale e-commerce platform to verify the effectiveness of MP-LLAVRec. The experimental results of four metirs, NDCG@10, NDCG@20, Recall@10 and Recall@20, showed that the method has a better performance than the baseline model.
1 Introduction
The recommendation system aims to predict products that users may be interested in based on their interests, historical interaction data, and other information, and recommend top-k products to them [1]. Traditional recommendation algorithms mainly include three approaches, namely, collaborative filtering [2] content-based filtering [3] and hybrid recommendation, which rely on user behavior, item features and a combination of both to generate recommendations, respectively. Traditionally, agricultural recommendation is mainly based on modelling the interactions between crops [4], pests, soil conditions [5] and treatments to recommend agricultural products suitable for specific climatic and geographical conditions. Secondly, by collecting and analyzing historical agricultural data [6], cultivation experience and knowledge of agricultural experts, recommender systems can be created to provide farmers with advice and guidance on cultivation, management and marketing.
In the context of agricultural e-commerce, the fundamental characteristics of consumers play a pivotal role in shaping their shopping behavior [7]. Constructing dynamic personal profiles or user group portraits not only facilitates a deeper understanding of consumers’ behavioral patterns and preferences but also allows for more accurate predictions of their future interests and needs [8]. However, users’ intentions often remain latent, embedded in the complex and nuanced interactions between consumers and products. If these latent intentions are not effectively captured and interpreted, the recommender system may be influenced by various confounding factors, ultimately compromising the accuracy and effectiveness of the recommendations [9]. In order to address the issue of information overload, personalized recommendation systems have emerged,which enhance users’ propensity to purchase by increasing homogeneity [10]. Based on the collaborative filtering algorithm, the recommendation of agricultural products made use of the user’s purchase history and preferences, and through in-depth analysis and comparison, it accurately filters out the agricultural products that are highly compatible with the user’s interests, ensuring the accuracy and effectiveness of the recommended content [11]. Recently, another study proposed a personalized agricultural knowledge service framework based on Generative Adversarial Networks (GAN) to protect user privacy. The framework combined textual CNN-LSTM algorithms for service prediction, aiming to improve the efficiency and accuracy of the recommendation system while ensuring user privacy and security [12].
When the user’s interaction information with the item or the item’s feature information is limited, these methods also encounter problems such as cold start, sparse interaction, and poor interpretability of recommendation results [13]. These issues greatly hinder the development of recommendation systems. Multimodal models are capable of capturing relationships between different modalities that cannot be accurately extracted from a single modality. Currently, the most common approach to incorporating multimodal data into recommendation algorithms is to extract the corresponding modal features from different modalities and then use the result of feature fusion as auxiliary information to represent the product [14]. In the context of e-commerce, images were frequently employed to represent products and convey a wealth of information about them [15]. VBPR [16] was the first to leverage visual feature information into recommendation algorithms, which combined the item extracted from deep network image features with traditional item feature representations to represent item features in a more comprehensive way. In recent years, with the development of graph neural networks, methods that capture user preferences by using user-item interaction graphs have demonstrated their powerful recommendation capability [14,17–19]. Therefore, fusing multimodal features with the framework of graph convolutional neural networks can not only enrich the data processing capability of recommendation algorithms, but also significantly enhance their potential for application in complex recommendation scenarios.
With the emergence of Large Multimodal Models (LMMs) such as LLAVA [20] and VisualCPM [21], the field of artificial intelligence has made significant progress in understanding and generating cross-modal content. The emergence of LMMs allows us to explore different forms of information, such as text and images, more deeply and integrate them for more comprehensive and accurate data processing and applications. Specifically, LLAVA facilitates the integration of the visual encoder with the Large Language Model (LLM) for the purpose of achieving generalized visual and linguistic understanding. LLAVA is capable of discerning human intentions in the context of visual tasks. It has been demonstrated to achieve new SoTA (state-of-the-art) accuracies when fine-tuned on ScienceQA, and it has also been shown to exhibit excellent visual chat functionality when fine-tuned on multimodal chat data [20]. Consequently, the LMM’s capabilities can be leveraged to enhance the product representation, thereby compensating for any information that may be absent from the original text. By leveraging the capabilities of LMMs, we are able to enhance product representations to compensate for information that may be missing from the original text. Taking e-commerce platforms as an example, by combining textual descriptions of products and related images, LMMs can provide richer and more comprehensive product information, which can provide users with more appropriate purchase references and help them make more informed purchasing decisions. In summary, the following issues remain to be addressed in the area of agricultural product recommendations:
1. The potential of using LMMs has not been fully explored. Although LMMs have achieved great success in other domains, their application in the field of multimodal recommendation has not been fully explored and utilized.
2. Presently, the majority of recommendation systems rely on product similarity as the primary means of modeling products. However, this approach frequently proves inadequate for fully accounting for the intricate interconnections between products. In the act of selecting products, users would not only attend to the attributes of individual items but will also considered the interrelationships between products, such as the complementarity of functions and the alignment of usage scenarios.
3. Recommendation algorithms from the user’s perspective need to be further explored. Most current agricultural product recommendation algorithms mainly focused on considering factors such as soil and crop, while user needs and preferences were often neglected. Although there have been studies focusing on recommendation algorithms from the user’s perspective, there were still some shortcomings. For instance, the impact of distinct modalities and analogous users on user modeling remains underappreciated, resulting in incomplete user feature extraction.
In order to solve the above problems, this paper has proposed an agricultural product recommendation algorithm based on LLAVA and user modal preference, MP-LLaVRec, which improved the network architecture of the FREEDOM [14] to satisfy the users’ needs and enhance the effectiveness of the recommendation system and the user experience. The main contributions of this paper are as follows:
1. We proposed an agricultural product recommendation algorithm, MP-LLAVRec, based on LLAVA and user modal preference. This model employed LLAVA to enrich product descriptions, incorporated product associations to derive more comprehensive product information, and investigated user preference representation to enhance user modeling.
2. We generated richer product performance descriptions through LLAVA, filling in the gaps of the original textual information to create more comprehensive product information.
3. We introduced product association relationships and combined with product similarities, thereby enhancing the ability to capture potential associations between products in multiple dimensions. This, in turn, optimized product modeling strategies, thus improving the accuracy of product representation.
4. A user modality preference extraction module was proposed. The user modality preference extraction module investigated the fundamental relationship between user representation and its representation on diverse modal data, thereby seeking to enhance the efficacy of multimodal recommendation systems when interactions were unclear, and to augment the precision and personalization of recommendations by analyzing the interactions between individuals with analogous preferences.
With this approach, we can fully utilize the powerful knowledge of LMM for data augmentation and accurately capture user preferences and product characteristics to provide more accurate and personalized recommendation services to users, thus improving the effectiveness of recommendation systems and user satisfaction. In comparison to the FREEDOM [14], MP-LLAVRec exhibited enhancements across all four evaluation indicators. In particular, the model demonstrated an improvement of 19.19% in Recall@10, 18.41% in Recall@20, 13.65% in NDCG@10, and 13.63% in NDCG@20, outperforming other baseline models.
2 Related work
2.1 Multimodal recommendation
Early recommendation algorithms were mainly based on collaborative filtering, which explored the similarity between users or items by using the user’s historical behavioral data or the content features of the items [22] to make personalized recommendations. With the booming development of e-commerce, social media, and other platforms, the introduction of multimodal information brings new opportunities and challenges to recommender systems. In this context, multimodal recommendation not only focused on the user’s shopping history and clicking behavior, but also provided a more personalized and accurate recommendation service for the user by integrating multi-element information such as image, text, audio, etc., which can be used to make personalized recommendations. POWERec [23] effectively and efficiently models modality-specific user interests through a single shared basic user embedding and different modality prompts.
With the rapid development of graph neural networks, this cutting-edge technology has been gradually introduced into the field of recommendation algorithms. In recommendation tasks, Graph Neural Networks (GNNs) were often used to learn node representations of users and items. GNNs are capable of not only capturing user-product relationships but also of identifying the inherent graphical structure present in the data [24]. Taking NGCF [25] as an example, the method captured user behavioral characteristics by performing iterative adjacency aggregation in the user-item view. MMGCN [17] improved recommendation performance by integrating multimodal information (e.g., text, images, etc.) to better capture user preferences for different modal contents. GRCN [18] improved recommendation performance by adaptively refining the structure of the interaction graph and identifying and pruning potential false positive edges. LATTICE [19] constructs a modality-aware graph structure learning layer that is able to learn the item graph structure from the multimodal information and combine the multimodal graphs. By exploiting graph convolution, items were able to derive useful higher-order information from the neighboring entries of their learned graph structures. FREEDOM [14] frozen item-item graphs and simultaneously denoised user-item graphs with degree-sensitive edge pruning based on LATTICE [19] for multimodal recommendation. This recommendation approach focused on exploring the potential relationship between users and items.
2.2 Recommendation algorithms based on large language models
The existing methods of using LLMs for recommendation are usually divided into two categories. The first approach is to utilize the large language model as a recommendation model. This model was based on users’ interests, browsing history, and preferences, and provides more targeted and relevant recommendations, thus increasing the likelihood that users will accept the recommendations and made purchases [26]. Approach that combined ChatGPT, traditional information retrieval, and sorting capabilities not only leverage ChatGPT’s strengths in deep understanding and natural generation, but also combines the accuracy of traditional information retrieval techniques with the efficiency of sorting techniques to improve ChatGPT’s recommendation capabilities [27]. In order to enhance the accuracy of LLM in discerning the relationship between users and products, PrOmpt Distillation employed a process of converting discrete prompts into continuous cue vectors, thereby improving the efficiency of LLM-based recommender system [28]. These studies have demonstrated the potential of LLMs as powerful recommendation models, but the research focus has mainly been on the direct use of LLMs for recommendation purposes. The second class of approaches performs data augmentation through large language models aimed at optimizing the input text for personalized content recommendation. LLMs were employed to automatically craft descriptive text for movies and books, leveraging few-shot prompting techniques for seamless integration into a recommender system [29]. The Llama4Rec enhanced the efficacy of traditional recommendation models and LLMs through the implementation of data augmentation and prompt augmentation strategies. Moreover, there were techniques for leveraging LLM to enhance graph representation, thereby improving recommendation capabilities. This was achieved by deepening the understanding of item attributes, strengthening the interaction between users and items, and analyzing user nodes in a natural language context [30]. This approach addressed the issues of data sparsity and low-quality side information in recommender systems. The results of these studies demonstrated that the augmentation and optimization of recommender systems through the use of LLMs could lead to a notable improvement in performance.
In contrast with the aforementioned methodologies, we devised a novel approach that integrated LLAVA and product associations. This strategy augmented the quality of product data and facilitated a comprehensive examination of potential inter-product relationships, thereby enhancing the precision of product representations. To ensure the accurate capture of user characteristics, a user modality preference extraction module was developed to facilitate a more in-depth examination of user representations through the construction of user preference features.
3 Materials and methods
The MP-LLaVRec is shown in Figure 1. It mainly consists of a LLaVA-based data augmentation, construction of the product isomorphism, and user modality preference extraction. Firstly, LLAVA is introduced as a data enhancement tool to solve the problem of inadequate and imprecise product descriptions in the original data set. Secondly, by integrating product association and similarity relations, a more comprehensive and accurate product representation is obtained. Finally, the user modality preference extraction module deeply mines the user preference characteristics in different modalities to identify the potential associations and mutual influences between users, thereby extracting user representations more accurately.
3.1 Problem statement
In this context, the symbols
In this matrix, if the user
3.2 LLaVA-based data augmentation
In traditional recommend systems, the quality of recommendations was closely tied to the completeness of product descriptions. Incomplete product descriptions may lead to suboptimal recommendation outcomes. To address this issue, we enhance the original product descriptions by generating corresponding text descriptions from product images using LLAVA. This supplementary approach aims to improve the overall effectiveness of the recommendations, as illustrated in Figure 2. In this study, we use a pre-trained model of LLAVA to generate textual descriptions of product images. The LLAVA model has been pre-trained on large-scale visual and linguistic data, demonstrating its excellent performance in multimodal tasks [20]. Specifically, the LLAVA-1.5 version was employed, which integrates CLIP’s visual coder and Vicuna’s linguistic decoder, fine-tuned end-to-end with instruction data generated by ChatGPT/GPT-4. Additional prompts are applied to the LMM through relevant complementary textual information that can accurately represent the produce’s functionality, instructions for use, etc. This ensures that the generated text meets the desired format and content requirements, as shown in Equation 2.
The generated text will be integrated into the original text and image as a new modality
This module offers a more comprehensive representation of information, while also employing other modal data to supplement any missing information. The incorporation of this data augmentation strategy enhances the precision and dependability of the recommender system, thereby optimizing the user experience and the quality of recommendations.
3.3 Construction of the product isomorphism
The majority of recommendation algorithms employed product similarity as a means of facilitating recommendations. Nevertheless, in the context of actual recommendations, the concept of similarity can only reflect the substitution relationship between two products. Once a user has purchased a specific product, the relevance of the substitution relationship is reduced. Consequently, greater emphasis should be placed on other attributes, such as complementarity and scene matching between products. Consequently, we integrate the associative relationships between products with their similarities to construct a more comprehensive model of products, thereby enhancing the precision of recommendations.
The cosine similarity metric is employed to assess the degree of similarity between two given produce items,as illustrated in Equation 3. This calculation is based on the embedding of the produce items in different modalities. The process of computing the cosine similarity involves identifying the k products that are most similar to the target agricultural product in the corresponding modality. This is followed by the construction of k-nearest neighbor agricultural product isomorphisms, denoted as
where
where
The factor
In order to comprehensively represent the top-k isomorphic graph of text modality pertaining to product description, it is imperative to aggregate both text modality and generative text. This aggregation is succinctly illustrated in Equation 7.
where
where
where,
3.4 User modal preference extraction
Current recommendation algorithms frequently failed to fully consider the user’s modal preference characteristics and their impact on user modeling. This results in an excessive reliance on user-product interaction in the extraction of user features. To address this issue, we propose the implementation of a user modal preference extraction module. This module employs a comprehensive approach to elucidate the intrinsic interconnections between user representations and their manifestations in disparate modal data. It is capable of discerning potential interactions between distinct users.
In the process of user-product interaction, it is common for two users to interact with the same product but provide disparate ratings. In this case, the evaluation method that relies solely on the interaction relationship and assumes that the two users possess identical interest characteristics fails to account for the discrepancy in product ratings, resulting in a certain degree of deviation in user modeling. This may ultimately lead to suboptimal recommendation model results. It is therefore necessary to consider not only the interaction between users and agricultural products, but also the data provided by user ratings. Based on the user-agricultural product heterogeneous graph, MP-LLAVRec extracts the

Figure 5. The process of establishing a user relationship network. The numbers within the graph indicate the frequency of ratings for the same products among users.
In accordance with the user relationship network
where
In order to obtain accurate representations of users with different modal preferences, a Multi-Layer Perceptron (MLP) is employed to perform feature transformation and nonlinear mapping on the user preference representation
The target user and each of its neighbor node features are aggregated through
where
In order to aggregate the information on all the constructed graphs, a multi-layer graph convolutional neural network is employed to learn and update the embedding of users with products. This is expressed in Equations 20, 21:
The embedding matrix
In MP-LLavRec, the user modal preference extraction module considers the user’s modal preferences in their entirety and assigns different affinities,
3.5 Optimization
Given the considerable amount of implicit data inherent in produce recommendation systems, particularly the absence of explicit ratings for produce items, the Bayesian Personalized Ranking (BPR Loss) method serves as the chosen loss function. BPR Loss is specifically crafted to maximize the contrast between the scores of positive and negative samples, leveraging matrix decomposition alongside user-produce rating matrices. For optimization, Bayesian maximization of the posterior probability is employed. The training data is represented as a multitude of triples
where
4 Results and discussion
4.1 Dataset
The experiments are conducted on the Garden dataset, a public review dataset provided by Amazon that covers waste materials, pesticides, and other related products. In addition to scrap and pesticides, other kinds of supplies, such as tools and seeds, are also included in the experimental data to expand its diversity and representativeness. The dataset contains information about users’ ratings of agricultural products, as well as textual information related to the agricultural products, including product names, brands, and descriptions. It is noteworthy that the dataset also provides visual information related to the products, in addition to textual information. Furthermore, in order to accurately construct the correlation relationship graph, the item relevance content in the dataset is introduced, and the details of the dataset are shown in Table 1.
4.2 Evaluation metrics
To evaluate the effectiveness of LLaVRec on the top N recommendations, we use two commonly used metrics:
where
Typically, we set N to 10 and 20. This means that we will evaluate the performance of our framework when providing the top 10 and the top 20 recommendations, respectively.
4.3 Implementation details
Following the existing work FREEDOM [14], the dataset was partitioned into training, validation, and testing sets with proportions of 80%, 10%, and 10%, respectively. This strategy was employed to ensure random selection of user-item interaction records in each set, minimizing potential bias. Additionally, all models underwent 1,000 iterations, with early stopping implemented after 20 rounds. Recall@20 was used as the stopping criterion on the validation set, and the model that achieved the best performance on the validation set was chosen for the final evaluation on the test set. MP-LLaVRec set the embedding size to 64 for all modeled users and products, and initialized the embedding parameters using the Xavier method with the Adam optimizer. Python was used as the main programming language, and Python version 3.7 was chosen, while Visual Studio Code was used as the integrated development environment [8].
For parallel computing tasks, we chose NVIDIA GeForce RTX 3090 as the graphics processing unit (GPU), along with CUDA 11.7 as the GPU parallel computing platform. This choice aims to fully utilize the computational power of GPUs and improve the efficiency of experiments. Meanwhile, we use PyTorch 1.13.1 as the deep learning framework to support model development and training in our experiments.
4.4 Baseline methods
To evaluate the effectiveness of our proposed model, we compare it with several traditional and state-of-the-art recommendation models:
VBPR [16] integrates visual features and implicit feedback data to enhance the efficacy of ranking tasks in personalized recommender systems.
MMGCN [17] exhibits the capability to capture both local and global feature information through the processing of graph data across various scales.
GRCN [18] enhances the efficacy and precision of graph convolutional networks in multimedia recommendation tasks involving implicit feedback by dynamically modifying the structure of the user-item interaction graph to identify and eliminate potential false alarm edges.
DualGNN [31] is based on user-microvideo bipartite graphs and user co-occurrence graphs, which utilise correlations between users to collaboratively mine specific fusion patterns for each user.
SLMRec [32] augments the effectiveness of recommendation algorithms by integrating graph neural networks and multi-task learning methodologies. This approach leverages data augmentation and contrastive learning techniques to unveil latent patterns within multimodal content, thereby enhancing recommendation accuracy and performance.
FREEDOM [14] elevates recommendation precision by freezing the item-item graph structure while denoising the user-item interaction graph structure. This strategy not only diminishes memory requirements but also enhances the computational efficiency of the underlying recommendation engine.
BM3 [33] eliminates the necessity for random negative instances, which facilitate interaction between users and items in the model. Instead, BM3 employs a potential embedding discard mechanism to perturb the original user’s and item’s embeddings.
DRAGON [34] improves the accuracy of recommender systems by constructing homogeneous graphs to enhance binary relationships between users and items, and by learning dual representations of users and items to capture their inter-relationships as well as intra-relationships.
MGCN [35] has been demonstrated to enhance the precision of multimedia recommender systems and the thoroughness of user preference modelling through behaviour-guided modal feature purification, multi-view information coding and behaviour-aware modal feature fusion.
POWERec [23] strategically leverages two core components: prompt information and weak modality enhancement. Initially, the algorithm integrates user interest learning with multimodal prompts to meticulously model the distinct user interests across modalities. This entails the utilization of individual user embedding alongside the integration of diverse modal prompts, consequently heightening the personalization level of recommendations. Additionally, the incorporation of weak modal enhancement training facilitates improved user interest learning within modalities exhibiting unreliable prediction outcomes.
4.5 Performance comparison
Table 2 compares the accuracy of MP-LLaVRec for product recommendation in terms of two evaluation metrics, Recall as well as NDCG, from which we can draw the following conclusions:
1) Compared to the benchmark model FREEDOM [14], MP-LLaVRec has improved in all four evaluation metrics, specifically, it has improved by 19. 19% on Recall@10, 18.41% on Recall@20, 13.65% on NDCG@10, and 13.63% on NDCG@20, outperforming the other baseline models. The improvement of the effect is mainly due to the data augmentation of agricultural products using LLAVA, the construction of agricultural product association relationships, and the representation of user preferences. Using LLAVA to enhance the data of agricultural products can effectively deal with the situation where the recommendation performance is affected by the absence of some modes. The correlation relationship of agricultural products aims to emphasize the direct relationship between products, which makes up for the shortcomings of the recommendation system based only on product similarity. The user preference representation takes the user as the first perspective, and learns the target user’s modal characteristics through its ratings related users.
2) The traditional VBPR model mainly makes recommendations based on mining implicit feedback and combining visual features. Therefore, VBPR tends to produce better results for products with strong visual elements. MMGCN, on the other hand, mainly constructs user-item graphs based on implicit feedback and adopts graph neural network message passing mechanism. In addition, MMGCN also considers the contents of different modal expressions. Therefore, on multimodal datasets, MMGCN can produce better recommendation results compared to VBPR.
3) In the graph-based multimodal recommendation algorithm, GRCN outperforms MMGCN by 15.65% on four metrics, primarily because it identifies and prunes potential noise, improving the performance of graph neural networks through graph refinement operations. SLMRec, which incorporates self-supervised learning as an auxiliary task, leverages data augmentation strategies like feature discarding and feature concealment. These techniques help the model better understand and generalize multimodal data in large datasets. However, in smaller datasets, these augmentation strategies may not have enough data to effectively capture the relationships between multimodal data, negatively affecting the representation of both user and product features. As a result, SLMRec performs worse than GRCN on this dataset.
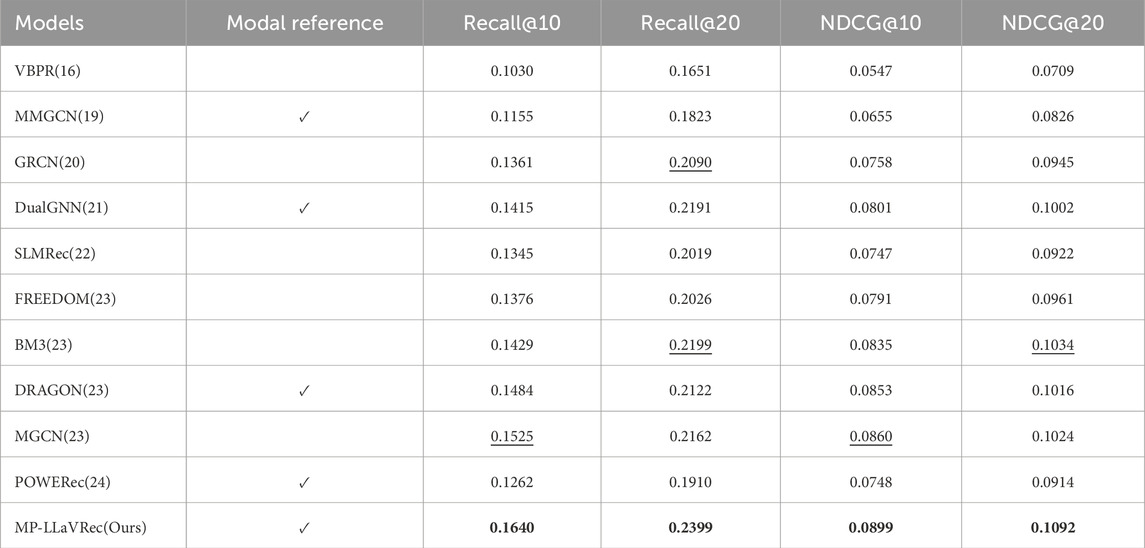
Table 2. Overall performance achieved by different recommendation methods in terms of Recall and NDCG.We mark the global best results on each dataset under each metric in boldface and the second best is underlined. Modal reference involves the consideration of different user modalities.
FREEDOM, the most effective model in the control group, excels by reducing the risk of overfitting—particularly when data is scarce—by freezing the item-item graph structure. In cases with small data volumes and noisy data, FREEDOM’s denoising strategy effectively minimizes the impact of noisy edges, ensuring accurate user representation. Compared to GRCN, FREEDOM improves all three metrics (Recall@10, NDCG@10, NDCG@20) with an average improvement of 2.38%. However, it slightly underperforms GRCN on Recall@20. This is because Recall, which measures the number of relevant items in the recommendation list, tends to be lower as the list grows. FREEDOM is more adept at capturing user interest in shorter lists, while GRCN, which dynamically updates the graph structure, performs better on longer lists. For NDCG, which accounts for the position of items in the list, FREEDOM excels at providing high-quality recommendations at the top, whereas GRCN’s flexibility allows it to maintain performance on longer lists, contributing to its better Recall@20 score.
POWERec’s Weak Modal Augmentation aims to enhance learning of user interests in weaker modalities by introducing hard negative samples. However, with smaller datasets, generating effective hard negatives is challenging, leading to poor learning and generalization. Additionally, POWERec is more susceptible to noise and outliers, whereas GRCN’s graph refinement helps reduce these effects.
The experimental results in Table 2 highlight the effectiveness of using LLAVA for product data augmentation. The introduction of associative relationships and the learning of user representations across modalities through a user relationship network with higher-order connectivity further enhance performance.
4.6 Ablation studies
MP-LLaVRec selected FREEDOM as the base model on which a series of studies were conducted to gradually introduce different key components to evaluate the impact of each component on recommendation performance. The specific components evaluated in this study were:
base + R: Based on the original model, only correlations between agricultural products are added;
base + oL: data augmentation of agricultural products using LLAVA and linking the results of the data augmentation directly to the original data without using any fusion method or improvement of the base model;
base + R + S: adding the correlations between products and the representation of user preferences to the original model;
base + L + S: the result of adding the user preference representation to the original model and using a specific fusion method combined with products data augmentation using LLAVA;
base + L + S + R(MP-LLaVRec): a specific fusion approach combined with LLAVA for data augmentation and the addition of product associations and the representation of user preferences;
We report the comparative results in the four metrics in Figure 6. The experimental results demonstrate that the integration of the LLAVA with agricultural product data leads to a significant performance improvement. This enhancement is primarily due to the LLAVA’s ability to generate more detailed product descriptions from images, effectively filling in gaps in the original textual information. As a result, the model’s capacity to process agricultural data is optimized. This improvement is especially valuable in scenarios where modal or sparse interaction data is lacking, as it enhances the model’s ability to capture the semantic intricacies of agricultural products. By augmenting the full range of product features, the data enhancement tool strengthens the model’s comprehension of the semantic subtleties inherent in agricultural data, enabling a more comprehensive interpretation.
Merely establishing correlations between products has limited impact on model performance, as simple product relationships fail to fully leverage the potential value embedded in user behavior data. However, combining product correlations with user preference representations has been shown to significantly improve model performance, highlighting the importance of the complex interplay between products in recommendation systems. This integration of user preferences is particularly beneficial, as it allows for the delivery of more personalized and targeted recommendations.
Furthermore, incorporating user modal preferences has demonstrated substantial improvements in performance, underscoring the critical role of user preferences in recommendation system. A comprehensive analysis of user-product interaction data enables the model to more accurately capture the personalized needs of users, further enhancing its effectiveness.
4.7 Hyperparameter sensitivity study
4.7.1 Text feature fusion
The text fusion ratio,
4.7.2 Multimodal feature fusion
The Multimodal Feature Fusion model extracts both image and text data from the raw data and combines it with the large multimodal model LLAVA to explore the feature representation that best represents the product. The influence of different modal information on the model is investigated by setting the initial value of the visual scale
4.7.3 Relative weights of associative relationships
The relative weights of associative relationships are of interest in this context. MP-LLaVRec integrates the associative relationships between products with the obtained similarity of agricultural products in TOP-K, with the objective of identifying the optimal degree of fusion that most accurately reflects the relationships between products. The initial value of the relative weight
5 Conclusion
In this paper, we propose a recommendation algorithm for agricultural products based on LLAVA and user modal preference called MP-LLaVRec. The model employs the inference capabilities of LLAVA to compensate for the absence of product information, thereby addressing the issue of inaccurate user modeling. We use the product performance representations generated by LLAVA to supplement the absence of original textual information and construct more comprehensive product information. In order to accurately portray user modal preference, we utilize the modal preference extraction module, which is based on users’ historical ratings and their behavioral data, to explore users’ modal preferences for different modal contents. Furthermore, the impact of associations on product representations is considered, thereby enabling the algorithm to comprehend the combination of agricultural products that may be of interest to the user. This initiative enables the recommendation system to identify users’ personalized needs with greater precision. MP-LLaVRec conducted experiments on Amazon’s public dataset and compared the results with ten baseline models from the literature. In comparison to the aforementioned baseline models, MP-LLaVRec exhibits superior performance.
Despite the significant results achieved in this study in agricultural product recommendation, there are still some shortcomings that deserve attention. For example, MP-LLaVRec’s dependence on different modalities may be uneven and the mining of domain-specific key features is still insufficient; the introduction of the LMM and multimodal selection mechanism increases the computational complexity, and there may be performance bottlenecks in large-scale or real-time scenarios. In addition, the model still has room for improvement in the dynamic trade-off between short-term and long-term interest modeling, the interpretability of recommendation results, and cross-domain adaptability. Future research can explore more efficient LMM architectures and advanced user behavior modeling techniques to further enhance model performance and applicability.
Data availability statement
The original contributions presented in the study are included in the article/supplementary material, further inquiries can be directed to the corresponding author.
Author contributions
PL: Conceptualization, Data curation, Formal Analysis, Methodology, Validation, Visualization, Writing–original draft, Writing–review and editing. LG: Conceptualization, Writing–review and editing. LZ: Funding acquisition, Writing–review and editing. LP: Writing–review and editing. CB: Conceptualization, Methodology, Writing–review and editing. LY: Project administration, Supervision, Writing–review and editing.
Funding
The author(s) declare that financial support was received for the research, authorship, and/or publication of this article. This study is financially supported by the Yunnan Provincial Science and Technology Major Project “Application and Demonstration of Digital Rural Governance Based on Big Data and Artificial Intelligence” (No. 202202AE090008) and “the Research and Demonstration on Intelligent Management of High Quality Beef Cattle Industry in Yunnan Plateau” (No. 202102AE090009).
Conflict of interest
The authors declare that the research was conducted in the absence of any commercial or financial relationships that could be construed as a potential conflict of interest.
Generative AI statement
The author(s) declare that no Generative AI was used in the creation of this manuscript.
Publisher’s note
All claims expressed in this article are solely those of the authors and do not necessarily represent those of their affiliated organizations, or those of the publisher, the editors and the reviewers. Any product that may be evaluated in this article, or claim that may be made by its manufacturer, is not guaranteed or endorsed by the publisher.
References
1. Ni J, Huang Z, Hu Y, Lin C. A two-stage embedding model for recommendation with multimodal auxiliary information. Inf Sci (2022) 582:22–37. doi:10.1016/j.ins.2021.09.006
2. Goldberg D, Nichols D, Oki BM, Terry D. Using collaborative filtering to weave an information tapestry. Commun ACM (1992) 35:61–70. doi:10.1145/138859.138867
3. Pereira N, Varma S. Survey on content based recommendation system. Int J Comput Sci Inf Technol (2016) 7:281–4.
4. Xu C, Zhao L, Wen H, Zhang Y, Zhang L. A novel cascaded multi-task method for crop prescription recommendation based on electronic medical record. Comput Electronics Agric (2024) 219:108790. doi:10.1016/j.compag.2024.108790
5. Patel K, Patel HB. A state-of-the-art survey on recommendation system and prospective extensions. Comput Electronics Agric (2020) 178:105779. doi:10.1016/j.compag.2020.105779
6. Ge W, Zhou J, Zheng P, Yuan L, Rottok LT. A recommendation model of rice fertilization using knowledge graph and case-based reasoning. Comput Electronics Agric (2024) 219:108751. doi:10.1016/j.compag.2024.108751
7. Wang Y, Lan J, Pan J, Fang L. How do consumers’ attitudes differ across their basic characteristics toward live-streaming commerce of green agricultural products: a preliminary exploration based on correspondence analysis, logistic regression and decision tree. J Retailing Consumer Serv (2024) 80:103922. doi:10.1016/j.jretconser.2024.103922
8. Xiao Y, Li C, Thürer M, Liu Y, Qu T. User preference mining based on fine-grained sentiment analysis. J Retailing Consumer Serv (2022) 68:103013. doi:10.1016/j.jretconser.2022.103013
9. Chen L, Zhu G, Liang W, Cao J, Chen Y. Keywords-enhanced contrastive learning model for travel recommendation. Inf Process and Management (2024) 61:103874. doi:10.1016/j.ipm.2024.103874
10. Molaie MM, Lee W. Economic corollaries of personalized recommendations. J Retailing Consumer Serv (2022) 68:103003. doi:10.1016/j.jretconser.2022.103003
11. Chen T, Liang Y, Huang T, Huang J, Liu C. Agricultural product recommendation model and e-commerce system based on cfr algorithm. In: 2022 IEEE 2nd International Conference on electronic technology, communication and information (ICETCI). 27-29 May 2022. IEEE (2022). p.931–4.
12. Wu H, Liu C, Zhao C. Personalized agricultural knowledge services: a framework for privacy-protected user portraits and efficient recommendation. The J Supercomputing (2024) 80:6336–55. doi:10.1007/s11227-023-05557-w
13. Wei W, Wang J, Li J, Xu M. A novel image recommendation model based on user preferences and social relationships. J King Saud University-Computer Inf Sci (2023) 35:101640. doi:10.1016/j.jksuci.2023.101640
14. Zhou X, Shen Z. A tale of two graphs: freezing and denoising graph structures for multimodal recommendation. In: Proceedings of the 31st ACM international conference on multimedia (2023). p.935–43.
15. Zhao L, Zhang M, Tu J, Li J, Zhang Y. Can users embed their user experience in user-generated images? evidence from jd. com. J Retailing Consumer Serv (2023) 74:103379. doi:10.1016/j.jretconser.2023.103379
16. He R, McAuley J. Vbpr: visual bayesian personalized ranking from implicit feedback. Proc AAAI Conf Artif intelligence (2016) 30. doi:10.1609/aaai.v30i1.9973
17. Wei Y, Wang X, Nie L, He X, Hong R, Chua T-S. Mmgcn: multi-modal graph convolution network for personalized recommendation of micro-video. In: Proceedings of the 27th ACM international conference on multimedia (2019). p.1437–45.
18. Wei Y, Wang X, Nie L, He X, Chua T-S. Graph-refined convolutional network for multimedia recommendation with implicit feedback. In: Proceedings of the 28th ACM international conference on multimedia (2020). p.3541–9.
19. Zhang J, Zhu Y, Liu Q, Wu S, Wang S, Wang L. Mining latent structures for multimedia recommendation. In: Proceedings of the 29th ACM international conference on multimedia (2021). p.3872–80.
21. Hu J, Yao Y, Wang C, Wang S, Pan Y, Chen Q, et al. Large multilingual models pivot zero-shot multimodal learning across languages. arXiv preprint arXiv:2308.12038 (2023).
22. Cao Y, Wang X, He X, Hu Z, Chua T-S. Unifying knowledge graph learning and recommendation: towards a better understanding of user preferences. In: The world wide web conference (2019). p.151–61.
23. Dong X, Song X, Tian M, Hu L. Prompt-based and weak-modality enhanced multimodal recommendation. Inf Fusion (2024) 101:101989. doi:10.1016/j.inffus.2023.101989
24. Park J, Ahn H, Kim D, Park E. Gnn-ir: examining graph neural networks for influencer recommendations in social media marketing. J Retailing Consumer Serv (2024) 78:103705. doi:10.1016/j.jretconser.2024.103705
25. Wang X, He X, Wang M, Feng F, Chua T-S. Neural graph collaborative filtering. In: Proceedings of the 42nd international ACM SIGIR conference on research and development in informationretrieval (2019). 165–74.
26. Kim J, Kim JH, Kim C, Park J. Decisions with chatgpt: reexamining choice overload in chatgpt recommendations. J Retailing Consumer Serv (2023) 75:103494. doi:10.1016/j.jretconser.2023.103494
27. Dai S, Shao N, Zhao H, Yu W, Si Z, Xu C, et al. Uncovering chatgpt’s capabilities in recommender systems. In: Proceedings of the 17th ACM conference on recommender systems (2023). p.1126–32.
28. Li L, Zhang Y, Chen L. Prompt distillation for efficient llm-based recommendation. In: Proceedings of the 32nd ACM international conference on information and knowledge management (2023). p.1348–57.
29. Acharya A, Singh B, Onoe N. Llm based generation of item-description for recommendation system. In: Proceedings of the 17th ACM conference on recommender systems (2023). p.1204–7.
30. Wei W, Ren X, Tang J, Wang Q, Su L, Cheng S, et al. Llmrec: large language models with graph augmentation for recommendation. In: Proceedings of the 17th ACM international conference on web search and data mining (2024). p.806–15.
31. Wang Q, Wei Y, Yin J, Wu J, Song X, Nie L. Dualgnn: dual graph neural network for multimedia recommendation. IEEE Trans Multimedia (2021) 25:1074–84. doi:10.1109/tmm.2021.3138298
32. Tao Z, Liu X, Xia Y, Wang X, Yang L, Huang X, et al. Self-supervised learning for multimedia recommendation. IEEE Trans Multimedia (2022) 25:5107–16. doi:10.1109/tmm.2022.3187556
33. Zhou X, Zhou H, Liu Y, Zeng Z, Miao C, Wang P, et al. Bootstrap latent representations for multi-modal recommendation. In: Proceedings of the ACM web conference 2023 (2023). p.845–54.
34. Zhou H, Zhou X, Zhang L, Shen Z. Enhancing dyadic relations with homogeneous graphs for multimodal recommendation. In: ECAI 2023. Amsterdam, Netherlands: IOS Press (2023). p.3123–30.
Keywords: data augmentation, user representations, multimodal recommendation, LLAVA, agricultural product recommendation
Citation: Li P, Gao L, Zhang L, Peng L, Bai C and Yang L (2025) MP-LLAVRec: an agricultural product recommendation algorithm based on LLAVA and user modal preference. Front. Phys. 13:1525353. doi: 10.3389/fphy.2025.1525353
Received: 09 November 2024; Accepted: 10 January 2025;
Published: 31 January 2025.
Edited by:
Minyu Feng, Southwest University, ChinaCopyright © 2025 Li, Gao, Zhang, Peng, Bai and Yang. This is an open-access article distributed under the terms of the Creative Commons Attribution License (CC BY). The use, distribution or reproduction in other forums is permitted, provided the original author(s) and the copyright owner(s) are credited and that the original publication in this journal is cited, in accordance with accepted academic practice. No use, distribution or reproduction is permitted which does not comply with these terms.
*Correspondence: Linnan Yang, MTk4NTAwOEB5bmF1LmVkdS5jbg==