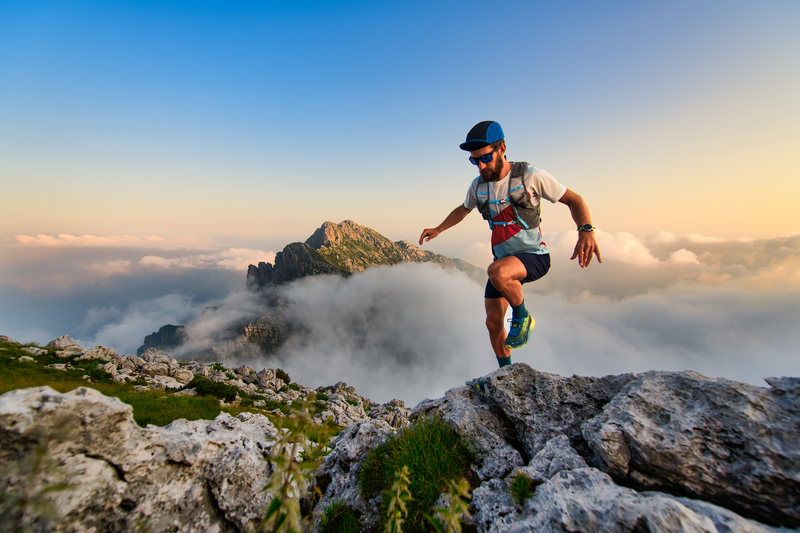
95% of researchers rate our articles as excellent or good
Learn more about the work of our research integrity team to safeguard the quality of each article we publish.
Find out more
EDITORIAL article
Front. Phys. , 09 December 2024
Sec. Radiation Detectors and Imaging
Volume 12 - 2024 | https://doi.org/10.3389/fphy.2024.1523545
This article is part of the Research Topic Pushing Frontiers - Imaging For Photon Science View all 19 articles
Editorial on the Research Topic
Pushing frontiers—imaging for photon science
The dramatic improvement in photon sources such as Free Electron Lasers (FELs) and Diffraction-Limited Storage Rings (DSLRs) over the last two decades has significantly expanded the range of science that is possible at these facilities. In order to take full advantage, detectors with similarly advanced capabilities are needed. Developing such detectors, however, is extremely challenging; they typically take a decade to deploy and often require several iterations, necessitating considerable resources. Their integration in experiments is also not trivial. As a result, many experiments are still detector limited, as described by Gruner et al.
Therefore, we have solicited papers on progress in this field. This editorial includes an overview of key challenges reported by the authors and new technologies they described that help overcome them. Of course, many other developments are underway; here, we largely focus on those submitted by the authors.
The development of new detectors for photon science presents several challenges. The first is to meet the well-documented [1, 2] performance increase of new FELs and DLSRs. Second, photon science detectors must accommodate a wide range of experimental operating modes (Gruner et al., Andresen et al., Armstrong et al.). Even within a single facility, detectors supporting a variety of applications are required (Graafsma et al.). They are also frequently adapted for experiments for which they were not originally optimized, and are increasingly fitted with multiple sensor types to address the need for wider X-ray energy ranges. Designing with all these possible cases in mind is difficult and time-consuming—and can lead to compromise solutions not optimised for any one experiment.
The range of requirements is not entirely open-ended, as some specifications have practical limits, and advancements in radiation sources can even lead to detector consolidation. For instance, at higher photon energies, there is a sensible limit to pixel size related to absorption length of secondary particles (Frojdh et al.), and the increasing brilliance of DLSRs requires event or frame rates comparable to the CW-pulse repetition rates of future FELs, suggesting that similar detectors may be suitable for both (Graafsma et al.). Despite this convergence in frame rate requirements, however, specific needs do persist for much higher frame rates (GHz) for burst imaging (Gruner et al.) and much slower, low-noise imaging (sub-Hz) for RIXS (Andresen et al.). This, and the fact that high repetition-rate CW operation at some facilities remains a long-term project, suggests requirements will remain divergent at least in the near future.
The increase in performance of modern detectors also poses challenges for downstream systems. Multi-megapixel detectors with MHz frame rates generate vast quantities of data. This needs not just to be captured but calibrated and stored for many years. The calibration itself is a major task, since for some detectors the number of parameters can exceed
A “gold standard” would be an integrating sensor with single-photon resolution which could convert to photon counts and compress to the Poisson limit for ultimate data reduction with zero science loss (Frojdh et al., Pennicard et al.).
When addressing these challenges, it is crucial to identify the primary bottleneck in the system, which could be anywhere from sensor to data transfer. If this bottleneck cannot be mitigated, optimizing other parts of the system for higher performance may be inefficient or unnecessary.
The need for detectors to span an energy regime from
Similarly, in the soft X-ray regime, several useful technologies exist. For monolithic systems such as the pnCCD (Ninkovic et al.), backside-illuminated CCDs (Goldschmidt et al.) and CMOS imagers (Andresen et al.), entrance window processing technologies have been developed that make these devices sensitive down to the double digit eV range with good efficiency and reasonable signal-to-noise ratio (SNR) thanks to their relatively low noise. High-quality entrance windows are key for any soft X-ray detector (Lee et al.). For hybrid detector systems, typically with higher noise due to the bump-bonding process, segmented LGAD sensors (Vignali et al., Sikorski et al.) and DEPFETs (Ninkovic et al.) provide good sensor options for the improvement of SNR in a different manner.
Technical advances in the commercial semiconductor market can also help improve performance. For example, CMOS technology nodes of 180 nm and below are routinely used in photon science ASICs. Their high transistor density allows much functionality to be implemented on-chip. This has enabled several developments, particularly in the high flux area. XIDER, CORDIA, and Matterhorn all use different methods to overcome challenges associated with the combined need for high frame or count rates and high dynamic range (Collonge et al., Graafsma et al., Frojdh et al.).
Cutting-edge commercial designs and even other scientific fields use much smaller nodes [3] than the 65 nm and 110 nm used here. However, commercial effort focuses on reducing the cost per transistor whereas for large area detector applications, the cost per area is most important, and this tends to increase as the node shrinks [4]. This may eventually limit what node is used for large-area applications. In addition, while smaller nodes are superior for digital circuitry, for analog circuits larger nodes have advantages as well. Older nodes may continue to be employed, or the use of chiplets to best match cost and performance may become more common.
New CMOS functionalities beyond node size also allow improved performance. However, these are sometimes not available for small-batch developments. A prominent example is 3-D integration, which has been commercially common for many years but has only been sporadically employed for photon science. Whether such technologies will permit higher-performing detectors in the future will likely be a question of access.
Advances can also be made when commercial detector systems in other fields turn out to be suitable for photon science use. In some cases, in particular in terms of cost and time, these constitute a viable or even better alternative to custom-developed systems.
Handling the vast amount of data produced by modern detectors is a particularly critical area, discussed in greater detail in the next section.
Data reduction and processing is a vast field which, even 10–15 years ago, was—at least in photon science—firmly linked to “data analysis” which occurred long after data was first recorded. Since then, source and detector advancements have resulted in a paradigm change. Today, data reduction during or shortly after detection is unavoidable to keep recorded data volumes manageable (Sobolev et al., Pennicard et al.). Reducing stored data volumes while maintaining science content may be ‘the’ key to future advances in photon science experiments. This is not merely a technical problem, but also has legal and social ramifications (Sobolev et al.).
Many in the photon science user community are reluctant to reduce raw data before detailed inspection, and data reduction is complicated by the vast range of experiments (Sobolev et al.). This contrasts with fields such as particle physics, where in-detector data reduction has been standard for decades (Pennicard et al.). For photon science, technique-specific data reduction is needed, and it is important to keep both reproducibility and improved data processing in mind—i.e., it must remain possible to recreate results from old processing tools even as improved tools allow better results or systems are updated (Schmidt et al.).
Data reduction can be carried out in the frontend ASIC itself (see ‘on-chip reduction’ examples in Pennicard et al.). However, most of the schemes submitted to this Research Topic take place in the processing FPGA or further downstream. Promising examples today often involve machine learning (ML) methods (Lin et al.). However, these are sometimes not transparent—making them difficult to understand and trust (Pennicard et al.). Partly as a result, the majority of processing is still performed without machine learning (Sztuk-Dambietz et al.), but it is clear that ML will become increasingly common.
From a detector developers’ viewpoint, the key point to realize is that the complexity of data processing and calibration depends largely on the detector design (Pennicard et al.). ASIC design decisions in particular, often among the first taken in the system design, can have a significant impact on the complexity of later data reduction processes. With ever-increasing raw data volumes, a system that delivers the most science content per recorded Gigabyte in a variety of scientific contexts is likely to become the most sought-after. Furthermore, simplifying system integration is also critical, and this is treated in the next section.
Running full-scale imaging systems at photon science facilities constitutes a challenge in itself. Partly, this is inherent in the diverse user needs and facility parameters, but it also originates in the imaging systems’ design. Prioritizing ease of operation, maintenance, calibration, and data processing during the design phase will significantly enhance user interest in the final system.
Anticipating and simplifying both assembly and disassembly of the full-scale system is crucial, as the associated risk and time in turn impacts decisions on replacement, refurbishment, or upgrades (Sztuk-Dambietz et al.). A clear and fast route to (re-)calibration is also critical in simplifying deployment and increasing adoption.
Even when designing individual components, one should keep in mind the envisioned system scale and strive for simplicity. Some detector systems have more than
To a facility, complexity is not only related to operating one imaging detector, but also to the range of systems in use. The more common components, the easier to operate the entirety of systems at a facility. Ideally, this means largely identical systems with, e.g., different geometric arrangements (AGIPD 1M vs. 4M (Graafsma et al.)) or sensor type (hybrids mated to high-Z, Si, or LGADs for instance (Graafsma et al., Hinger et al., Vignali et al., Collonge et al.)). Even “just” shared control, DAQ, or cooling systems already reduce operational complexity for the facility.
It is also important to note that the facility will choose the pragmatic route to a functional user experiment—this might mean running a well-integrated and stable detector outside its usual envelope (Sikorski et al.), or using a stable or already-installed imaging system over a fledgling, ultra-fragile one, trading stability for maximized performance. For detector development, this means that the simpler to use and optimize the system, the more likely it will actually get used at its full potential.
The bottom line to keep in mind as an imaging system developer is: “Data quality is the paramount measure of detector performance” (Sztuk-Dambietz et al.)—and too-complex calibration or module exchange can compromise this just as much as a noisy frontend.
The development and optimization of imaging detectors for photon science is a wide and vibrant field, and progress is being made on many fronts—including many outside the scope of this Research Topic. Exciting challenges remain, and new ones develop as experiments as well as sources advance. The community as a whole can look forward to the future, and the many exciting developments yet to come.
IS: Writing–original draft, Writing–review and editing. CW: Writing–original draft, Writing–review and editing. JZ: Writing–original draft, Writing–review and editing.
The author(s) declare that no financial support was received for the research, authorship, and/or publication of this article.
The authors would like to thank all the authors and reviewers involved in this Research Topic for their contributions and hard work. They would also like to acknowledge the help and advice of all their colleagues, including several fruitful discussions on specific topics. CW acknowledges support from DESY (Hamburg, Germany), a member of the Helmholtz Association HGF.
The authors declare that the research was conducted in the absence of any commercial or financial relationships that could be construed as a potential conflict of interest.
All claims expressed in this article are solely those of the authors and do not necessarily represent those of their affiliated organizations, or those of the publisher, the editors and the reviewers. Any product that may be evaluated in this article, or claim that may be made by its manufacturer, is not guaranteed or endorsed by the publisher.
1. Huang Z Brightness and coherence of synchrotron radiation and fels moycb101. Proc IPAC (Shanghai, China) (2013).
2. Pellegrini C. The history of x-ray free-electron lasers. Eur Phys J H (2012) 37:659–708. doi:10.1140/epjh/e2012-20064-5
3. Traversi G, Gaioni L, Ratti L, Re V, Riceputi E. Characterization of a 28 nm cmos technology for analog applications in high energy physics. IEEE Trans Nucl Sci (2024) 71:932–40. doi:10.1109/TNS.2024.3382348
Keywords: X-ray imaging detectors, sensors, readout ASICs, data reduction, machine learning, detector operation, synchrotrons, free-electron lasers
Citation: Sedgwick I, Wunderer CB and Zhang J (2024) Editorial: Pushing frontiers—imaging for photon science. Front. Phys. 12:1523545. doi: 10.3389/fphy.2024.1523545
Received: 06 November 2024; Accepted: 07 November 2024;
Published: 09 December 2024.
Edited and reviewed by:
Cinzia Da Via, The University of Manchester, United KingdomCopyright © 2024 Sedgwick, Wunderer and Zhang. This is an open-access article distributed under the terms of the Creative Commons Attribution License (CC BY). The use, distribution or reproduction in other forums is permitted, provided the original author(s) and the copyright owner(s) are credited and that the original publication in this journal is cited, in accordance with accepted academic practice. No use, distribution or reproduction is permitted which does not comply with these terms.
*Correspondence: Iain Sedgwick, aWFpbi5zZWRnd2lja0BzdGZjLmFjLnVr; Cornelia B. Wunderer, Y29ybmVsaWEud3VuZGVyZXJAZGVzeS5kZQ==; Jiaguo Zhang, amlhZ3VvLnpoYW5nQHBzaS5jaA==
Disclaimer: All claims expressed in this article are solely those of the authors and do not necessarily represent those of their affiliated organizations, or those of the publisher, the editors and the reviewers. Any product that may be evaluated in this article or claim that may be made by its manufacturer is not guaranteed or endorsed by the publisher.
Research integrity at Frontiers
Learn more about the work of our research integrity team to safeguard the quality of each article we publish.