- 1Big Data and Information Retrieval School, HSE University, Moscow, Russia
- 2Cybersecurity Research Center, University of Bernardo O’Higgins, Santiago, Chile
- 3Graduate School of Business, HSE University, Moscow, Russia
Our study is based on the hypothesis that stock exchanges, being nonlinear, open and dissipative systems, are capable of self-organization to the edge of a phase transition. To empirically support the hypothesis, we find segments in hourly stock volume series for 3,000 stocks of publicly traded companies, corresponding to the time of stock exchange’s stay to the edge of a phase transition. We provide a theoretical justification of the hypothesis and present a phenomenological model of stock exchange self-organization to the edge of the first-order phase transition and to the edge of the second-order phase transition. In the model, the controlling parameter is entropy as a measure of uncertainty of information about a share of a public company, guided by which stock exchange players make a decision to buy/sell it. The order parameter is determined by the number of buy/sell transactions by stock exchange players of a public company’s shares, i.e., stock’s volume. By applying statistical tests and the AUC metric, we found the most effective early warning measures from the set of investigated critical deceleration measures, multifractal measures and reconstructed phase space measures. The practical significance of our study is determined by the possibility of early warning of self-organization of stock exchanges to the edge of a phase transition and can be extended with high frequency data in the future research.
1 Introduction
More than 35 years ago, P. Bak together with C. Tang suggested that in nonlinear systems far from equilibrium, complex holistic properties may emerge through their self-organization into a critical state [1]. Subsequently, the theory of self-organized criticality (SOC) was formed, the main provisions of which have found application in sociology, biological evolution, seismology, economics and other sciences (e.g., see the papers [2–7, 7–9]). The theory of self-organization at the edge of phase transitions has found applications in cognitive and social science (e.g., see the papers [10, 11]).
The basic model of SOC theory is the sandpile cellular automaton (SCA), which demonstrates how complex holistic properties emerge in a model system with simple rules as a result of self-organization of the automaton into a critical state (e.g., see the papers [12, 13]). The simplest model of SCA is the following model. Suppose that the nodes of the lattice graph are assigned integer numbers (the number of grains of sand in the cells). Then we increase by one the numbers assigned to randomly chosen nodes of the graph (add one grain of sand in the cells). If the number (grains of sand),
Each iteration of the SCA simulation is followed by its perturbation, by adding one grain of sand to randomly selected cells at a time, and relaxation, by collapsing unstable cells. Starting from some critical iteration,
In the context of mean-field theory of phase transitions, the control parameter of the SCA is determined by the ratio of the number of particles in the cells to the total number of cells of the SCA, the order parameter is determined by the ratio of the number of unstable cells to the total number of cells of the SCA (e.g., see the paper [14]). The transition of the SCA from the subcritical phase to the critical state corresponding to the critical value of the control parameter occurs as a result of self-organization of the SCA and does not require precise adjustment of the control parameter to the critical value. This is a fundamental difference between self-organization into a critical state and a classical phase transition of the first or second kind, for which precise tuning of the control parameters to critical values is required.
Our study is based on the hypothesis that stock exchanges, being nonlinear, open and dissipative systems, are able to self-organize into a critical state. The theoretical justification of the hypothesis and a phenomenological model of stock exchange self-organization into a critical state are presented in Subsection 3.2. This econophysical model is based on the isomorphism of the SCA model and the stock exchange in the context of systems theory. In the model, the control parameter is defined by entropy as a measure of uncertainty of information about a stock of some public company, based on which the stock exchange traders make a decision to buy/sell it. The order parameter is determined by the number of buy/sell transactions by stock exchange traders of shares of some public company, i.e., stock’s volume.
To quantitatively substantiate the hypothesis, we determined time intervals corresponding to the time of the stock exchange’s stay in a critical state,
The practical significance of our study is determined by the possibility of early warning of self-organization of stock exchanges into a critical state (e.g., see the papers [15, 16]). We identified the most effective early warning measures from a wide range of investigated early warning measures (the simplest critical slowing down measures, multifractal measures and chaotic measures). The methods for computing the measures and extracting the most effective early warning measures are presented in Subsection 2.3. The results obtained and their discussion are presented in Subsection 3.3. The detection of a precursor to such self-organization gives investors a reason to pay attention to a stock that is likely to have a large trading volume expected after some time (early warning time). To the stock exchange trading regulator, precursors provide a tool to distinguish between normal market behavior and large one-off manipulations in investigations. We investigated the effectiveness of a wide range of early warning measures: simple critical slowing down measures, multifractal measures and chaotic measures.
The main conclusions, as well as the possibilities and limitations of the empirical results obtained and the proposed model are presented in Conclusion.
Existing studies on the empirical validation of stock market self-organization into a critical state are limited to the analysis of daily world stock indices (e.g., see the papers [17–23]) or daily stock prices of public company shares (e.g., see the papers [24–29]). Studies of financial series with daily intervals allow us to identify time intervals of the critical state only in the case of slow self-organization of the stock exchange into a critical state, when the time interval corresponds to several days. We used a 1 hour interval series, which enabled us to identify a large number of time intervals of several hours corresponding to stock exchange critical states, as well as intervals of several days. We also analyzed stock exchange samples of larger size (dynamic series at 1 hour intervals for stocks of more than 2,600 public companies) and used a larger number of early warning measures. Accordingly, the results we obtain are more reliable and representative than those obtained earlier. In addition, we provide a theoretical justification of the critical behavior of stock exchanges within the framework of the proposed model of self-organization into a critical state with an order parameter corresponding to the number of exchange transactions on shares of a public company.
2 Data set and methods
2.1 Model time series generated by sandpile cellular automata
As test dynamic series, that is, series to determine the required number of iterations in moving average and moving variance in the effective detection of critical iteration,
There are two main reasons why we examined the sandpile model and the time series that the model generates. First, on the sandpile model we managed to find out under which conditions we can talk about similarity in critical transitions between model and real financial data, which will be discussed in more detail in Subsection 2.2. Secondly, we used the sandpile model as a model of the stock exchange, which allowed us to theoretically justify the possibility of self-organization of the exchange at the edge of a phase transition (see Subsection 3.2).
Let
The feature of the Manna rule that distinguishes it from other rules is that each unstable vertex transmits to neighboring (connected) vertices a random number of particles that is equal to the total number of edges of that vertex.
Starting from iteration
The considered scenario of self-organization of the automaton to the critical state corresponds to its self-organization to the edge of the second-order phase transition. For self-organization of the automaton to the edge of the first-order phase transition, it is enough to consider in the Manna rule that the collapse of an unstable node
2.2 Stock volume series and time intervals for critical state
As the source of the real data, we elected to utilize hourly volumes of stock trading for the assets comprising the Russell 3,000 index (exclusive of pre- and post-market data, given their markedly lower liquidity levels), for the preceding 2 years, with the exclusion of companies experiencing data unavailability. This resulted in 2,667 time series, each comprising 3,498 observations. We elected to utilize volumes as they are more conducive to the viability assessment of the model, given that these series are more proximate to the theoretical ones and exhibit a paucity of trends in the data. As an alternative data frequency, 1-minute and 30-minute data were considered. However, both data sets exhibited an issue of mass automatic trade executions close to the astronomical hour end, resulting in a large number of singular spikes. It is possible to mitigate the impact of these automatic spikes to some extent by providing researchers with direct access to the market bids data, rather than statistical aggregates. However, in this case, we were constrained to working with the final time series.
In order to define critical transitions for systems it is necessary to create additional rules that define the criteria for such transitions. The primary criterion is that the moving average of the time series (MA100) increases by 20% in comparison to the volumes of the preceding five iterations. The secondary criterion is that this regime change persists for a minimum of 10 iterations following the transition. It should be noted that the logic described may require modification for systems exhibiting significantly different characteristics. However, in the base case scenario, it should remain equally effective.
The rationale behind the selection of these parameters is as follows:
• MA100 – modification of the first moment of the distribution, which is a well-established early warning measure. Furthermore, 100 iterations were chosen as a highly stringent threshold, enabling the removal of outliers in the data set.
• A 20% increase was selected as it defines the severity of the shift and was chosen based on the simulations with sandpile automaton with Manna rules on the Chung-Lu random graph in comparison to white noise and random walk. The 20% level was deemed appropriate for filtering jumps that occurred in the random time series, while also enabling the identification of transitions from the time series generated by complex systems.
• A comparison to the five iterations preceding the current iteration allows for the filtration of trends and the isolation of actual transitions from the data set.
• A minimum of ten iterations following the transition permits the filtration of sudden outliers that do not result in short- or mid-term changes to the system.
In order to filter time series for modelling purposes, we have elected to employ a further criterion, namely, that there must be a minimum of 800 iterations prior to the critical transition (e.g., see the paper [26]), without the occurrence of other transitions. This threshold was selected on the basis that the majority of early warning measures necessitate the availability of sufficiently wide windows in order to function effectively, without the introduction of artefacts. In this particular case, the initial 500 iterations will be utilized for this purpose, with the remaining 300 employed for prediction purposes, given that all relevant metrics have been duly calculated.
2.3 Early warning measures
In Subsection 2.3 we present a brief description of methods for computing early warning measures (EWMs) for the self-organization into a critical state. The analysis of the behavior of EWMs as the system approaches
Let
We investigated the behavior of EWMs directly related to the critical slowing down of the system (SCA and stock exchange) as it approaches
2.3.1 Measures of critical slowing down
Computationally, the simplest measures of critical deceleration are variance,
The power-law scaling exponent,
2.3.2 Multifractal measures
The specific features of the behavior of multifractal EWMs as the system approaches
To calculate the parameters of the multifractal spectrum, we used the wavelet leader method and
where
2.3.3 Measures of reconstructed phase space
As EWMs, for the calculation of which requires the reconstruction of the phase space of the dynamical system, we used the correlation dimension of the phase space,
We used the Takens theorem (see the paper [41]) to reconstruct the phase space of the stock volume series,
where
The time
To estimate the values of
where
There exists a spectrum of Lyapunov exponents characterizing the separation rate of infinitely close phase space trajectories (e.g., see the paper [43]). The largest Lyapunov exponent,
Previously (see the paper [44]), we introduced the notion of EWM, defined in terms of the number of false early warning signals,
3 Results and their discussion
3.1 Time intervals for critical state of stock exchange
Following the implementation of all filters mentioned in Subsection 2.2, a total of 967 time series were identified as exhibiting critical transitions in accordance with the predefined criteria. For all of the aforementioned time series, metrics were calculated in accordance with the specifications outlined in Subsection 2.3. Additionally, the 8-hour dynamics and variance of these instruments were calculated (as daily trading sessions on the US stock exchanges last for 8 h), which further reduced the sample size. However, the resulting observations still numbered nearly 281.4 thousand. Subsequently, observations in the time series are divided into two categories: those that are close to a critical transition and those that are not. Eight distinct closeness horizons (
In order to predict the probability of an iteration belonging to the “close to the critical transition” group, the probit model has been selected (see the paper [46]). The simplicity and high interpretability of the model would facilitate the straightforward observation of the efficiency of the measures and their derivatives. Two sets of models were constructed: one using all variables, and another with only one variable at a time. This was done to ascertain whether there were any differences in the final impact on quality prediction. In the first set of models, the importance of each variable was calculated as a share of those where the p-value of the coefficient was less than 5%. In the second set, the metric was the largest time horizon that would still achieve an AUC higher than 0.75. In addition to the AUC, two sample Kolmogorov-Smirnov (KS) tests (see the paper [47]) were employed to measure the capacity of our models to effectively differentiate between positive and negative observations.
Table 1 shows us that all of the variables (white–no statistically significant impact on the quality of the prediction, yellow–significant in some of the modifications of the variable, green–significant in most of the modifications) except for the Hurst exponent, correlation dimension and the second cumulant of wavelet leader can be at least partially useful for the task of critical transition prediction, which mostly follows previous research on this topic and tells us that at least for the financial data classification models can be applied with high level accuracy and interpretability.
3.2 Phenomenological model of stock exchange self-organization into a critical state
As shown in Subsection 3.1, a stock exchange self-organizes into a critical state and stays in this state for a certain number of hours, determined by the share of a public company that is traded on the exchange. In other words, each segment of a stock exchange has a different time duration for it to be in a critical state. By a stock exchange segment we mean a set of trading platforms (world stock exchanges) and market traders involved in buying/selling a share of some public company. Hereinafter we use the term stock exchange and understand it as a segment of the stock exchange.
A stock exchange in a critical state is characterized by a near-1 autocorrelation for stock’s volume and a power law for the power spectral density of stock’s volume with degree exponent from 1 to 2. The dynamics of a system with such characteristics is known as the avalanche-like dynamics of the system observed when it is in a critical state, also known as the edge of a phase transition (e.g., see the paper [14]). One of the first and most studied models of self-organization of systems into a critical state is the SCA model, which explains the spontaneous emergence of a system into a critical state with its avalanche-like behavior. Therefore, we used SCA not only as a system generating test dynamical series (see Subsection 2.1), but also as a basic, systemically isomorphic model of SCA in the context of systems theory, the stock market model. In other words, when building a stock exchange model, we use the analogy of structure (Chung-Lu graph of SCA and complex network of exchange transaction network), the nature of elements (stable/unstable vertices of SCA and passive/active stock exchange traders) and links (collapse of unstable vertices of SCA and buy/sell transaction of a public company share) between the elements of SCA and stock exchange.
Let
Let
Thus, each exchange trader with some number of shares can be in both an active state, denoted
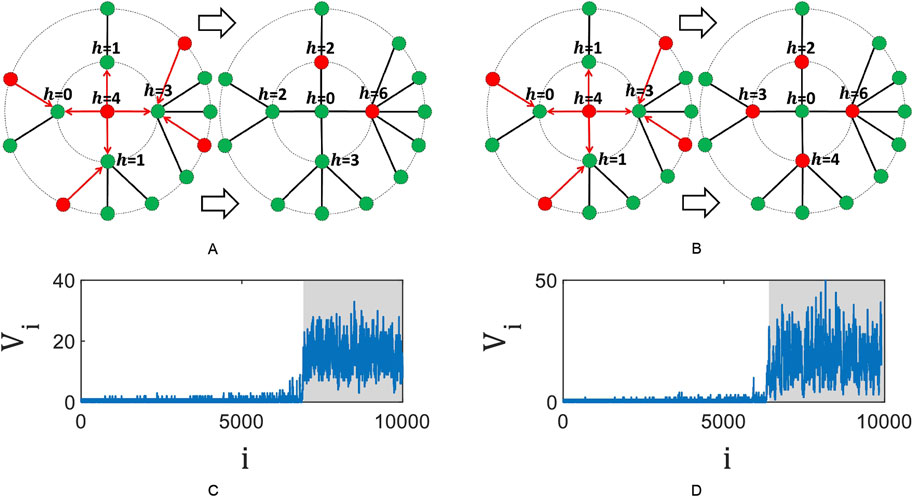
Figure 1. Local exchange transactions leading to self-organization of the stock exchange to the edge of the second-order phase transition (A) and to the edge of the first-order phase transition (B). The symbol
Self-organization of the stock exchange into a critical state occurs as a result of its pumping (perturbation) and relaxation at each iterative step. Each iteration starts with pumping and ends with complete relaxation of the stock exchange. Information pumping of the stock exchange leads to an increase in entropy or to an increase in the volatility of the stock, i.e., to an increase in the possibility of the stock price to change in any direction. Relaxation of the stock exchange occurs as a result of local exchange transactions of buying/selling a share and is formally defined by the following rules:
where
The model based on the Equation 4 explains the phenomenon of self-organization of the stock exchange into a critical state starting from some critical iteration
The above described self-organization of the stock exchange corresponds to its self-organization to the edge of the second-order phase transition. To describe the self-organization of the stock exchange to the edge of the first-order phase transition, the following changes in the rules of model (1) are sufficient. Any stock exchange trader
Note that the proposed models which are based on the Equation 5 determine the self-organization of the stock exchange into a critical state, which does not require fine-tuning of the control parameter
3.3 Early warning signals for stock exchange self-organization into a critical state
One of the results of our calculations is the independence of the behavior of the series for any of the EWMs in the vicinity of the critical onset from the specific public company for which the EWM series was calculated. The EWMs series differ only in their noise and early warning time (see Subsection 3.1). Apparently, the self-organization of a stock exchange into a critical state is a universal phenomenon. Therefore, we will limit ourselves to discussing the behavior of a series of EWMs for stock exchange transactions of, for example, Ameris Bancorp. This company is a bank holding company that, through its subsidiary Ameris Bank, provides banking services to its retail and commercial customers.
Figure 2 shows the behavior of the moving average smoothed series of EWMs that are obtained for the stock volume series of Ameris Bancorp from 10:30 7 February 2022 to 15:30 p.m. 5 February 2024. The smoothing of these series reduced the number of false early warning signals.
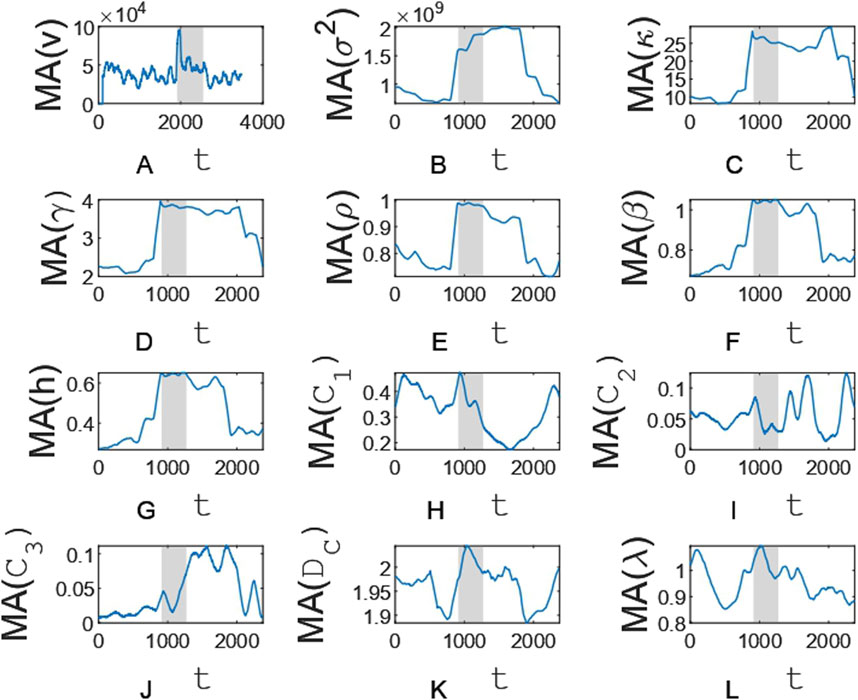
Figure 2. Moving average series for the stock volume series (A), variance (B), kurtosis (C), skewness (D), autocorrelation at lag-1 (E), power-law scaling exponent of the power spectral density (F), generalized Hurst exponent (G), position of the multifractal spectrum maximum (H), multifractal spectrum width (I), multifractal spectrum skewness (J), correlation dimension (K), and largest Lyapunov exponent (L). The gray region indicates the edge of a phase transition.
The MA100 series obtained for the stock volume series increases sharply in the vicinity of the critical point,
The above described behavior of the MA100 series is a consequence of the critical slowdown of the stock exchange, the manifestation of which is an increase in the average amplitude of stochastic fluctuations of the order parameter (stock volume). Indeed, in the vicinity of
Other evidence of the critical slowing down of the stock market in the vicinity of
Another sign of the stock volume series approaching
Let us consider the behavior of the series of EWMs, the calculation of which is based on the reconstruction of the phase space of the stock exchange. As the stock exchange approaches
4 Conclusion
The stock exchange self-organizes to the edge of a phase transition. The duration of a stock exchange at the edge ranges from 7 to 19 trading hours and depends on the public company whose shares are traded on the stock exchange. We set such durations for public company stocks from the Russel 3,000 index, which measures the performance of the 3,000 largest US companies by market capitalization. Perhaps the result of finding time intervals corresponding to the edge of a phase transition for more public company stocks would be a longer range of trading day durations. In addition, further research of the time intervals should be focused on the analysis of the stock volume series with higher frequency, such as every second and every minute series, but adjusted for the volumes of pre-planned execution of deals. Analyzing such series will allow you to identify the time intervals that cannot be identified in hourly stock volume. For example, high-frequency trading implies the conclusion of a large number of buy/sell transactions in a fraction of a second and it may take several seconds for the stock exchange to self-organize to the edge of a phase transition. If the duration of the stock exchange on the edge of a phase transition is less than 1 h, the analysis of the hourly stock volume series will not allow to identify the time interval corresponding to the edge. The best identification will be obtained when analyzing the second-by-second series for the stock volume. In addition, the transition to more frequent stock volume series will allow to obtain segments of series corresponding to the edge, of longer length and possibly of sufficient length to obtain a reliable estimate for the power-law scaling exponent of the power spectral density. Comparison of such estimates will allow us to determine which of the critical states, i.e., the edge of the phase transition of the first or second kind, corresponds to the detected time interval.
The sandpile cellular automaton model of self-organization to the edge of a phase transition is based on the idea that information drives stock markets (e.g., see the paper [54]). Self-organization of a stock exchange occurs in a discrete number of steps, each of which begins with an information perturbation of the stock exchange and ends with its relaxation. If the information pumping results in supra-critical uncertainty, or entropy, in the price behavior of a stock for some traders, then the stock exchange relaxation occurs as a result of these traders’ execution of stock buy/sell transactions, which reduces the uncertainty in the price behavior of the stock for the traders. We have considered implementations of the model under the assumption that all traders are characterized by a single critical level of uncertainty. In the context of effective market hypothesis such assumption is quite reasonable, but it is not applicable when analyzing the stock market in the context of fractal market hypothesis. Therefore, further improvement of the model should be focused on the study of the influence of the type and parameters of the probability distribution of critical uncertainty on the behaviour of the stock volume series when the stock exchange approaches the edge of a phase transition, as well as on the edge. Another direction of the model improvement is the introduction of an assumption about the existence of some critical uncertainty of price behaviour, which determines the condition of buying a share of a public company. Moreover, the critical uncertainty when buying a share is not equal to the critical uncertainty when selling it.
The studied early warning measures, first of all MA100, variance, kurtosis and skewness as the most effective ones, can be used to detect early warning signals for self-organization of the stock exchange to the edge of a phase transition in real-time early warning systems. Such signals are important for the regulator of trading on the stock exchange, as they allow detecting illegal exchange operations. The volume indicator reflects an increase or decrease in the activity of traders on the stock exchange. Therefore, early detection of the time interval in the stock volume series corresponding to the stock exchange’s edge will allow a trader to make reasonable and timely changes in his trading strategy. As a rule, traders correlate the volume indicator with the direction of the stock price movement. If the stock price is rising along with the volume, the price growth is likely to continue. High volume (25% higher than average) when the stock price reaches a new high is a harbinger of a strong increase in the stock price. Traders should refrain from selling existing shares and/or buy shares while they are cheap and sell them when they rise in price. If the share price is declining while volume is rising, the stock market is dominated by stock sellers - the trader should refrain from speculating in the stock.
Data availability statement
The datasets presented in this study can be found in online repositories. The names of the repository/repositories and accession number(s) can be found below: https://github.com/lebedevaale/early_warning_model.
Author contributions
AD: Conceptualization, Formal Analysis, Funding acquisition, Methodology, Validation, Writing–original draft. AL: Data curation, Investigation, Resources, Software, Validation, Visualization, Writing–review and editing. VK: Data curation, Funding acquisition, Project administration, Supervision, Writing–review and editing. VD: Conceptualization, Data curation, Funding acquisition, Investigation, Project administration, Visualization, Writing–review and editing.
Funding
The author(s) declare that financial support was received for the research, authorship, and/or publication of this article. The work is an output of a research project implemented as part of the Basic Research Program at the National Research University Higher School of Economics (HSE University).
Conflict of interest
The authors declare that the research was conducted in the absence of any commercial or financial relationships that could be construed as a potential conflict of interest.
Generative AI statement
The author(s) declare that no Generative AI was used in the creation of this manuscript.
Publisher’s note
All claims expressed in this article are solely those of the authors and do not necessarily represent those of their affiliated organizations, or those of the publisher, the editors and the reviewers. Any product that may be evaluated in this article, or claim that may be made by its manufacturer, is not guaranteed or endorsed by the publisher.
References
1. Bak P, Tang C, Wiesenfeld K. Self-organized criticality: an explanation of the 1/f noise. Phys Rev Lett (1987) 59:381–4. doi:10.1103/PhysRevLett.59.381
2. Watkins NW, Pruessner G, Chapman SC, Crosby NB, Jensen HJ. 25 Years of self-organized criticality: concepts and controversies. Space Sci Rev (2016) 198:3–44. doi:10.1007/s11214-015-0155-x
3. Walter N, Hinterberger T. Self-organized criticality as a framework for consciousness: a review study. Front Psychol (2022) 13:911620. doi:10.3389/fpsyg.2022.911620
4. Plenz D, Ribeiro TL, Miller SR, Kells PA, Vakili A, Capek EL. Self-organized criticality in the brain. Front Phys (2021) 9:639389. doi:10.3389/fphy.2021.639389
5. Zhukov D. How the theory of self-organized criticality explains punctuated equilibrium in social systems. Methodological Innov (2022) 15:163–77. doi:10.1177/20597991221100427
6. Tadiс B, Melnik R. Self-organized critical dynamics as a key to fundamental features of complexity in physical, biological, and social networks. Dynamics (2021) 1:181–97. doi:10.3390/dynamics1020011
7. Zhukov D. Personality and society in the theory of self-organized criticality. Changing Societies and Personalities (2023) 7:10–33. doi:10.15826/csp.2023.7.2.229
8. Smyth WD, Nash JD, Moum JN. Self-organized criticality in geophysical turbulence. Scientific Rep (2019) 9:3747. doi:10.1038/s41598-019-39869-w
9. Mikaberidze G, Plaud A, D'Souza RM. Dragon kings in self-organized criticality systems. Phys Rev Res (2023) 5:L042013. doi:10.1103/PhysRevResearch.5.L042013
10. Alodjants AP, Bazhenov AY, Khrennikov AY, Bukhanovsky AV. Mean-field theory of social laser. Scientific Rep (2022) 12:8566. doi:10.1038/s41598-022-12327-w
11. Alodjants AP, Tsarev DV, Avdyushina AE, Khrennikov AY, Boukhanovsky AV. Quantum-inspired modeling of distributed intelligence systems with artificial intelligent agents self-organization. Scientific Rep (2024) 14:15438. doi:10.1038/s41598-024-65684-z
12. Jarai AA. The sandpile cellular automaton. Complexity Comput (2018) 27:79–88. doi:10.1007/978-3-319-65558-1_6
13. Shapoval A, Shnirman M. Explanation of flicker noise with the Bak-Tang-Wiesenfeld model of self-organized criticality. Phys Rev E (2024) 110:014106. doi:10.1103/PhysRevE.110.014106
14. Buendia V, di Santo S, Bonachela JA, Muñoz MA. Feedback mechanisms for self-organization to the edge of a phase transition. Front Phys (2020) 8:333. doi:10.3389/fphy.2020.00333
15. Song S, Li H. Early warning signals for stock market crashes: empirical and analytical insights utilizing nonlinear methods. EPJ Data Sci (2024) 13:16. doi:10.1140/epjds/s13688-024-00457-2
16. Ran M, Tang Z, Chen Y, Wang Z. Early warning of systemic risk in stock market based on EEMD-LSTM. PLoS One (2024) 19:e0300741. doi:10.1371/journal.pone.0300741
17. Bartolozzi M, Leinweber D, Thomas A. Self-organized criticality and stock market dynamics: an empirical study. Physica A: Stat Mech its Appl (2005) 350:451–65. doi:10.1016/j.physa.2004.11.061
18. Stanley HE, Amaral LAN, Buldyrev SV, Gopikrishnan P, Plerou V, Salinger MA. Self-organized complexity in economics and finance. PNAS (2002) 99:2561–5. doi:10.1073/pnas.022582899
19. Goncalves CP. Chaos-induced self-organized criticality in stock market volatility-an application of smart topological data analysis. Int J Swarm Intelligence Evol Comput (2023) 12:331. doi:10.35248/2090-4908.22.12.331
20. Chandra A, Reinstein A. A study of production, stock prices and self-organized criticality. Rev Account Finance (2004) 3:20–39. doi:10.1108/eb043406
21. Kaki B, Farhang N, Safari H. Evidence of self-organized criticality in time series by the horizontal visibility graph approach. Scientific Rep (2022) 12:16835. doi:10.1038/s41598-022-20473-4
22. Diks C, Hommes C, Wang J. Critical slowing down as an early warning signal for financial crises? Empirical Econ (2019) 57:1201–28. doi:10.1007/s00181-018-1527-3
23. Kang BS, Park C, Ryu D, Song W. Phase transition phenomenon: a compound measure analysis. Physica A: Stat Mech its Appl (2015) 428:383–95. doi:10.1016/j.physa.2015.02.044
24. Biondo AE, Pluchino A, Rapisarda A. Order book, financial markets, and self-organized criticality. Chaos, Solitons and Fractals (2016) 88:196–208. doi:10.1016/j.chaos.2016.03.001
25. Schmidhuber C. Financial markets and the phase transition between water and steam. Physica A (2022) 592:126873. doi:10.1016/j.physa.2022.126873
26. Dmitriev A, Lebedev A, Kornilov V, Dmitriev V. Multifractal early warning signals about sudden changes in the stock exchange states. Complexity (2022) 2022:8177307. doi:10.1155/2022/8177307
27. Biondo AE, Pluchino A, Rapisarda A. Modeling financial markets by self-organized criticality. Phys Rev E (2015) 92:042814. doi:10.1103/PhysRevE.92.042814
28. Rao B, Yi D, Zhao C. Self-organized criticality of individual companies: an empirical study. Third Int Conf Nat Comput (ICNC 2007) (2007) 481–7. doi:10.1109/ICNC.2007.654
29. Zhang D, Zhuang Y, Tang P, Peng H, Han Q. Financial price dynamics and phase transitions in the stock markets. The Eur Phys J B (2023) 96:35. doi:10.1140/epjb/s10051-023-00501-6
30. Chung F, Lu L. Connected components in random graphs with given expected degree sequences. Ann Combinatorics (2002) 6:125–45. doi:10.1007/pl00012580
31. Manna SS. Sandpile models of self-organized criticality. Curr Sci (1999) 77:388–93. Available from: https://www.jstor.org/stable/24102958. (Accessed December 24, 2024).
32. di Santo S, Burioni R, Vezzani A, Muñoz MA. Self-organized bistability associated with first-order phase transitions. Phys Rev Lett (2016) 116:240601. doi:10.1103/PhysRevLett.116.240601
33. George SV, Kachhara S, Ambika G. Early warning signals for critical transitions in complex systems. Physica Scripta (2023) 98:072002. doi:10.1088/1402-4896/acde20
34. Zhao L, Li W, Yang C, Han J, Su Z, Zou Y. Multifractality and network analysis of phase transition. PLoS ONE (2017) 12:e0170467. doi:10.1371/journal.pone.0170467
35. Hasselman F. Early warning signals in phase space: geometric resilience loss indicators from multiplex cumulative recurrence networks. Front Physiol (2022) 13:859127. doi:10.3389/fphys.2022.859127
36. Dmitriev A, Lebedev A, Kornilov V, Dmitriev V. Twitter self-organization to the edge of a phase transition: discrete-time model and effective early warning signals in phase space. Complexity (2023) 2023:1–13. doi:10.1155/2023/3315750
37. Astfalck LC, Sykulski AM, Cripps EJ. Debiasing Welch’s method for spectral density estimation. Biometrika (2024) 111:1313–29. doi:10.1093/biomet/asae033
38. Shanshan Z, Jiang Y, He W, Mei Y, Xie X, Wan S. Detrended fluctuation analysis based on best-fit polynomial. Front Environ Sci (2022) 10. doi:10.3389/fenvs.2022.1054689
39. Achour R, Li Z, Selmi B, Wang T. A multifractal formalism for new general fractal measures. Chaos, Solitons and Fractals (2024) 181:114655. doi:10.1016/j.chaos.2024.114655
40. Nazarimehr F, Jafari S, Hashemi Golpayegani SMR, Sprott JC. Can Lyapunov exponent predict critical transitions in biological systems? Nonlinear Dyn (2017) 88:1493–500. doi:10.1007/s11071-016-3325-9
41. Takens F. Detecting strange attractors in turbulence. Dynamical systems and turbulence, warwick 1980. Lecture Notes Mathematics (1981) 898:366–81. doi:10.1007/BFb0091924
42. Weber I, Oehrn CR. NoLiTiA: an open-source toolbox for non-linear time series analysis. Front Neuroinformatics (2022) 16:876012. doi:10.3389/fninf.2022.876012
43. Rosenstein MT, Collins JJ, De Luca CJ. A practical method for calculating largest Lyapunov exponents from small data sets. Physica D: Nonlinear Phenomena (1993) 65:117–34. doi:10.1016/0167-2789(93)90009-P
44. Dmitriev A, Lebedev A, Kornilov V, Dmitriev V. Effective precursors for self-organization of complex systems into a critical state based on dynamic series data. Front Phys (2023) 11. doi:10.3389/fphy.2023.1274685
45. Mooney CZ, Duval RD. Bootstrapping: a nonparametric approach to statistical inference. Newbury Park, CA, USA: Sage Publications (1993).
46. Chib S. Analysis of multivariate probit models. Biometrika (1998) 85:347–61. doi:10.1093/biomet/85.2.347
47. Berger VW, Zhou Y. Kolmogorov-Smirnov test: overview. Wiley StatsRef: Statistics Reference Online (2024). doi:10.1002/9781118445112.stat06558
48. Tao B, Dai HN, Wu J, Ho IWH, Zheng Z, Cheang CF. Complex network analysis of the bitcoin transaction network. IEEE Trans Circuits Syst Express Briefs (2022) 69:1009–13. doi:10.1109/TCSII.2021.3127952
49. Jihun P, Cho CH, Lee JW. A perspective on complex networks in the stock market. Front Phys (2022) 10. doi:10.3389/fphy.2022.1097489
50. Huang W, Wang H, Wei Y, Chevallier J. Complex network analysis of global stock market co-movement during the COVID-19 pandemic based on intraday open-high-low-close data. Financial Innovation (2024) 10:7. doi:10.1186/s40854-023-00548-5
51. Moghadam HE, et al. Complex networks analysis in Iran stock market: the application of centrality. Physica A (2019) 531:121800. doi:10.1016/j.physa.2019.121800
52. Wang Z, Zhang G, Ma X, Wang R. Study on the stability of complex networks in the stock markets of key industries in China. Entropy (2024) 26:569. doi:10.3390/e26070569
53. Liu XF, Tse CK. A complex network perspective of world stock markets: synchronization and volatility. Int J Bifurcation Chaos (2012) 22:1250142. doi:10.1142/S0218127412501428
Keywords: phase transition, self-organized criticality, early warning signals, sandpile cellular automata, stock exchange, econophysical modeling, trading
Citation: Dmitriev A, Lebedev A, Kornilov V and Dmitriev V (2025) Self-organization of the stock exchange to the edge of a phase transition: empirical and theoretical studies. Front. Phys. 12:1508465. doi: 10.3389/fphy.2024.1508465
Received: 09 October 2024; Accepted: 24 December 2024;
Published: 22 January 2025.
Edited by:
Fei Yu, Changsha University of Science and Technology, ChinaReviewed by:
Yousef Azizi, Independent Researcher, Zanjan, IranAndrei Khrennikov, Linnaeus University, Sweden
Copyright © 2025 Dmitriev, Lebedev, Kornilov and Dmitriev. This is an open-access article distributed under the terms of the Creative Commons Attribution License (CC BY). The use, distribution or reproduction in other forums is permitted, provided the original author(s) and the copyright owner(s) are credited and that the original publication in this journal is cited, in accordance with accepted academic practice. No use, distribution or reproduction is permitted which does not comply with these terms.
*Correspondence: Andrey Dmitriev, YS5kbWl0cmlldkBoc2UucnU=