- 1School of Finance and Economics, Jiangsu University, Zhenjiang, Jiangsu, China
- 2Department of Mathematical Sciences, University of Lakki Marwat, Lakki Marwat, Pakistan
- 3School of Mechanical Engineering, Jiangsu University, Zhenjiang, Jiangsu, China
- 4Institute of Numerical Sciences, Kohat University of Science and Technology (KUST), Kohat, Pakistan
- 5Department of Quantitative Analysis, College of Business Administration, King Saud University, Riyadh, Saudi Arabia
This article aims to analyze the two-dimensional (2D) nanofluid (Ag/C2H6O2) flow past an exponentially stretched sheet. The magnetic field impact, heat source/sink, and convection in the thermal profile are taken into account. The complexity of the problem is reduced by introducing a dimensionless group of functions. The reduced model is transformed into a system of first-order ordinary differential equations (ODEs). This system is further analyzed with the artificial neural network (ANN), which is trained using the Levenberg–Marquardt algorithm. The whole dataset is sub divided into three parts: training (
1 Introduction
Fluids including air, water, and plasma are the most frequently encountered substances in human life. Without a thorough knowledge of these fluids’ transport properties, their industrial utilization is not feasible. Several industrial operations, such as the coating and coloring of constantly moving metal sheets, extrusion of polymer sheets, drawing of copper wires, extrusion of polyvinyl chloride, thin film coating on photographic films, and plastic sheets, include flows over a stretching surface. Due to its practical implications, the study of fluid flows driven by stretching surfaces is a popular topic these days. Byron [1] was the first to investigate the boundary layer flow of a viscous fluid on a continuously moving surface. Lawrence [2] achieved a crowded type solution for a 2D flow, limited by a linear stretching sheet. Numerous assumptions have been taken into consideration when analyzing this ground-breaking research. Andersson [3] investigated how slipping forces affect a stretching surface. The work of Lawrence [2] is expanded upon by Donald [4] for the 3D case. Liu [5] provided the heat transfer analysis for a second-grade electrically conducting fluid across a stretched surface. While discussing the boundary layer flow caused by an exponentially growing surface, Ishak et al. [6] considered the radiation effect. The HNF mixed convective flow phenomenon is examined by Waini et al. [7]. for an exponentially expanding/constricting surface. Gowda et al. [8]. analyzed computationally the Stefan effect for a second-grade fluid flow past a curved stretched sheet. The role of magnetic dipoles in the flow of ferromagnetic NF past a stretching sheet is presented by Gowda et al. [9] in their 2013 investigation. Asghar et al. [10] used the generalized Fourier strategy to analyze the convective heat transfer for Williamson fluid flows past an unstable sheet.
The phrase “nanofluid” was first used by Choi [11] in a study presented at the ASME Winter Annual Meeting. The thermal conductivity of the nanofluids is better than that of water, making them an innovative type of fluid, including small solid particles. Microparticle applications involve heat transfer, according to several recent inventions. Nanofluids are fluids used in conventional heat transfer that dissipate nanoscale flammable particles. Medical uses for nanofluids include the use of gold nanoparticles to treat malignant tumors and the development of tiny explosives to eliminate malignancies. Jacopo [12] investigated the convective heat transmission in nanofluids with a new type of nanofluid model. Nadeem and Lee [13]. examined the boundary layer flow of the nanofluid that flows past an elongated surface. Convective boundary conditions are used by Mustafaa et al. [14] to characterize the boundary layer flow on the exponentially stretched surface. The nanofluid phenomenon across a porous stretched surface is explained by Bhattacharyya and Layek [15]. Waqas et al. [16] studied the thermally radiative MHD nanofluid flow by utilizing the Robin conditions. Ghosh and Mukhopadhyay [17]. reported the fluxes in the NF flow past a stretching sheet. Sulaiman et al. [18] discusses the 3D flow of microorganisms that contain nanofluids. Ghosh and Mukhopadhyay [19]. described the transfer of heat for an NF flow past an exponentially declining sheet. Ali et al. [20] described numerically the nanofluid phenomenon for an exponentially expanding surface by taking non-uniform heat fluxes. The numerical study for the thermal analysis of the new wavy absorber tube within a solar system is provided by Sheikholeslami et al. [21]. They considered the two-phase model of the nanofluids that contain oil and CuO nanoparticles. They concluded that the friction factor is decreased by
Magnetohydrodynamics (MHD) is the study of how electrically conducting fluids behave under the influence of the applied magnetic field. The terms magneto (which refers to a magnetic field), hydro (which refers to a liquid), and dynamic (which refers to motion) form the term magnetohydrodynamic. This kind of fluid can be found in electrodes, liquid crystals, seawater, and solitons [25]. The conductor develops a potential when an electric field and a magnetic field move in relation to one another, which results in current flowing between the endpoints in accordance with Faraday’s law of electromagnetic induction [26–28]. This law is used to create MHD power. Currents may flow through an electrically conductive fluid that is flowing through magnetic fields, polarizing the fluid and altering the magnetic field in the process. Alfvén [29] referred to such a fluid having magnetohydrodynamics (MHD). The dynamics of the microorganisms for the Carreau–Yasuda layer is analyzed by Asghar et al. [30]. The mechanism and uses of the MHD flow in a variety of industrial processes have been the subject of numerous studies [31–33]. Benos et al. [34] used the Hamilton–Crosser model to theoretically examine the natural convective MHD flow of CNT-based NF. Asghar et al. [35] studied the flow past a wavy curved sheet in the presence of low Reynolds number. In another study, Asghar et al. [36]. analyzed the bacterial motion past a slime with the Oldroyd-4 constant. A more recent survey can be found in Refs. [37–40]. The solution strategy is important for the analysis of the nonlinear problems [41]. Recently, artificial intelligence (AI) methodologies have been broadly used for a variety of nonlinear problems. Among them, Shafiq et al. [42, 43]. used the ANN for the analysis of the exponential distribution. They have analyzed the Weibull distribution through the ANN and compared the results by using a numerical strategy. Bhadauria et al. [44] studied the THNF flow past a cone and disk by using the supervised learning ANN approach. Ali et al. [45] studied the Ostwald–de Waele model for the flow through the cavity by using the ANN based on the Levenberg–Marquardt algorithm. Srilatha et al. [46] studied the nanofluid flow past a porous rotating disk by using the ANN. Brunton et al. [47] explained the applications of machine learning in fluid mechanics. Amini and Mohaghegh [48] analyzed the machine learning in a porous media. They implemented the ANN by considering proxy modeling. Eivazi et al. [49] studied the experimental fluid mechanics under the impact of machine learning. A more recent survey on the nanofluid flow by using the ANN can be found in Refs. [50–52].
The above analysis clarifies that the choice of nanofluids for the transfer of heat is important. In this work, we will use silver (Ag) nanoparticles in the base fluid C2H6O2 to form a new nanofluid and briefly explain the flow of Ag/C2H6O2 past an unstable stretched sheet. The magnetic parameter is applied perpendicular to the sheet along the
The layout of the article is categorized as follows: Section 2 presents a physical description of the problem together with its mathematical relation. The proposed methodology is briefly explained in Section 3, while the training procedure is explained in Section 3.1. The approximation and impact of various pertinent parameters are explained in Section 4, while the conclusion is provided in Section 5.
2 Problem formulation
We consider a constant, incompressible, and two-dimensional nanofluid flow past a nonlinear stretching surface. A magnetic field with strength
By assuming the above conditions, we have [20]
The boundary conditions from the physical problem can be written as [20, 53]
Here,
Assume the following [53]:
Now, by substituting Equation 5 into Equations 1–4, we obtain
Here,

Table 2. Thermo-physical properties of the base fluid and nanoparticles [54].
3 Proposed methodology
This work aims to implement the machine learning strategy known as the artificial neural network (ANN). The ANN is a nature-inspired algorithm that uses the structure of the human brain. It receives the input and trains the neurons in the hidden layers to produce the output. Nowadays, the ANN is widely used for different purposes, including future prediction of economic prosperity, weather prediction, and security purposes. The ANN has opened a new era in machine learning with its wide range of applications see the references [55, 56].
The ANN is also very fault-tolerant and continues its functioning even if some parts stop working. In addition, ANNs are helpful for modeling complicated systems because they can detect nonlinear correlations in data. Finally, ANNs are helpful for many real-world applications because they can accurately map inputs to outputs as suggested in the reference [57]. ANNs are based on interconnected neurons and nodes. They receive the input and perform various operations back and forth to generate the output.
We reduce the system of Equations 6–8 to a first-order system as given below:
The corresponding B.Cs are as follows:
In general, for an unknown function
We introduce the following sigmoid function to obtain the results for
3.1 Weight training
The generation of output results with the training phase in the hidden layer needs to be analyzed in detail. Before the implementation of the ANN, the systems of Equations 9, 10 are solved using the bvp4c. Bvp4c uses the finite difference scheme and implements the Lobatto IIIa formula. This formula is derived from the collection of polynomials that provide a continuous, fourth-order, accurate, and uniform solution. We assume
and
4 Results and discussions
The results obtained are presented in Figures 2–6 and Table 3. The state variables are displayed under the influence of various pertinent parameters, together with AEs, regression lines, and validation of results given in Equations 13–15. In addition, the results are presented in the form of a table for various choices of the nanofluid volume fraction.
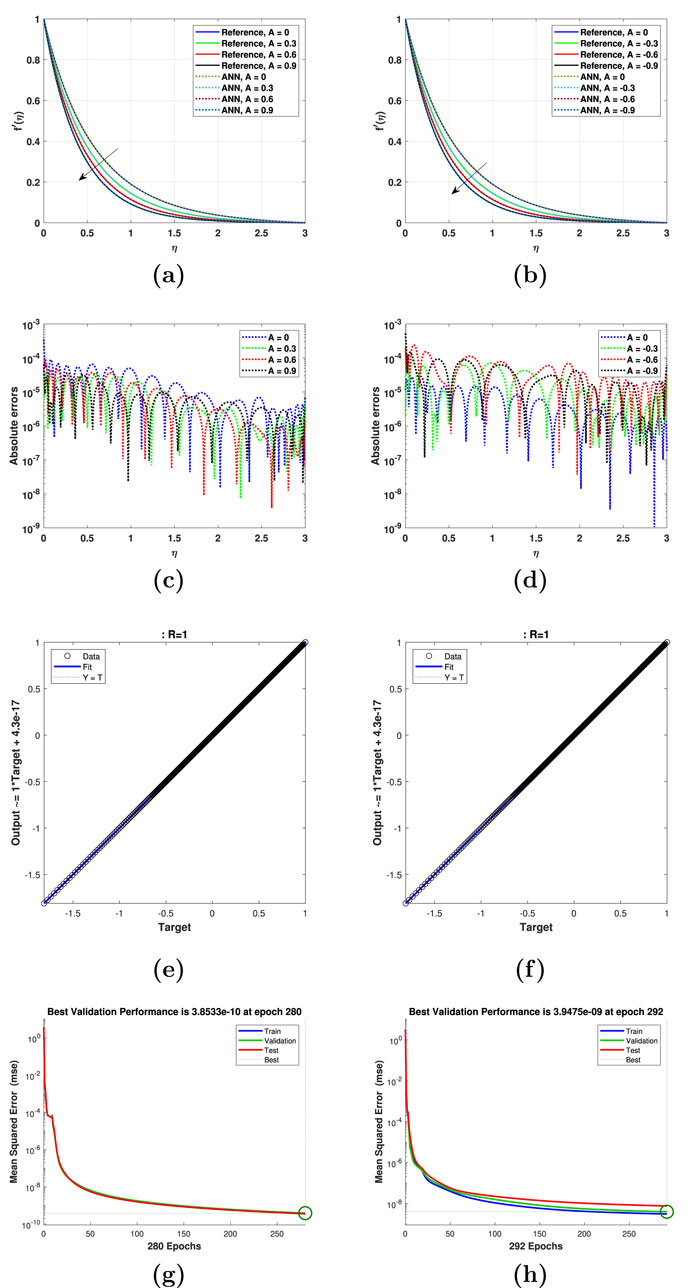
Figure 2. Impact of the space-dependent parameter
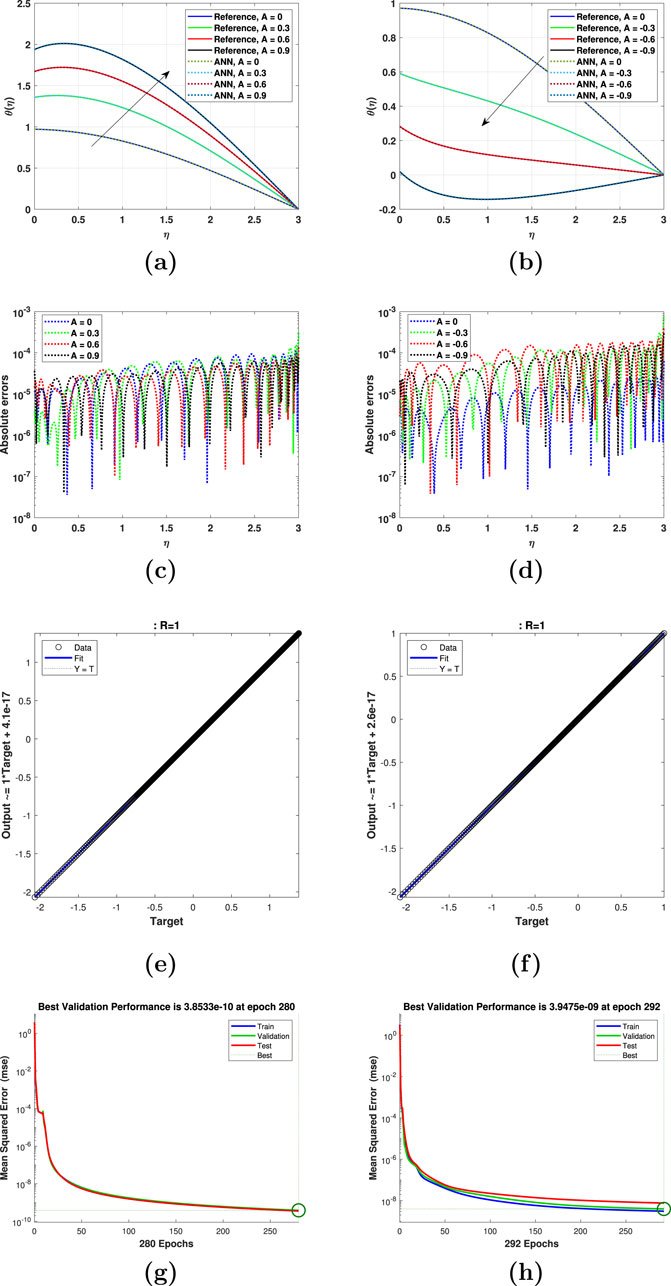
Figure 3. Impact of the space-dependent parameter
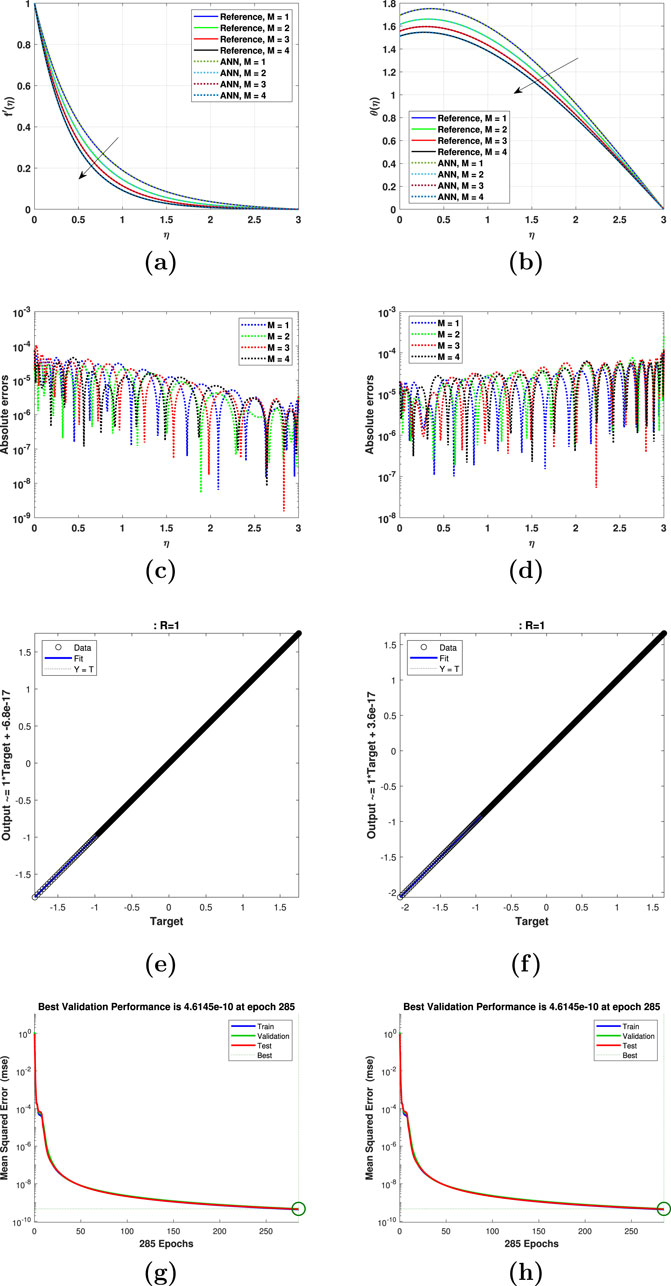
Figure 4. Impact of the magnetic parameter
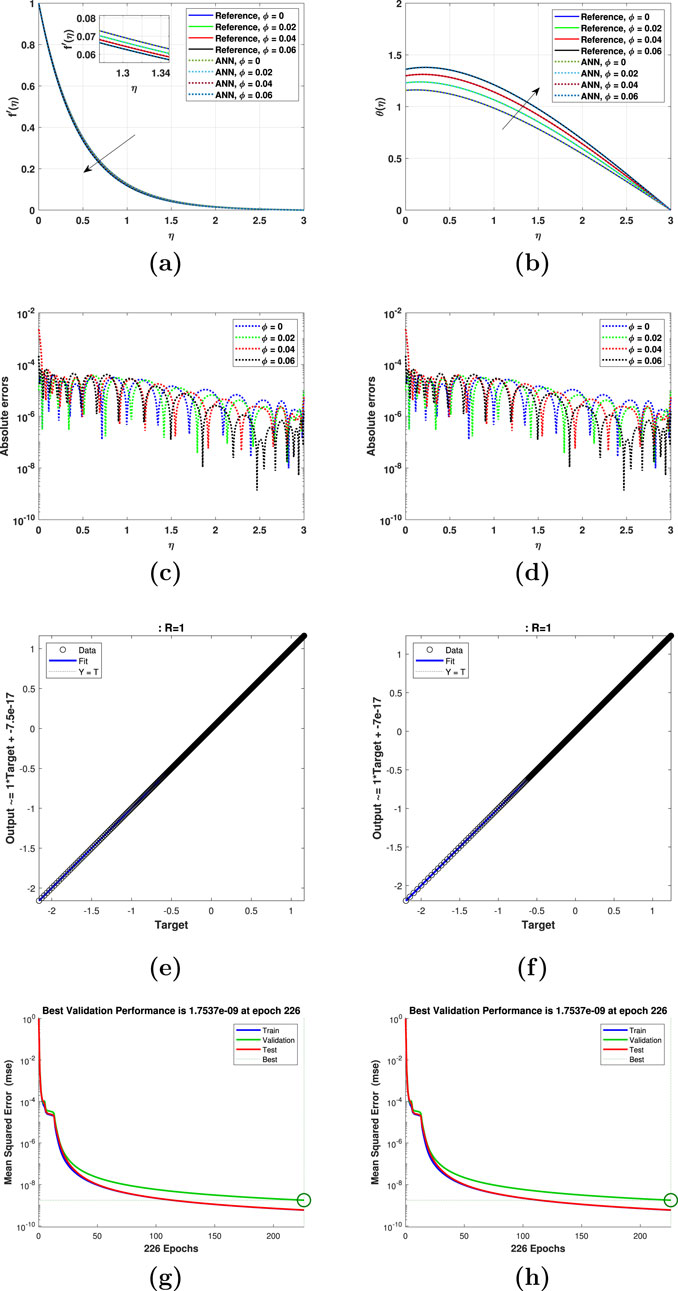
Figure 5. Impact of the nanoparticle volume fraction
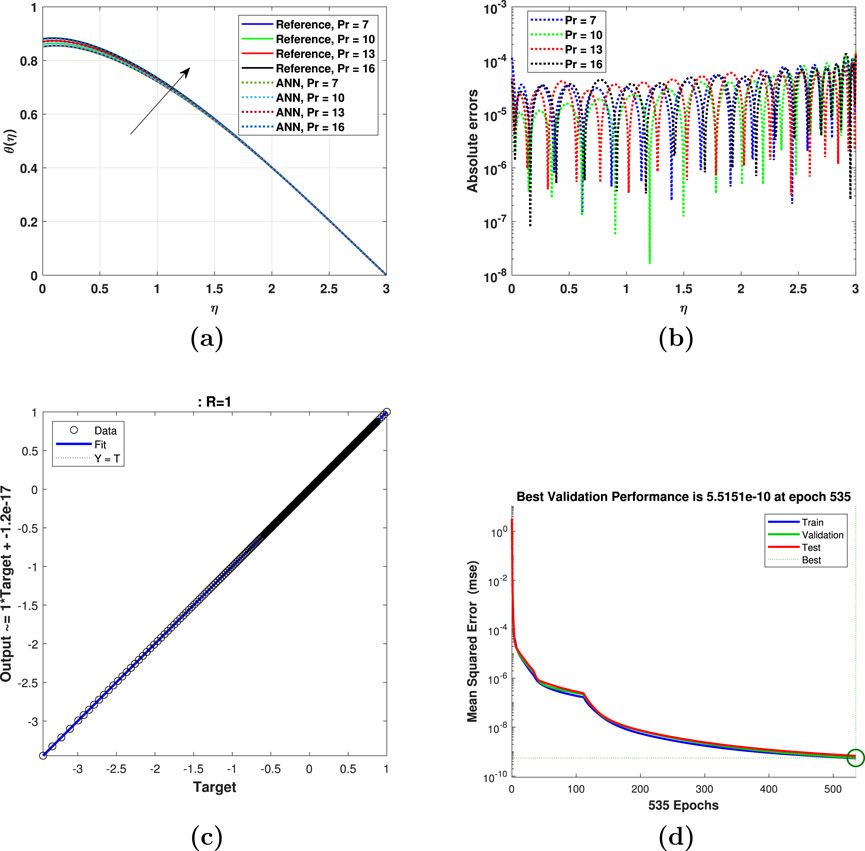
Figure 6. Impact of Prandtl number
A shown in Figure 2A, the impact of the space-dependent parameter
The impact of the magnetic parameter for its increasing values is displayed in Figure 4. Figures 4A, B shows the results for the velocity gradient and thermal profiles, respectively. The larger values of
The impact of the volume fraction
The impact of the Prandtl number
The AEs for various choices of
5 Conclusion
This article provides a comprehensive analysis of a new type of ethylene glycol-based nanofluid with silver nanoparticles. The shape of nanoparticles and other important parameters for the thermal as well as the velocity profile are discussed in detail. We observed the following points:
Data availability statement
The original contributions presented in the study are included in the article/supplementary material, further inquiries can be directed to the corresponding authors.
Author contributions
AU: conceptualization, data curation, formal analysis, methodology, software, visualization, and writing–review and editing. HY: conceptualization, formal analysis, project administration, resources, supervision, validation, visualization, and writing–original draft. Waseem: conceptualization, data curation, formal analysis, methodology, resources, software, validation, and writing–original draft. AS: data curation, formal analysis, investigation, methodology, software, validation, and writing–review and editing. FA: data curation, formal analysis, funding acquisition, investigation, project administration, software, visualization, and writing–review and editing. EI: conceptualization, data curation, formal analysis, funding acquisition, investigation, resources, software, and writing–review and editing.
Funding
The author(s) declare that financial support was received for the research, authorship, and/or publication of this article.
Acknowledgments
Researchers Supporting Project Number (RSPD2024R1060), King Saud University, Riyadh, Saudi Arabia.
Conflict of interest
The authors declare that the research was conducted in the absence of any commercial or financial relationships that could be construed as a potential conflict of interest.
Publisher’s note
All claims expressed in this article are solely those of the authors and do not necessarily represent those of their affiliated organizations, or those of the publisher, the editors, and the reviewers. Any product that may be evaluated in this article, or claim that may be made by its manufacturer, is not guaranteed or endorsed by the publisher.
References
1. Byron CS. Boundary-layer behavior on continuous solid surfaces: I. boundary-layer equations for two-dimensional and axisymmetric flow. AIChE J (1961) 7(1):26–8. doi:10.1002/aic.690070108
2. Lawrence JC. Flow past a stretching plate. Z für Angew Mathematik Physik ZAMP (1970) 21:645–647. doi:10.1007/BF01587695
3. Andersson HI. Slip flow past a stretching surface. Acta Mechanica (2002) 158(1-2):121–5. doi:10.1007/bf01463174
4. Donald Ariel P. Generalized three-dimensional flow due to a stretching sheet. ZAMM-Journal Appl Mathematics Mechanics/Zeitschrift für Angew Mathematik Mechanik: Appl Mathematics Mech (2003) 83(12):844–52. doi:10.1002/zamm.200310052
5. Liu I-C. Flow and heat transfer of an electrically conducting fluid of second grade in a porous medium over a stretching sheet subject to a transverse magnetic field. Int J Non-Linear Mech (2005) 40(4):465–74. doi:10.1016/j.ijnonlinmec.2004.07.008
6. Ishak A, et al. Mhd boundary layer flow due to an exponentially stretching sheet with radiation effect. Sains Malaysiana (2011) 40(4):391–5.
7. Waini I, Ishak A, Pop I. Mixed convection flow over an exponentially stretching/shrinking vertical surface in a hybrid nanofluid. Alexandria Eng J (2020) 59(3):1881–91. doi:10.1016/j.aej.2020.05.030
8. Gowda RJP, Mehmet Baskonus H, Kumar RN, Prasannakumara BC, Prakasha DG. Computational investigation of stefan blowing effect on flow of second-grade fluid over a curved stretching sheet. Int J Appl Comput Mathematics (2021) 7(3):109. doi:10.1007/s40819-021-01041-2
9. Gowda RJP, Kumar RN, Prasannakumara BC, Nagaraja B, Gireesha BJ. Exploring magnetic dipole contribution on ferromagnetic nanofluid flow over a stretching sheet: an application of stefan blowing. J Mol Liquids (2021) 335:116215. doi:10.1016/j.molliq.2021.116215
10. Asghar Z, Kousar M, Waqas M, Irfan M, Bilal M, Khan WA. Heat generation in mixed convected williamson liquid stretching flow under generalized fourier concept. Appl Nanoscience (2020) 10:4439–44. doi:10.1007/s13204-020-01500-0
11. Choi SUS, Eastman JA. Enhancing thermal conductivity of fluids with nanoparticles. Argonne, IL (United States): Argonne National Lab.ANL (1995). Technical report.
13. Nadeem S, Lee C. Boundary layer flow of nanofluid over an exponentially stretching surface. Nanoscale Res Lett (2012) 7:94–6. doi:10.1186/1556-276x-7-94
14. Mustafaa M, Hayat T, Obaidat S. Boundary layer flow of a nanofluid over an exponentially stretching sheet with convective boundary conditions. Int J Numer Methods Heat and Fluid Flow (2013) 23(6):945–59. doi:10.1108/hff-09-2011-0179
15. Bhattacharyya K, Layek GC. Magnetohydrodynamic boundary layer flow of nanofluid over an exponentially stretching permeable sheet. Phys Res Int (2014) 2014:1–12. doi:10.1155/2014/592536
16. Waqas M, Asghar Z, Khan WA. Thermo-solutal robin conditions significance in thermally radiative nanofluid under stratification and magnetohydrodynamics. The Eur Phys J Spec Top (2021) 230(5):1307–16. doi:10.1140/epjs/s11734-021-00044-w
17. Ghosh S, Mukhopadhyay S. Nanofluid flow past an exponentially porous stretching sheet with heat and mass fluxes. Acta Technica (2016) 61:17–29.
18. Sulaiman M, Ali A, Islam S. Heat and mass transfer in three-dimensional flow of an oldroyd-b nanofluid with gyrotactic micro-organisms. Math Probl Eng (2018) 2018:1–15. doi:10.1155/2018/6790420
19. Ghosh S, Mukhopadhyay S. Flow and heat transfer of nanofluid over an exponentially shrinking porous sheet with heat and mass fluxes. Propulsion Power Res (2018) 7(3):268–75. doi:10.1016/j.jppr.2018.07.004
20. Ali A, Sajjad A, Asghar S. Thermal-diffusion and diffusion-thermo effects in a nanofluid flow with non-uniform heat flux and convective walls. J Nanofluids (2019) 8(6):1367–72. doi:10.1166/jon.2019.1683
21. Sheikholeslami M, Said Z, Jafaryar M. Hydrothermal analysis for a parabolic solar unit with wavy absorber pipe and nanofluid. Renew Energy (2022) 188:922–32. doi:10.1016/j.renene.2022.02.086
22. Sheikholeslami M. Modeling investigation for energy storage system including mixture of paraffin and zno nano-powders considering porous media. J Pet Sci Eng (2022) 219:111066. doi:10.1016/j.petrol.2022.111066
23. Gowda RJP, Rauf A, Naveen Kumar R, Prasannakumara BC, Shehzad SA. Slip flow of casson–maxwell nanofluid confined through stretchable disks. Indian J Phys (2022) 96(7):2041–9. doi:10.1007/s12648-021-02153-7
24. Asghar Z, Ali Shah R, Ali N. A computational approach to model gliding motion of an organism on a sticky slime layer over a solid substrate. Biomech Model Mechanobiology (2022) 21(5):1441–55. doi:10.1007/s10237-022-01600-6
25. Wang Y. Multiple positive solutions for mixed fractional differential system with p-laplacian operators. In: Boundary value problems (2019). p. 1–17.
26. Zhao Y, Sun Y, Liu Z, Bai Z. Basic theory of differential equations with mixed perturbations of the second type on time scales. In: Advances in difference equations (2019). p. 1–15.
27. Mi L. The exact asymptotic behavior of blow-up solutions to a highly degenerate elliptic problem. Boundary Value Probl (2015) 2015:216–2. doi:10.1186/s13661-015-0482-6
28. Asghar Z. Enhancing motility of micro-swimmers via electric and dynamical interaction effects. The Eur Phys J Plus (2023) 138(4):357. doi:10.1140/epjp/s13360-023-03963-w
30. Asghar Z, Ali Shah R, Ali N. A numerical framework for modeling the dynamics of micro-organism movement on carreau-yasuda layer. Soft Comput (2023) 27(13):8525–39. doi:10.1007/s00500-023-08236-3
31. Farooq U, Lu D, Munir S, Ramzan M, Suleman M, Hussain S. Mhd flow of maxwell fluid with nanomaterials due to an exponentially stretching surface. Scientific Rep (2019) 9(1):7312. doi:10.1038/s41598-019-43549-0
32. Guo X, Fu Z. An initial and boundary value problem of fractional jeffreys’ fluid in a porous half space. Comput and Mathematics Appl (2019) 78(6):1801–10. doi:10.1016/j.camwa.2015.11.020
33. Sharada K, Shankar B. Mhd mixed convection flow of a casson fluid over an exponentially stretching surface with the effects of soret, dufour, thermal radiation and chemical reaction. World J Mech (2015) 5(09):165–77. doi:10.4236/wjm.2015.59017
34. Th Benos L, Karvelas EG, Sarris IE. A theoretical model for the magnetohydrodynamic natural convection of a cnt-water nanofluid incorporating a renovated Hamilton-crosser model. Int J Heat Mass Transfer (2019) 135:548–60. doi:10.1016/j.ijheatmasstransfer.2019.01.148
35. Asghar Z, Khan MWS, Shatanawi W, Gondal MA, Ghaffari A. An ifdm analysis of low Reynolds number flow generated in a complex wavy curved passage formed by artificial beating cilia. Int J Mod Phys B (2023) 37(19):2350187. doi:10.1142/s0217979223501874
36. Asghar Z, Shatanawi W, Hussain S. Biomechanics of bacterial gliding motion with oldroyd-4 constant slime. The Eur Phys J Spec Top (2023) 232(6):915–25. doi:10.1140/epjs/s11734-022-00723-2
37. Zi Y, Wang Y. Positive solutions for caputo fractional differential system with coupled boundary conditions. In: Advances in difference equations (2019). p. 1–12.
38. ZeinEldin RA, Ullah A, El-Wahed Khalifa HA, Ayaz M. Analytical study of the energy loss reduction during three-dimensional engine oil-based hybrid nanofluid flow by using cattaneo–christov model. Symmetry (2023) 15(1):166. doi:10.3390/sym15010166
39. Ullah A, Fatima N, Alharbi KAM, Elattar S, Khan W, Khan W. A numerical analysis of the hybrid nanofluid (ag+ tio2+ water) flow in the presence of heat and radiation fluxes. Energies (2023) 16(3):1220. doi:10.3390/en16031220
40. Asghar Z, Khan MWS, Gondal MA, Ghaffari A. Channel flow of non-Newtonian fluid due to peristalsis under external electric and magnetic field. Proc Inst Mech Eng E: J Process Mech Eng (2022) 236(6):2670–8. doi:10.1177/09544089221097693
41. Liu Z, Ding Y, Liu C, Zhao C. Existence and uniqueness of solutions for singular fractional differential equation boundary value problem with p-laplacian. Adv Difference Equations (2020) 2020(1):83. doi:10.1186/s13662-019-2482-9
42. Shafiq A, Colak AB, Ahmad Lone S, Sindhu TN, Muhammad T. Reliability modeling and analysis of mixture of exponential distributions using artificial neural network. Math Methods Appl Sci (2024) 47(5):3308–28. doi:10.1002/mma.8178
43. Shafiq A, Çolak AB, Sindhu TN. Reliability investigation of exponentiated weibull distribution using ipl through numerical and artificial neural network modeling. Qual Reliability Eng Int (2022) 38(7):3616–31. doi:10.1002/qre.3155
44. Bhadauria BS, Yaseen M, Kumar Rawat S, Pant M, Pant M. Designing machine learning based intelligent network for assessment of heat transfer performance of ternary hybrid nanofluid flow between a cone and a disk: case of mlp feed forward neural network. Comput and Mathematics Appl (2024) 169:17–38. doi:10.1016/j.camwa.2024.06.003
45. Ali AR, Mahmood R, Asghar A, Majeed AH, Behiry MH. Ai-based predictive approach via ffb propagation in a driven-cavity of ostwald de-waele fluid using cfd-ann and levenberg–marquardt. Scientific Rep (2024) 14(1):11024. doi:10.1038/s41598-024-60401-2
46. Srilatha P, Gowda RJP, Madhu J, Nagaraja KV, Gamaoun F, Kumar RSV, et al. Designing a solid–fluid interface layer and artificial neural network in a nanofluid flow due to rotating rough and porous disk. J Therm Anal Calorim (2024) 149(2):867–78. doi:10.1007/s10973-023-12706-z
47. Brunton SL, Noack BR, Koumoutsakos P. Machine learning for fluid mechanics. Annu Rev Fluid Mech (2020) 52(1):477–508. doi:10.1146/annurev-fluid-010719-060214
48. Amini S, Mohaghegh S. Application of machine learning and artificial intelligence in proxy modeling for fluid flow in porous media. Fluids (2019) 4(3):126. doi:10.3390/fluids4030126
49. Eivazi H, Wang Y, Vinuesa R. Physics-informed deep-learning applications to experimental fluid mechanics. Meas Sci Technol (2024) 35(7):075303. doi:10.1088/1361-6501/ad3fd3
50. Shafiq A, Çolak AB, Sindhu TN. Optimization of bioconvective magnetized walter’s b nanofluid flow towards a cylindrical disk with artificial neural networks. Lubricants (2022) 10(9):209. doi:10.3390/lubricants10090209
51. Sheraz Junaid M, Aslam MN, Asim Khan M, Saleem S, Riaz MB. Thermal analysis of a viscoelastic maxwell hybrid nanofluid with graphene and polythiophene nanoparticles: insights from an artificial neural network model. Alexandria Eng J (2024) 94:193–211. doi:10.1016/j.aej.2024.03.029
52. Urooj A, Hassan QMU, Raja MAZ, Ayub K, Nisar KS, Shoaib M. Numerical treatment for radiative hybrid nanofluid flow over a stretching sheet. Results Eng (2024) 22:102209. doi:10.1016/j.rineng.2024.102209
53. Hussain S, Rasheed K, Ali A, Vrinceanu N, Ahmed A, Shah Z. A sensitivity analysis of mhd nanofluid flow across an exponentially stretched surface with non-uniform heat flux by response surface methodology. Scientific Rep (2022) 12(1):18523. doi:10.1038/s41598-022-22970-y
54. Abid N, Ramzan M, Chung JD, Kadry S, Chu Y-M. Comparative analysis of magnetized partially ionized copper, copper oxide–water and kerosene oil nanofluid flow with cattaneo–christov heat flux. Scientific Rep (2020) 10(1):19300. doi:10.1038/s41598-020-74865-5
55. Acar Boyacioglu M, Kara Y, Kaan Baykan Ö. Predicting bank financial failures using neural networks, support vector machines and multivariate statistical methods: a comparative analysis in the sample of savings deposit insurance fund (sdif) transferred banks in Turkey. Expert Syst Appl (2009) 36(2):3355–66. doi:10.1016/j.eswa.2008.01.003
56. Hamid R, Plaksina T. Application of artificial intelligence techniques in the petroleum industry: a review. Artif Intelligence Rev (2019) 52(4):2295–318. doi:10.1007/s10462-018-9612-8
57. Wang D, He H, Liu D. Intelligent optimal control with critic learning for a nonlinear overhead crane system. IEEE Trans Ind Inform (2017) 14(7):2932–40. doi:10.1109/tii.2017.2771256
58. Wang J, Ali Shah N, Almutairi B, Kwon OK, Chung JD. Bvp4c approach and duality of hybrid nanofluid over extending and contracting sheet with chemical reaction and cross-diffusion effects. Results Phys (2024) 57:107362. doi:10.1016/j.rinp.2024.107362
59. Ullah A, Yao H, Ikramullah NAO, El-Sayed MS. A neuro-computational study of viscous dissipation and nonlinear arrhenius chemical kinetics during the hypodicarbonous acid-based hybrid nanofluid flow past a riga plate. ZAMM-Journal Appl Mathematics Mechanics/Zeitschrift für Angew Mathematik Mechanik:e202400208. doi:10.1002/zamm.202400208
Nomenclature
0 Reference condition
Keywords: artificial neural network, convection, ethylene glycol, heat transfer, magnetic field, nanofluid, nonlinear problems, thermal energy
Citation: Ullah A, Yao H, Waseem , Saboor A, Awwad FA and Ismail EAA (2024) A qualitative analysis of the artificial neural network model and numerical solution for the nanofluid flow through an exponentially stretched surface. Front. Phys. 12:1408933. doi: 10.3389/fphy.2024.1408933
Received: 29 March 2024; Accepted: 12 August 2024;
Published: 17 September 2024.
Edited by:
Francisco Vega Reyes, University of Extremadura, SpainReviewed by:
Zeeshan Asghar, Prince Sultan University, Saudi ArabiaAndaç Batur Çolak, Istanbul Commerce University, Türkiye
Copyright © 2024 Ullah, Yao, Waseem, Saboor, Awwad and Ismail. This is an open-access article distributed under the terms of the Creative Commons Attribution License (CC BY). The use, distribution or reproduction in other forums is permitted, provided the original author(s) and the copyright owner(s) are credited and that the original publication in this journal is cited, in accordance with accepted academic practice. No use, distribution or reproduction is permitted which does not comply with these terms.
*Correspondence: Asad Ullah, asad@ujs.edu.cn; Hongxing Yao, hxyao@ujs.edu.cn