- 1School of Finance and Economics, Jiangsu University, Zhenjiang, Jiangsu, China
- 2Department of Mathematical Sciences, University of Lakki Marwat, Lakki Marwat, Pakistan
- 3Department of Statistics and Business Analytics, United Arab Emirates University, Al-Ain, United Arab Emirates
- 4Department of Quantitative Analysis, College of Business Administration, King Saud University, Riyadh, Saudi Arabia
- 5Department of Mathematics, Faculty of Education, Ain Shams University, Heliopolis, Cairo, Egypt
This work aims to analyze the impacts of the magnetic field, activation of energy, thermal radiation, thermophoresis, and Brownian effects on the hybrid nanofluid (HNF) (Ag++silicon oil) flow past a porous spinning disk. The pressure loss due to porosity is constituted by the Darcy–Forchheimer relation. The modified Buongiorno model is considered for simulating the flow field into a mathematical form. The modeled problem is further simplified with the new group of dimensionless variables and further transformed into a first-order system of equations. The reduced system is further analyzed with the Levenberg–Marquardt algorithm using a trained artificial neural network (ANN) with a tolerance, step size of 0.001, and 1,000 epochs. The state variables under the impacts of the pertinent parameters are assessed with graphs and tables. It has been observed that when the magnetic parameter increases, the velocity gradient of mono and hybrid nanofluids (NFs) decreases. As the input of the Darcy–Forchheimer parameter increases, the velocity profiles decrease. The result shows that as the thermophoresis parameter increases, temperature and concentration increase as well. When the activation energy parameter increases, the concentration profile becomes higher. For a deep insight into the analysis of the problem, a statistical approach for data fitting in the form of regression lines and error histograms for NF and HNF is presented. The regression lines show that
1 Introduction
A new generation of hybrid nanofluids (HNFs) has been created as a result of emerging technologies [1]. Unlike nanofluids (NFs), which only contain one metal nanoparticle, HNFs contain many metallic nanoparticles. A simple NF is created when water is added to
Rotating machinery is a vital component of numerous industries. The rotating disk has many industrial applications, and therefore, its analysis is very important. An increasing variety of industries, including the aviation, automotive, and marine sectors, are using these rotating objects in various parts [23, 24]. The use of irregularly thickened disks is growing, mostly due to financial limitations and the requirement to improve mechanical properties. Rotating disks are necessary for the operation of many pieces of industrial equipment. As a result, researchers working on this subject have recently launched a number of initiatives. Shah et al. [25] investigated the Hall current for the 3D NF flow extending surface by considering the Cattaneo–Christov (C.C.) heat flux model. For example, the analysis of NF flow resulting from rotating disks with different thicknesses and relatively uniform responses was done by Hayat et al. [26]. In a different work, Hayat et al. [27] investigated the flow between two stretchable rotating disks in a porous medium using the C.C. heat flux simulation. The analysis of heat and mass for the 3D NF flow past an elastic sheet is investigated by Khan et al. [28]. Qayyum et al. [29] studied the entropy and dissipation of the MHD Williamson fluid between two rotating disks. Jyothi et al.’s investigation deals with the effect that magnetic fields and heat radiation have on CNT convection in NF within rotating elastic disks [30]. Pourmehran et al. [31] used the Patel model and Brownian motion to study heat exchange and NF flow between two rotating disks. A more recent work on rotating surfaces can be found in [32–35].
The applications of artificial intelligence (AI) cannot be denied. AI covered all areas of research, including medical, engineering, and technology [36–40]. The engineering applications of AI are briefly reviewed by Nti et al. [41]. Jang et al. studied the AI applications for the recognition of pathways and enzymes in metabolic engineering. Sofos et al. [42] reviewed the applications of AI in the field of fluid mechanics. Kartik et al. [43] analyzed the inviscid flow field by using AI. Amini and Mohaghegh [44] used AI by analyzing the fluid flow in a porous medium. The squeezing model with the help of AI is analyzed by Almalki et al. [45]. The irreversibility impact of considering the carbon nanotubes during the viscous fluid flow is analyzed by Zubair et al. [46]. They used a supervised learning-based AI approach to analyze the fluid flow. The MHD HNF flow during the rotating frame for heat and mass transfer is investigated by Shoaib et al. [47]. They used the numerical result as a reference solution for the neural network and analyzed the problem for various impacts of the pertinent parameters and related statistical analysis. The thermal slip and absorption impacts on the HNF flow during the rotating disk are investigated by Shoaib et al. [48]. They used AI for computational purposes. Ali et al. [49] used the Levenberg–Marquardt backpropagation search path for training the neural network for the analysis of the water-based CNT HNF fluid past an unstable spinning disk.
The magnetization of conducting fluids is the subject of research in the field known as magnetohydrodynamics (MHD). MHD engagement detects the interaction of ferromagnetic or fluid metal particles in the presence of an electromagnetic field and current. The MHD model connects the electrohydrodynamic Maxwell equations and fluid computations with the Lorentz force as a result of magnetism. The processes that generate Lorentz force and capacitive electric charge generally seem to be completely opposite to one another. Due to a decrease in concentration, there is an increase in temperature, growing velocity, and Joule heating as the joule number increases. Babu and Sandeep [50] studied the 3D slip effects for the MHD NF flow past an unstable sheet. Ghadikolaei et al. [51] studied the MHD stagnant-point flow of a
A comparative analysis of the HNF and NF is carried out by implementing the neural network. A graphical abstract is presented in Figure 1.
2 Problem formulation
Assume a steady HNF flow past a spinning disk that is axially symmetric along the
Considering the Buongiorno model with modifications, we have the following basic equations for the fluid flow [54, 55]:
with the B.Cs [56].
Here,
Applying Eq. 8 to Eqs 1–6, we obtain [55]:
Here,
In addition, the tangential
or
The local Nusselt number
where
The HNF models and physical properties of the NFs used in this study are displayed in Tables 1,2.
3 Proposed methodology
Artificial neural networks (ANNs) are computer models that simulate the human brain structure. The human system, from a neurological point of view, is very complex. ANNs are made up of nodes, which are interconnected to form layers with varying degrees of processing depth. Nodes are connected processing components. These combine to create intricate processing circuits that identify input patterns and generate responses. By training layers that correlate responses with incoming data with specific pattern knowledge, the patterns can be learned. The network gains knowledge through practice, and when linked to other networks, it gains knowledge through information sharing. The only layers in an ANN’s structure are the input layer, a hidden layer that is introduced first, and the output layer. Applications of neural networks are being used across numerous industries to address issues with security, the economy, and other factors. In the data-intensive era, neural networks have created new opportunities for study and application [60, 61]. The more recent trends in other disciplines of AI can be found in [61–63]. For a solution of Eqs 9–13, we first transform the given system into a first-order system. For this, we have [55]
The B.Cs are as follows [55]:
The two important steps of ANN are shown in Figure 3. During the process, after providing the input, the weights are trained for
The results for the state variables can be obtained by introducing the sigmoid function
3.1 Training of the weights
This section explains how the neural network is trained to find the output. The system given in Eq. 19, 20 is solved with the bvp4c, which is a well-known MATLAB built-in code for solving boundary value problems. The infinity is set at
and
4 Results and discussions
The impact of the silicon oil-based HNF (
The effect of various parameters on the radial and axial velocities is described in Figure 4A–H. The larger values of the velocity slip parameter
The absolute errors (AEs) to minimize the
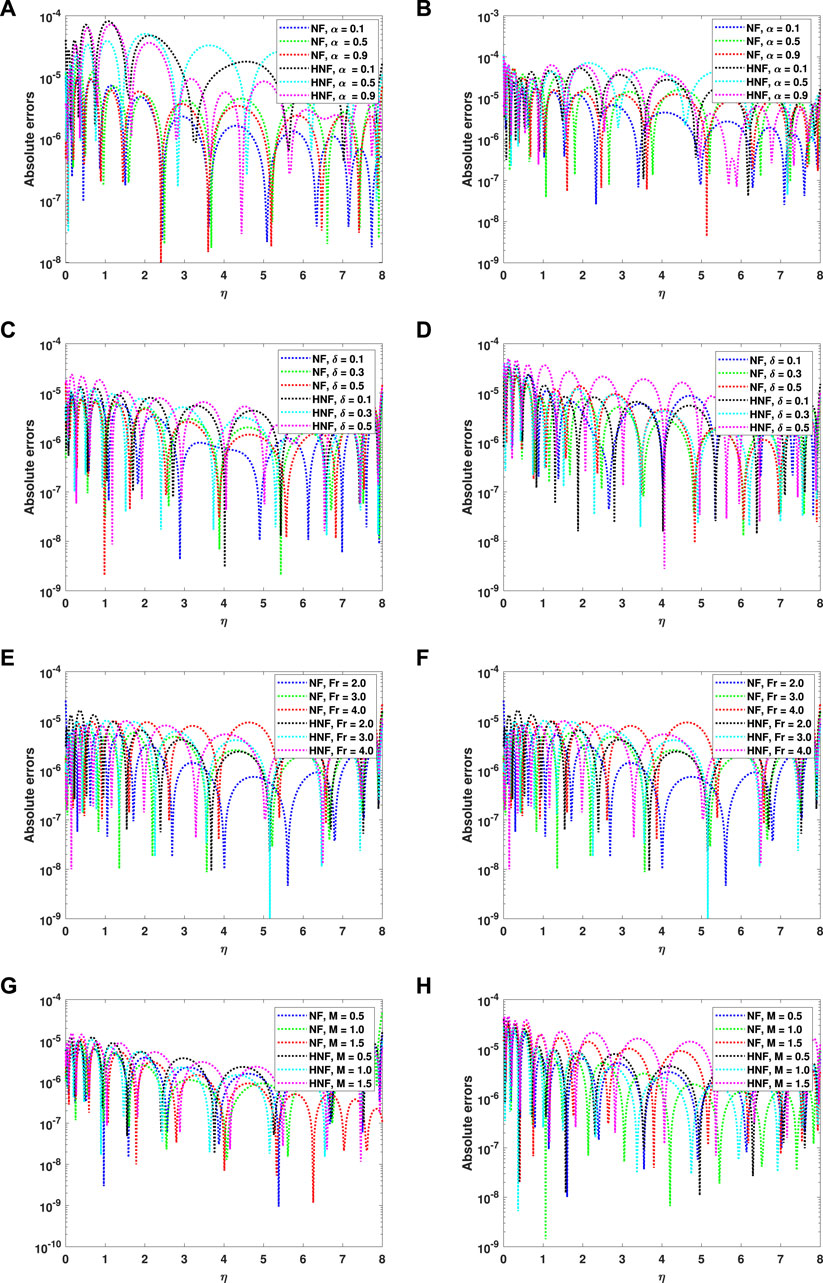
Figure 5. Absolute error for the impact of (A)
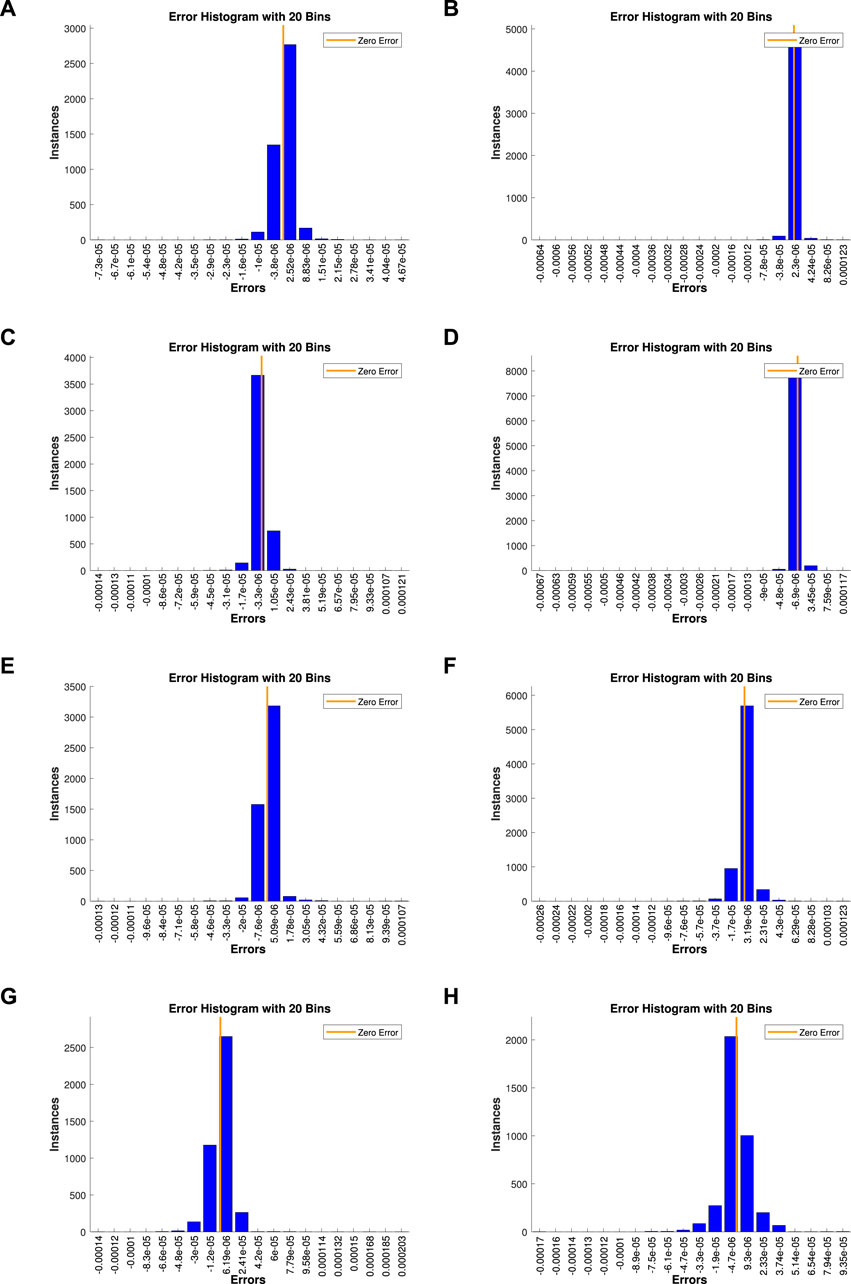
Figure 6. Error histogram for the impact of (A)
The effect of
The validations for the total performance of each parameter are presented in Figure 8A–D. These figures demonstrate the mean square error (ms) vs the total iterations performed. Four different curves are used for the trained, validated, tested, and best data. The performance for
The impact of
The corresponding mean square error for the varying values of
The error histograms for all these parameters
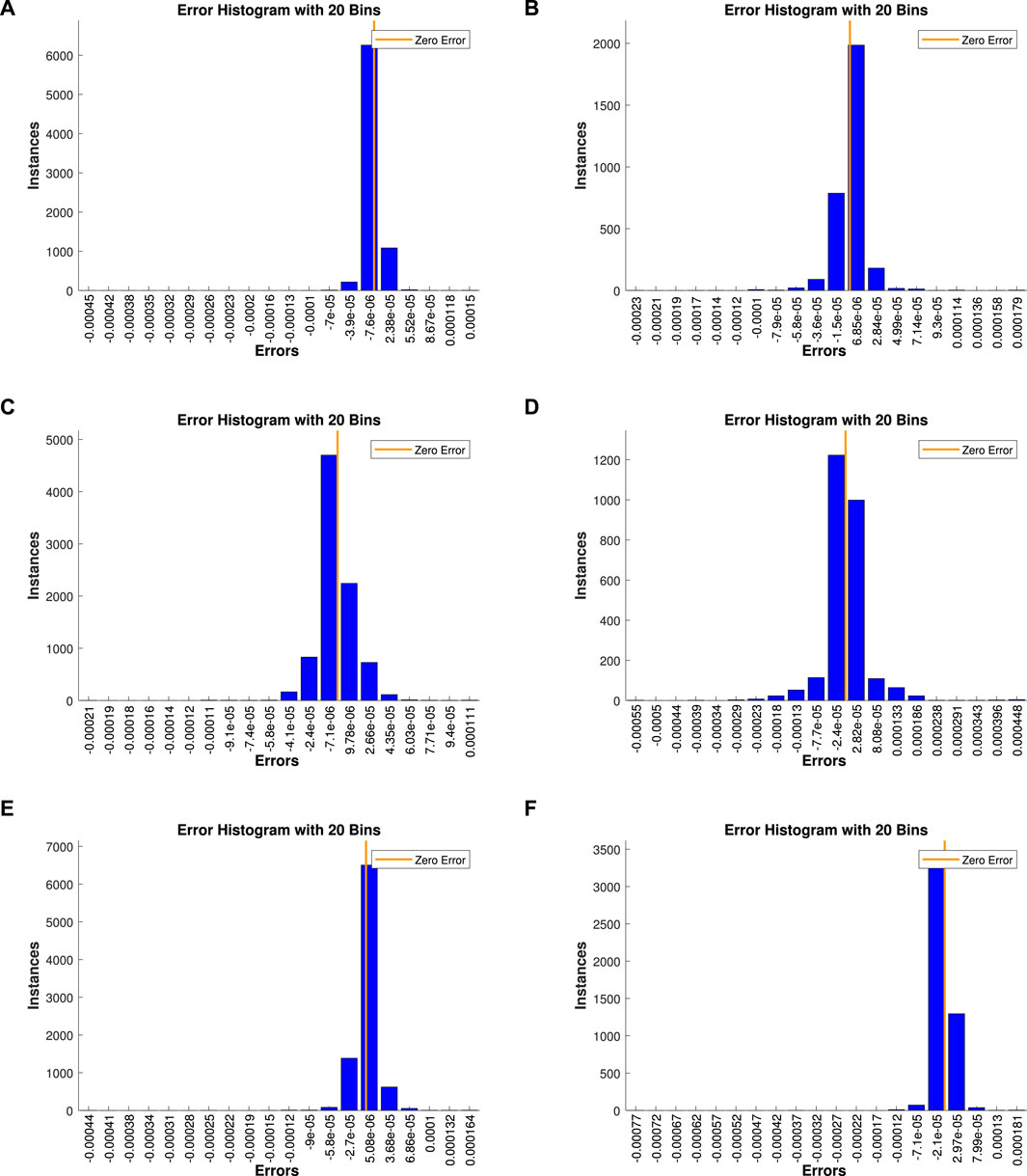
Figure 11. Error histogram for the effect of (A)
The regression lines for the impact of the parameters
The validation performance for the impacts of
4.1 Validation of results
The current approach is validated by considering the numerical values of Nusselt number and skin friction for
5 Conclusions
This article covers the neural network applications of the silicon-oil based HNF flow past a spinning disk using the Buongiorno model modifications. The impacts of various pertinent parameters for the thermal, concentration, and the velocity profiles are briefly described. We conclude the following:
Data availability statement
The original contributions presented in the study are included in the article/Supplementary Material, further inquiries can be directed to the corresponding authors.
Author contributions
AU: writing–original draft, validation, software, resources, methodology, investigation, formal analysis, and conceptualization. HY: writing–original draft, visualization, supervision, software, resources, project administration, methodology, and formal analysis. FU: writing–original draft, validation, software, methodology, investigation, and data curation. HA: writing–original draft, validation, software, resources, methodology, investigation, and formal analysis. EI: writing–original draft, visualization, validation, software, methodology, investigation, funding acquisition, and formal analysis. FA: writing–original draft, visualization, software, project administration, methodology, investigation, funding acquisition, and data curation. AS: writing–original draft, visualization, software, formal analysis, and data curation.
Funding
The author(s) declare that financial support was received for the research, authorship, and/or publication of this article.
Acknowledgments
Researchers Supporting Project number (RSPD2024R1060), King Saud University, Riyadh, Saudi Arabia.
Conflict of interest
The authors declare that research was conducted in the absence of any commercial or financial relationships that could be construed as a potential conflict of interest.
Publisher’s note
All claims expressed in this article are solely those of the authors and do not necessarily represent those of their affiliated organizations, or those of the publisher, the editors, and the reviewers. Any product that may be evaluated in this article, or claim that may be made by its manufacturer, is not guaranteed or endorsed by the publisher.
References
1. Kumar Devendiran D, Amirtham VA. A review on preparation, characterization, properties and applications of nanofluids. Renew Sust Energ Rev (2016) 60:21–40. doi:10.1016/j.rser.2016.01.055
2. Choi SUS, Eastman JA. Enhancing thermal conductivity of fluids with nanoparticles. Technical report. Argonne, IL (United States): Argonne National Lab.ANL (1995).
3. Nadeem S, Abbas N, Khan AU. Characteristics of three dimensional stagnation point flow of hybrid nanofluid past a circular cylinder. Results Phys (2018) 8:829–35. doi:10.1016/j.rinp.2018.01.024
4. Suresh S, Venkitaraj KP, Selvakumar P, Chandrasekar M. Effect of al2o3–cu/water hybrid nanofluid in heat transfer. Exp Therm Fluid Sci (2012) 38:54–60. doi:10.1016/j.expthermflusci.2011.11.007
5. Subba Reddy Gorla R, Siddiqa S, Mansour MA, Rashad AM, Salah T. Heat source/sink effects on a hybrid nanofluid-filled porous cavity. J Thermophys Heat Transfer (2017) 31(4):847–57. doi:10.2514/1.t5085
6. Tayebi T, Ali JC. Free convection enhancement in an annulus between horizontal confocal elliptical cylinders using hybrid nanofluids. Numer Heat Transfer, A: Appl (2016) 70(10):1141–56. doi:10.1080/10407782.2016.1230423
7. Acharya N. On the flow patterns and thermal control of radiative natural convective hybrid nanofluid flow inside a square enclosure having various shaped multiple heated obstacles. The Eur Phys J Plus (2021) 136(8):889. doi:10.1140/epjp/s13360-021-01892-0
8. Tayebi T, Ali JC. Natural convection enhancement in an eccentric horizontal cylindrical annulus using hybrid nanofluids. Numer Heat Transfer, Part A: Appl (2017) 71(11):1159–73. doi:10.1080/10407782.2017.1337990
9. Ali JC, Dogonchi AS, Ganji DD. Magneto-hydrodynamic flow and heat transfer of a hybrid nanofluid in a rotating system among two surfaces in the presence of thermal radiation and joule heating. AIP Adv (2019) 9(2). doi:10.1063/1.5086247
10. Asghar Z, Shatanawi W, Ali Shah R, Gondal MA. Impact of viscoelastic ooze slime on complex wavy gliders near a solid boundary. Chin J Phys (2023) 81:26–36. doi:10.1016/j.cjph.2022.10.013
11. Asghar Z, Ali Shah R, Ali Pasha A, Rahman MM, Khan MWS. Controlling kinetics of self-propelled rod-like swimmers near multi sinusoidal substrate. Comput Biol Med (2022) 151:106250. doi:10.1016/j.compbiomed.2022.106250
12. Asghar Z, Shatanawi W, Hussain S. Biomechanics of bacterial gliding motion with oldroyd-4 constant slime. Eur Phys J Spec Top (2023) 232(6):915–25. doi:10.1140/epjs/s11734-022-00723-2
13. Asghar Z, Ahmed E, Shatanawi W, Gondal MA. An exact solution for directional cell movement over jeffrey slime layer with surface roughness effects. Phys Fluids (2023) 35(4). doi:10.1063/5.0143053
14. Asghar Z, Ali Shah R, Shatanawi W, Ali N. Fene-p fluid flow generated by self-propelling bacteria with slip effects. Comput Biol Med (2023) 153:106386. doi:10.1016/j.compbiomed.2022.106386
15. Asghar Z, Shah RA, Shatanawi W, Ali N. A theoretical approach to mathematical modeling of sperm swimming in viscoelastic ellis fluid in a passive canal. Archive Appl Mech (2023) 93(4):1525–34. doi:10.1007/s00419-022-02343-7
16. Asghar Z, Khan MWS, Shatanawi W, Gondal MA, Ghaffari A. An ifdm analysis of low Reynolds number flow generated in a complex wavy curved passage formed by artificial beating cilia. Int J Mod Phys B (2023) 37(19):2350187. doi:10.1142/s0217979223501874
17. Acharya N. Framing the impacts of highly oscillating magnetic field on the ferrofluid flow over a spinning disk considering nanoparticle diameter and solid–liquid interfacial layer. J Heat Transfer (2020) 142(10):102503. doi:10.1115/1.4047503
18. Yarmand H, Zulkifli NWBM, Gharehkhani S, Shirazi SFS, Alrashed AAAA, Ali MAB, et al. Convective heat transfer enhancement with graphene nanoplatelet/platinum hybrid nanofluid. Int Commun Heat Mass Transfer (2017) 88:120–5. doi:10.1016/j.icheatmasstransfer.2017.08.010
19. Mesgari Abbasi S, Rashidi A, Nemati A, Arzani K. The effect of functionalisation method on the stability and the thermal conductivity of nanofluid hybrids of carbon nanotubes/gamma alumina. Ceramics Int (2013) 39(4):3885–91. doi:10.1016/j.ceramint.2012.10.232
20. Usman Sajid M, Ali HM. Thermal conductivity of hybrid nanofluids: a critical review. Int J Heat Mass Transfer (2018) 126:211–34. doi:10.1016/j.ijheatmasstransfer.2018.05.021
21. Kada B, Ali Pasha A, Asghar Z, Khan MWS, Bin Aris I, Shaikh MS. Carreau–yasuda fluid flow generated via metachronal waves of cilia in a micro-channel. Phys Fluids (2023) 35(1). doi:10.1063/5.0134777
22. Asghar Z, Ali Shah R, Ali N. A numerical framework for modeling the dynamics of micro-organism movement on carreau-yasuda layer. Soft Comput (2023) 27(13):8525–39. doi:10.1007/s00500-023-08236-3
23. Hayat T, Khan MI, Qayyum S, Khan MI, Alsaedi A. Entropy generation for flow of sisko fluid due to rotating disk. J Mol Liquids (2018) 264:375–85. doi:10.1016/j.molliq.2018.05.022
24. Sajid M, Sadiq MN, Ali N, Javed T. Numerical simulation for homann flow of a micropolar fluid on a spiraling disk. Eur J Mechanics-B/Fluids (2018) 72:320–7. doi:10.1016/j.euromechflu.2018.06.008
25. Sha Z, Dawar A, Alzahrani EO, Kumam P, Khan AJ, Saeed I. Hall effect on couple stress 3d nanofluid flow over an exponentially stretched surface with cattaneo christov heat flux model. IEEE Access (2019) 7:64844–55. doi:10.1109/access.2019.2916162
26. Hayat T, Rashid M, Imtiaz M, Ahmed A. Nanofluid flow due to rotating disk with variable thickness and homogeneous-heterogeneous reactions. Int J Heat Mass Transfer (2017) 113:96–105. doi:10.1016/j.ijheatmasstransfer.2017.05.018
27. Hayat T, Qayyum S, Imtiaz M, Ahmed A. Flow between two stretchable rotating disks with cattaneo-christov heat flux model. Results Phys (2017) 7:126–33. doi:10.1016/j.rinp.2016.12.007
28. Khan AS, Nie Y, Shah Z, Dawar A, Khan W, Islam S. Three-dimensional nanofluid flow with heat and mass transfer analysis over a linear stretching surface with convective boundary conditions. Appl Sci (2018) 8(11):2244. doi:10.3390/app8112244
29. Qayyum S, Khan MI, Hayat T, Alsaedi A, Tamoor M. Entropy generation in dissipative flow of williamson fluid between two rotating disks. Int J Heat Mass Transfer (2018) 127:933–42. doi:10.1016/j.ijheatmasstransfer.2018.08.034
30. Jyothi K, Reddy PS, Suryanarayana Reddy M. Influence of magnetic field and thermal radiation on convective flow of swcnts-water and mwcnts-water nanofluid between rotating stretchable disks with convective boundary conditions. Powder Tech (2018) 331:326–37. doi:10.1016/j.powtec.2018.03.020
31. Pourmehran O, Sarafraz MM, Rahimi-Gorji M, Ganji DD. Rheological behaviour of various metal-based nano-fluids between rotating discs: a new insight. J Taiwan Inst Chem Eng (2018) 88:37–48. doi:10.1016/j.jtice.2018.04.004
32. Jalili P, Ahmadi Azar A, Jalili B, Ganji DD. A novel technique for solving unsteady three-dimensional brownian motion of a thin film nanofluid flow over a rotating surface. Scientific Rep (2023) 13(1):13241. doi:10.1038/s41598-023-40410-3
33. Allehiany FM, Bilal M, Alfwzan WF, Ali A, Eldin SM. Numerical solution for the electrically conducting hybrid nanofluid flow between two parallel rotating surfaces subject to thermal radiation. AIP Adv (2023) 13(7). doi:10.1063/5.0154720
34. Yasir M, Khan M, Alqahtani AS, Malik MY. Mass transpiration effect on rotating flow of radiative hybrid nanofluid due to shrinking surface with irregular heat source/sink. Case Stud Therm Eng (2023) 44:102870. doi:10.1016/j.csite.2023.102870
35. Weerasiri L, Krishna Reddy Duvvuru S, Das S. Numerical simulation of a thin liquid film over a heated rotating disc and cone. Int J Therm Sci (2024) 196:108730. doi:10.1016/j.ijthermalsci.2023.108730
36. Strong AI. Applications of artificial intelligence and associated technologies. Science (2016) 5(6).
37. Shukla AK, Janmaijaya M, Abraham A, Muhuri PK. Engineering applications of artificial intelligence: a bibliometric analysis of 30 years (1988–2018). Eng Appl Artif Intelligence (2019) 85:517–32. doi:10.1016/j.engappai.2019.06.010
38. Shahzad H, Sadiq MN, Li Z, Algarni S, Alqahtani T, Irshad K. Scientific computing of radiative heat transfer with thermal slip effects near stagnation point by artificial neural network. Case Stud Therm Eng (2024) 54:104024. doi:10.1016/j.csite.2024.104024
39. Chen Y, De Luca G. Technologies supporting artificial intelligence and robotics application development. J Artif Intelligence Tech (2021) 1(1):1–8. doi:10.37965/jait.2020.0065
40. Ghalambaz S, Abbaszadeh M, Sadrehaghighi I, Younis O, Ghalambaz M, Ghalambaz M. A forty years scientometric investigation of artificial intelligence for fluid-flow and heat-transfer (aifh) during 1982 and 2022. Eng Appl Artif Intelligence (2024) 127:107334. doi:10.1016/j.engappai.2023.107334
41. Kofi Nti I, Felix Adekoya A, Weyori BA, Nyarko-Boateng O. Applications of artificial intelligence in engineering and manufacturing: a systematic review. J Intell Manufacturing (2022) 33(6):1581–601. doi:10.1007/s10845-021-01771-6
42. Sofos F, Stavrogiannis C, Exarchou-Kouveli KK, Akabua D, George C, Karakasidis TE. Current trends in fluid research in the era of artificial intelligence: a review. Fluids (2022) 7(3):116. doi:10.3390/fluids7030116
43. Karthik PC, Sasikumar J, Baskar M, Poovammal E, Kalyanasundaram P. Field equations for incompressible non-viscous fluids using artificial intelligence. The J Supercomputing (2022) 78(1):852–67. doi:10.1007/s11227-021-03917-y
44. Amini S, Mohaghegh S. Application of machine learning and artificial intelligence in proxy modeling for fluid flow in porous media. Fluids (2019) 4(3):126. doi:10.3390/fluids4030126
45. Mabrook Almalki M, Salem Alaidarous E, Adnan Maturi D, Raja MAZ, Shoaib M. Intelligent computing technique based supervised learning for squeezing flow model. Scientific Rep (2021) 11(1):19597. doi:10.1038/s41598-021-99108-z
46. Zubair G, Shoaib M, Khan MI, Naz I, Ali A, Raja MAZ, et al. Intelligent supervised learning for viscous fluid submerged in water based carbon nanotubes with irreversibility concept. Int Commun Heat Mass Transfer (2022) 130:105790. doi:10.1016/j.icheatmasstransfer.2021.105790
47. Shoaib M, Raja MAZ, Rehman Khan MA, Tauseef Sabir M, Sooppy Nisar K, Iltaf I, et al. Investigation of heat and mass transfer for an mhd hybrid nanofluid flow over a rotating disk: a numerical investigation with supervised neural network. Waves in Random and Complex Media (2022) 1–24. doi:10.1080/17455030.2022.2128228
48. Shoaib M, Raja MAZ, Touseef Sabir M, Sooppy Nisar K, Jamshed W, Felemban BF, et al. Mhd hybrid nanofluid flow due to rotating disk with heat absorption and thermal slip effects: an application of intelligent computing. Coatings (2021) 11(12):1554. doi:10.3390/coatings11121554
49. Ali F, Awais M, Ali A, Vrinceanu N, Shah Z, Tirth V. Intelligent computing with levenberg–marquardt artificial neural network for carbon nanotubes-water between stretchable rotating disks. Scientific Rep (2023) 13(1):3901. doi:10.1038/s41598-023-30936-x
50. Jayachandra Babu M, Sandeep N. Three-dimensional mhd slip flow of nanofluids over a slendering stretching sheet with thermophoresis and brownian motion effects. Adv Powder Tech (2016) 27(5):2039–50. doi:10.1016/j.apt.2016.07.013
51. Ghadikolaei SS, Yassari M, Sadeghi H, Hosseinzadeh K, Ganji DD. Investigation on thermophysical properties of tio2–cu/h2o hybrid nanofluid transport dependent on shape factor in mhd stagnation point flow. Powder Technol (2017) 322:428–38. doi:10.1016/j.powtec.2017.09.006
52. Ghadikolaei SS, Hosseinzadeh K, Yassari M, Sadeghi H, Ganji DD. Boundary layer analysis of micropolar dusty fluid with tio2 nanoparticles in a porous medium under the effect of magnetic field and thermal radiation over a stretching sheet. J Mol Liquids (2017) 244:374–89. doi:10.1016/j.molliq.2017.08.111
53. Palwasha Z, Saeed Khan N, Shah Z, Islam S, Bonyah E. Study of two-dimensional boundary layer thin film fluid flow with variable thermo-physical properties in three dimensions space. AIP Adv (2018) 8(10). doi:10.1063/1.5053808
54. Kumar M, Mondal PK. Irreversibility analysis of hybrid nanofluid flow over a rotating disk: effect of thermal radiation and magnetic field. Colloids Surf A: Physicochemical Eng Aspects (2022) 635:128077. doi:10.1016/j.colsurfa.2021.128077
55. Fawad Malik M, Ali Shah SA, Bilal M, Mohamed H, Mahmood I, Ali A, et al. New insights into the dynamics of heat and mass transfer in a hybrid (ag-tio2) nanofluid using modified buongiorno model: a case of a rotating disk. Results Phys (2023) 53:106906. doi:10.1016/j.rinp.2023.106906
56. Miklavčič M, Wang CY. The flow due to a rough rotating disk. Z für Angew Mathematik Physik ZAMP (2004) 55:235–46. doi:10.1007/s00033-003-2096-6
57. Awan AU, Ali B, Shah SAA, Oreijah M, Guedri K, Eldin SM. Numerical analysis of heat transfer in ellis hybrid nanofluid flow subject to a stretching cylinder. Case Stud Therm Eng (2023) 49:103222. doi:10.1016/j.csite.2023.103222
58. Bhatti MM, Öztop HF, Ellahi R, Sarris IE, Doranehgard MH. Insight into the investigation of diamond (c) and silica (sio2) nanoparticles suspended in water-based hybrid nanofluid with application in solar collector. J Mol Liquids (2022) 357:119134. doi:10.1016/j.molliq.2022.119134
59. Zhang J, Cui H, Han S, Li Z, Lu J. Research on the rheological properties of a silicone oil-based ferrofluid. Korea-Australia Rheology J (2023) 35(3):179–90. doi:10.1007/s13367-023-00065-y
60. Acar Boyacioglu M, Kara Y, Kaan Baykan Ö. Predicting bank financial failures using neural networks, support vector machines and multivariate statistical methods: a comparative analysis in the sample of savings deposit insurance fund (sdif) transferred banks in Turkey. Expert Syst Appl (2009) 36(2):3355–66. doi:10.1016/j.eswa.2008.01.003
61. Hamid R, Plaksina T. Application of artificial intelligence techniques in the petroleum industry: a review. Artif Intelligence Rev (2019) 52(4):2295–318.
63. Wang D, He H, Liu D. Intelligent optimal control with critic learning for a nonlinear overhead crane system. IEEE Trans Ind Inform (2017) 14(7):2932–40. doi:10.1109/tii.2017.2771256
64. Raza J, Raza M, Mustaq T, Qureshi MI. Supervised machine learning techniques for optimization of heat transfer rate of cu-h2o nanofluid flow over a radial porous fin. Multidiscipline Model Mater Structures (2023) 19(4):680–706. doi:10.1108/mmms-08-2022-0153
65. Tizakast Y, Kaddiri M, Lamsaadi M, Makayssi T. Machine learning based algorithms for modeling natural convection fluid flow and heat and mass transfer in rectangular cavities filled with non-Newtonian fluids. Eng Appl Artif Intelligence (2023) 119:105750. doi:10.1016/j.engappai.2022.105750
66. Bhattacharya A, Majumdar P. Artificial intelligence-machine learning algorithms for the simulation of combustion thermal analysis. Heat Transfer Eng (2024) 45(2):176–93. doi:10.1080/01457632.2023.2178282
67. Aylı E, Kocak E. Supervised learning method for prediction of heat transfer characteristics of nanofluids. J Mech Sci Tech (2023) 37(5):2687–97. doi:10.1007/s12206-023-0442-5
68. Hemmat M, Toghraie D, Amoozad F. Prediction of viscosity of mwcnt-al2o3 (20: 80)/sae40 nano-lubricant using multi-layer artificial neural network (mlp-ann) modeling. Eng Appl Artif Intelligence (2023) 121:105948. doi:10.1016/j.engappai.2023.105948
69. Yin C, Zheng L, Zhang C, Zhang X. Flow and heat transfer of nanofluids over a rotating disk with uniform stretching rate in the radial direction. Propulsion Power Res (2017) 6(1):25–30. doi:10.1016/j.jppr.2017.01.004
Keywords: hybrid nanofluid, thermal radiation, Buongiorno model, thermal transport, artificial intelligence, nanoparticles, soft computing
Citation: Ullah A, Yao H, Ullah F, Alqahtani H, Ismail EAA, Awwad FA and Shaaban AA (2024) Insight into the thermal transport by considering the modified Buongiorno model during the silicon oil-based hybrid nanofluid flow: probed by artificial intelligence. Front. Phys. 12:1372675. doi: 10.3389/fphy.2024.1372675
Received: 18 January 2024; Accepted: 12 June 2024;
Published: 23 July 2024.
Edited by:
Ali Mohebbi, Shahid Bahonar University of Kerman, IranReviewed by:
Hasan Shahzad, Dongguan University of Technology, ChinaAli Zabihi, Rowan University, United States
Copyright © 2024 Ullah, Yao, Ullah, Alqahtani, Ismail, Awwad and Shaaban. This is an open-access article distributed under the terms of the Creative Commons Attribution License (CC BY). The use, distribution or reproduction in other forums is permitted, provided the original author(s) and the copyright owner(s) are credited and that the original publication in this journal is cited, in accordance with accepted academic practice. No use, distribution or reproduction is permitted which does not comply with these terms.
*Correspondence: Asad Ullah, YXNhZEB1anMuZWR1LmNu; Hongxing Yao, aHh5YW9AdWpzLmVkdS5jbg==