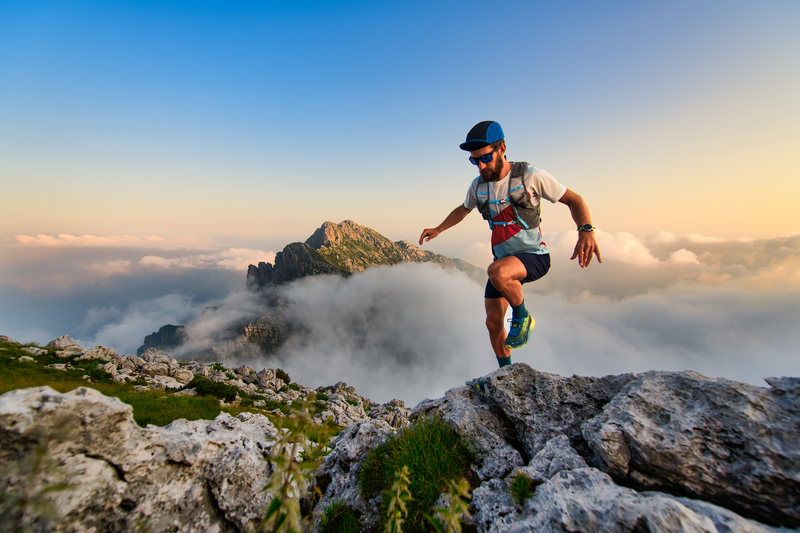
95% of researchers rate our articles as excellent or good
Learn more about the work of our research integrity team to safeguard the quality of each article we publish.
Find out more
ORIGINAL RESEARCH article
Front. Phys. , 18 July 2024
Sec. Social Physics
Volume 12 - 2024 | https://doi.org/10.3389/fphy.2024.1371233
This article is part of the Research Topic Real-World Applications of Game Theory and Optimization View all 17 articles
Introduction: The rise of autonomous vehicles has brought about a transformative shift in transportation, witnessing the coexistence of human-driven and autonomous vehicles on highways in the United States, Europe, and China. This coexistence poses challenges to traffic operations, particularly in intricate scenarios like highway ramps. The interaction between autonomous truck platoons, displaying heightened maneuverability, and human-driven vehicles has emerged as a critical concern. Consequently, this research aims to propose and investigate three avoidance modes (overall, gap and cross) employed by truck platoons, evaluating their comprehensive impact on human-driven vehicles.
Methods: Multiple scenarios are simulated utilizing the Simulation of Urban Mobility (SUMO) software, collecting data on three distinctive avoidance modes concerning Travel Time (TT) and Time to Collision (TTC). Employing principles of game theory, a comprehensive assessment is undertaken to evaluate the traffic efficiency and safety of each mode. Comparative analyses against a no-avoidance baseline are conducted, offering a holistic evaluation of each mode’s applicability across diverse scenarios.
Results: The findings highlight the commendable performance of gap mode and overall mode in enhancing traffic efficiency, while cross mode excels in fortifying traffic safety. Overall, the gap mode emerges as the optimal choice among the three.
Discussion: This study introduces a game-theoretic approach to managing human-machine mixed traffic flow, establishing a foundational framework for theoretical research in decision-making for emerging mixed traffic environments. It considers safety and efficiency perspectives across different types of traffic entities. The insights gained contribute to the evolving discourse on the integration of autonomous vehicles into existing traffic systems, addressing the intricate challenges posed by the coexistence of various vehicle types on highways.
With the development of logistics industry and the intensification of market competition, truck transportation plays a crucial role in cargo transportation. However, truck transportation also faces many challenges, such as traffic congestion, environmental pollution, and energy consumption. To address these issues, researchers have begun to consider using autonomous truck platoons to reduce transportation costs, improve transportation efficiency, and reduce environmental impacts. In practical scenarios, an excessively long truck platoon needs to avoid other cars in specific areas, so how to choose an avoidance mode is currently one of the research topics. Game theory-based methods can provide effective decision support for autonomous truck platoon system, which helps to choose the best avoidance mode.
The emergence of autonomous driving technology has given rise to various new technology, equipment, and theoretical approaches related to autonomous driving. Based on such development, truck platoon as a new freight transportation mode has demonstrated significant advantages in terms of reducing energy consumption [1, 2], operating costs [3, 4], and improving road capacity [5, 6]. Truck platoons are two or more trucks in a fleet that are connected using vehicle-to-vehicle communication and autonomous driving support systems. When these trucks are connected to each other on certain sections (e.g., on a highway), they automatically maintain a close-headway platoon [7]. This means that these trucks must be equipped with Level 1 or Level 2 and even higher autonomous driving systems [8]. However, the current state of autonomous driving technology is insufficient to support full truck platoon driving in the entire road network. Instead, truck platoon is applicable only in specific road scenarios. Moreover, due to the spatial characteristics of truck platoons, they are more suitable in the wider road scenarios [9]. Thus, it is crucial to explore the safety aspects and assess the impact on the traffic environment when implementing truck platoons in specific scenarios.
Previous research has investigated various aspects of truck platooning in specific road environments. Zhao focused on optimizing overall traffic efficiency by examining the appropriate length of truck platoons in off-ramp regions [10]. Chandra explored how different highway routes affect the accessibility of truck platoons [11]. Faber studied the impact of different truck platoon characteristics on traffic safety and efficiency in the context of highway merging scenarios [12]. It can be seen that the highways are recognized as suitable environments for truck platoon driving, particularly during merging and diverging scenarios where human-driven vehicles (HVs) frequently interact with truck platoons. Scholars have conducted studies on truck platooning during highway merging situations [13–15]. However, fewer studies have been conducted for the diversion scenario. Especially, how can truck platoons meet the needs of HVs on the adjacent lanes waiting for getting off the highway when passing through the highway diversion area. Tabibi suggested that high traffic volumes make it challenging for the vehicles to find gaps to cross the truck platoon [16].
Recent research has focused on the avoidance strategies of the connected autonomous truck platoons. For example, Wu applied the intra-platoon field theory in the feasible gap model [17]. Shi proposed a cooperative connected autonomous vehicle (CAV) lane-changing model to build more CAV platoon in mixed traffic conditions [18]. Wang designed a collaborative lane change control algorithm based on model predictive control algorithm, which could reduce the influence of lane change behavior on traffic flow [19].
The above studies showed that CAV platoon could improve the lane capacity and decrease the average time headway in a great deal [20, 21]. However, in a mixed traffic flow scenario (HV + CAV), the benefits of platooning can be influenced by various factors, including traffic signals, highway ramps, vehicle types, and human errors. Few of these factors were considered or discussed in past studies. Therefore, further studies are needed to explore these issues.
This study focuses on the highway off-ramp scenario to analyze the impact of truck platoon with different avoidance modes on the traffic flow when surrounded with human-driven vehicles. The objective of this study is to provide an effective method to assess the impact of different truck platoon avoidance modes on traffic flow, which helps to improve traffic efficiency and safety. It is assumed that all the vehicles in the simulation experiment are in the state of vehicle networking. The lane-changing information of human-driven vehicles can be obtained directly by the truck platoons. Besides, the truck platoons travel on the right lane that is compliant with the traffic rules in China. Simulation experiments based on SUMO were conducted in this study. Meanwhile, collision risk factor and traffic efficiency are also quantified to evaluate the applicability of each avoidance mode.
The rest of the study is organized as follows. Section 2 analyzes the lane-changing scenarios of HVs near the off-ramp areas and proposes three truck platoon avoidance modes. Section 3 describes the design of the simulation experiments. Section 4 proposes to evaluate and analyze the performance of each avoidance mode. Section 5 discusses the impact mechanisms of different avoidance modes. Section 6 summarizes the whole work and its contributions and limitations.
The technical difficulty and complexity of the truck platoon model is higher than that of the car platoon model. The flexibility of car platoons is stronger, and the control strategies can be applied on all lanes. However, truck platoons can only operate on designated lanes and have lower traffic rights than cars or car platoons on special road sections. Therefore, the avoidance of truck platoons is one of its characteristics, on the other hand its driving mode is also constrained. Therefore, in this study, a two-lane highway off-ramp scenario was designed, with the assumption that the truck platoon travels on the second lane (the right one). As the truck platoon approached the off-ramp, evasive maneuvers became necessary to satisfy the lane change requirements of HVs, as shown in Figure 1.
Figure 1. The lane-changing scenarios of human-driven vehicles (A) Continuous human-driven lane-changing vehicles (B) Discrete human-driven lane-changing vehicles.
Based on the above scenarios, three avoidance modes of the truck platoon are proposed as follows.
(1) Overall mode: All trucks in the platoon will change lanes to allow the green HVs to access the off-ramp. Once the HVs have entered the ramp, the platoon will transition back to the right lane.
(2) Gap mode: The platoon will disperse to create additional space for the green HVs to perform their lane changes. After the HVs have completed the off-ramp maneuver, the sub-platoons will merge to form the original long platoon configuration.
(3) Cross mode: In this mode, the platoon will dissolve into three sub-platoons. The lead platoon continues straight ahead. The center platoon will change lanes for the green HVs. The rear platoon will continue straight ahead and keep a safe distance from the last green HVs.
In the overall mode, the whole truck platoon will change lanes and return to the original lane after the HV vehicles goes off ramp. In the gap mode, the truck platoon stays on the same lane, then the platoon will split into two sub-platoons to provide additional space for off-ramp vehicles, and will re-merge after the HV vehicles go off ramp. In the cross mode, the truck platoon will split into three sub-platoons, then the middle sub-platoon changes the lane to give way to the HV vehicles, and will return to the original lane after the HV vehicles go off ramp.
The illustration of the three modes is shown in Table 1.
In this study, a highway simulation scenario was constructed based on SUMO. This highway included two lanes and an off-ramp area, as shown in Figure 2.
In our study, 40 drivers participated in simulation experiments in Silab simulator. The lane-changing position of each driver was recorded to obtain the lane change area. The lane-changing positions of HVs on the upstream of highway off-ramps were recorded. Based on this, this study determined the HVs’ lane-changing area for HVs upstream of the highway off-ramps as shown in Figure 3.
The speed limit of all vehicles was 120 km/h. The HVs entered the simulation lane randomly. The off-ramp vehicles must complete the lane-changing behavior before entering the no lane-changing area. In the continuous HVs scene, the four continuous off-ramp vehicles kept driving with a safe time of headway like other HVs until they reach the lane-changing area. Then they could change lane freely.
The trucks could keep driving by platoon and disband to yield by the TraCI module (Traffic Control Interface) in SUMO. Through the TraCI module, parameters such as the platoon status and vehicle trajectory in the platoon could be obtained in real time. At the same time, the vehicle status could be modified and controlled in the simulation scenario. The key parameters mentioned above are shown in Table 2.
In this study, the traffic flow volume of HVs around the truck platoon was regarded as an important factor in the mode choice [22]. Therefore, five ratio levels (K) of the off-ramp vehicles were simulated in SUMO, including K = 10%, K = 20%, K = 30%, K = 40%, K = 50%. K can be calculated as Eq. 1.
where, ORVs is the number of off-ramp vehicles in the scenario. GVs is the number of going-straight vehicles in the scenario.
Each mode was simulated in the three kinds of traffic flow volume, as shown in Table 3. The three kinds of traffic flow volume is based on the service level 1 to 4 in “Technical Standard of Highway Engineering (JTG B01-2014)” of China.
In this study, the truck platoon was assumed to be equipped with V2V communication and automatic cruise control (ACC) system. Therefore, different following models should be adopted for the truck platoon and HVs.
IDM, as a basic model to study the dynamic changes of traffic, is mainly modeled based on speed, inter-vehicle distance and the speed difference between the rear vehicle and the front vehicle [23, 24]. Therefore, this study uses IDM as the car-following model for human-driven vehicles in the simulation scenarios.
The acceleration function in IDM is shown as Eqs 2, 3
where,
The CACC car-following model based previous studies is used to describe the driving behavior between trucks [25–27]. Corresponding equations are listed in Eqs 4–6.
where,
In the SUMO, the vehicle needs to determine whether the gap on the target lane satisfies the minimum safe gap before executing the lane-changing command [28]. If it is satisfied, the lane-changing behavior would be executed, otherwise it needs to wait for a safer gap before changing lanes.
The safe gap of vehicles is calculated as follows.
where,
In the simulation experiments, the average value of the travel time (AVG TT) and the minimum value of time to collision (minTTC) of all HVs were recorded and calculated by Eqs 11, 12. AVG TT shows the overall travel efficiency of all vehicle in the simulation scenarios. MinTTC shows the worst safety situation influenced by the avoidance modes. Therefore, the best avoidance mode needs to decrease the negative impact on efficiency and increase the safety of the whole off-ramp area.
where,
The relative value (RV) of TT and minTTC between the avoidance mode and no-avoidance mode were calculated by Eqs 13, 14.
where,
In Figure 4, it can be observed that the increase of the traffic flow volume generally leads to a noticeable upward trend in the average travel time (AVG TT) of HVs across various ORVs ratio scenarios. To illustrate the impact of three avoidance modes on GVs, ORVs and overall HVs, the detailed analysis is presented as follows.
As shown in Figure 4, among the 15 scenarios (5 × 3 = 15, five ratio levels and three traffic flow volume), the gap mode performed best in 13 scenarios and the overall mode performed best in two scenarios in the discrete flow scenario. In these scenarios, these two modes made the HVs’ travel time shorter than the no-avoidance mode (keeping going-straight without any avoidance behavior). As shown in Figure 5, the gap mode performed best in 13 scenarios in the continuous flow scenario. Therefore, the gap mode is applicable in most scenarios. The overall mode is only suitable for a few scenarios. The cross mode did not help to decrease the GVs’ travel time, but increased it in almost all scenarios.
As shown in Figure 5, the gap mode performed best in 13 scenarios. It was found that the gap mode was still benefit for the majority of the ORVs in the discrete flow scenario. The overall mode had negative influence on the ORVs’ travel time (the minimum travel time of ORVs changed from 2s to 4s). However, in the continuous flow scenario, no-avoidance showed good performance in 14 scenarios. This means that the avoidance behavior of truck platoon is not useful to reduce the ORVs’ travel time.
The AVG TT represented the overall impact of different modes on all nearby HVs.
As shown in Figure 4, the gap mode performed well in 12 scenarios and the overall mode performs well in three scenarios in the discrete flow scenario. As for the continuous flow scenario, the gap mode performed well in 13 scenarios and the overall mode performs well for the HV in the three scenarios.
The above results show that in the discrete flow scenario, adopting a certain avoidance mode (overall mode or gap mode) is useful to improve the traffic efficiency near the off-ramp. The gap mode obviously showed the best performance among the three modes to reduce the HVs’ AVG TT in the continuous scenario.
In the discrete flow, when the traffic flow was between 1800–2,300 pcu/h, the overall mode and gap mode had different performance in decreasing AVG TT of HVs with the increase of K. However, in the medium flow (2,400–2,900 pcu/h) or high flow (3,000–3,500 pcu/h), only the gap mode could decrease the AVG TT of HVs. Therefore, the gap mode was more suitable for medium and high traffic flow volume scenarios. It can be concluded that the cross mode had a poor performance in reducing the AVG TT of HVs. On the contrary, the gap mode was the best choice in improving the driving efficiency in the mixed traffic flow near the off-ramp.
In this study, the relative value of AVG TT is used to measure the driving efficiency. The small AVG TT value can result in more efficient avoidance mode. The relative value of minTTC is used to measure the driving safety. The large minTTC value means a safer avoidance mode. Therefore, a perfect avoidance mode can reach the relative maximum value (maxZ) of two aspects. The calculation model is Eq. 15.
where, E is the maximum relative value of average travel time in GVs and ORVs, S is the minimum relative value of minTTC in lane-changing area, q is the rate of flow volume, which includes low, medium and high, K is the ratio levels of the off-ramp HVs, which includes 10%, 20%, 30%, 40% and 50%,
To get the solution of the above equation, the Euclidean Distance based single-objective linear programming method is applied to calculate the maximum Z-value. The mode with large Z-value is safer and more efficient in the specific scenario. When Z > 0, the avoidance mode is more superior than no-avoidance mode in terms of safety and efficiency. Otherwise, when Z < 0, the performance of the avoidance mode is not as good as no-avoidance mode. When Z = 0, it means that the avoidance mode and no-avoidance have the same effect, so it is not necessary to take avoidance behavior for the ORVs.
Based on this, the Z-value of each avoidance mode in the discrete flow and continuous flow scenarios are obtained and shown in Figure 6 and Figure 7.
The above two figures show that gap mode (the grey line) had the highest Z-value among the 15 discrete flow and 15 continuous flow scenarios. The gap mode was suitable for 70% off-ramp scenarios. The overall mode was suitable for 23.3% off-ramp scenarios, and the cross mode was suitable for only 6.7% off-ramp scenarios.
In the cases of Z < 0, three avoidance mode had different limitations among the 30 situations.
• Cross mode
The Z-value of this mode were below 0 in nearly half of the 30 scenarios. It performed better under the high ORVs ratio in discrete traffic flow. In the continuous flow, it was the best choice under the 20% ORVs ratio in the medium traffic flow. However, in the rest circumstances, its performance was not stable and was inferior to the other two modes.
• Overall mode
The overall mode performed badly in the high discrete traffic low under low ORVs ratio (K = 10%, 20%, 30%) and the medium discrete traffic low under high ORVs ratio (K = 30%, 40%, 50%). In the continuous flow, this mode is not an option for the medium traffic flow under low ORVs ratio (K = 10% and K = 30%) and the low traffic flow under high ORVs ratio (K = 50%).
• Gap mode
It had no benefit for the low traffic flow under low ORVs ratio (K = 10% and K = 20%) and the medium/high traffic flow under high ORVs ratio (K = 50%/K = 40%).
Therefore, the gap mode was the best choice for the truck platoon to improve the safety and efficiency for the mixed traffic flow in the most scenarios. The other two modes were preferred in some specific scenarios. Table 4 and Table 5 show the game analysis results of all scenarios.
In low traffic volume and low-level ORVs ratio, the overall mode is optimal for discrete off-ramp vehicles. For other cases at low ORVs ratio, the gap mode and cross mode are better choice. In medium traffic flow, the cross mode and gap mode are optimal for continuous off-ramp vehicles. In high traffic volume, the overall mode is optimal if the ORV ratio is K = 50% or 40% but it does not perform well in medium and low traffic flow for continuous off-ramp vehicles. For other cases at high flow rate, the gap mode is optimal.
The simulation results show that in the two-lane highway off-ramp area, the AVG TT of HVs increases with the traffic flow volume under mixed traffic flow. This is because when the volume is low, HVs can drive freely at the ideal speed or designed speed. It is easy for them to slow down and change lanes near the ramp, which can result in small influence on the other vehicles or truck platoon. With the increase of traffic flow, the traffic density near the off-ramp will also increase. In this situation, once the ORVs decelerate, several GVs following the ORVs need to decelerate, which leads to a decrease in the average speed and travel time of HVs. This phenomenon indicates that the off-ramp demand and state are the basis to evaluate the need and performance of truck platoon avoidance mode. It can be seen that the above results have some similarity with the previous studies. For example, Shen [29] mentioned that in order to improve the traffic efficiency of urban expressways, V2V technology was needed near the expressway off-ramp and downstream intersections as collaborative control areas. This indicates that the status of the off-ramp is an important scenario when evaluating the demand and performance of the truck platoon avoidance modes. The traffic volume and off-ramp vehicle ratio were considered in past studies [20, 30], in which high traffic volume or ORV ratio would result in the platoon-single vehicle congestion, so short platoon modes were preferred. This is consistent with the results in Tables 4, 5 that gap mode is more suitable in high traffic volume and ORV ratio scenarios, and overall mode is the best mode in low traffic volume and ORV ratio scenarios. On the other hand, this research also has some difference with previous studies. Some studies focused on the operation characteristics and safety effects [31]. The lateral and longitudinal steady control is the key factors to guarantee the safety of truck platoons and other vehicles near the off-ramp. However, this study does not focus on the micro-aspect of single truck operation, but applies best avoidance mode to enhance the overall safety and efficiency of off-ramp area, which can be applied in most two-lane highway off-ramp scenarios. Different from Liu et al.’s study [20] and Wang et al.’s study [32], three avoidance modes were proposed and analysed in the off-ramp simulation experiments, other than the single sub-platoon control mode or merging scenarios. This provides a macro-decision strategy for platoon management system. According to the simulation and game analysis results, the main findings are discussed as follows.
The results show that there are obvious differences in efficiency gain among three avoidance modes. In some continuous flow scenarios, if the truck platoon takes no-avoidance action, the ORVs’ AVG TT of most scenarios are smaller than the three modes. The reason is that the continuous lane-changing demand requires a large gap in the truck platoon to ensure the safe off-ramp behavior. Therefore, when truck platoon slows down or changes lanes to yield for a high rate of continuous ORVs, the following ORVs and ORVs on the adjacent lane have to slow down as well, which results in the increase of ORVs’ AVG TT. To overcome this problem, the gap mode is a solution to provide ORVs with enough space to change lanes before the lane-changing area. It makes the truck platoon to split and leave enough space for ORVs to change lanes. This can not only reduce the waiting time for the ORVs, but also release the left lane for the GVs to drive freely. Therefore, this mode greatly reduces the traffic delay and improves the HVs and ORVs’ driving efficiency.
The poor performance of the cross mode is due to the low gap acceptance between the sub-platoon. HVs have to follow the sub-platoon for a few seconds to seek for a lane-changing opportunity. On the other hand, before the ORVs change lanes, they need to check the traffic state on the target lane. If there are already several ORVs in the “cross gap” (the gap between the first and the third sub-platoon), the following ORVs have to wait until the target lane has enough space. These two aspects are the main reasons for the large AVG TT result when the truck platoon taking the cross mode.
Considering the lane-changing characteristics of truck platoon, the overall mode yields for the ORVs by changing the whole platoon to the left lane. This requires no additional control of sub-platoon. However, the overall mode has some limitations in some situations. For example, when the HVs travel on the left lane near the off-ramp, the truck platoon cannot directly change lanes. The container trucks in truck platoon must change lanes one by one according to the nearby traffic conditions. Sometimes, the following trucks have to look for another appropriate lane-changing opportunity after the leading truck changes lanes. This will reduce the average speed of HVs on the left lane, which results in the decrease of the HVs’ driving efficiency.
When the ORVs reach the off-ramp area, they need enough space on the right lane to meet their lane-changing demand. In the no-avoidance state, the truck platoon occupies 100 m on the right lane, so that the ORVs have two choices to get off the highway: a) overtaking the whole truck platoon before reaching the end line of the lane-changing area. b) keeping a distance with the truck platoon, and waiting for the truck platoon passing the off-ramp exit, then finding the appropriate opportunity to change lanes. If the ORVs need to get off the ramp quickly, they must sacrifice the safety to decrease the relative distance and to increase the speed. Therefore, the most unsafe status usually appears in this period.
If the traffic flow volume increases constantly and reaches a high flow volume, the traffic density will increase. Therefore, when HVs are closer to the off-ramp and still do not change to the right lane, they will develop a strong intention to change lanes. If truck platoon disbands enough space, ORVs will adjust the speed between the two sub-platoons. Otherwise, there will be a risk of rear-end accident. In the simulation results, this phenomenon is reflected with low AVG min TTC of HVs.
Generally, the avoidance of truck platoon near the highway off-ramp can increase traffic safety. The gap mode provides great space for ORVs to meet their lane-changing requirement. However, the gap mode will cause the rear sub-platoon to decelerate, which will increase the conflict probability between the rear sub-platoon and the following HVs. In addition, if the leading platoon does not accelerate enough, the ORVs cannot overtake the platoon before the lane-changing area. In this case, they must wait on the left lane at the off-ramp exit, which results in traffic congestion (choice b). The overall mode allows the ORVs to change lanes freely by clearing the right lane, which reduces the conflicts between HVs and other vehicles. However, during the lane-changing process of each container truck, the conflict probability between truck platoon and HVs climbs. The HVs following or travelling in parallel with truck platoon must decelerate to the same speed as the platoon to change lane safely. Therefore, how to balance the truck lane-changing behavior and traffic efficiency is an important issue of this avoidance mode. The cross mode combines the advantages and disadvantages of the other two modes. It includes the lane change and disband behavior of platoons, so it provides proper gap for ORVs to change lanes. Compared with the other two modes, the minTTC of cross mode is larger. This is because the ORVs in this mode usually change lanes at a relative slower speed, which sacrifices the travel efficiency but improves safety.
A comprehensive evaluation of different avoidance modes in mixed traffic flow necessitates an examination from two key perspectives: travel efficiency and safety. The findings indicate that the gap mode consistently demonstrates superior performance across various scenarios, while the overall mode exhibits commendable efficiency in several instances. Conversely, the cross mode proves applicable in only a limited number of scenarios. Comparative analysis against a no-avoidance state reveals that all three modes contribute to enhancing the efficiency and safety of both human-driven vehicles (HVs) and truck platoons to a certain extent.
The results of game analysis highlight the gap mode as the optimal choice, displaying the most favourable impact on travel efficiency and safety, especially with increasing traffic volume and off-ramp vehicle ratio. Although not always the primary choice in specific situations, the gap mode remains a viable option, particularly for static truck platoon control systems. In cases where individual trucks within the platoon can be monitored and controlled, the avoidance mode should be dynamically tailored to match the traffic environment, as outlined in Table 4 and Table 5.
This study introduces three avoidance modes for truck platoons in the off-ramp area of a two-lane highway within a human-machine mixed traffic flow context. Employing game theory to analyze the safety and efficiency of each avoidance mode, the study concludes that the gap mode proves most effective in enhancing travel safety and efficiency. By segmenting the long platoon into multiple sub-platoons, the gap mode generates ample spaces for multiple off-ramp vehicles.
However, in scenarios characterized by high traffic volume and consecutive high-speed vehicles waiting to exit the highway, the overall mode emerges as a preferable option over the gap mode. In the overall mode, all trucks shift lanes to the left, significantly reducing travel time for nearby vehicles. Additionally, the overall mode demonstrates adaptability to various Off-Ramp Vehicle (ORV) ratio levels in low traffic volume, ensuring greater safety for high-speed human-driven vehicles compared to other avoidance modes.
Despite these findings, this study still has some limitations. Firstly, all vehicles in the scenarios are assumed to be connected in the Intelligent Transportation Systems environment, but information transmission delay in avoidance mode is not analyzed. Secondly, the study only focuses on the differences between the three modes without considering the position, speed, and length of sub-platoons for each mode. Thirdly, the evaluation indicators for HVs do not include other factors, such as queue length and deceleration rate. Consequently, future research needs to conduct field tests to investigate the safety, traffic efficiency, and response delay of diverse avoidance modes in the Vehicle-to-Everything (V2X) environment. Detailed micro-macro evaluation of different modes would be preferred to obtain more accurate avoidance strategies.
The raw data supporting the conclusion of this article will be made available by the authors, without undue reservation.
YL: Conceptualization, Methodology, Writing–original draft, Funding acquisition. LW: Writing–original draft, Writing–review and editing. ZX: Formal Analysis, Writing–original draft, Writing–review and editing. WS: Data curation, Software, Writing–original draft.
The author(s) declare that financial support was received for the research, authorship, and/or publication of this article. This research is sponsored by the National Natural Science Foundation of China, grant number 52202419.
The authors would like to thank the support of their colleagues and M.S. candidates in the Logistics Research Center, Shanghai Maritime University, China.
Author WS is employed by Lianyungang New Harbour Terminal Co., Ltd., Jiangsu, China.
The remaining authors declare that the research was conducted in the absence of any commercial or financial relationships that could be construed as a potential conflict of interest.
All claims expressed in this article are solely those of the authors and do not necessarily represent those of their affiliated organizations, or those of the publisher, the editors and the reviewers. Any product that may be evaluated in this article, or claim that may be made by its manufacturer, is not guaranteed or endorsed by the publisher.
1. Tsugawa S, Jeschke S, Shladovers SE. A review of truck platooning projects for energy savings. IEEE Trans Intell Vehicles (2016) 1:68–77. doi:10.1109/tiv.2016.2577499
2. Alam A, Besselink B, Turri V, Mårtensson J, Johansson KH. Heavy-duty vehicle platooning for sustainable freight transportation: a cooperative method to enhance safety and efficiency. IEEE Control Syst Mag (2015) 35(6):34–56. doi:10.1109/MCS.2015.2471046
3. Bösch PM, Becker F, Becker H, Axhausen KW. Cost-based analysis of autonomous mobility services. Transport Policy (2018) 64:76–91. doi:10.1016/j.tranpol.2017.09.005
4. Wadud Z, MacKenzie D, Leiby P. Help or hindrance? The travel, energy and carbon impacts of highly automated vehicles. Transportation Res A: Pol Pract (2016) 86:1–18. doi:10.1016/j.tra.2015.12.001
5. Motamedidehkordi N, Margreiter M, Benz T. Effects of connected highly automated vehicles on the propagation of congested patterns on freeways. In: Proceedings of the 95th Annual Meeting of the Transportation Research Board; Washington DC, United States (2016). p. 16–1802.
6. Ramezani H, Shladover SE, Lu XY, Altan OD. Micro-simulation of truck platooning with cooperative adaptive cruise control: model development and a case study. Transportation Res Rec (2018) 2672(19):55–65. doi:10.1177/0361198118793257
7. Bouchery Y, Hezarkhani B, Stauffer G. Coalition formation and cost sharing for truck platooning. Transportation Res B: Methodological (2022) 165:15–34. doi:10.1016/j.trb.2022.08.007
8. Calvert SC, Schakel WJ, van Arem B. Evaluation and modelling of the traffic flow effects of truck platooning. Transportation Res C: Emerging Tech (2019) 105:1–22. doi:10.1016/j.trc.2019.05.019
9. Ali A, Garcia G, Martinet P. The flatbed platoon towing model for safe and dense platooning on highways. IEEE Intell Transportation Syst Mag (2015) 7(1):58–68. doi:10.1109/mits.2014.2328670
10. Zhao C, Li L, Li J, Li K, Li Z. The impact of truck platoons on the traffic dynamics around off-ramp regions. IEEE Access (2021) 9:57010–9. doi:10.1109/access.2021.3072070
11. Chandra S, Thai T. Analyzing freight truck platoon accessibility with route deviations. Sustainability (2022) 14(4):2130–17. doi:10.3390/su14042130
12. Faber T, Sharma S, Snelder M, Klunder G, Tavasszy L, van Lint H. Evaluating traffic efficiency and safety by varying truck platoon characteristics in a critical traffic situation. Transportation Res Rec (2020) 2674(10):525–47. doi:10.1177/0361198120935443
13. Buisson C, Keyvan-Ekbatani M, Wagner P. Impede autonomous vehicles merging at on-ramps. In: Proceedings of the Transportation Research Board 97th Annual Meeting; Washington DC, United States (2018). p. 18–00938.
14. Duret A, Wang M, Leclercq L. Truck platooning strategy near merge: heuristic-based solution and optimality conditions. In: Proceedings of the Transportation Research Board 97th Annual Meeting; Washington DC, United States (2018). p. 18–00902.
15. Scarinci R, Heydecker B. Control concepts for facilitating motorway on-ramp merging using intelligent vehicles. Transport Rev (2014) 34(6):775–97. doi:10.1080/01441647.2014.983210
16. Tabibi M. Automated truck traffic: design and Control of automated truck traffic at motorway ramps (2014). Available from: http://resolver.tudelft.nl/uuid:d1009e78-be81-4140-8930-5028ffe455c0 (Accessed January 15, 2024).
17. Wu R, Li L, Lu W, Rui Y, Ran B. Improving the safe operation of platoon lane changing for connected automated vehicles: a novel field-driven approach. Appl Sci (2021) 11(16):7287–17. doi:10.3390/app11167287
18. Shi Y, He Q, Huang Z. Capacity analysis and cooperative lane changing for connected and automated vehicles: entropy-based assessment method. Transportation Res Rec (2019) 2673(8):485–98. doi:10.1177/0361198119843474
19. Wang D, Hu M, Wang Y, Wang J, Qin H, Bian Y. Model predictive control-based cooperative lane change strategy for improving traffic flow. Adv Mech Eng (2016) 8(2):168781401663299–17. doi:10.1177/1687814016632992
20. Liu Y, Wang X, Li L, Cheng S, Chen Z. A novel lane change decision-making model of autonomous vehicle based on support vector machine. IEEE Access (2019) 7:26543–50. doi:10.1109/access.2019.2900416
21. Jing S, Hui F, Zhao X, Rios-Torres J, Khattak AJ. Cooperative game approach to optimal merging sequence and on-ramp merging control of connected and automated vehicles. IEEE Trans Intell Transportation Syst (2019) 20(11):4234–44. doi:10.1109/tits.2019.2925871
22. Zhang C, Wang B, Yang S, Zhang M, Gong Q, Zhang H. The driving risk analysis and evaluation in rightward zone of expressway reconstruction and extension engineering. J Adv Transportation (2020) 2020:1–13. doi:10.1155/2020/8943463
23. Zhou M, Qu X, Jin S. On the impact of cooperative autonomous vehicles in improving freeway merging: a modified intelligent driver model-based approach. IEEE Trans Intell Transportation Syst (2017) 18(6):1–7. doi:10.1109/tits.2016.2606492
24. Saifuzzaman M, Zheng Z. Incorporating human-factors in car-following models: a review of recent developments and research needs. Transportation Res Part C: Emerging Tech (2014) 48:379–403. doi:10.1016/j.trc.2014.09.008
25. Milanés V, Shladover SE. Modeling cooperative and autonomous adaptive cruise control dynamic responses using experimental data. Transportation Res Part C: Emerging Tech (2014) 48:285–300. doi:10.1016/j.trc.2014.09.001
26. Xiao L, Wang M, Van Arem B. Realistic car-following models for microscopic simulation of adaptive and cooperative adaptive cruise control vehicles. Transportation Res Rec (2017) 2623:1–9. doi:10.3141/2623-01
27. Xiao L, Wang M, Schakel W, van Arem B. Unravelling effects of cooperative adaptive cruise control deactivation on traffic flow characteristics at merging bottlenecks. Transportation Res Part C: Emerging Tech (2018) 96:380–97. doi:10.1016/j.trc.2018.10.008
29. Shen H. Research on control strategies and methods of entrances and exits of urban expressway-Take Beijing Expressway as an example. Road Traffic Sci Tech (2018) 13–9.
30. Pang M, Huang J. Cooperative control of highway on-ramp with connected and automated vehicles as platoons based on improved variable time headway. J Transportation Eng A: Syst (2022) 148(7):04022034. doi:10.1061/jtepbs.0000683
31. Liu Z, Ma Q, Shao H, Fang R, Lin T. Study on the exit characteristics and safety impact of single lane exit ramp of expressway. Highway (2023) 333–42.
Keywords: truck platoon, avoidance mode, mixed traffic flow, off-ramp area, game theory
Citation: Li Y, Wang L, Xuan Z and Shen W (2024) Game-theory based truck platoon avoidance modes selection near the highway off-ramp in mixed traffic environment. Front. Phys. 12:1371233. doi: 10.3389/fphy.2024.1371233
Received: 16 January 2024; Accepted: 03 June 2024;
Published: 18 July 2024.
Edited by:
Dun Han, Jiangsu University, ChinaReviewed by:
Francisco Alonso, University of Valencia, SpainCopyright © 2024 Li, Wang, Xuan and Shen. This is an open-access article distributed under the terms of the Creative Commons Attribution License (CC BY). The use, distribution or reproduction in other forums is permitted, provided the original author(s) and the copyright owner(s) are credited and that the original publication in this journal is cited, in accordance with accepted academic practice. No use, distribution or reproduction is permitted which does not comply with these terms.
*Correspondence: Yi Li, bGl5aTIwNTU5OEBzaG10dS5lZHUuY24=
Disclaimer: All claims expressed in this article are solely those of the authors and do not necessarily represent those of their affiliated organizations, or those of the publisher, the editors and the reviewers. Any product that may be evaluated in this article or claim that may be made by its manufacturer is not guaranteed or endorsed by the publisher.
Research integrity at Frontiers
Learn more about the work of our research integrity team to safeguard the quality of each article we publish.