- School of Mechanical Engineering, Nanjing University of Science and Technology, Nanjing, China
The traditional theory of dynamic open-loop control of fuzes has been unable to satisfy the demand for smart fuze control. After analyzing time–space recognition and process control as the basis of fuze detonation control, three key issues of smart fuze control are summarized herein. Furthermore, three types of controllers are constructed, namely, detection, state, and detonation controllers, which achieve the precise detonation control of ammunition under the interference of complex multi-physics environments, ensuring efficient damage to the target. Based on the above-mentioned controllers, the design theory of the ternary cascade control of the smart fuze is established. In addition, the technical system of the ternary cascade control theory is reviewed, and the latest progress in control strategy design, environment modeling, target modeling, and other fields is summarized in detail. Finally, the development demands and technology trends in intelligent fuze control technology for future intelligent warfare are explored.
1 Introduction
A fuze is an important system responsible for controlling the initiation, opening, separation, and ignition of ammunition, and its technical level is the key factor in determining the precise damage inflicted by ammunition. It has been the core technology of key developments in various countries. Fuze theory and technology involve many disciplines and are the crystallization of multidisciplinary integration. Advanced fuzes refer to smart and intelligent fuzes; a smart fuze is the primary stage of the intelligent fuze, which is characterized by the crosslinking of combat information, environment and target detection, rapid information processing, and transient accurate control functions. The development of a smart fuze is divided into three categories: extension power, proximity explosion, and penetration. The fuze is the “brain” that controls the detonation of ammunition, according to a predetermined demand, detected environment, and targets. It can also be applied for the ignition, opening, and separation of ammunition. The fuze is used in the entire lifecycle of ammunition and is the core component to ensure the safety of weapons and ammunition before detonation and achieve efficient damage to a specific target after detonation at the first hit [1–4]. Fuze is one of the most important core subsystems in the field of weapons and equipment. It is a high-density integrated small opto-electromechanical system that integrates many functions such as sensing and detection, communication and interaction, information processing, control, and execution. It is required to be equipped with smart bullets, mines, intercontinental missiles, and nuclear bombs.
Currently, the development of fuzes takes the development connotation of advanced fuzes as the main line, the whole-life safety and transient accurate initiation control as the theoretical basis, and the technical difficulties such as miniaturization, serialization, and environmental utilization as the safety mechanisms that need to break through. Environment and target detection need to overcome technical difficulties such as the mathematical representation of databases [5], feature classification, and rapid extraction of multivariate available factors [6]. Cross-linking and devices need to overcome technical difficulties such as transient information transmission modes of platforms and weapon fuzes [7], adaptive demodulation and anti-jamming, and anti-jamming device design in energy flow and information flow transmission. Sensing and detection need to overcome technical difficulties such as multi-source sensor integration, multi-source detection information fusion, anti-interference, and miniaturization of detection systems [8]. It is necessary to overcome technical difficulties such as multi-type target attribute cognition and modeling, rapid target feature recognition, and precise detonation control with delay compensation [9]. Range and azimuth control need to overcome technical difficulties such as fast interlocking delay control, multi-source information transient acquisition of target orientation, and target weak spot identification. Tests and evaluations need to overcome the advanced fuze performance of laboratory verification platform design, comprehensive fuze performance of the shooting range verification method, performance evaluation of the mathematical model, and other technical difficulties [10]. The development of fuze is expected to reach a new level, paving the way for the development of intelligent fuzes.
In the future, fuze products will be an important part of the weapon systems, combining acoustic, optical, mechanical, electrical, and magnetic functionalities into one; fuze development mainly relies on new design theory support, and new ammunition development needs traction. At the same time, it is necessary to overcome key technological challenges, such as developing new functional materials [11], all kinds of new system sensors and processors, new micro-nano processing and micro-system integration processes [12], new explosion transmission and initiation agents, and new test methods, and systematically develop advanced fuze design theories, verification methods, and evaluation systems.
Some scholars have done some recent research to improve the control accuracy of fuzes in this respect [5–7, 13, 14] and designed a dual-wavelength frequency-modulated continuous-wave (FMCW) laser beam anti-aerosol interference target recognition and distance determination system. An adaptive fuze-warhead coordination method based on the backpropagation artificial neural network (BP-ANN) is proposed in [8, 15], which adaptively calculates the initiation delay using missile-target intersection parameters. The damage probability of different radial positions along the same gun line is calculated in order to determine the best initiation position [9–11, 16]. Based on time–frequency joint features, a naive Bayes classifier (NBC) with minimal risk is established for target and interference recognition to improve the adaptability of the proposed method in complex environments [16–18]. The improvement of some detectors (HPD) in improving the detection efficiency is studied, which will guide future fuze detection and identification of targets. These studies can effectively improve the accuracy of current fuze control.
Based on the modern warfare mode, the fuze is promoted to develop in the direction of information, dexterity, and intelligence. Its combat objects are transformed from single-domain and single-type targets to multi-domain and multi-type targets, and its flight speed is increased to ultra-high speed. As a result, the fuze faces serious non-linear effects such as coupling interference between various environmental noises and detection signals, state and interface transient changes under a high-speed intersection of ammunition/target, multi-field coupling oscillation, and delay drift during high-speed penetration. In a complex environment, multi-source information acquisition and decoupling, multi-type target attribute recognition, delay compensation, transient precision, and initiation control are important directions for smart fuzes.
The core technology to achieve precise attack and efficient damage is time–space recognition and process control technology, which means that the fuze should accurately decide the optimal detonation position in a three-dimensional (3D) space and output the detonation command at the required time, adapting to the different speeds and altitudes of the ammunition and targets. The zero-time point after launching, the 3D coordinates of flight space, velocity, flight altitude, and optimal damage radius are decided through a dynamic mathematical model and the constraints of targets and the environment.
Throughout the fuze industry, traditional fuzes have realized detonation control in one-dimensional (1D) and two-dimensional (2D) spaces. 1D control only outputs the detonation command at a pre-set time or the moment the ammunition contacts the target, resulting in poor control accuracy and low damage efficiency. 2D control has a significantly improved damage efficiency based on the dynamic open-loop control of the fuze. The fuze detects the projectile, target, and environment at a specific time or in a specific period and outputs the detonation command within a limited period. However, the damage efficiency of the ammunition has still not been maximized in 2D control. To adapt to a more complex modern combat environment, a 3D control that includes the target distance, azimuth, and detonation timing multidimensional space is urgently required. Moreover, the traditional dynamic open-loop control can hardly satisfy the requirements of 3D control in a more complex battlefield environment, and a new control system is urgently required [19].
The main contributions of this article are as follows:
(1) Aiming at the time–space recognition and process control of the smart fuze, some key factors with important effects on the control of fuze are discussed, and a ternary cascade controller for smart fuzes is proposed.
(2) A self-triggering time-delay compensation state controller, environment-adaptive detection controller, and virtual closed-loop detonation controller, which constitute the ternary cascade controller, are designed to realize efficient damage and 3D detonation control of smart fuzes.
(3) The technical system of ternary cascade control theory is summarized, and the development demand and technical trend of intelligent fuze control technology of future warfare are prospected.
2 Problem formulation
2.1 Essence of fuze control: time–space recognition and process control
2.1.1 Time–space recognition of the fuze
The fuze space–time recognition refers to the recognition of the time and space range control quantity of each specific stage after the fuze is launched with the ammunition. These stages are the bore movement stage, the air or water movement stage, and the stage before reaching the target [4].
The time variables at each stage include muzzle exit time, ballistic apex time, flight time, time at a certain distance from the target, and detonation time delay; the space variables in each stage include flight displacement, speed, angle, distance error, detonation distance, and error control amount.
Among the fuze time–space recognition information mentioned above, the sources of information can be divided into pre-set and detectable environmental and target information. The pre-set information is obtained by cross-linking the weapon system and fuze information, and the detectable environmental and target information is obtained using the fuze-dedicated sensor.
2.1.2 Process control of the fuze
Fuze process control means that the fuze completes the specified precise detonation control before reaching the target or after invading it [4].
The constrained variables of the process control are the zero-time point after launching, 3D coordinates of the flight space, speed and altitude, best damage radius, and detonation distance after invading the target.
To maximize the damage efficiency of the ammunition, different output control quantities can be selected based on the constraints of different target types, and they depend on the constraints of target environments such as space, air, near-ground, sea surface, underwater, and underground. For air targets, the range and azimuth of the detected target can be selected for real-time detonation; for ground-moving vehicles and active forces, a reasonable detonation height can be selected for output; and for people and facilities in underground hard targets, a reasonable detonation distance and time can be selected after penetrating the target.
Therefore, to maximize the damage efficiency of warheads, time–space recognition and process control are particularly important for the development of fuzes and should be the main directions for the development of fuze control theory.
2.2 Three key problems of smart fuze control
For the precise detonation control process of a fuze, the damage efficiency of ammunition to the target is divided into three zones: the zone with the greatest damage efficiency is the damage zone, the fuzzy zone is one in which slight damage is caused, and the safe zone is one in which the target cannot be damaged.
For traditional mechanical or electromechanical fuzes, only part of the ammunition can be detonated in the damage zone using point control (1D control), but most of the burst points occur in the fuzzy zone; the distance between the detonation position and the target is large, and the ammunition damage efficiency is low.
To improve the damage ability of ammunition, a surface control (2D control) fuze with 2D constraints of detonation distance and time was proposed. Most of the burst points occur on the edge of the damage or fuzzy zones. Compared with the traditional mechanical or electromechanical fuzes, the distribution range of burst points is close to the target, which improves the damage effect of the ammunition.
The future battlefield with a strong interference places higher requirements on the accurate detonation ability of ammunition. Reliance on the existing point or surface control cannot achieve efficient damage to the target.
Therefore, a volume control (3D control) smart fuze with 3D constraints of target distance, azimuth, and time is proposed herein to ensure that the ammunition detonates within the damage zone.
To establish the control model of smart fuzes, fuze time–space recognition and process control must solve the following three key problems: multi-physical field environment detection, diversified target recognition, and precise detonation control.
2.2.1 Coupling effect of strong interference on fuze multi-physical detection
The natural and enemy interference environments contain two types of interference: information and energy interference. The coupling of multi-physical field environments [20–23] distorts the detection signal of a fuze, which affects the accuracy of obtaining information of the actual environment. Therefore, the decoupling and environmental information should be rapidly recognized to ensure that the detection information is available.
Using the fuze of an electromagnetic gun as an example, during the launching process, the ammunition and fuze are subjected to a force impact of thousands of g lasting milliseconds; simultaneously, they are interfered with by a Tesla-level magnetic field. A temperature field will inevitably be generated under the action of the friction between the guide rail and armature exit, which is subject to high-temperature thermal shock during the launch process [24–27]. Therefore, the coupling interference between multi-physical fields affects the safety and reliability of the fuze.
To address the problem of environmental interference in multiple physical fields, an anti-interference control method for multi-source fusion detection is proposed. By establishing a multi-physics environment database, the characteristic value of the environment can be quickly extracted online.
The proportion of each detection source in the total detection information is adjusted dynamically by comparing the characteristic value with the value of the current environmental detection information.
Subsequently, effective and rapid decoupling of strong multi-physical field environmental interference can be realized.
2.2.2 Effect of time delay on the timeliness of a fuze control system
The open-loop control mode of the traditional fuze can only detect at a certain time or period, and the timings of detection and detonations have restrictions. If the time delay is not considered in the control model, the damage efficiency of ultra-high-speed ammunition will be reduced (for rendezvous speeds greater than Mach 5, if there is a time delay of 2–6 ms, the distance error reaches 3.4–10.2 m).
To realize precise detonation control, the effect of time delay on the fuze control system should be studied, and an effective time-delay estimation and compensation should be realized. By introducing the prior information set by the weapon platform and the detection information of a multi-source detection module transmitted by the surrounding weapon platform combined with dynamic weight depth fusion, high-precision estimation and compensation of time delay can be realized.
2.2.3 Optimal matching of the diversified targets and detonation controller
As shown in Figure 1, for different targets, the fuze must adopt different detonation methods to achieve the optimal detonation effect [28]. Therefore, the matching relationship between the target type and the optimal detonation method must be solved.
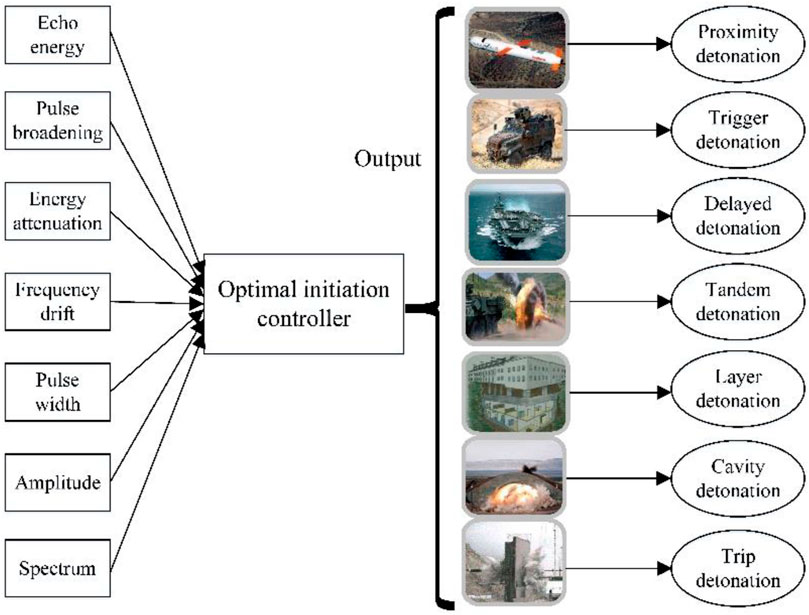
FIGURE 1. Schematic diagram of the target matching optimal detonation output. Adapted with permission from [Precision Detonation Control Problem of Smart Fuze in Complex Wide-Area Battlefield] by Zhang He, Yu Hang, Dai Ke Ren, Liu Peng, Yang Yu Xin, Ma Xiang], licensed under [Accession number: 20224613131862], [Acta Armamentarii, 2022 lancet (10) : 2527-2533. The DOI: 10.12382 / BGXB. 2021.0530.]
However, the detonation control process of the fuze is at the millisecond level; thus, the detonation control can only adopt an open-loop control structure. For the traditional fuze, numerous live ammunition damage experiments and exhaustive parameters were used to determine the best control strategy. In response to this challenge, a strategy combining offline virtual closed-loop training with online fast decision-making was proposed. In the offline state, the optimal detonation control parameters are obtained using a large amount of data training; in the online state, the optimal detonation control parameters are matched with the onboard data information using the setting information and the information transmitted by different platforms before and after the launch; subsequently, the optimal detonation mode is quickly generated for different targets.
3 Technical solutions: ternary cascade controller
To satisfy the requirements of smart fuzes to detonate warheads accurately and timely in a complex battlefield and to realize the maximum damage efficiency, the ternary cascade control theory of smart fuzes is established herein, and the schematic diagram is shown in Figure 2.
As Figure 2 shows, first, the ternary cascade controller is constructed based on the functions of environment and targets, which are the environmental adaptive detection controller, self-triggering time-delay compensation state controller, and virtual closed-loop detonation controller. The detection and state controllers adopt feedback control, and the detonation controller uses open-loop control with a virtual closed loop.
In the detection stage, the smart fuze uses a multi-source detector to collect information on the environment and target, transforming it into corresponding characteristic values through environment and target functions; the obtained characteristic values are input into the state and detonation controllers, and the current state quantity of the smart fuze is generated by the state controller; the fuze state quantity is input to the detonation controller as an important parameter of the detonation time and model of the smart fuze. Simultaneously, the state quantities are fed back to the detection controller to dynamically adjust the weight factor of each detection source and optimize the detection mode until the detonation controller outputs the detonation command.
In this ternary cascade control system of the smart fuze, to realize accurate environment and target recognition, the database of environment and target characteristics should be established through laboratory, range, fuze warhead cooperation, and simulation tests.
The environment database primarily contains the characteristic data collected from various types of conventional ammunition during launch and flight. This includes information on parameters such as the transmission overload, strong magnetic field, strong electric field, and temperature field during the transmission process, and the geomagnetic field, natural environment, and enemy interference environment during the flight. In the target database, the attacked targets are classified by features such as space, air, surface ship, underwater, near-ground, and underground targets.
With the support of the above-mentioned environment and target databases, the corresponding environment and target feature functions are formed through mathematical statistics. Therefore, after the ammunition is launched, the actual environment and target detected by the fuze output the corresponding environment and target characteristic values through the above-established characteristic function.
On one hand, the environmental characteristic values are input into the state controller. Combined, it dynamically adjusts the weight of the time delay value estimated by each detection source to minimize the error of time-delay estimation, and it generates the fuze state quantity to be input into the detection and detonation controllers.
On the other hand, according to the target characteristic values, the target type and best damage mode can be determined. The detonation controller determines the optimal detonation mode for the target, according to the fuze state quantity and target characteristic values, and it outputs the detonation command to complete the detonation.
3.1 Detection controller: fusion of multi-source information against environmental interference
The modern combat environment has become more complex, breaking the traditional boundaries of land, sea, air, and space and forming an integrated, wide-area battlefield. In such a battlefield, the randomness of the natural environment and complexity of the battlefield are superimposed on each other, which requires the fuze to realize a safe and reliable detonation control of ammunition in a more severe and extreme environment.
Therefore, a fusion control method for multi-source information against environmental interference is proposed, and an adaptive environment-detection controller is designed. The flow diagram of the method is shown in Figure 3.
First, by analyzing the typical interference environment experienced before and after the fuze is launched through many offline experimental tests, an environment multi-physics database is formed. With the support of the database, probability and statistics calculations are used to model and obtain the environment-recognition function, namely, the environmental reference model. Subsequently, the environmental characteristic values of the ith detection source at time t can be expressed as
The deviation in the characteristic value between the actual measured and standard values can be expressed as
where
From the deviation in the characteristic value (
where
When the obtained weighting factor is input, the detection controller forms feedback adjustment parameters and optimizes the method of each detection source. The controller expression is
where
After adjusting the detection signal, we obtain
The aim of the above methodology is to minimize the noise, i.e.,
3.2 State controller: time-delay estimation and compensation assisted by prior information
Owing to the extremely high relative flight speed of the ammunition and target, the rendezvous window is at the sub-millisecond level. Therefore, the time delay of the fuze control system has a significant effect on the accuracy of the final detonation.
The time delay in the fuze can be divided into the following parts: detection time, signal processing time, and actuator time delays.
To solve the detection time delay, an estimation and compensation control method assisted by prior information is proposed, and a self-triggered time-delay compensation state controller is constructed. The control flowchart is shown in Figure 4.
First, the input information source of the state controller is expanded, and the prior information set is introduced using the weapon platform and detection information on the multi-source detection module and transmitted by the surrounding weapon platform. By comparing the time delay estimated by the multiple detection module with the standard time delay input by the weapon platform, the time-delay estimation error (
If
Next, the obtained time-delay estimation error (
The time-delay estimation error can be expressed as
where
The state controller can be obtained as
where
where n is the number of detectors and
Since the operating frequency of the CPU is known, the signal processing time delay can be estimated according to the amount of data executed:
where k is the number of detonation mechanisms and
3.3 Detonation controller: target-adaptive matching using a virtual closed-loop design
Owing to the diversity of targets attacked by ammunition, to achieve efficient damage, the fuze must have the ability of fast target recognition and matching decision to generate the corresponding detonation output mode and then detonate the warhead in different modes to achieve efficient destruction. Considering that the target attributes are coupled and associated with multiple physical quantities and the computing resources of the onboard system hardware are relatively weak, it is impossible to achieve rapid target recognition and matching decision by relying on online realization. Therefore, the method of offline training and online use is proposed for the rapid decision of the detonation output mode. Subsequently, we designed a virtual closed-loop detonation controller. The flowchart of the virtual closed-loop detonation controller is shown in Figure 5.
At offline moments, the damage experiment is conducted according to the target subdivision type to form the output of the effective damage-detonation reference model (
In an actual combat mode, the multi-source detection information, target feature database, and information set by the weapon platform are used to obtain target subdivisions. The optimal detonation controller of the fuze determines the detonation output time and mode by combining the target subdivision and the current state quantity of the fuze.
According to the above analysis, the damage effect difference can be expressed as
where
Differentiating (Eq. 11) with respect to
The detonation controller can be obtained as
where
4 Specific technical system and practical application
The theory of ternary cascade control consists of three parts, and the following review will explore the content shown in Figure 6.
4.1 Multi-source fusion detection anti-environment interference control
4.1.1 Multi-physics environment modeling
To address the launching process of smart fuze ammunition, a multi-physical environment model must first be established to implement the multi-source fusion anti-environmental interference control method proposed in this paper. With the support of the multi-physical environment model and the fusion of multi-source environment anti-interference methods, the environment adaptive detection controller, which significantly increases the detection accuracy and reliability of the smart fuze, is proposed.
During the ammunition launching stage, a large impact and overload must be produced, which will have a significant effect on the structural reliability and stability of the fuze detection system. Therefore, the effect of launching on the performance of the fuze detection system must be studied.
In [29], the effect of a dynamic shock on the optical properties of a light transmission window of a laser measurement system was studied. The optical characteristics of the light guide window after a high overload were analyzed using a combination of dynamics and optical numerical simulation. The results indicated that as the impact acceleration increases, the central brightness of the exit spot through the transparent window decreases, the spot size increases, and the energy diverges. In [21], the influence mechanism of a conical shockwave on the forward detection of a pulsed laser was studied, and an analytical method was proposed to study this mechanism at arbitrary Mach numbers and cone angles. The analytical expression of the pulsed laser echo waveform under the effects of the shockwave was established as
where the specific parameter explanation is available in [21].
For the new launch environment of electromagnetic guns, the strong electromagnetic and electric fields generated by the launching platform will strongly interfere with the ammunition, affecting the reliability and safety of the projectile. In the process of electromagnetic propulsion, the extremely harsh multi-physical environment of the fuze is the key problem restricting its practical application. Therefore, the launch environment must be quantitatively analyzed to provide relevant data and support for the later design of fuzes for electromagnetic guns. In [24], motivated by the lack of research on the distribution characteristics of magnetic and electric fields in electromagnetic railgun chambers under dynamic conditions, a numerical calculation method was proposed. Based on the magnetic diffusion equation and Ampere’s law, using the measured dynamic experimental data and the magnetic flux density at the gun tail, the current density values in the armature and guide rail areas were determined using the finite element calculation. Additionally, the magnetic and electric field distribution characteristics of each inspection point in the front and rear axes of the armature were calculated.
To analyze the magnetic field distribution characteristics of the c-type armature electromagnetic railgun bore, [25] established a calculation model by considering the dynamic contact pressure. The movement characteristics of the armature in the hole were obtained, and the current distribution inside the rail and armature was analyzed. [30] expanded on [24]: the magnetic field distribution in the bore of the electromagnetic railgun under the actual contact condition of the armature and guide rail was studied. Using the modeling method, the magnetic field distribution characteristics in the bore of the railgun under the condition of armature movement were analyzed, and the study observed that the peak flux density decreases rapidly with the increase in the distance from the armature.
Furthermore, most of the previous modeling studies ignored or over-idealized the coupling effect between different physical fields, which results in a large deviation between the simulation and actual results. [26] established a multi-field coupling dynamic model of the electromagnetic propulsion process, where EM, MM, and TM represent electromagnetic, mechanical, and temperature fields, respectively;
However, an actual battlefield environment is very complicated, and the projectile will encounter various natural phenomena during the flight. Therefore, to analyze the laser detection performance of the natural environment such as fog, smoke, rain, and clouds that may be encountered during the flight of a laser smart fuze, [31] studied the characteristics of the fog backscattering signal of an FMCW laser fuze and established a Monte Carlo simulation model of FMCW laser transmission in the fog. In [32], the multiple scattering model of an FMCW laser fuze in a smoke environment and the precise distance determination algorithm were studied, and the multiple-scattering model of an FMCW laser fuze in a smoke environment was established. It can be expressed as
where the specific parameter explanation is available in [33].
[34] studied a laser fuze in flying environments, where it encounters non-spherical raindrops; the analytical model of the optical scattering of the infrared laser is
where the specific parameter explanation is available in [34], and the optical scattering characteristics of laser radiation when encountering 1.064-
Based on [32, 35], we studied the propagation characteristics of pulsed lasers in rain by establishing a Monte Carlo model of pulsed laser propagation in rain. Details of the specific model are available in [35]. In [33], the structure of the backscattered echo of the laser fuze cloud layer was studied. Using the low-visibility (less than 100 m) cloud as the research object based on the Mie theory and Monte Carlo method, the laser detection model in the cloud was established.
Most of the above-mentioned studies focused on environmental modeling or signal analysis, or they proposed methods to enhance fuze adaptability in an environment. According to previous test methods, many range tests were still required to verify the accuracy of the proposed anti-environmental interference program. In [36], based on the
4.1.2 Multi-source fusion anti-interference
To resist an enemy and natural interference on the safety and reliability of a proximity fuze, a proximity fuze with a single detection mode cannot reliably make effective analyses on the environment and target information. Therefore, a fuze with multi-mode compound detection was introduced to improve the anti-interference ability.
After the development of military technology, the types of proximity fuzes with multi-mode detection methods include radio and laser composite fuzes, millimeter-wave active and passive composite fuzes, radio and electrostatic composite fuzes, laser and electrostatic composite fuzes, radio and passive infrared composite fuzes, and active radio and passive infrared and electrostatic three-mode compound fuzes [37]. In [38], a combined infrared and radio fuze was proposed, which significantly improved the reliability and practicability of the fuze. To address the problem that a laser fuze is easily interfered with by clouds, tall trees, and leaves during flight, [39] proposed a laser fuze with a dual-wavelength detection mode, which uses the difference in the reflection signals of two different wavelengths to identify targets and resist interference. In [40], a target recognition algorithm for laser and infrared composite scanning fuze was proposed. By building a laser and infrared array scanning model, the algorithm extracted the features of the top stereo contour and top infrared radiation image of the target and fused the relevant information.
To solve the problem of laser detection technology against cloud, smoke, rain, and snow interference, [41] proposed a combined detection technology of frequency-modulated continuous-wave laser and radio.
Let the distances and measurement accuracies measured by the laser and radio be
Generally, the accuracy of laser ranging is higher than that of radio ranging, that is,
In [42], a more in-depth study on the technology of the frequency modulation continuous wave system laser and radio composite detection fuze was conducted; the calculation model of the backscattered signal of the laser fuze in the smoke environment was realized (19), and the prototype of the composite detection system fuze was developed.
Based on the composite fuze composed of infrared, laser, and millimeter-wave detectors, the Dempster–Shafer evidence theory was used for information fusion, and a neural network was combined to construct basic probability assignments [43]. The schematic diagram is shown in Figure 7.
For high-value ammunition such as missiles, missile-borne radar can be used for target detection and identification. Therefore, when the detection method of the laser–radar system is adopted, it can be easily polluted by noise, such as background light and electronic noise. To increase the signal-to-noise ratio of echo signals and reduce the obstacles to subsequent signal detection, [43] developed a new grasshopper optimization algorithm to obtain the optimal parameter combination of the optimal variational mode decomposition. Subsequently, the Hausdorff distance was used to select the corresponding mode and combine the basis function to reconstruct the signal. In addition, wavelet transform denoising processing was performed on the relevant patterns to obtain a higher signal-to-noise ratio from the reconstructed signal. In [44], a realization and evaluation method for a direction-determining proximity fuze sensor for air defense missiles was introduced. The Legendre sequence adopted two-phase shift keying modulation, which can improve the suppression ability of clutter signals.
The development of modern military attack and defense weaponry is always conducted simultaneously. When the detection method and anti-jamming strategy are improved, the counter-measuring ability of the jammer to prevent the target from being detected is also improved. Therefore, a smart fuze must be able to counter a jammer frequency sweep. In [45], to improve the anti-sweeping interference ability of the frequency modulation fuze, an anti-jamming method based on timing and related detection was proposed; the distance information on the projectile, dual-channel timing, and time interval serves as the criteria for the detonation control signal output of the fuze. The anti-sweep frequency interference ability of the frequency modulation fuze was improved. The schematic diagram is shown in Figure 8.
To address the problem of poor fixed-distance accuracy of a pseudo-random frequency hopping fuze and difficult engineering implementation, [46] proposed a new method of fuze signal processing based on signal reconstruction. The reflected echo signal was coherently integrated with a set of local reference signals. Subsequently, the peak value exceeding the preset threshold can be used as the fixed distance of the fuze. The output signal is
and the specific parameter explanation is available in [46].
To improve the performance of the anti-sweep frequency interference of the hybrid-modulated continuous-waveform proximity fuze, the response of a hybrid-modulated continuous-waveform proximity fuze under swept-frequency interference was analyzed. [47] proposed an anti-jamming method based on a new average-range side lobe. The output signal of the fuze after interference is expressed as
The signal processing process is shown in Figure 9. In [48], an anti-deception jamming method for the detection of linear frequency modulation continuous waves was proposed. The echo model of multiple scattering centers in the millimeter-wave linear frequency-modulated continuous wave detection system was analyzed as
The objectives of the multi-source fusion anti-environmental interference control method proposed in this paper are to establish an environment multi-physical database, construct an environment recognition function through the corresponding probability and statistics method, and form an environment reference model. In [21, 23, 29], the environment model of the strong electromagnetic environment launching process, a signal echo model of laser proximity fuze under shockwave, and the stress change of the mechanism were established, respectively.
In [31, 32, 34, 35], the environmental reference models of fog, rain, and cloud were established, and the echo signal of the laser fuze in this environment was studied. In addition, the performance of a laser fuze in a dusty environment can be evaluated using the simulated dusty environment established in [36].
The multi-physical environment modeling in [21, 24, 28, 29, 31, 35, 36] included most of the environment models during the launch and flight of the laser fuze, as well as the simulated dust environment constructed for testing the performance of the laser fuze.
In [21, 24, 28, 29, 31, 35, 36], most of the environment models of the laser fuze during launching flight and the simulated dust environment for testing the performance of laser fuze were established. For the electromagnetic railgun fuze, [17–20] formed a relatively complete model of the electric and magnetic field environment in the bore through simulations and experiments. The above models can support the construction of an environment multi-physics database of a smart fuze, but the environment models required by various fuzes still require expansion to enrich the database.
4.2 Time-delay estimation and compensation control assisted by prior information
4.2.1 Time-delay estimation algorithm
Further research is required on the effect of time delay on a fuze control system to increase the damage efficiency of ammunition and realize the accurate estimation and compensation of the time delay of smart fuzes.
To facilitate the study of the effect of time delay on the fuze system, an offline simulation device can be used to test the research object. From the perspective of hardware, to study the detonation time delay of air defense missiles, [49] proposed a hardware design for a real-time tracking system based on digital signal processing (DSP)/field-programmable gate array (FPGA). A second-order debiased conversion measurement Kalman filter was used to estimate the burst time-delay control parameters. With the corresponding time delay estimation algorithm, the system can improve time-delay processing. In [50], the estimated time of the driving time delay of a Doppler fuze was studied. A gray-scale weighted least squares criterion based on the image proposed was used to estimate the driving time delay; its expression is
where the specific parameter explanation is available in [51].
4.2.2 Detection controller: fusion of multi-source information against environmental interference
In this paper, the weapon platform information should be introduced as a priori information in the design of a smart fuze controller; therefore, the information cross-linking technology between the weapon platform and the fuze contributes significantly to ensuring the reliability and accuracy of the fuze. The existing common cross-linking methods are wired energy and information synchronous transmission, and wireless energy and information synchronous transmission. The wireless setting is divided into electromagnetic induction, radio frequency, and laser settings. The wireless setting has the advantages of convenient structure design and fast setting. The wired setting has the advantages of not being interfered with by the external environment, a simple structure, and high setting reliability.
[52] proposed a new 2D communication technology to improve the transmission data rate and three-impedance characteristics of a network ammunition communication link. In this technology, orthogonal frequency division multiplexing (OFDM) was used for 1D data transmission. The G-function-driven subcarrier frequency change law was used for 2D data transmission, breaking through the traditional use of carrier amplitude, frequency, and phase-modulated information. Furthermore, they established a new 2D communication model based on differential frequency hopping (DFH)–OFDM, as shown in Figure 10.
[22] studied the wireless synchronous transmission method of fuze energy and information based on magnetic resonance. To realize the wireless energy supply and information synchronous setting between the setter and ammunition fuze, a pair of separation coils in the strong coupling state of magnetic resonance was proposed.
The mapping relationship is
In [53], aiming at the problems of the short effective transmission distance and low transmission efficiency of the current electromagnetic induction setting method, to realize the fast and efficient transmission of information between the setting device and the fuze, a three-coil magnetic coupling resonance setting information transmission system was proposed. [54] proposed a wireless setting method of fuzes based on magnetic resonance. With this method, two types of information synchronous loading methods based on intermittent energy modulation and resonant modulation were designed, and the load modulation technology was used to realize the reverse transmission of information.
However, the wireless setting strategy has some disadvantages, such as low-setting reliability and weak anti-interference. In [55], a general wired setting system scheme of electronic time fuzes was proposed.
The setting technology based on wired and wireless combinations was studied [56]. The study considered the advantages of wired and wireless settings. The wired and wireless composite setting system diagram is shown in Figure 11.
4.2.3 Time-delay compensation algorithm
To predict and compensate for the time delay, [57] designed an improved linear predictor to compensate for the time delay in position information. The system state was considered to change linearly within the time delay interval. Therefore, the system state can be expressed as
In [58], an improved bidirectional time stamp exchange method was proposed to apply to the field of fuze clock synchronization in a variable-temperature environment to reduce the effect of time delays on fuze systems. This method uses a predetermined fixed-length symbol instead of absolute time exchange for clock synchronization, and it uses the temperature drift statistics of the fuze clock source and the measurement results of the set information synchronization phase to predict the temperature drift; the schematic diagram is shown in Figure 12.
To reduce the information interaction between the weapon platform and the fuze and improve the combat efficiency, the time delay can be adjusted adaptively to solve the time-delay problem. In [59], aiming at the problem that conventional air defense artillery shells cannot achieve accurate detonation, a mathematical model of fuze adaptive time delay based on spectrum characteristics was proposed. In the mathematical model, the actual target was processed into a volume target, the Doppler signal spectrum information about the target was extracted, the rendezvous parameters and the total time delay of the fuze were estimated, the target size was adjusted to compensate for the time delay, and the fuze starting area was set. The relationship between ammunition and target rendezvous parameters and spectrum information is given by
The adaptive time delays for target and warhead size compensation are
The self-triggering delay state controller proposed in this paper realizes the information cross-linking with a weapon platform, the estimation and compensation time delays of a fuze, and the dynamic adjustment of the time-delay weight of each detection source.
First, the time-delay estimation algorithm proposed in this paper was improved and summarized from [22, 49, 50] to guarantee the accuracy of the time-delay estimation. Second, because a priori information must be provided by the weapon platform, this requires high reliability of cross-platform information cross-linking to satisfy the need for accurate estimation of time delay. The wireless, wired, and hybrid induction settings constructed in [52–56] can support the successful implementation of this algorithm. Finally, based on [57, 58, 60], the time-delay compensation algorithm was used to compensate for the estimated time delay value of the fuze detection system to solve the effect of time delays on the timeliness of the fuze control system.
The research results of the above-mentioned references on time-delay estimation and compensation supported the design of the self-triggering time-delay state controller of the smart fuze. However, to realize the high-efficiency detection, decision, and control capabilities of the smart fuze, a higher-accuracy and simple time-delay estimation and compensation algorithm is required. For information cross-linking, the references primarily provide three information transmission methods, all of which can input the prior information on the weapon platform to the state controller. To reduce the effect of time delays on smart fuze systems, high-speed, high-reliability settings and coding methods must be further studied.
4.3 Design of a virtual closed-loop target-adaptive detonation controller
4.3.1 Diversified target modeling
To realize the accurate detonation control of the fuze, target types must be distinguished accurately. The accuracy of target classification directly determines the detonation effect of ammunition. Therefore, research on the target classification method is necessary to achieve efficient damage of the weapon system.
For hard targets, [59] conducted a real-time numerical calculation study of the penetration depth of projectiles into concrete targets. A fuzzy method for the real-time calculation of penetration depth was proposed. Using fuzzy mathematics to classify the membership degree of materials, the deceleration rate can be expressed as
where
Furthermore, to simulate the penetration process of a hard target with a longer pulse width, the improved vertical air hammer test device was used in [59]. The measured impact signal characteristics were compared with the acceleration of the actual penetration process, and the results indicated that they were in good agreement.
Multi-layer hard targets are always the focus of penetrating ammunition attacks. However, the relevant characteristic information on multi-layer hard targets can be obtained through the range recovery test. However, this method has the disadvantages of low reliability and high cost. Therefore, the multi-layer target penetration process must be simulated, and the relevant target characteristic data must be obtained. In [61], aiming at the laboratory simulation experiment of a hard-target-penetration fuze, a simulation experiment of the multi-layer hard-target-penetration process using multiple impact testing machines was proposed. The paper introduces the layer counting strategy of the hard-target-penetration fuze and discusses the feasibility of using the impact process to simulate the acceleration signal of the penetration. The block diagram of the test system is shown in Figure 13.
To effectively attack a hard target, both the characteristics of the target and the protection mechanism of the fuze during the penetration process must be studied. To reveal the dynamic response mechanism of the fuze circuit module during the penetration process, [62] introduced the mechanical vibration theory to theoretically analyze and calculate the penetration process, and they proposed a simplified penetration warhead-fuze system dynamic model. The warhead dynamics model can be expressed as
The dynamic model of the fuze protection system is
Because the relative speed of ammunition interacting with the target is very high, the signal processing time of the laser proximity fuze is limited. Therefore, the laser echo signal model of the intersection process should be studied offline to improve the laser detection system and increase the efficiency of a laser proximity fuze.
In [63], to address the problem of the low damage probability of a single-attack rocket, a laser circumferential detection system was designed, which could improve the target-acquisition probability of an attack rocket. The analytical formula of the pulse laser echo waveform of the plane target and the minimum detectable optical power is derived as follows:
Combined with the terminal ballistic characteristics of the attack rocket, the rendezvous model (32) of the laser circumferential detection system was established.
4.3.2 Burst point control of a corrected fuze
Two methods can be used to increase the damage efficiency of ammunition: first, increasing the charge of ammunition to enhance the detonation damage ability; and second, improving the precision of an ammunition attack to achieve high-efficiency damage under the same charge. For the fuze, a type of fuze with a correctable trajectory was proposed, which could realize the controllable burst point and complete the efficient damage of the target.
In [64], a general guidance strategy of a 2D course-correction fuze based on perturbation canard control was proposed. During the flight, the guidance command can be formed by calculating the simple correction term. The trajectory model of the fuze used in this paper can be considered as follows:
where the above variables belong to the 3D vector and the definition of variables is detailed in [58]. In [65], the actual physical trajectory of the center of mass (or its 6-degree-of-freedom (DOF) model) can be considered the solution to (33). Subsequently, the control input u can be expressed as
where the specific control process is described in [60].
In [65], the guidance and control strategy of a fixed canard wing 2D trajectory correction fuze was studied. First, the correction control mechanism of offset motion was studied, and the phase lag of steering response was observed to be the main factor of correction control. Second, a prediction method of impact-point deviation based on perturbation theory was proposed. The predicted impact-point deviation formula based on perturbation theory can be obtained as
To increase the accuracy of a trajectory-correction fuze, a new type of fuze was proposed in [66]. The transverse and forward components of the trajectory response under control were studied, and the actual miss distance was obtained using the feedback of the imager. The deployment time of the front canard and the roll angle of the front fuze were derived and used as inputs of the control system.
The strategy of the trajectory correction fuze in [66] was as follows: when the projectile enters the descending orbit, the image sensor begins to seek the target at a predetermined time and send feedback to the trajectory deviation. The roll angle and canard deployment time of the projectile are obtained using the deviation, and the fuze was driven to rotate independently. After canard deployment, an aerodynamic control force was generated to reduce the trajectory deviation.
In [67], a unique trajectory correction fuze with fewer sensors and actuators was proposed. An analytical dynamic response model for fast calculation of terminal control force was derived. Subsequently, the effects of velocity, rotation speed, and pitch on the dynamic response were studied using an analytical dynamic response model, which can be expressed as
A new electromechanical video-stabilization strategy for a trajectory-correction fuze was proposed in [67]. The parameters of an infrared image sensor, providing video output and the method of video stabilization, were calculated. A sub-block segmentation method based on an adaptive global gray threshold was proposed. In addition, the gray projection algorithm was used to estimate the global motion vector by calculating the correlation between adjacent frame curves.
In [64], an autopilot with a load factor of pitch/yaw axis was designed for 155-mm rotationally stabilized ammunition. Aiming at a 7-DOF non-linear mathematical model—(37) and (38)—of the entire trajectory, the q-LPV model acquisition method and the autopilot design in Figure 14 were provided.
The translational dynamics model is
The altitude airframe dynamics can be expressed as
4.3.3 Burst point control of a proximity fuze
After the proximity fuze is detonated, the damage to the target caused by the fragments produced by the warhead should be studied to determine damage effectiveness. In [68], the relationship between warhead fragment space parameters and target was studied, and the calculation model of the damage unit hitting the damage target position was established. Furthermore, the calculation function of the damage area of a warhead under different rendezvous altitudes was obtained, and the calculation model of the damage probability of the warhead fragment was derived.
The condition for fragments to hit the target is
At the time t of the projectile and target rendezvous, the coordinate position of the target in the fragment concentration can be obtained as
For the proximity fuze, the width of the target damage zone generated by the initiating warhead is
The probability of k fragments hitting the target can be expressed as
According to the results of the impact of warhead fragments on the damage effectiveness studied in [68], an optimal detonation point and direction must exist to improve the damage effectiveness. Therefore, the proximity smart fuze is required to have a directional detonation function.
In [69], aiming at the problem that the traditional fixed-beam inclination fuze cannot satisfy the requirements of a fuze warhead coordination of new generation air defense missiles, a detonation control method based on the two-dimensional beam control fuze was proposed. The plane-phased array technology was introduced into the fuze antenna, which realizes the 2D control of the beam and establishes the best detonation angle and azimuth model of the fuze.
The best detonation angle model is
The best detonation azimuth model is
To achieve the best directional detonation requirement for conventional ammunition, [70] established the best detonation time delay model (45) and angle model (46) of the pulsed laser circumferential detection system for the projectile and target rendezvous environment:
where
and
In [20], the optimal detonation model and simulation of multi-detection points for the synchronous scanning circumferential-view pulse laser fuze were studied. A target velocity model with multiple detection points was established, the geometric center position of the target feature was calculated, and the optimal detonation time and azimuth angle calculation model were established.
The established multi-target velocity model can be considered as
The best detonation conditions must satisfy
and
[71] researched the fuze warfare coordination of artillery-launched electromagnetic pulse projectiles in two stages. First, a model of the electromagnetic pulse bomb killing area was established, and the optimal detonation point was analyzed to satisfy the conditions. Second, the optimal detonation timing model was established, and the cause of the delay error was analyzed. The schematic diagram of the electromagnetic pulse bomb is shown in Figure 15. The relationship that the best detonation point
4.3.4 Burst point control of a penetration fuze
The core of burst point control in a penetration fuze is to identify the overload threshold accurately and rapidly, and output the detonation signal within the shortest time.
In [72], a simulation modeling method of separating the projectile body and fuze was proposed to obtain the penetration acceleration signal of the fuze. In particular, short-time shielding methods were used to avoid multiple layers, owing to oblique penetration. To improve the real-time performance of the penetration detonation control system, [73] used DSP as the core, combined with AD7656, and replaced the quartz crystal with an all-silicon oscillator. A miniaturized real-time information processing penetration detonation control system was constructed, which can resist high impacts and overloads; a trapezoidal integral method was used to calculate real-time penetration depth online. The expression is
The current penetration-fuze layer target recognition algorithm frequently uses a fixed threshold or multiple thresholds to analyze the target layer signal. The setting of thresholds frequently relies on a priori data, which is not universal. In response to this problem, [74] proposed an adaptive threshold layer target-recognition algorithm. According to the sensor signal collected by the fuze, the method can calculate the decision threshold of the next layer in real time and then determine the current layer number.
The signal processing method proposed in [64] can be expressed as
The principle is to convolve the acceleration and switching signals in a specific time window and then add them according to a certain weight to obtain the final signal. In (51),
The adaptive dynamic threshold algorithm is
where
In this paper, a virtual closed-loop target adaptive detonation control method was proposed, which aims at achieving the best damage effect of smart fuzes on different targets. Offline time, based on the research of [59, 61–64, 75], combined with a large amount of range test data, the target feature database was constructed to form the target function. Owing to different targets, the best damage mode was different. The mapping relationship between the target characteristic value and the detonation mode was constructed from [20, 64–74, 76–80] and the measured data. The mapping relationship was used to optimize the parameters of the detonation controller to obtain the optimal controller. At online moments, multi-source detection information, target feature databases, and weapon platform setting information output the target characteristic value through the recognition function. The optimal detonation controller quickly decides the fuze detonation method based on the characteristic value and state information on the target to achieve maximum damage effects.
The research results in the above references were efficient, but to enrich the target database, it is still necessary to study thin armor, active force, high maneuvering targets, etc., and cooperate with the corresponding burst point control algorithm to ensure that the optimal detonation controller uses the target characteristic value to achieve efficient damage.
5 Prospects for the development of fuze technology: from smart to intelligence
For the future direction of fuze development, we adhere to the demand- and technology-driven orientation and closely focus on the development of fuze technology with new combat modes, environments, weapon systems, and attack targets. To realize the further development of smart fuze technology, the following are the new requirements and development directions of fuze technology in the future:
(1) In response to the new requirements of the ammunition combat mode, a multi-domain and multi-target-coordinated detection, cross-linking, attack fuze technology must be established. Through the fuze, it can be interconnected and coordinated on the integrated battlefield of land, sea, air, and space and form a more powerful precision attack capability.
(2) Aiming at the new requirements of a wide-area combat environment, the environment and target database covering the entire process from service processing to weapon platform, launch, flight, detonation, and landing are further improved to place the entire lifecycle of the fuze under safe and reliable precise control.
(3) Aiming at the new requirements of weapon systems, the electromagnetic railgun and other new weapons with stronger damage effectiveness are focused. To overcome the reliability and timeliness of fuze under long pulse width, high overload mechanical impact, and Tesla-level strong magnetic field coupling interference, a new type of intelligent fuze suitable for ultra-high speed ammunition should be studied.
(4) Aiming at the new requirement of attacking high-strength hard targets, smart fuze technology suitable for hypervelocity penetration ammunition is being established, and the high-efficiency damage of concrete-protective structure targets with tens of meters of high strength is formed.
6 Conclusion
(1) Three types of controllers were constructed based on the three key challenges: environment-adaptive detection controller, self-triggering time-delay compensation state controller, and virtual closed-loop detonation controller.
(2) The ternary cascade control design theory of the smart fuze was established, which improves the precise detonation control of the smart fuze and the efficient damage ability of the ammunition.
(3) The technical system of the above-mentioned ternary cascade control theory is reviewed, and the latest progress in control strategy design, environment modeling, target modeling, and other fields is summarized in detail.
(4) The development demand and technology trend of intelligent fuze control technology in future intelligent wars are prospected.
Based on the existing smart fuze control theory, further research on the intelligent fuze control theory and technology is required, and the design theory of the fuze and warhead coordination of intelligent ammunition under network information should be established. By exploring new technology, developing new modes, and constructing new systems, we can realize the surge in the development from smart fuzes to intelligent fuzes.
Author contributions
PL: conceptualization, data curation, software, and writing–original draft. JL: conceptualization, investigation, supervision, and writing–original draft. HY: conceptualization, formal analysis, methodology, resources, and writing–review and editing. HZ: methodology, resources, supervision, validation, and writing–review and editing.
Funding
The author(s) declare that no financial support was received for the research, authorship, and/or publication of this article.
Conflict of interest
The authors declare that the research was conducted in the absence of any commercial or financial relationships that could be construed as a potential conflict of interest.
The reviewer KD declared a shared affiliation with the author(s) to the handling editor at the time of review.
Publisher’s note
All claims expressed in this article are solely those of the authors and do not necessarily represent those of their affiliated organizations, or those of the publisher, the editors, and the reviewers. Any product that may be evaluated in this article, or claim that may be made by its manufacturer, is not guaranteed or endorsed by the publisher.
References
1. Zhang H, Jiang XH. Target detection and recognition technology. Beijing: Beijing Institute of Technology Press (2014).
2. Zhang H, Li CS. Basic theory and application of smart fuze design. Beijing: Beijing Institute of Technology Press (2019).
5. Pinto RMR, Gund V, Dias RA, Nagaraja KK, Vinayakumar KB. CMOS-Integrated aluminum nitride MEMS: a review. J Microelectromechanical Syst (2022) 31(4):500–23. doi:10.1109/jmems.2022.3172766
6. Bouza M, Altieri A, Galarza CG. Robust target classification using UWB sensing. IEEE Access (2023) 11:44267–77. doi:10.1109/access.2023.3273152
7. Mian T, Choudhary A, Fatima S. Multi-sensor fault diagnosis for misalignment and unbalance detection using machine learning. IEEE Trans Industry Appl (2023) 59:5749–59. doi:10.1109/tia.2023.3286833
8. Mbarek B, Ge M, Pitner T. An adaptive anti-jamming system in HyperLedger-based wireless sensor networks. Wireless Networks (2022) 28(2):691–703. doi:10.1007/s11276-022-02886-1
9. Pirayesh H, Zeng H. Jamming attacks and anti-jamming strategies in wireless networks: a comprehensive survey. IEEE Commun Surv tutorials (2022) 24(2):767–809. doi:10.1109/comst.2022.3159185
10. Suseendran G, Akila D, Vijaykumar H, Jabeen TN, Nirmala R, Nayyar A. Multi-sensor information fusion for efficient smart transport vehicle tracking and positioning based on deep learning technique. The J Supercomputing (2022) 78:6121–46. doi:10.1007/s11227-021-04115-6
11. Adla N, Bhukya PK, Arnepalli DN. Coupled thermo-hydro-mechanical model for simulating thermal history-induced voids in buffer material. Geomechanics Energ Environ (2023) 36:100513. doi:10.1016/j.gete.2023.100513
12. Muldoon K, Song Y, Ahmad Z, Chen X, Chang MW. High precision 3D printing for micro to nano scale biomedical and electronic devices. Micromachines (2022) 13(4):642. doi:10.3390/mi13040642
13. Zhihua P, Chengtian S, Bohu L, Xiaowen W, Mengqian D, Hong S. Accurate ranging of dual wavelength FMCW laser fuze under different types of aerosol interference. IEEE Sensors J (2022) 22(19):18953–60. doi:10.1109/jsen.2022.3196547
14. Pramudita AA, Lin DB, Dhiyani AA, Ryanu HH, Adiprabowo T, Yudha EA. FMCW radar for non contact bridge structure displacement estimation. IEEE Trans Instrumentation Meas (2023) 72:1–14. doi:10.1109/tim.2023.3292960
15. Hanif A, Muaz M, Hasan A, Adeel M. Micro-Doppler based target recognition with radars: a review. IEEE Sensors J (2022) 22(4):2948–61. doi:10.1109/jsen.2022.3141213
16. Ruiz M, Nieto J, Costa V, Craciunescu T, Peluso E, Vega J, et al. Acceleration of an algorithm based on the maximum likelihood bolometric tomography for the determination of uncertainties in the radiation emission on JET using heterogeneous platforms. Appl Sci (2022) 12(13):6798. doi:10.3390/app12136798
17. Zambon P. Enhanced DQE and sub-pixel resolution by single-event processing in counting hybrid pixel electron detectors: a simulation study. Front Phys (2023) 11:1123787. doi:10.3389/fphy.2023.1123787
18. Kovacic I, Rakaric Z, Kanovic Z, Rajs V. Metastructure with integrated internal oscillators of constant, linearly and nonlinearly varying natural frequency. Front Phys (2022) 10:934998. doi:10.3389/fphy.2022.934998
19. Zhang H. Development of ammunition demands and promotion of fuze technology. J Ordnance Equipment Eng (2018) 39(03):1–5. doi:10.11809/bqzbgcxb2018.03.001
20. Zhang H, Li HX, Ding LB, Zha BT. Modeling and simulation of optimal initiation of multiple detection points for synchronous scanning peripheral pulse laser fuze. Infrared Laser Eng (2020) 49(04):9–15.
21. Yao ZC, Zhang H, Zhang XJ, Huang ZX, Gan L, Zhu HY. Analysis on the influence mechanism of conical shock wave on pulsed laser forward detection. Optik (2019) 183. doi:10.1016/j.ijleo.2019.02.093
22. Li CS, Zhang H. Research on wireless power and information synchronous transmission method based on magnetic resonance for fuzes. Acta Armamentarii (2011) 32(05):537–42.
23. Guo B, Ren K, Li Z, Chen R. Modelling on shock-induced energy release behavior of reactive materials considering mechanical-thermal-chemical coupled effect. Shock and Vibration (2021) 2021:1–12. doi:10.1155/2021/6669573
24. Yin Q, Zhang H, Li HJ. Analysis of in-bore magnetic and electric fields in electromagnetic railgun under dynamic condition. Acta Armamentarii (2017) 38(06):1059–66. doi:10.3969/j.issn.1000-1093.2017.06.003
25. Yin Q, Zhang H, Li HJ, Yang YX. Analysis of in-bore magnetic field in C-shaped armature railguns. Defence Tech (2019) 15(01):83–8. doi:10.1016/j.dt.2018.07.009
26. Dai KR, Yang YX, Yin Q, Zhang H. Theoretical model and analysis on the locally concentrated current and heat during electromagnetic propulsion. IEEE Access (2019) 7(7):164856–66. doi:10.1109/access.2019.2952981
27. Yang YX, Dai KR, Yin Q, Liu P, Yu D, Li HJ, et al. In-bore dynamic measurement and mechanism analysis of multi-physics environment for electromagnetic railguns. IEEE Access (2021) 9(9):16999–7010. doi:10.1109/access.2021.3053255
28. Li XQ, Xiang AH, Li HX. Calculation and assessment on damage effect of system target. Acta Armamentarii (2008)(01) 57–62. doi:10.3321/j.issn:1000-1093.2008.01.013
29. Gan L, Zhang H, Wang JH, Liu B. Influence of dynamic impact on optical properties of optical transmission window of laser measurement system. Optik (2020) 202:163643. doi:10.1016/j.ijleo.2019.163643
30. Yin Q, Zhang H, Li HJ, Yang YX. Analysis of in-bore magnetic field in electromagnetic railgun considering the realistic armature-rail contact status. Acta Armamentarii (2019) 40(03):464–72. doi:10.3969/j.issn.1000-1093.2019.03.003
31. Zhang W, Li Y, Huang ZH. Research on the characteristics of fog backscattering signals for frequency modulated continuous wave laser fuze. Optik (2016) 127(20):9046–55. doi:10.1016/j.ijleo.2016.06.129
32. Zhang W, Li Y, Huang ZH, Ma C. Fog backscattering interference suppression algorithm for FMCW laser fuze based on normalized frequency spectrum threshold. Optik - Int J Light Electron Opt (2017) 131:188–93. doi:10.1016/j.ijleo.2016.11.084
33. Wang FJ, Chen HM, Ma C, Xu LX. Construction of backscattering echo caused by cloud in laser fuze. Optik (2018) 171:153–60. doi:10.1016/j.ijleo.2018.06.028
34. Guo J, Zhang H, Zhang XJ. Analytical model for optical scattering of infrared laser by nonspherical raindrops. Int J Opt (2015) 1:1–6. doi:10.1155/2015/376898
35. Guo J, Zhang H, Zhang XJ. Propagating characteristics of pulsed laser in rain. Int J Antennas Propagation (2015) 4:1–7. doi:10.1155/2015/292905
36. XuChen YS, Zhang H, Zha BT, Zhen Z, Chen JY. Numerical simulation of dust concentration distribution in confined space. Acta Armamentarii (2020) 41(3):618–24. doi:10.3969/j.issn.1000-1093.2020.03.023
37. Liu YL, Li XJ, Lu CP, Li WX. Comparison on anti-jamming performance of composite fuze. Guidance and fuze (2019) 40(1):6–9. doi:10.3969/j.issn.1671-0576.2019.01.002
39. Chen ZT, Ma J. Dual wavelength laser fuze. J Detect Control (2008) 30(5):1–3. doi:10.3969/j.issn.1008-1194.2008.05.001
40. Guo JC, Liu X, Yuan J, Lu H. Target recognition algorithm for laser/infrared composite scanning fuze. Laser and infrared (2020) 50(2):184–91. doi:10.3969/j.issn.1001-5078.2020.02.010
41. Fu C, Pan X, Song CT. Frequency Modulated Continuous Wave laser and radio compound detecting technology. J Terahertz Sci Electron Inf Tech (2016) 14(1):40–5.
42. Duan YB. Research on detection technology of frequency modulated continuous -wave system laser and radio combination fuze. Beijing: Beijing Institute of Technology (2017).
43. Jiao PB, Huang CQ, Cai J, Ding DL, Wang Z. Target recognition of compound fuze based on temporal-spatial information fusion. J Detect Control (2014) 36(5):47–50.
44. Choi J, Jung M, Yeom K. A design and assessment of a direction finding proximity fuze sensor. IEEE SENSORS JOURNAL (2013) 13(8):3079–89. doi:10.1109/jsen.2013.2260326
45. Kong ZJ, Hao XH, Li P, Wang Z. Research on anti-frequency sweeping jamming method for frequency modulation fuze based on timing sequence and correlation detection. Acta Armamentarii (2017) 38(8):1483–9. doi:10.3969/j.issn.1000-1093.2017.08.004
46. Tu YC, Si C, Wu W. Pseudo-random frequency hopping fuze technology based on signal reconstruction. IET Signal Process. (2016) 10(3):302–8. doi:10.1049/iet-spr.2015.0410
47. Chen QL, Hao XH, Kong ZJ, Yan XP. Anti-sweep-jamming method based on the averaging of range side lobes for hybrid modulation proximity detectors. IEEE Access (2020) 8(8):33479–88. doi:10.1109/access.2020.2974042
48. Wang MW, Xiao ZL, Gao W, Rong YJ. An anti-deception jamming method for LFMCW detector. Acta Armamentarii (2019) 40(4):753–61. doi:10.3969/j.issn.1000-1093.2019.04.010
49. Zhang LZ, Wu PL, Zhang XY. Air defense missile detonation delay control Based on FPGA/DSP. WSEAS Trans Syst (2013) 12(4):202–11.
50. Li YZ, Ge ZQ, Liu Y. Actuation delay-time estimation with imaging fuze. J Beijing Inst Tech (2017) 26(4):557–62.
51. Hua T, Dai K, Zhang XJ, Yao ZC, Wang HJ, Xie KF, et al. Optimal VMD-based signal denoising for laser radar via Hausdorff distance and wavelet transform. IEEE Access (2019) 7:167997–8010. doi:10.1109/access.2019.2949063
52. Zhou F, Fan FF, Jiang YQ, Feng YX. Two-dimensional new communication technology for networked ammunition. IEEE ACCESS (2020) 8:133725–33. doi:10.1109/access.2020.3011031
53. Li WX, Zhang H, Ding LB, Li CS. Two-way transmission technology of setting information of magnetic coupling resonance fuze. Fire control and command control (2014) 39(7):89–92.
54. Li CS, Zhang H. Wireless setting method of fuze based on magnetic resonance. J Detect Control (2014) 36(2):1–6.
55. Hong L, Zhang H, Ding LB. Design of universal wire setting system of electronic time fuze. Manufacturing automation (2012) 34(16):113–6. doi:10.3969/j.issn.1009-0134.2012.8(x).34
56. Qiu TY. Research on setting technology based on wired and wireless combination. Nanjing: Nanjing University of Science and Technology (2019).
57. Guo XW, Pan KD, Wang QJ, Wen Y. Robust adaptive sliding-mode control of a permanent magnetic spherical actuator with delay compensation. IEEE ACCESS (2020) 8:128096–105. doi:10.1109/access.2020.3008152
58. Liao X, Zhang H, Li HJ, Gao K. Clock synchronization between fuze and weapon platform under variable temperature. J Detect Control (2018) 40(4):11–5.
59. Zhang DM, Gao SQ, Liu HP, Liu ZB. Real-time numerical calculation for penetration depth of projectiles into concrete targets. Mech Based Des Structures Machines (2018) 46(4):520–31. doi:10.1080/15397734.2017.1362985
60. Zhang FP, Liu SY, Dai L. Fuze adaptive delay time mathematics model based on spectrum feature. J Detect Control (2011) 33(2):31–4.
61. Man XF, Zhang H, Mao SJ, Wang XF. Study of physical simulation experiment of penetration fuze layer-count burst point control. Acta Armamentarii (2014) 35(10):1556–61. doi:10.3969/j.issn.1000-1093.2014.10.006
62. Chen SS, Liu B, Zhao H, Liu J. Dynamic modeling and simulation for penetration warhead-fuze system. Acta Armamentarii (2020) 41(4):625–33. doi:10.3969/j.issn.1000-1093.2020.04.001
63. Chen SS, Zhang H, Xu XB. Modeling and simulation of acquisition for ground target by pulsed laser circular-viewing detection. Infrared Laser Eng (2018) 47(2):113–23.
64. Spilios T, Florian S, Philippe W. Robust gain-scheduled autopilot design for spin-stabilized projectiles with a course-correction fuze. Aerospace Sci Tech (2015) 42:477–89. doi:10.1016/j.ast.2014.12.027
65. Wang Y, Song W, Fang D Guidance and control design for a class of spin-stabilized projectiles with a two-dimensional trajectory correction fuze. Int J Aerospace Eng (2015) 5:1–15. doi:10.1155/2015/908304
66. Li RP, Li DG, Fan JR. Correction strategy of mortars with trajectory correction fuze based on image sensor. Sensors (Basel, Switzerland) (2019) 19(5):1211–26. doi:10.3390/s19051211
67. Zhang C, Li DG. Mechanical and electronic video stabilization strategy of mortars with trajectory correction fuze based on infrared image sensor. Sensors (Basel, Switzerland) (2020) 20(9):2461–75. doi:10.3390/s20092461
68. Li H, Sang X, Zhang X Modeling and analysis of target damage under the multi-posture projectile and target intersection. IEEE ACCESS (2019) 7:135732–41. doi:10.1109/access.2019.2942694
69. Yang J, Jian JL, Ren HB, Xu Y. Initiation control of two dimensional beam controlled Fuze. J Detect Control (2016) 38(4):52–7.
70. Xu XB, Zhang H. Optimum directional detonating technology for pulsed laser circumferential detection. Acta photonica sinica (2016) 45(5):13–8.
71. Shi YL, Zhang H, Ma SJ, Gu L. Coordination of fuze and warhead for gun-launched electron-magnetic pulse bomb on actual ballistics. Hign Power Laser Part beams (2014) 26(11):244–9. doi:10.11884/HPLPB201426.115004
72. Li HJ, Hu HC. Layer counting detonating control method for fuze based on penetration overload numerical simulation. J Nanjing Univ Sci Tech (2016) 40(6):715–9.
73. Liu ZB, Gao SQ, Niu SH, Liu HP. Real-time information sampling and processing and penetration depth identification of penetrating detonation control system. Transaction Beijing Inst Tech (2016) 36(10):1025–30.
74. Huang SL, Zhu HZ, Cheng XL, Xie YB. Research on adaptive threshold setting method for layer-count in penetration fuze. Acta Armamentarii (2020) 41(09):1762–71. doi:10.3969/j.issn.1000-1093.2020.09.008
75. Man XF, Men SY, Mao SJ, Zhang H. Using air hammer device simulate hard target penetration of process. J Detect Control (2016) 38(3):90–3.
76. Robinson JWC, Stromback P. Perturbation based guidance for a generic 2D course correcting fuze. Boston, MA, United states: AIAA International (2013).
77. Li RP, Li DG, Fan JR. Dynamic response analysis for a terminal guided projectile with a trajectory correction fuze. IEEE ACCESS (2019) 7:94994–5007. doi:10.1109/access.2019.2928718
78. Zhou Y, Cao R, Li P. A target spatial location method for fuze detonation point based on deep learning and sensor fusion. Expert Syst Appl (2023) 238:122176. doi:10.1016/j.eswa.2023.122176
79. Huang D, Yan X, Dai J, Wang X, Liu Y. Cognitive interference decision method for air defense missile fuze based on reinforcement learning. Defence Tech (2023). doi:10.1016/j.dt.2023.04.002
Keywords: smart fuze, time–space recognition, process control, ternary cascade control theory 1, control technology
Citation: Liu P, Li J, Yu H and Zhang H (2024) Innovation of control technology for smart fuzes: precise detonation and efficient damage via a ternary cascade controller. Front. Phys. 11:1309373. doi: 10.3389/fphy.2023.1309373
Received: 13 October 2023; Accepted: 27 November 2023;
Published: 11 January 2024.
Edited by:
Teddy Craciunescu, National Institute for Laser Plasma and Radiation Physics, RomaniaReviewed by:
Lukáš Hubka, Technical University of Liberec, CzechiaKeren Dai, Nanjing University of Science and Technology, China
Copyright © 2024 Liu, Li, Yu and Zhang. This is an open-access article distributed under the terms of the Creative Commons Attribution License (CC BY). The use, distribution or reproduction in other forums is permitted, provided the original author(s) and the copyright owner(s) are credited and that the original publication in this journal is cited, in accordance with accepted academic practice. No use, distribution or reproduction is permitted which does not comply with these terms.
*Correspondence: Hang Yu, hangyu@njust.edu.cn
†These authors have contributed equally to this work