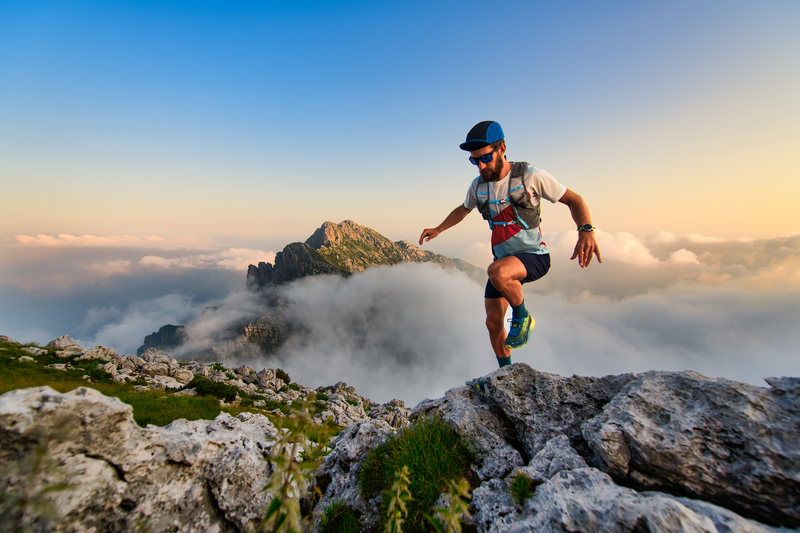
95% of researchers rate our articles as excellent or good
Learn more about the work of our research integrity team to safeguard the quality of each article we publish.
Find out more
ORIGINAL RESEARCH article
Front. Phys. , 19 December 2023
Sec. Radiation Detectors and Imaging
Volume 11 - 2023 | https://doi.org/10.3389/fphy.2023.1308321
This study presents the performance assessment of a novel hybrid pixel detector. The electron counting application-specific integrated circuit (ASIC), named KITE, was optimized for 4D STEM applications and bump-bonded to a silicon sensor that is suitable for electron energies in the range 30–200 keV. The KITE ASIC was developed for high frame rates, currently up to 120 kfps, and high count rates, owing to fast signal shaping times and instant retrigger technology, which allows operation in a unique non-paralyzable counting mode. Investigated quantities include the spectral response, the identification of optimal threshold energies, an estimation of the threshold trimming accuracy, the analysis of the single-event multiplicity distributions, the count rate capabilities, and imaging performance metrics MTF and DQE. To highlight the capabilities of the detector, the best value of 10% of linearity loss was achieved at 84 Mcts/s/pix, the best value of count rate “cutoff” at nearly 150 Mcts/s/pix, and DQE (0) between 0.75 and 0.82 up to the electron energy of 160 keV.
During the last decades, various techniques based on scanning transmission electron microscopy (STEM) have been developed as prominent means for the investigation of sample structure and properties at the nanometer scale [1–3]. Technological breakthroughs in electron optics, in particular the advent of aberration-corrected devices, pushed the spatial resolving capabilities of modern instruments down to the sub-Angstrom regime [4, 5]. This development has brought the high-resolution STEM (HR-STEM) sample characterization to the forefront in many disciplines spanning across material sciences, physics, and chemistry. Further sub-technique development allowed for the study of material phenomena at the atomic level, such as atomic electric fields [6, 7] and atomic charge distributions [8–10].
During the STEM experiment, a small focused electron probe with a defined convergence angle is scanned across the specimen in a raster fashion. At each scanning position, a slice of the reciprocal space1 is projected onto the detectors. Conventional STEM microscopes are equipped with an array of circular and annular-shaped charge-integrating devices. Each detector channel collects electrons at different scattering angles as defined by the physical size of the detector and the magnification of the projection system (commonly referred to as the camera length). The corresponding portion of the transmitted electrons is integrated, and each detector channel returns a single value at each pixel, as the beam is scanned across the specimen. This intensity is then displayed as the brightness of the corresponding pixel. On the one hand, a key advantage of such detectors lies in the high acquisition speed, with dwell times2 typically on the order of microsecond or even less. Short dwell times are necessary in order to acquire a full image in a reasonably short time to counteract the detrimental effects of specimen drifts and radiation damage [3]. Although fast, these systems provide very limited resolution in reciprocal space. A first improvement was the introduction of segmented detectors, where the reciprocal space of circular/annular detectors is subdivided into several channels. This approach allows for the formation of differential phase-contrast (DPC) images to visualize electromagnetic fields in the specimen [11]. However, the richness of information available in the reciprocal space can only be accessed with fine sampling of the diffraction space by using a pixelated detector. This technique is commonly referred to as 4D STEM. The potential benefits of accessing the complete information contained within it and the scattering distribution using pixelated detectors were demonstrated, e.g., in the field of STEM imaging [12, 13], strain measurement [14], charge density and distribution imaging [15, 16], and electron ptychography [17, 18]. To achieve a widespread use, pixelated detectors need to fulfill a set of demanding performance requirements. First, the frame rate should be in the range of 10–100 kfps (as stated in [3]) or higher. Second, the dynamic range should be high—in the order of 10 pA/pix, approximately 6.2 ⋅107 electrons per second per pixel (el/s/pix)—with single-electron sensitivity, which allows simultaneously detecting both the central portion of the scattering distribution containing the majority of electrons and the higher-angle scattered signal, occurring with much lower probability.
In a recent work [19], we introduced a novel electron counting application-specific integrated circuit (ASIC) named KITE, which, in combination with a suitable hybrid pixel semiconductor sensor, aimed at offering a balanced solution to the abovementioned requirements. The chip key features are a pixel size of 100 μm × 100 µm arranged in a matrix of 192 × 192 elements; a pixel front-end electronics that is compatible with both the hole and electron collection modes required by standard silicon and high-Z sensors (acting as direct detection layers). The detector exhibits no dark counts, due to a counting threshold well above the noise level, and no spurious counts associated to the readout operation, since data are digitized in the pixel, allowing the detection of single electron events, while maintaining extremely high count rate capability up to 108 counts per second per pixel (cts/s/pix), owing to the instant retrigger technology [20]. The detector design allows for continuous frame rates as high as 120 kfps, extendable to ∼500 kfps with an improved readout hardware, with massive readout parallelization and two on-chip data compression mechanisms, namely, floating-point counter encoding and 2 × 2 pixel digital binning.
Flexibility in the choice of the sensor material allows optimally addressing the wide range of electron energies expected in 4D STEM applications, which can typically span from 30 keV to 300 keV. For instance, high-Z sensors such as cadmium zinc telluride (CZT) are better indicated for electron energies ≥200 keV because of their higher stopping power, which translates into a superior modulation transfer function (MTF) and higher detective quantum efficiency (DQE) at a high spatial frequency. On the other hand, the silicon sensor is more suitable for lower energies given their superior response uniformity, stability, and higher DQE at zero spatial frequency (DQE (0)), due to high material maturity in the semiconductor industry. In [19], we reported the first performance assessment of the KITE ASIC bump-bonded to a CZT sensor at the electron energies of 200 keV and 300 keV. In this work, we report the performance assessment of the KITE ASIC bump-bonded to a silicon sensor at the electron energies ranging from 30 keV to 200 keV. This article is meant to present a comprehensive characterization of the detector system and serve as a reference during 4D STEM detector selection and operation. Examples of early 4D STEM results using the KITE ASIC can be found in [21, 22].
To ease the reading, we report a summary of the KITE ASIC features which were described in detail previously in [19].
The chip was designed and fabricated in the commercially available UMC 110 nm CMOS technology process with eight metal layers. Enclosed layout transistors (ELTs) were used to ensure a higher tolerance toward ionizing radiation [23]. Figure 1 shows the high-level block diagram of the ASIC, comprising three main blocks organized in a modular and hierarchical structure: the pixel array, row, column and configuration control blocks, and the I/O pad ring. Pixels have a physical size of 100 μm × 100 µm and are arranged in a gapless matrix of 192 × 192 elements. Ancillary electronics and wire-bond pads for power, control, and readout are located at all the four sides of the die, as the ASIC is intended for single-chip hybrid detector assembly.
FIGURE 1. Simplified block diagram of the KITE ASIC [19].
The pixel front-end electronics consists of a charge-sensitive amplifier (CSA) with selectable gain that accepts bipolar input signals and is compatible with both the hole and electron collection mode required by standard silicon sensors and the most commonly used high-Z sensors, namely, gallium arsenide (GaAs), cadmium telluride (CdTe), and CZT. The feedback network includes a transistor operated in the Ohmic regime to provide a path for the sensor leakage current and for a fast signal discharge. The CSA, which is optimized toward speed, can shape signals as fast as ∼6 ns FWHM, according to the chip settings.
The CSA directly feeds a single-ended differential amplifier acting as comparator stage for the thresholding mechanism. The comparator includes a 6-bit digital-to-analog converter (DAC) trimming circuit for precise individual-pixel threshold equalization and the instant retrigger technology [20], which allows the system to work a specific non-paralyzable mode by counting the time-over-threshold of piled-up signals in multiples of a predefined and selectable retrigger time τR. The retrigger block, which is enabled and disabled by an internal register, can deliver retrigger times as low as ∼10 ns, thus significantly increasing the response linearity range toward high count rates, reaching count rate saturation at 108 ct/s/pix. The comparator/retrigger output feeds the counter logic that comprises two 12-bit ripple counters allowing for continuous readout mode operation3.
A high frame rate capability was obtained in two ways. First, top and bottom chip peripheries feature 64 single-ended, serial data out (SDO) lines each for massive parallel readout, which occurs from the middle of the chip to the periphery. Second, the two types of on-chip data compression mechanisms were implemented, namely, the floating-point counter encoding and the 2 × 2 pixel digital binning. The floating-point counter encoding converts the 12-bit counter value from an integer to an 8-bit floating-point representation on-the-fly and with negligible overhead, using 5 bits for the mantissa and 3 bits for the exponent and implementing the implicit leading bit technique, yielding a compression factor of 3/2. The maximum representable numbers for the integer and floating-point representation are similar, 4,095 and 4,032, respectively. The discretization relative error introduced by the floating-point representation is below ∼3% over all the counting range and, in any case, not higher than the one obtained by the Poisson statistics of the incoming beam. The 2 × 2 pixel digital binning mechanism merges the counts of four neighboring pixels at the counter level (hence digitally), yielding a compression factor of 4. The two mechanisms are enabled independently, thus yielding an overall compression factor of 6. Figure 2 shows a simplified schematic diagram of the signal readout from the front-end electronics of a representative pixel to the corresponding serial data output pin. Currently limited by the 10-Gb/s bandwidth of the readout hardware, the frame rate ranges from 20 kfps in the normal mode up to 120 kfps with both compression mechanisms enabled. Without this limitation, VHDL simulations show that it would be, in principle, possible to reach frame rates ranging from 120 kfps in the normal mode up to ∼500 kfps with both compression mechanisms enabled, with a generated data rate in the order of 45 Gb/s. In terms of power consumption, static dissipation is 1.25 W while the dynamic dissipation is at most ∼4 W.
FIGURE 2. Simplified schematic diagram of the signal readout scheme. The pixel matrix is ideally divided into two halves, read out in a row-by-row fashion by the top periphery (not shown) and the bottom periphery, respectively. Each serial data out line collects data from three pixel columns. Overall, 128 SDO are read out in parallel by the external readout electronics.
A KITE ASIC was bump-bonded to a commercially available silicon sensor manufactured by Hamamatsu, with a thickness of 450 µm and a pixel size matching the size of the chip, i.e., 100 μm. The bias voltage applied to the uniform, aluminized side of the sensor was +400 V. The adoption of such relatively high voltage assures fast carrier drift time to the collecting electrodes, while minimizing charge sharing effects due to thermal diffusion and possible ballistic deficit (with consequent slowing down of the count rate performance) due to very fast shaping times of the front-end electronics. The assembly was glued and wire-bonded to a support PCB which was mounted on a vacuum flange equipped with a feed-through connector to the readout board. The readout board also provides the trigger signal and ASIC power supplies. The flange was manufactured with a hollow pipe serpentine connected to a chiller for active water cooling at 20°C. The readout board was connected to a Linux server for control (through Python API) and data storage via a single fiber-optic cable. As previously mentioned, the detector was bandwidth-limited to 10 Gb/s by the readout board and fiber-optic connection to the server.
For the experimental characterization, we used both X-ray and electron radiation. As an X-ray source, we used a GE ISOVOLT TitanE 160 kVp tube with a W anode, exciting fluorescence radiation from a set of elemental targets. As an electron source, we used a FEI Tecnai F20 microscope, which is able to deliver an electron beam with energies up to 200 keV. Although the electron energy can be tuned virtually in a continuous manner, we restricted our study to a discrete set comprising 30 keV, 40 keV, 60 keV, 80 keV, 100 keV, 120 keV, 160 keV, and 200 keV, as they are the most common in TEM applications. The detector assembly was mounted on axis at the bottom of the microscope column, and a Faraday cup was also available for absolute flux reference. Figure 3 shows a schematic view of the experimental setup when used in the electron microscope.
As a tool for obtaining a deeper understanding of the underlying physics and for cross-checking purposes with the experimental data, we set up a Monte Carlo-based simulation framework that comprises the following steps. First, a set of statistical relevant number of electron tracks (“events”) and the corresponding energy deposition in the sensor material is obtained using the FLUKA Monte Carlo code4 [24, 25]. Then, a custom-developed Python code performs the computation of the signal collection at the pixel, taking into account charge sharing effects due to the electron track size, thermal diffusion of the charge cloud treated as a Gaussian blurring and proportional to the absorption depth, and the electronic noise introduced by the front-end electronics. The computed signals undergo a thresholding process mimicking the counting behavior of the ASIC, and finally, specific image analysis is performed, according to the case.
The choice of threshold energy plays a critical role in optimizing the detector performance in view of its use in the field, as it influences two of the most prominent figure of merits, namely, DQE and count rate capability, in opposite ways. On the one hand, lower threshold values typically imply higher DQE values. This happens because at lower thresholds, a higher fraction of events is detected and, therefore, the “information content” of the image, of which DQE is a measure, is higher. Given that the dependence of DQE on the threshold is non-trivial, we relied on numerical simulations and took DQE (0) as a representative of the overall DQE behavior. On the other hand, higher threshold values imply higher count rate capabilities, the reason being that at higher thresholds, the detector dead time, corresponding to the time-over-threshold of the analog signal in the pixel front-end electronics, decreases, and therefore, the system paralysis occurs at higher incoming event rates. In this case, the dependence of the count rate capability on threshold energy is on a first-order approximation proportional.
Figure 4 shows the simulated DQE (0) as a function of threshold energy and for the incoming electron energies under study. Since the behavior at the several electron energies is significantly different, we identified optimal threshold values on an individual basis. A first constraint emerges from the observation that for thresholds more than half the incoming electron energy, all DQE (0) curves exhibit an abrupt drop, limiting the choice of the threshold below this limit. In this range, we can differentiate two cases.
i. DQE (0) is roughly constant for electron energies between 60 keV and 120 keV. Threshold values were then chosen as high as possible toward high count rate capabilities, which are only limited by the minimum allowed retrigger time (∼10 ns).
ii. DQE (0) mildly improves for decreasing thresholds for electron energies below 40 keV and above 160 keV. For simplicity and consistency, we limited the number of thresholds to one per electron energy, relying on a reasonable trade-off between DQE (0) and count rate performance.
FIGURE 4. Simulated DQE (0) values as a function of the threshold energy, for the incoming electron energies under study. The chosen threshold energy values are indicated with a symbol.
Table 1 reports the chosen values of threshold energy. These values are assumed throughout the rest of this study, unless otherwise indicated.
By sweeping the comparator threshold voltage, it is possible to measure the integral energy spectrum of detected radiations. By derivation, the differential energy spectrum is obtained. If the detected spectrum presents known and identifiable peaks, it is possible to create a correspondence between threshold voltage and incoming energy, the so-called energy calibration curve. Figure 5A shows the median5 differential energy spectra recorded for the several incoming electron energies, as a function of threshold energy. The energy calibration curve that was used to translate the threshold value from voltage, expressed in DAC units (DAC u.) to energy is shown in Figure 6, where black symbols corresponds to the position of the full-energy peaks, and the solid gray line to their fitting with an hyperbola function (which was found to nicely describe the comparator non-linear behavior), the blue line to the differential non-linearity (DNL), i.e., the fitting error and the red line to the obtain the computed result, as the derivative of the calibration curve. According to the energy spectra of Figure 5A, it is to be noted that up to the electron energy of 120 keV, the curves show distinct full-energy peaks, with a peak-to-background ratio in the range 3.9–8.9. Only for higher energies the charge sharing component becomes more significant, particularly for 200 keV electrons. This is due to the increased lateral spread of the electron energy deposition track in the silicon sensor which spans over multiple, neighboring pixels, with very low probability of having the full electron energy released in the volume pertaining to a single pixel. A more quantitative analysis is provided in Section 3.4.
FIGURE 5. Differential energy spectra measured for the several incoming electron energies as a function of the threshold energy (A). Fitting of the Mo fluorescence spectrum with the analytical pixel response model of [26] (B).
FIGURE 6. Energy calibration curve, with the corresponding comparator threshold voltages of the full-energy peaks shown in black symbols. The solid gray line represents the fitting with a hyperbola function, and blue symbols indicate the differential non-linearity (DNL), i.e., the fitting error and the solid red line the gain.
Using the analytical pixel response model described in [26] as a fitting function, it was possible to extract information regarding the charge cloud widening due to thermal diffusion when it reaches the collecting pixel and the pure electronic noise component of the front-end analog stage. We chose to fit the X-ray fluorescence spectrum of Mo, as it best suits the requirements of monochromaticity and point-like interactions required by the model. In addition, the Kβ peak was excluded from fitted data. The fitting result is shown in Figure 5B , together with the indication of Kα at 17.48 keV and of the less intense Kβ at 19.61 keV. The fitting parameters are a charge cloud size of 5.4 µm rms and an electronic noise of 568 eV rms. As a measure of the goodness-of-fit, we chose the normalized root mean square error (NRMSE), defined as follows:
where yi corresponds to the experimental data points and
Due to unavoidable microelectronic process variations, the effective value of the threshold voltage may vary across the pixel matrix. A careful threshold trimming, performed on a pixel-wise basis, is, therefore, necessary to assure an accurate and precise detector spectral response. We carried out threshold trimming at three energies, namely, the noise level, corresponding to approximately 6 keV with the used gain, the Sn Kα fluorescence peak at 25.27 keV, and the Gd Kα fluorescence peak at 42.99 keV. For the last two, we used the algorithm described [27]. Figure 7A shows the trimbit map computed at the noise level. No large-area variations were visible, and the threshold non-uniformity seems, therefore, to be dominated by random pixel-to-pixel fluctuations. Figure 7B shows the trimbit distributions for the three energies. No significant differences were visible, which means that the threshold fluctuations are dominated by a constant offset, rather than gain variations. In any case, the trimbit map at any desired threshold energy within the calibrated range is obtained with good approximation by linear interpolation between first neighbors.
FIGURE 7. Trimbit map computed at the noise level (∼6 keV) (A) and corresponding trimbit distribution plus the ones obtained using Sn and Gd fluorescence spectra (B).
The effectiveness of threshold trimming was evaluated by retrieving the statistical distribution of Sn Kα fluorescence peaks over the pixels ensemble before and after the application of the trimbit map. The result is shown in Figure 8. The untrimmed distribution has a standard deviation of 3.14 DAC u. rms, corresponding to 5.51 keV rms; the trimmed distribution has a standard deviation of 0.27 DAC u. rms, corresponding to 0.48 keV rms, improving by a factor 11.48.
FIGURE 8. Sn Kα fluorescence peak distributions computed over the pixels ensemble before and after the application of the trimbit map.
Residual non-uniformities in the detector response, known as fixed pattern noise that is mostly obtained from sensor inhomogeneities, are finally addressed and compensated by a flat-field correction.
The random nature of electron energy deposition tracks found in the sensor causes individual events to be recorded possibly over multiple, neighboring pixels. The number of firing pixels per event, or single-event multiplicity, is, therefore, described by its probability distribution function, denoted as Pm, which is, in general, also a function of the threshold energy. To measure such distributions, we acquired a series of images in a condition of extremely low electron flux illumination such that not only individual clusters are clearly distinguishable but also the probability of cluster overlapping is minimal. Since it is not possible to directly measure the fraction of events leading to 0 counts, we computed it indirectly from the experimental knowledge of the average single-event multiplicity obtained with the Faraday cup available in the microscope. Figure 9 shows the single-event multiplicity distributions measured for each of the incoming electron energy, with thresholds according to Table 1. For energies up to 100 keV, the behavior is very similar and strongly peaked at the cluster size value of 1. For increasing energies, the distributions shifts toward higher values as a consequence of increased lateral straggling relatively to the dimension of the pixel. To understand the dependence of the lateral straggling on the incoming electron energy, we can refer to the simulation results shown in Figure 10C, where we report the radius containing a certain fraction of the total deposited energy as a function of the electron energy, averaged over 1 million electron tracks. For example, the 95% of the total average deposited energy is achieved at a distance from the impinging point going from 6 um for 30 keV electrons up to 137 um for 200 keV electrons, in a superlinear manner. Figure 10A shows the corresponding top-view distribution of the average energy deposition for the specific case of 100 keV electrons. For completeness, Figure 10B shows the corresponding radial distribution across the sensor depth.
FIGURE 9. Single-event multiplicity distribution measured for the several incoming electron energies. Thresholds are according to Table 1.
FIGURE 10. (A,B) Simulated average energy deposition distributions for 100 keV electrons, impinging at the center of the coordinate system. (x, y) represent the directions of the pixel matrix, and z represents the direction along the sensor depth. (C) Simulated lateral straggling as a function of the electron energy intended as radius containing a certain fraction of the total average deposited energy. Corresponding values are also indicated in the plot in (C).
Table 2 shows the experimental mean values of the single-event multiplicity distributions, together with their simulated counterpart. The level of agreement is satisfactory for electron energies in the range 40 keV–120 keV, while the results deviate at lower and higher energies. At lower energies, we impute the discrepancy to the imperfect knowledge of the composition and thickness of the entrance window of the silicon sensor, which constitutes a dead layer. At higher energies, the simulation model starts to face limitations as it does not take into account the complex phenomena of signal induction by charge motion on the pixel matrix. The computation of induced signals at the pixels, in fact, does not take into account i) the possibility of having bipolar current signals induced on neighboring, non-collecting or partially collecting pixels [28], as predicted by Ramo’s theorem [29] and made observable by the fast shaping times of the front-end electronics; ii) ballistic deficits originating from the combined effect of differences in collection time due to the generation of charge at different depths in the sensor6 and again the fast shaping times.
The count rate curves were measured by illuminating the detector with an electron beam of constant intensity on a progressively decreasing area—obtained by focusing the beam through the condenser lenses of the microscopes—in order to increase the flux density per pixel. The incoming count rates were obtained from the knowledge of the size of the illuminated area and assuming a linear relationship between incoming and recorded rates at low beam intensities. Figure 11 shows the count rate curves for the several incoming beam energies acquired in the paralyzable counting mode, i.e., with the retrigger mechanism disabled. The curves exhibit the typical non-monotonic paralyzable behavior, with maximum recorded count rates ranging approximately from 30 Mcts/s/pix at 30 keV to 35 Mcts/s/pix at energies greater or equal than 100 keV.
FIGURE 11. Count rate curves in the paralyzable counting mode (retrigger disabled). Dashed lines between experimental points are to guide the eye. The ideal 1:1 curve is shown as a reference.
Figure 12 shows, in symbols, the count rate curves acquired in the non-paralyzable counting mode, i.e., with the retrigger mechanism enabled. The retrigger time τR, specific to each incoming energy, was set as short as possible without incurring in spurious double counts at low fluxes. The curves exhibit a characteristic monotonic, saturating non-paralyzable behavior. Saturation values—corresponding to 1/τR (Eq. 2)—range approximately from 60 Mcts/s/pix at 30 keV and 40 keV up to 105 Mcts/s/pix at 100 keV.
FIGURE 12. Count rate curves in the non-paralyzable counting mode (retrigger enabled). Experimental data are shown with symbols and the fittings with Eq. 2 in solid lines. The ideal 1:1 curve and the “cutoff” values are shown as a reference.
As a measure of the response linearity, it is typical to refer to the incoming rate yielding 10% recorded count rate loss. For the non-paralyzable counting mode, they range from 41.5 Mcts/s/pix at 30 keV to 84.3 Mcts/s/pix at 100 keV. Table 3 shows the full list of values for all the probed electron energies. Because of the availability of the Faraday cup in the microscope, it was possible to translate values of incoming count rates into incoming electron rates by measuring the absolute electron flux. The formerly reported values at 30 keV and 100 keV become 45.9 Mel/s/pix (7.3 pA/pix) and 98.0 Mel/s/pix (15.7 pA/pix), respectively.
The characteristic non-paralyzable counting mode provided by the retrigger capability was found to be analytically described with the relation as follows[30]:
where n is the incoming count rate, m is the recorded count rate, and τP is the effective pulse time width, which is related to the actual (non-rectangular) pulse shape. In order to achieve a better understanding of the timing properties of the ASIC, Eq. (2) was used as a fitting function on the experimental data, and the result is shown in Figure 12 in solid lines. The fitting parameters τP and τR are shown in Table 3. The values of τR are in the range 9.4 ns–15.4 ns, as expected from the saturation limit of count rate curves, while the values of τP lies in the range 10.5 ns–21.3 ns, always slightly larger than the corresponding τR as a consequence of the actual, non-rectangular pulse shape, as explained in [30].
The knowledge of the relation between incoming and recorded count rates allows for the correction of the recorded counts, thus extending the linear range of the system response. Due to intrinsic pixel-to-pixel and chip-to-chip variations, though, the rectification becomes inaccurate for increasing fluxes. From the experience obtained in the field, we arbitrarily defined an upper limit to this correction called count rate “cutoff,” as the value of the incoming rate corresponding to a slope of 0.2. The full list of cutoff values is also reported in Table 3, and they span from 86.8 Mcts/s/pix (88.8 Mel/s/pix, 14.2 pA/pix) at 40 keV to 149.4 Mcts/s/pix (142.0 Mel/s/pix, 22.7 pA/pix) at 120 keV.
Imaging properties were investigated in terms of MTF and DQE. The MTF was obtained using the standard slanted-edge technique; i.e., a highly absorbing edge was placed in front of the detector surface slightly tilted with respect to the pixel matrix orientation (∼3°) in order to allow for an oversampling factor of approximately 20. Direct outcomes of the measurement was the edge spread function (ESF), which was derived to obtain the line spread function (LSF), whose Fourier transform was the MTF. Data acquisition was performed under low-flux intensity condition to maintain the system in a linear regime of operations, and the images were flat-field corrected to eliminate the fixed pattern noise. Figure 13 shows the MTF at several incoming electron energies up to twice the Nyquist frequency of the system (fNy = 5 mm-1), the location of the first node of the cardinal sine corresponding to the MTF of the ideal 100-µm pixel size. The MTF is the highest for energies up to 80 keV, and then, it degrades progressively for increasing energies. This behavior is qualitatively coherent with the loss of spatial resolution as pointed out in the analysis of single-event multiplicities in Section 3.4.
FIGURE 13. MTF for the several incoming electron energies. The cardinal sine curve of the ideal 100-µm pixel with a perfect rectangular response is also shown as a reference.
DQE was obtained through the following relation [31]:
where NNPS corresponds to the normalized noise power spectrum and Q is the electron flux intensity. The NNPS was obtained by computing the average power spectral density on the series of images taken under uniform illumination, in a low-flux intensity condition, flat-field corrected, and normalized to the average recorded signal. Figure 14 shows the NNPS for the several electron energies multiplied by the scalar Q for normalization purposes. In this manner, the behavior of the ideal pixel matrix with uncorrelated (white) Poisson noise leads to a flat curve of value 1, also shown as a term of comparison. In reality, pixels exhibit noise correlation and its amount increases for increasing electron energies, as a consequence of the larger single-event multiplicity. The result is a “low-pass” filtering effect as clearly visible in the plot. Finally, Figure 15 shows the resulting DQE curves. DQE (0) are all grouped within the interval 0.75–0.82 except the one of 200 keV electrons which is significantly lower at 0.67. It can be useful to recall that DQE (0) can be written as a function of the first two statistical momenta of the single-event multiplicity distribution Pm as follows [32]:
which implies that “wider” single-event multiplicity distributions negatively affect DQE (0), as it happens in particular at 200 keV. Table 4 shows the full list of experimental DQE (0) as well as the corresponding values obtained through simulations. The results are in excellent agreement with a deviation ≤4% up to 160 keV, while a more substantial deviation is observed for the 200-keV case due to the same modeling inaccuracies, as described in Section 3.4. At higher spatial frequencies, DQE do not exhibit significant degradation, except for energies ≥160 keV where the loss in terms of MTF is dominating over the (beneficial) decrease in terms NNPS.
FIGURE 14. NNPS for the several incoming electron energies multiplied by Q for the normalization purposes. The constant curve of the ideal 100-µm pixel matrix, with uncorrelated noise (white noise), is also shown as a reference.
FIGURE 15. DQE for the several incoming electron energies. The squared cardinal sine curve of the ideal 100-µm pixel is also shown as a reference.
Electron radiation in the considered energy range poses no serious harm to the integrity of the sensor/readout chip assembly. On the one hand, the minimum energy needed for an incoming electron to create lattice damage in the silicon sensor is approximately 250 keV [33], above the upper boundary of expected usage. On the other hand, the 450-µm-thick silicon sensor constitutes a very effective radiation shielding layer with respect to the ASIC. Monte Carlo simulations have indeed shown that even in the worst case of an electron energy of 300 keV, the average dose delivered to the sensitive layer of ASIC electronics is only 157 RAD per 1010 primaries, particularly due to fluorescence and bremsstrahlung X-rays produced during the electron–semiconductor interaction. From experience with previous chips implemented with the same technology, the minimal dose needed to observe the first effects of radiation damage (i.e., threshold energy shifts) is approximately 0.1–1 MRAD.
We presented the performance assessment of the KITE counting ASIC bump-bonded to a 450-µm-thick silicon sensor with a pixel size of 100 µm for the incoming electron energies of 30 keV, 40 keV, 60 keV, 80 keV, 100 keV, 120 keV, 160 keV, and 200 keV. We investigated the spectral response, obtaining the energy calibration curve, and by fitting the spectrum of Mo Kα fluorescence with an analytical pixel response function, we estimated the electronic noise of the front-end electronics to 820 eV rms and a charge cloud size at the pixel side to 5.1 µm rms. We identified optimal threshold energy values as trade-off between DQE (0) and count rate capabilities and evaluated the impact of the threshold trimming algorithm on threshold dispersion, which improves by a factor 11.5 from 5.51 keV rms to 0.48 keV rms at the Sn Kα fluorescence peak. We characterized the single-event multiplicity distributions and the consequence of the random nature of electron energy deposition in the sensor. Their average values lies in the range 0.91–1.51 for increasing incoming electron energy. Count rate capabilities were evaluated in both paralyzable (retrigger mechanism disabled) and non-paralyzable counting modes (retrigger mechanism enabled). In the latter case, the notable point of 10% count rate linearity loss occurs from a minimum of 41.5 Mcts/s/pix (45.9 Mel/s/pix, 7.3 pA/pix) at 30 keV to a maximum of 84.3 Mcts/s/pix (98.0 Mel/s/pix, 15.7 pA/pix) at 100 keV. Count rate “cutoff” values, upper limit for a reliable response rectification, span from a minimum of 86.8 Mcts/s/pix (88.8 Mel/s/pix, 14.2 pA/pix) at 40 keV to a maximum of 149.4 Mcts/s/pix (142.0 Mel/s/pix, 22.7 pA/pix) at 120 keV. The imaging performances were qualified in terms of MTF and DQE. DQE (0) lies in the range 0.75–0.82 at all electron energies except 200 keV, where it decreases to 0.67. At higher spatial frequencies of only above 160 keV, the DQE suffers some degradation due to the impact of longer electron tracks.
The raw data supporting the conclusion of this article will be made available by the authors, without undue reservation.
PZ: Conceptualization, Data curation, Formal analysis, Investigation, Methodology, Visualization, Writing–original draft, and Writing–review and editing. JV: Writing–review and editing and Writing–original draft. GM: Methodology, Software, and Writing–review and editing. SB: Resources and Writing–review and editing. AD: Resources and Writing–review and editing. RS: Resources and Writing–review and editing. CH: Resources and Writing–review and editing. MM: Resources and Writing–review and editing. CS-B: Resources and Writing–review and editing. DS: Resources and Writing–review and editing. NL: Writing–reviewing. LP: Resources and Writing–review and editing.
The authors declare that no financial support was received for the research, authorship, and/or publication of this article.
The authors would like to thank V. Radicci and V. Boccone for their help with the Monte Carlo simulations.
PZ, JV, GM, SB, AD, RS, CH, MM, CS-B, DS, NL, and LP were employed by DECTRIS Ltd.
All claims expressed in this article are solely those of the authors and do not necessarily represent those of their affiliated organizations, or those of the publisher, the editors, and the reviewers. Any product that may be evaluated in this article, or claim that may be made by its manufacturer, is not guaranteed or endorsed by the publisher.
1The reciprocal space is an abstract space where basis vectors are expressed in terms of frequency instead of distance. It is a convenient mathematical tool to visualize the result of the Fourier transform of spatial functions, e.g., diffraction patterns.
2The dwell time corresponds to the time the beam spends on each pixel in the scanning pattern.
3Continuous readout operation is achieved by reading a counter while the other is being written in an alternated manner. Switching between counters has negligible time overhead.
4v. 4–2.1. The physics was set to multiple Coulomb scattering with the cutoff energy of 1 keV for electrons and 100 eV for photons. Fluorescence was enabled, and no biasing was used.
5The median was performed over the whole pixel matrix.
6Collection time differences for 200 keV electrons can be up to 5–6 ns, over a total collection time in the order of 15 ns.
1. Erni R, Rossell MD, Kisielowski C, Dahmen U. Atomic-resolution imaging with a sub-50-pm electron probe. Phys Rev Lett (2009) 102:096101. doi:10.1103/PhysRevLett.102.096101
2. Ophus C. Four-Dimensional scanning transmission electron microscopy (4D-STEM): from scanning nanodiffraction to ptychography and beyond. Microsc Microanalysis (2019) 25:563–82. doi:10.1017/s1431927619000497
3. MacLaren I, Macgregor TA, Allen CS, Kirkland AI. Detectors—the ongoing revolution in scanning transmission electron microscopy and why this important to material characterization. APL Mater (2020) 8:110901. doi:10.1063/5.0026992
4. Batson PE, Dellby N, Krivanek OL. Sub-ångstrom resolution using aberration corrected electron optics. Nature (2002) 418:617–20. doi:10.1038/nature00972
5. Haider M, Uhlemann S, Schwan E, Rose H, Kabius B, Urban K. Electron microscopy image enhanced. Nature (1998) 392:768–9. doi:10.1038/33823
6. Shibata N, Seki T, Sánchez-Santolino G, Findlay SD, Kohno Y, Matsumoto T, et al. Electric field imaging of single atoms. Nat Commun (2017) 8:15631. Article number: 15631. doi:10.1038/ncomms15631
7. Campanini M, Erni R, Yang C-H, Ramesh R, Rossell MD. Periodic giant polarization gradients in doped BiFeO3 thin films. Nano Lett (2018) 18(2):717–24. doi:10.1021/acs.nanolett.7b03817
8. Sánchez-Santolino G, Lugg NR, Seki T, Ishikawa R, Findlay SD, Kohno Y, et al. Probing the internal atomic charge density distributions in real space. ACS Nano (2018) 12(9):8875–81. doi:10.1021/acsnano.8b03712
9. Lozano JG, Martinez GT, Jin L, Nellist PD, Bruce PG. Low-dose aberration-free imaging of Li-rich cathode materials at various States of charge using electron ptychography. Nano Lett (2018) 18(11):6850–5. doi:10.1021/acs.nanolett.8b02718
10. Campanini M, Gradauskaite E, Trassin M, Yi D, Yu P, Ramesh R, et al. Imaging and quantification of charged domain walls in BiFeO3. Nanoscale (2020) 16:9186–93. doi:10.1039/D0NR01258K
11. Shibata N, Findlay SD, Kohno Y, Sawada H, Kondo Y, Ikuhara Y. Differential phase-contrast microscopy at atomic resolution. Nat Phys (2012) 8:611–5. doi:10.1038/nphys2337
12. Ahmed S, Bianchini M, Pokle A, Munde MS, Hartmann P, Brezesinski T, et al. Visualization of light elements using 4D STEM: the layered-to-rock salt phase transition in LiNiO2 cathode material. Adv Energ Mater (2020) 10:25:2001026. doi:10.1002/aenm.202001026
13. Wen Y, Ophus C, Allen CS, Fang S, Chen J, Kaxiras E, et al. Simultaneous identification of low and high atomic number atoms in monolayer 2D materials using 4D scanning transmission electron microscopy. Nano Lett (2019) 19(9):6482–91. doi:10.1021/acs.nanolett.9b02717
14. Zachman MJ, Madsen J, Zhang X, Ajayan PM, Susi T, Chi M. Interferometric 4D-STEM for lattice distortion and interlayer spacing measurements of bilayer and trilayer 2D materials. Micro Nano Small (2021) 17(28):2100388. doi:10.1002/smll.202100388
15. Gao W, Addiego C, Wang H, Yan X, Hou Y, Ji D, et al. Real-space charge-density imaging with sub-ångström resolution by four-dimensional electron microscopy. Nature (2019) 575:480–4. doi:10.1038/s41586-019-1649-6
16. Wu L, Meng Q, Zhu Y. Mapping valence electron distributions with multipole density formalism using 4D-STEM. Ultramicroscopy (2020) 219:113095. Epub 2020 Aug 26. PMID: 32905856. doi:10.1016/j.ultramic.2020.113095
17. Yang H, Rutte R, Jones L, Simson M, Sagawa R, Ryll H, et al. Simultaneous atomic-resolution electron ptychography and Z-contrast imaging of light and heavy elements in complex nanostructures. Nat Commun (2016) 7:12532. doi:10.1038/ncomms12532
18. Jiang Y, Chen Z, Han Y, Deb P, Gao H, Xie S, et al. Electron ptychography of 2D materials to deep sub-ångström resolution. Nature (2018) 559:343–9. doi:10.1038/s41586-018-0298-5
19. Zambon P, Bottinelli S, Schnyder R, Musarra D, Boye D, Dudina A, et al. KITE: high frame rate, high count rate pixelated electron counting ASIC for 4D STEM applications featuring high-Z sensor. Nucl Inst. Methods Phys Res A (2023) 1048:167888. doi:10.1016/j.nima.2022.167888
20. Loeliger T, Broennimann C, Donath T, Schneebeli M, Schnyder R, Trueb P. The new PILATUS3 ASIC with instant retrigger capability. In: 2012 IEEE nuclear science symposium and medical imaging conference record (NSS/MIC) N6-2 (2012). p. 610–5.
21. Plotkin-Swing B, Haas B, Mittelberger A, Dellby N, Hotz M, Hrncirik P, et al. 100,000 diffraction patterns per second with live processing for 4D-STEM, microsc. Microanal (2022) 28(1):422–4. doi:10.1017/S1431927622002392
22. Stroppa DG, Meffert M, Hoermann C, Zambon P, Bachevskaya D, Remigy H, et al. From STEM to 4D STEM: ultrafast diffraction mapping with a hybrid-pixel detector. Microsc Today (2023) 31(2):10–4. doi:10.1093/mictod/qaad005
23. Anelli G, Campbell M, Delmastro M, Faccio F, Floria S, Giraldo A, et al. Radiation tolerant VLSI circuits in standard deep submicron CMOS technologies for the LHC experiments: practical design aspects. IEEE Trans Nucl Sci (1999) 46(6):1690–6. doi:10.1109/23.819140
24. Böhlen T, Cerutti F, Chin M, Fassò A, Ferrari A, Ortega P, et al. The FLUKA code: developments and challenges for high energy and medical applications. Nucl Data Sheets (2014) 120:211–4. doi:10.1016/j.nds.2014.07.049
25. Ferrari A, Sala PR, Fasso A, Ranft J. FLUKA: a multi-particle transport code, program version (2005). [INFN-TC-05-11].
26. Zambon P, Radicci V, Rissi M, Broennimann C. A fitting model of the pixel response to monochromatic X-rays in photon counting detectors. Nucl Inst. Methods Phys Res A (2018) 905:188–92. doi:10.1016/j.nima.2018.07.069
27. Zambon P, Trueb P, Rissi M, Broennimann C. A wide energy range calibration algorithm for X-ray photon counting pixel detectors using high-Z sensor material. Nucl Inst. Methods Phys Res A (2019) 925:164–71. doi:10.1016/j.nima.2019.01.095
28. Castoldi A, Guazzoni C, Parsani T, Riccio F, Zambon P. Laser mapping of the inter-strip response in double sided silicon strip detectors for particle identification. J Instrum (2015) 10:C01017. doi:10.1088/1748-0221/10/01/c01017
29. Ramo S. Current induced by electron motion. Proc I.R.E (1939) 27(9):584–5. doi:10.1109/JRPROC.1939.228757
30. Zambon P. Dead time model for X-ray photon counting detectors with retrigger capability. Nucl Inst. Methods Phys Res A (2021) 994:165087. doi:10.1016/j.nima.2021.165087
31. Bath M. Evaluating imaging systems: practical applications. Radiat Prot Dosimetry (2010) 139:26–36. doi:10.1093/rpd/ncq007
32. McMullan G, Cattermole DM, Chen S, Henderson R, Llopart X, Summerfield C, et al. Electron imaging with Medipix2 hybrid pixel detector. Ultramicroscopy (2007) 107:401–13. doi:10.1016/j.ultramic.2006.10.005
Keywords: 4D STEM, electron counting detector, high frame rate, high count rate, KITE ASIC, hybrid pixel
Citation: Zambon P, Vávra J, Montemurro G, Bottinelli S, Dudina A, Schnyder R, Hörmann C, Meffert M, Schulze-Briese C, Stroppa D, Lehmann N and Piazza L (2023) High-frame rate and high-count rate hybrid pixel detector for 4D STEM applications. Front. Phys. 11:1308321. doi: 10.3389/fphy.2023.1308321
Received: 06 October 2023; Accepted: 30 November 2023;
Published: 19 December 2023.
Edited by:
Lucio Pancheri, University of Trento, ItalyReviewed by:
Markus Kuster, European X-Ray Free Electron Laser, GermanyCopyright © 2023 Zambon, Vávra, Montemurro, Bottinelli, Dudina, Schnyder, Hörmann, Meffert, Schulze-Briese, Stroppa, Lehmann and Piazza. This is an open-access article distributed under the terms of the Creative Commons Attribution License (CC BY). The use, distribution or reproduction in other forums is permitted, provided the original author(s) and the copyright owner(s) are credited and that the original publication in this journal is cited, in accordance with accepted academic practice. No use, distribution or reproduction is permitted which does not comply with these terms.
*Correspondence: P. Zambon, pietro.zambon@dectris.com
Disclaimer: All claims expressed in this article are solely those of the authors and do not necessarily represent those of their affiliated organizations, or those of the publisher, the editors and the reviewers. Any product that may be evaluated in this article or claim that may be made by its manufacturer is not guaranteed or endorsed by the publisher.
Research integrity at Frontiers
Learn more about the work of our research integrity team to safeguard the quality of each article we publish.