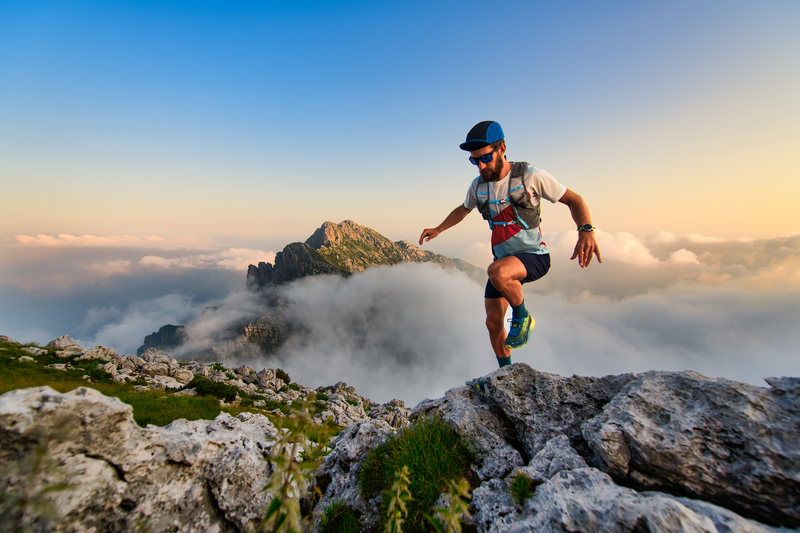
94% of researchers rate our articles as excellent or good
Learn more about the work of our research integrity team to safeguard the quality of each article we publish.
Find out more
ORIGINAL RESEARCH article
Front. Phys. , 31 October 2023
Sec. Social Physics
Volume 11 - 2023 | https://doi.org/10.3389/fphy.2023.1293177
This article is part of the Research Topic Compartmental Models for Social Interactions View all 6 articles
The popularity of online social networks (OSNs) promotes the co-propagation of multiple types of information. And there exist inevitably competitive interactions between these information, which will significantly affect the spreading trend of each information. Besides, the coupled topology of multi-layer interconnects exhibited in OSNs will also increase the research complexity of information propagation dynamics. To effectively address these challenges, we propose a novel competitive information propagation model on multi-layer interconnected networks, where the tendency of an individual to become a positive or negative spreader depends on the weighted consideration of local and global prevalence. Then the basic reproduction number is calculated via next-generation matrix method. And under the critical conditions of the basic reproduction number, the asymptotic stability of information-free and information-endemic equilibria is theoretically proven through Lyapunov stability theory. Besides, an optimal control problem involving two heterogeneous controls is formulated, aiming at achieving the best suppression performance of negative information with the minimum control cost. According to Cesari theorem and Pontryagin minimum principle, the existence and analytical formulation of optimal solutions are derived. Extensive numerical experiments are conducted to prove the correctness of our theoretical results, and evaluate the effectiveness of our proposed control strategies. This study can provide useful insights into the modeling and control of multiple information propagation considering multi-layer network topology and individual adaptive behavior.
With the continuous development of modern technology, online social networks (OSNs) have gradually become essential mediums for people to exchange opinions, which contributes to large-scale information propagation in different forms including rumor, awareness, influence and so on. It is well-known that the ubiquitous information propagation will significantly affect human daily life in turn. In particular, the spread of positive influence involving a certain city will help it absorb more talents and accelerate its productivity [1–3]. The awareness propagation about COVID-19 protection may save many human lives and reduce unnecessary economic losses [4–7]. And the rumor spreading about side effects of vaccine therapy can decrease the vaccination rates and hinder the effectiveness of disease interventions [8–10]. Therefore, exploring the dynamical process of information propagation is of great practical significance and has attracted much attention from the academic community. A large number of related work has followed on the mathematical modeling of information propagation dynamics, employing classical SIS, SIR and other derivative models. For example, Yu et al. [11] established an ignorance-discussant-spreader-remover rumor spreading model, and analyzed the local asymptotic stability of four equilibria. Yin et al. [12] took into account the emotion categories and choices of user communities on OSNs, and constructed an emotion-based susceptible-forwarding-immune propagation dynamic model to investigate the evolution process of emotional information and guide public sentiment. Zhu et al. [13] proposed a novel susceptible-latent-breaking-recovered propagation model to explore the dynamic behavior of industrial viruses in the SCADA system.
However, a considerable amount of previous work assumes that only single information propagates on OSNs, which is far beyond the practical case. In view of the individuals’ differences including nationality, ethnicity, education and personality, their opinions towards a specific event is generally diverged into two opposite sides, which can be considered as positive information and negative information. Typically, different groups of people always have different evaluations on a city, where local residents tend to prefer good evaluations, while nonresidents may make bad evaluations due to their terrible experiences. Then good evaluations promote the spread of positive influences, and bad evaluations lead to the spread of negative influences [14–16]. The effectiveness of COVID-19 prevention measures, such as vaccination and physical distancing, also simultaneously spreads through the crowd in dual forms of rumor and truth [17–21]. In particular, the government will disclose the truth about the effectiveness of prevention measures, and the supporters may actively promote the spread of truth. However, some opponents inevitably worry about the side effects of prevention measures and spread rumors. More attention should be paid to the spreading process of competitive information, so that the interaction between multiple information can more truly reflect the complexity of information propagation on OSNs. On the other hand, OSNs also form a multi-layer structure due to the clustering nature of the population, and the coupling between any layers will significantly affect the dynamic trend and steady-state prevalence of information propagation compared to single-layer networks. Antonopoulos et al. [22] studied the opinion dynamics over multiplex networks with heterogeneous confidence thresholds and general initial opinion distributions. Through probability analysis, they derived the analytical expressions for the critical thresholds of confidence bound considering certain regularity conditions of the networks, which reveals the significant impacts of structural multiplexity and initial distribution on the critical thresholds. Furthermore, their numerical simulations also explored the consensus behavior of agents in diverse network topologies. Yin et al. [23] constructed an environment-based susceptible-forwarding-immune model of information propagation on multi-platform networks, which reflects the complexity of actual social network topologies and the interaction between various social platforms. They also parameterized the model using COVID-19 data, and conducted the parameter sensitivity analysis. Wang et al. [24] investigated the multi-lingual SIR dynamic process of rumor spreading, which regarded each language community as a layer, and establish the rumor cross-transmitted mechanism in different lingual environments. Both the basic reproduction number and the stability of the rumor-free/endemic equilibrium were systematically discussed. These studies reveal many new characteristics how multi-layer network topologies intervene information propagation trend. However, although lots of work has separately investigated the potential effects of multiple information coexistence and multi-layer network topology, the attempts to synthesize the two aspects may reveal more interesting conclusions, which have not been well explored. Therefore, in order to more comprehensively explore the information propagation dynamics on OSNs, an improved model taking both the multiple information interaction and multi-layer interconnected networks needs to be further developed and analyzed.
Actually, individuals in OSNs are not only the carriers of information propagation, but also take certain adaptive behaviors according to the information prevalence. The herd behavior that individuals tend to follow others when making decisions is one of the most common social behaviors, and has recently attracted substantial attention from the research community [25–28]. However, to the best of our knowledge, there is few work to introduce individual herd behavior into the multiple information propagation on multi-layer networks, where herd behavior may change the mechanism of state transition and thus determine the dominance relationship between multiple information. Under the framework of multiple information propagation, each individual may consider both the local situation of its neighbors and the global situation of all individuals before deciding which type of information to spread. This adaptive herd behavior will affect the coupling characteristics between multiple information, and interfere with the evolution trend and prevalence of various information. Therefore, the consideration of individual herd decision-making behavior will also improve our understanding of information propagation dynamics.
One of the primary purposes for modeling information propagation is to control the spreading processes of interest. There are two main streams of control strategies, namely, heuristic control and optimal control [29–33]. Heuristic control strategy employs the real-time information prevalence to construct adaptive control function, aiming to effectively suppress/promote the specific information spreading, while optimal control strategy constructs the adaptive control function to maximize/minimize a well-designed objective function. Lin et al. [34] established a novel SWIR model with uncertain mental state to describe the spreading process of fraudulent information, and further formulated an optimal problem of two synergistic control strategies to minimize the total cost constraint involving individual losses and control resource consumption. Li et al. [35] proposed a multifactor-based information model considering information content and node characteristics, and also constructed an efficient heuristic algorithm of seed nodes selection to solve the targeted influence maximization problem. Wang et al. [36] proposed a novel computational model to present the temporal dynamics of the positive and negative information spread, and devised a nonlinear feedback control mechanism to perform three synergetic intervention strategies with minimal system expenses. During multiple information propagation, our control strategies are aimed at promoting the diffusion of positive information and suppressing the outbreak of negative information. Due to the resource limitations in reality, decision-makers always attempt to achieve the most powerful suppress performance of negative information with the lowest control intensity, which forms a trade-off between control cost and propagation cost. This trade-off can be formulated as an optimization problem, and the corresponding optimal solutions need to be further discussed, which can provide theoretical guidance and reference significance for practical resource scheduling.
Motivated by the above analysis, we aim to develop a novel framework that can integrate competitive information propagation, multi-layer interconnected networks and individual adaptive behavior. Our main contributions are summarized as follows.
• We propose a novel competitive information propagation model on multi-layer interconnected networks. This model can not only efficiently describe the competing phenomenon of positive and negative information coexistence in the real world, but also analyze the correlation between information propagation and multi-layer topology in OSNs;
• We construct a novel compartmental model with individual herd behavior towards information propagation, where the individual’s inclination to be a positive or negative spreader depends on a weighted balance between global and local information prevalence. This consideration reflects the coupled characteristics of individual response and information propagation, that is, individuals generally take adaptive behavior towards the received information, and this behavior may further affect the trend of information propagation;
• We analytically deduce the basic reproduction number
• We design two heterogeneous controls to suppress the prevalence of negative information. Considering the cost constraints in reality, we construct an optimization problem involving a trade-off between the negative propagation cost and the control cost. To solve the optimization problem, we further discuss the existence, the analytic formulation and the uniqueness of the optimal solution.
The rest of this paper is organized as follows. In Section 2, a competitive information propagation model is formulated within the framework of multi-layer interconnected network. Stability analysis of information-free and information-endemic equilibria is carried out in Section 3. The formulation of optimal control problem is conducted to discuss the existence, analytic formulation and uniqueness of the optimal solution in Section 4. Extensive numerical simulations are conducted to verify our presented theoretical results in Section 5. Some conclusions are drawn in Section 6.
In this section, we formulate a spreading model SIAIBHR of competitive negative and positive information on multi-layer interconnected networks.
For simplicity, we here consider a two-layer interconnected network and denote them as layers 1 and 2 respectively, whose relevant conclusions can be easily generalized to the other cases of multi-layer networks with more layers. These two layers can be depicted as different countries, cities or communities in the real world. The individuals on two respective layers belong to different groups. Each individual has both the neighbors within the same layer and the acquaintances within other layer, which contributes to the intra-layer and inter-layer edges. Then denote the intra-layer average degree of the layer 1 and 2 as
FIGURE 1. The schematic diagram of (A) multi-layer interconnected networks and (B) state transition mechanism.
During the spreading dynamics SIAIBHR, all individuals are divided into five status compartments: Susceptible (S), Infected by positive information (IA), Infected by negative information (IB), Hesitate (H) and Recovered (R). Specifically, S state represents an individual who is unknown to any type of information but potentially infected via its intra-layer or inter-layer edges; IA state represents a spreader who is infected with positive information; IB state represents a spreader who is infected with negative information; H state represents an individual who has received two types of information but is hesitant to become which one; R state represents an individual who has no interest in spreading either positive or negative information. We further define the state density of S, IA, IB, H, R in layer 1 and 2 at time t as S1(t), S2(t),
Considering that the dynamic process of information propagation has the same time scale as the evolution of involved population numbers, we introduce the birth and mortality rates of population dynamics. And the birth rate and mortality rate of our dynamics are presented as ξ and δ respectively. Denote
The birth rate will increase the quantitative basis of susceptible individuals, which can potentially raise the infection risk in the population and promote the information prevalence. And the opposite condition holds for the mortality rate. The relative size of birth and mortality rates determines the evolutionary trend of population numbers. In real life, both birth and mortality rates have two meanings: 1) For the birth rate, on the one hand, it refers to natural birth rate, which means that the initial population can give birth to newborns and these newborns can participate in the information propagation; on the other hand, it also refers to immigrated birth rate, which means that the external population may move to the regions where the initial population is located due to work, education and tourism. After their immigration, they can also participate in the information propagation. 2) For mortality rate, on the one hand, it refers to natural mortality rate, which means that some individuals in the initial population will die due to accidents and old age, and these deaths may be excluded for the information propagation; on the other hand, it also refers to emigration mortality rate, which means that some initial individuals may move to other regions for diverse reasons, and their emigration makes them no longer involved in the information propagation. Taking the propagation process in layer 1 as an example, the state transition mechanism of SIAIBHR model can be described as.
• Some newborn individuals will be filled into the network with birth rate ξ to participate in the information propagation process. Without loss of generality, these newborn individuals are assumed to be susceptible; Individuals in all five compartments are removed from the network with mortality rate δ;
• Individuals in S status may become infectious spreader of positive/negative information associating with their intra-layer infected neighbors in
• Individuals in
• Individuals in H status may tend to be the spreaders of positive (negative) information with transmission rate
• Individuals in
The dynamic evolution of information propagation for layers 1 and 2 can be derived as
where
For Eqs 2.4, 2.5, the local information prevalence is equivalent to the density of information spreaders in the layer where individuals reside, while the global information prevalence is defined as the average density of information spreaders in two layers. ω1 and ω2 are the weighted coefficients of local prevalence of negative/positive information in layer 1 and 2. β1, β2 are the scaling factors. Without loss of generality, we set β1 = β2 = 1 here.
In this section, we firstly study the basic reproduction number of dynamical systems (2.2) and (2.3) through the next-generation matrix method [37]. Secondly, the existence and stability of information-free equilibrium and information-endemic equilibrium are discussed by means of analysis technique, Routh-Hurwitz judgment, Lyapunov method and LaSalle’s invariance principle.
In order to facilitate the calculation of the basic reproduction number, denote
Then we can rewrite the dynamical systems (2.2) and (2.3) as
where
Obviously, there exists an information-free equilibrium
where
Therefore, the next-generation matrix can be calculated as
The basic reproduction number
Combined the above analysis, the stability properties of the information-free equilibrium E0 and information-endemic equilibrium E* are given in the following theorems.
With
Theorem 3.1. For the dynamical systems (2.2) and (2.3), the information-free equilibrium E0 is locally asymptotically stable if
Proof: The local stability of the information-free equilibrium E0 of the dynamical systems (2.2) and (2.3) is determined by the eigenvalues of the corresponding Jacobian matrix
Then the eigenvalues of
Furthermore, we can derive the maximum eigenvalue
Thus, it is obvious that as long as
Next, we investigate the global stability of the information-free equilibrium E0.
Theorem 3.2. The information-free equilibrium E0 of the dynamical systems (2.2) and (2.3) is globally asymptotically stable if
Proof: Construct the following Lyapunov function
where
The derivative of V(t) towards t can be calculated as
Significantly,
When
with the similar condition holding for system (2.3).
Theorem 3.3. The information-endemic equilibrium E* of the dynamical systems (2.2) and (2.3) is globally asymptotically stable if
Proof: Construct the following Lyapunov function
for the dynamical system (2.2) in layer 1, we define
where g(x) = x − 1 − ln x ≥ g (1) = 0, and
Furthermore, we define
Then, we can obtain
The derivative of V1(t) towards t can be calculated as
where
For convenience, we define
Therefore, combining Eq. 3.15 and Eqs 3.20—3.23, we can derive
Next, by taking c1 ≥ c2 = c3 = c4 > 0, we have
Besides,
In conclusion, we have completed the proof of asymptotic stability for information-free and information-endemic equilibria. Note that the Lyapunov theory we employ is not the unique method to prove system stability. As an alternative, Shang [38] proposed a novel framework that can analytically obtain the explicit solutions of dynamical systems using Lie algebra methods. Once the explicit solutions can be available, an effort may be made to derive the limit values of these explicit solutions when t → ∞. And the convergence of the limit values can reflect the stability of dynamical systems.
In order to suppress the prevalence of negative information, we introduce two heterogeneous controls: one is direct control mode v1(t), which persuades negative information spreaders to become positive information spreaders; the other is indirect control mode v2(t), which guides hesitant individuals to become positive information spreaders. Define QC as the Lebesgue square integrable control set of all admissible values of v1(t) and v2(t) over time interval
where the first term corresponds to the infection cost of negative information propagation in the network, and the second and third terms are the costs brought by the implementation of controls. Our goal is to achieve the most powerful inhibition effect on negative information with the lowest control intensity, which forms a trade-off between the two kinds of costs. d1, d2 and d3 are the weight factors of the three costs.
Correspondingly, the Lagrangian of our optimal problem can be formed as
then define adjoint functions
Theorem 4.1. There exists a set of optimal controls
Proof: The existence of optimal control strategies can be proven by the Cesari theorem [39]. Cesari theorem indicates that if the optimization problem for our dynamical systems (2.2) and (2.3) satisfies the following five conditions, the optimal solutions of two controls v1(t) and v2(t) will exist.
(1) The control set and state variables are non-empty;
(2) The control set is closed and convex;
(3) The right-hand side of systems (2.2) and (2.3) is bounded above by a linear function with the state and control;
(4) The integrand in the objective function is convex with respect to the controls v1(t), v2(t);
(5) There exists a constant C1 > 1 and positive number C2 and C3 such that
Next, we will prove that the dynamical systems (2.2) and (2.3) satisfy the above five conditions. Obviously, if the system is uniformly Lipschitz continuous, the sets of QC and solutions to initial values are non-empty. Besides, state variables are non-empty such that condition (1) is satisfied. The characteristics of control space and objective function can be verified by definition, meeting conditions (2) and (4).
The systems (2.2) and (2.3) can be rewritten as
where
Furthermore, we can verify
where
Then we have
where
Therefore, the function D is uniformly Lipschitz continuous and satisfies the required bound in Cesari theorem. The control set QC belongs to the closed set
Furthermore, we set C1 = 2,
Here we employ Pontryagin minimum principle with fixed final time to analytically derive the optimal solution.
Theorem 4.2. Given an optimal control pair v1(t) and v2(t) and the corresponding solutions to the system (2.2) and (2.3), there exist adjoint functions φ1(t), φ2(t), φ3(t), φ4(t), φ5(t), φ6(t), φ7(t), φ8(t), satisfying
with boundary conditions
Furthermore, the optimal solutions of
Proof: While characterizing the properties of the optimal solution, the costate conditions must be satisfied in the system of costate differential equations as
Then, by the optimal conditions, we have
Let
Considering the property of the control space, we obtain
Thus we have the optimal controls
In summary, the proof of theorem 4.2 has been completed.
Theorem 4.3. For a relatively short control time T, the states and the adjoint functions at the optimum and the optimal control strategies are unique.
Proof: Considering two different control states and their corresponding adjoint functions as
Then we define S
1 = e
αt
p
1,
After substituting the above values into systems (2.2) and (2.3), we can obtain
Similarly, we can obtain other equations for
Furthermore,
Combining all of these equations, we can obtain
where
If α is chosen such that
In this section, we perform extensive numerical simulations to 1) corroborate the theoretical results of stability analysis towards information-free and information-endemic equilibria; 2) explore the influence of spreading parameters on dynamic trend and steady-state prevalence; 3) discuss the competition between two types of information; and 4) investigate the effectiveness of our optimal control strategies.
In order to verify the correctness of our theoretical modeling in characterize the competitive information propagation process on multi-layer interconnected networks, we first conduct the time evolution of each status according to Eqs 2.2, 2.3 and Monte Carlo (MC) simulations under different cases of spreading parameters. As shown in Figure 2, the MC simulation results are observed to match well with the theoretical results, which indicates that our formulations can accurately reflect the competitive spreading mechanism. Furthermore, we can calculate the basic reproduction number
FIGURE 2. Time evolution of each state density via several combinations cases. The symbols correspond to Monte Carlo (MC) simulations and the dotted lines represent the theoretical modeling of dynamic equation (ODE). The shared parameter settings are
It is well-acknowledged that spreading parameters are the key factors affecting the steady-state prevalence of information propagation, so it is necessary to explore the correlation between some important spreading parameters and the information prevalence. Note that the dynamic process of information in layer 1 is influenced by three aspects: 1) the interaction between individuals in layer 1 via intra-layer edges presented as
• Figure 3A describes the changing trend of steady-state density of
• Figure 3B illustrates how
• Figure 3C depicts the changing trend of
• Figure 3D provides the changing trend of steady-state
• Figure 3E shows the changing trend of steady-state
FIGURE 3. Phase diagram of correlation between spreading parameters and system steady-state characteristics. (A)
In order to further verify the correctness of our Theorems 3.1–3.3 on the stability analysis of information-free and information-endemic equilibria, we construct the evolution trajectory of infection states
FIGURE 4. The evolution trajectory of infection states
Furthermore, we explore the effects of weights ω
1 and ω
2 on the dynamical trend of positive and negative information. It is remarkable that larger ω
1 and ω
2 indicate that hesitant individuals consider the local information prevalence when deciding which information to believe, while smaller ω
1 and ω
2 imply that hesitant individuals consider the global information prevalence more. Figure 5 shows the evolution of
FIGURE 5. The infection states
To assess the performance of the proposed control mechanism, we introduce the following five control strategies, including our optimal control and other four baselines.
• No control: The system evolves naturally without implementing any control, namely, v 1(t) = 0, v 2(t) = 0;
• Constant control 1: The controls are time invariant, namely, v 1(t) = 0.15, v 2(t) = 0.15;
• Constant control 2: The controls are time invariant, namely, v 1(t) = 0.25, v 2(t) = 0.25;
• Heuristic control: The controls are referred as feedback mechanism based on
• Optimal control: The controls follow Eqs 4.11—4.21.
Figure 6 illustrates the comparative results of cumulative costs under five strategies. As can be observed from Figure 6A, both the optimal and heuristic controls achieve the lower total cost in the control interval, while the total costs with no control and two constant controls are relatively high. Under no control strategy, even without the introduction of control cost, the corresponding infection cost will be very high because the current information prevalence is the largest. For the two constant controls, they merely focus on the control performance without any cost constraints. Therefore, although constant controls have significant suppression effect on the diffusion of negative information, their larger control intensity leads to the higher control costs. The results in Figures 6B, C also confirm these explanations. As the heuristic control strategy is a feedback mechanism to the time-varying infection prevalence, it can still inhibit the prevalence of negative information to a certain extent, but the control performance is worse than constant controls and optimal control. Another interesting phenomenon is that although our optimal control strategy can significantly inhibit the spread of negative information, the control intensity of the two heterogeneous costs is quite different. From Figures 6C,D, the intervention effect on the spreaders of negative information is significantly stronger than that on the hesitant individuals, which may be because hesitate status has no infectivity and does not significantly promote the spread of negative information. This also indicates that in order to achieve the ideal control performance, decision-makers should attach more importance on the direct restrictions towards the sources of negative information, instead of indirect control with little benefits towards hesitate individuals.
FIGURE 6. The total cost, infection cost, control cost1 and control cost2 under five different control strategies. (A) time v.s. total cost; (B) time v.s. infection cost; (C) time v.s. control
Figure 7 presents the time-varying trend comparison of positive/negative information prevalence
FIGURE 7. The infection states
In this paper, we have investigated the propagation dynamics of competitive information on multi-layer interconnected networks. On the one hand, we explored the correlation between information propagation and multi-layer network topology. On the other hand, we introduced individual adaptive behavior towards information propagation, i.e., the tendency of an infected individual to become a spreader of positive or negative information depends on the weighted consideration of local and global information prevalence. Under such propagation mechanism, the basic reproduction number
We provide two possible directions in the future: 1) our model assumes that the spreading parameters and the inter-layer average degree are time-invariant. However, in real life, information propagation is a rather complicated process associated with numerous social and cognitive psychology factors. Therefore, our model will be improved if the spreading parameters and topology settings are described as time-dependent variables driven by more social and psychological factors; 2) Our current control strategies are continuous within the specific time interval, however, in practical scenario, decision-makers can only intervene in the spreading processes at discrete time due to cost constraints. Therefore, we can introduce discrete controls to formulate the optimization problem, and discuss the optimal intensity and interval of these controls.
The original contributions presented in the study are included in the article/Supplementary material, further inquiries can be directed to the corresponding author.
LC: Conceptualization, Formal Analysis, Funding acquisition, Methodology, Project administration, Supervision, Writing–original draft, Writing–review and editing. HZ: Formal Analysis, Methodology, Software, Validation, Writing–original draft. XW: Formal Analysis, Methodology, Software, Validation, Writing–review and editing. XA: Formal Analysis, Methodology, Software, Validation, Visualization, Writing–review and editing.
The author(s) declare financial support was received for the research, authorship, and/or publication of this article. This work was supported by the Shandong Social Science Planning Fund Program under Grant No. 20CGLJ24.
The authors declare that the research was conducted in the absence of any commercial or financial relationships that could be construed as a potential conflict of interest.
All claims expressed in this article are solely those of the authors and do not necessarily represent those of their affiliated organizations, or those of the publisher, the editors and the reviewers. Any product that may be evaluated in this article, or claim that may be made by its manufacturer, is not guaranteed or endorsed by the publisher.
1. Lenormand M, Gonçalves B, Tugores A, Ramasco JJ. Human diffusion and city influence. J R Soc Interf (2015) 12:20150473. doi:10.1098/rsif.2015.0473
2. Jin C, Li B, Jansen SJT, Boumeester HJFM, Boelhouwer PJ. What attracts young talents? Understanding the migration intention of university students to first-tier cities in China. Cities (2022) 128:103802. doi:10.1016/j.cities.2022.103802
3. Jiang H, Jiang P, Wang D, Wu J. Can smart city construction facilitate green total factor productivity? A quasi-natural experiment based on China’s pilot smart city. Sustain Cities Soc (2021) 69:102809. doi:10.1016/j.scs.2021.102809
4. Sardar T, Nadim SS, Rana S. Detection of multiple waves for COVID-19 and its optimal control through media awareness and vaccination: study based on some Indian states. Nonlinear Dyn (2023) 111:1903–20. doi:10.1007/s11071-022-07887-5
5. Tiwari PK, Rai RK, Khajanchi S, Gupta RK, Misra AK. Dynamics of coronavirus pandemic: effects of community awareness and global information campaigns. Eur Phys J Plus (2021) 136:994. doi:10.1140/epjp/s13360-021-01997-6
6. Rai RK, Khajanchi S, Tiwari PK, Venturino E, Misra AK. Impact of social media advertisements on the transmission dynamics of COVID-19 pandemic in India. J Appl Math Comput (2022) 68:19–44. doi:10.1007/s12190-021-01507-y
7. Yuan Y, Li N. Optimal control and cost-effectiveness analysis for a COVID-19 model with individual protection awareness. Phys A: Statist Mech Appl (2022) 603:127804. doi:10.1016/j.physa.2022.127804
8. Pertwee E, Simas C, Larson HJ. An epidemic of uncertainty: rumors, conspiracy theories and vaccine hesitancy. Nat Med (2022) 28:456–9. doi:10.1038/s41591-022-01728-z
9. Harper T, Attwell K. How vaccination rumours spread online: tracing the dissemination of information regarding adverse events of covid-19 vaccines. Int J Public Health (2022) 30:1604228. doi:10.3389/ijph.2022.1604228
10. Islam MS, Kamal AHM, Kabir A, southern DL, Khan SH, Hasan SMM, et al. COVID-19 vaccine rumors and conspiracy theories: the need for cognitive inoculation against misinformation to improve vaccine adherence. Plos One (2021) 16(5):e0251605. doi:10.1371/journal.pone.0251605
11. Yu Z, Lu S, Wang D, Li Z. Modeling and analysis of rumor propagation in social networks. Inf Sci (2021) 580:857–73. doi:10.1016/j.ins.2021.09.012
12. Yin F, Xia X, Zhang X, Zhang M, Lv J, Wu J. Modelling the dynamic emotional information propagation and guiding the public sentiment in the Chinese Sina-microblog. Appl Math Comput (2021) 396:125884. doi:10.1016/j.amc.2020.125884
13. Zhu Q, Zhang G, Luo X, Gan C. An industrial virus propagation model based on SCADA system. Inf Sci (2023) 630:546–66. doi:10.1016/j.ins.2022.12.119
14. Lucarelli A, Olof BP. City branding: a state-of-the-art review of the research domain. J Place Manage Dev (2011) 4(1):9–27. doi:10.1108/17538331111117133
15. Perez-Cornejo C, Rodríguez-Gutierrez P, Quevedo-Puente E. City reputation and the role of sustainability in cities. Sustain Dev (2023) 31(3):1444–55. doi:10.1002/sd.2459
16. Delgado-García JB, Quevedo-Puente E, Blanco-Mazagatos V. The impact of city reputation on city performance. Reg Stud (2018) 52(8):1098–110. doi:10.1080/00343404.2017.1364358
17. Aloweidi A, Bsisu I, Suleiman A, Abu-Halaweh S, Almustafa M, Aqel M, et al. Hesitancy towards COVID-19 vaccines: an analytical cross–sectional study. Int J Environ Res Public Health (2021) 18(10):5111. doi:10.3390/ijerph18105111
18. Wu J, Tang B, Bragazzi NL, Nah K, McCarthy Z. Quantifying the role of social distancing, personal protection and case detection in mitigating covid-19 outbreak in Ontario, Canada. J Math Ind (2020) 10(1):15–2. doi:10.1186/s13362-020-00083-3
19. Jarvis CI, Van Zandvoort K, Gimma A, Prem K, Klepac P, Rubin GJ, et al. Quantifying the impact of physical distance measures on the transmission of COVID-19 in the UK. BMC Med (2020) 18:124. doi:10.1186/s12916-020-01597-8
20. Sharevski F, Huff A, Jachim P, Pieroni E. (Mis) perceptions and engagement on twitter: COVID-19 vaccine rumors on efficacy and mass immunization effort. Int J Inf Manag Data Insights (2022) 2(1):100059. doi:10.1016/j.jjimei.2022.100059
21. Fan J, Wang X, Du S, Mao A, Du H, Qiu W. Discussion of the trust in vaccination against COVID-19. Vaccines (2022) 10(8):1214. doi:10.3390/vaccines10081214
22. Antonopoulos CG, Shang Y. Opinion formation in multiplex networks with general initial distributions. Sci Rep (2018) 8:2852. doi:10.1038/s41598-018-21054-0
23. Yin F, Pan Y, Tang X, Wu C, Jin Z, Wu J. An information propagation network dynamic considering multi-platform influences. Appl Math Lett (2022) 133:108231. doi:10.1016/j.aml.2022.108231
24. Wang J, Jiang H, Ma T, Hu C. Global dynamics of the multi-lingual SIR rumor spreading model with cross-transmitted mechanism. Chaos Sol Fract (2019) 126:148–57. doi:10.1016/j.chaos.2019.05.027
25. Wang J, Wang YQ, Li M. Rumor spreading considering the herd mentality mechanism. In: 36th Chinese Control Conference; 26-28 July 2017; Dalian, China (2017). p. 1480–5.
26. Xiao Y, Chen D, Wei S, Li Q, Wang H, Xu M. Rumor propagation dynamic model based on evolutionary game and anti-rumor. Nonlinear Dyn (2019) 95:523–39. doi:10.1007/s11071-018-4579-1
27. Wang Q, Yang X, Xi W. Effects of group arguments on rumor belief and transmission in online communities: an information cascade and group polarization perspective. Inf Manage (2018) 55:441–9. doi:10.1016/j.im.2017.10.004
28. Hosni AIE, Li K, Ahmad S. Minimizing rumor influence in multiplex online social networks based on human individual and social behaviors. Inf Sci (2020) 512:1458–80. doi:10.1016/j.ins.2019.10.063
29. Zareie A, Sakellariou R. Minimizing the spread of misinformation in online social networks: a survey. J Netw Comput Appl (2021) 186:103094. doi:10.1016/j.jnca.2021.103094
30. Yan R, Li Y, Wu W, Li D, Wang Y. Rumor blocking through online link deletion on social networks. ACM Trans Knowl Discov Data (2019) 13(2):1–26. doi:10.1145/3301302
31. Wang B, Chen G, Fu L, Song L, Wang X. Drimux: dynamic rumor influence minimization with user experience in social networks. IEEE Trans Knowl Data Eng (2017) 29(10):2168–81. doi:10.1109/tkde.2017.2728064
32. Cheng Y, Huo L, Zhao L. Stability analysis and optimal control of rumor spreading model under media coverage considering time delay and pulse vaccination. Chaos Sol Fract (2022) 157:111931. doi:10.1016/j.chaos.2022.111931
33. Chen S, Jiang H, Li L, Li J. Dynamical behaviors and optimal control of rumor propagation model with saturation incidence on heterogeneous networks. Chaos Sol Fract (2020) 140:110206. doi:10.1016/j.chaos.2020.110206
34. Lin Y, Wang X, Hao F, Jiang Y, Wu Y, Min G, et al. Dynamic control of fraud information spreading in mobile social networks. IEEE Trans Syst Man Cybern Syst (2021) 51(6):3725–38. doi:10.1109/tsmc.2019.2930908
35. Li L, Liu Y, Zhou Q, Yang W, Yuan J. Targeted influence maximization under a multifactor-based information propagation model. Inf Sci (2020) 519:124–40. doi:10.1016/j.ins.2020.01.040
36. Wang X, Wang X, Min G, Hao F, Chen CLP. An efficient feedback control mechanism for positive/negative information spread in online social networks. IEEE Trans Cybern (2022) 52(1):87–100. doi:10.1109/tcyb.2020.2977322
37. Van den Driessche P, Watmough J. Reproduction numbers and sub-threshold endemic equilibria for compartmental models of disease transmission. Math Biosci (2002) 180:29–48. doi:10.1016/s0025-5564(02)00108-6
38. Shang Y. Lie algebraic discussion for affinity based information diffusion in social networks. Open Phys (2017) 15(1):705–11. doi:10.1515/phys-2017-0083
Keywords: competitive information propagation, local-global prevalence, multi-layer networks, individual adaptive behavior, optimal control
Citation: Cao L, Zhao H, Wang X and An X (2023) Competitive information propagation considering local-global prevalence on multi-layer interconnected networks. Front. Phys. 11:1293177. doi: 10.3389/fphy.2023.1293177
Received: 12 September 2023; Accepted: 12 October 2023;
Published: 31 October 2023.
Edited by:
Ayse Peker-Dobie, Istanbul Technical University, TürkiyeReviewed by:
Yilun Shang, Northumbria University, United KingdomCopyright © 2023 Cao, Zhao, Wang and An. This is an open-access article distributed under the terms of the Creative Commons Attribution License (CC BY). The use, distribution or reproduction in other forums is permitted, provided the original author(s) and the copyright owner(s) are credited and that the original publication in this journal is cited, in accordance with accepted academic practice. No use, distribution or reproduction is permitted which does not comply with these terms.
*Correspondence: Li Cao, Y2xqeTcxN0AxNjMuY29t
Disclaimer: All claims expressed in this article are solely those of the authors and do not necessarily represent those of their affiliated organizations, or those of the publisher, the editors and the reviewers. Any product that may be evaluated in this article or claim that may be made by its manufacturer is not guaranteed or endorsed by the publisher.
Research integrity at Frontiers
Learn more about the work of our research integrity team to safeguard the quality of each article we publish.