- 1Labvantage - Biomax GmbH, Planegg, Germany
- 2Seven Past Nine GmbH, Schopfheim, Germany
- 3School of Geography, Earth and Environmental Sciences, University of Birmingham, Birmingham, United Kingdom
- 4Nanoinformatics Department, NovaMechanics Ltd., Nicosia, Cyprus
- 5Department of Bioinformatics—BiGCaT, Research Institute of Nutrition and Translational Research in Metabolism, Faculty of Health, Medicine and Life Sciences, Maastricht University, Maastricht, Netherlands
- 6Entelos Institute, Larnaca, Cyprus
- 7School of Chemical Engineering, National Technical University of Athens, Athens, Greece
- 8School of Physics, University College Dublin, Belfield, Dublin, Ireland
- 9Institute of Cancer and Genomic Sciences, University of Birmingham, Birmingham, United Kingdom
- 10Ideaconsult Ltd., Sofia, Bulgaria
- 11TEMAS Solutions GmbH, Hausen, Switzerland
- 12UK Centre for Ecology & Hydrology, Lancaster, United Kingdom
- 13UK Centre for Ecology & Hydrology, Wallingford, United Kingdom
- 14Department Biosciences & Medical Biology, Paris Lodron University of Salzburg, Salzburg, Austria
- 15Hellenic Military Academy, Vari, Greece
In mediaeval Europe, the term “commons” described the way that communities managed land that was held “in common” and provided a clear set of rules for how this “common land” was used and developed by, and for, the community. Similarly, as we move towards an increasingly knowledge-based society where data is the new oil, new approaches to sharing and jointly owning publicly funded research data are needed to maximise its added value. Such common management approaches will extend the data’s useful life and facilitate its reuse for a range of additional purposes, from modelling, to meta-analysis to regulatory risk assessment as examples relevant to nanosafety data. This “commons” approach to nanosafety data and nanoinformatics infrastructure provision, co-development, and maintenance is at the heart of the “NanoCommons” project and underpins its post-funding transition to providing a basis on which other initiatives and projects can build. The present paper summarises part of the NanoCommons infrastructure called the NanoCommons Knowledge Base. It provides interoperability for nanosafety data sources and tools, on both semantic and technical levels. The NanoCommons Knowledge Base connects knowledge and provides both programmatic (via an Application Programming Interface) and a user-friendly graphical interface to enable (and democratise) access to state of the art tools for nanomaterials safety prediction, NMs design for safety and sustainability, and NMs risk assessment, as well. In addition, the standards and interfaces for interoperability, e.g., file templates to contribute data to the NanoCommons, are described, and a snapshot of the range and breadth of nanoinformatics tools and models that have already been integrated are presented Finally, we demonstrate how the NanoCommons Knowledge Base can support users in the FAIRification of their experimental workflows and how the NanoCommons Knowledge Base itself has progressed towards richer compliance with the FAIR principles.
1 Introduction
Imagine you could easily see which nanomaterials (NMs) have been characterised in any of the >60 past and ongoing European Union (EU) NanoSafety Cluster projects. Imagine you could access tools for automatic analysis of transmission electron microscopy (TEM) images and connect the resulting NM size characterisation to models predicting the distribution of, or occupational exposure to, the specific NM. Imagine you could connect quantitative structure-activity relationship (QSAR), physiology-based pharmacokinetic (PBPK) and adverse outcome pathway (AOP) based models to predict the toxic effects of a specific NM, and use this information to re-design/modify the NM using tools for safe and sustainable by design NMs development. If none of these appeal to you, do not waste your time reading on, because this seamless integration of data, analysis tools, and predictive modelling is the vision of “the NanoCommons”, a collaborative, open infrastructure for nanoinformatics applied to materials design and safety and sustainability assessment. Here, one of the central components of the NanoCommons, its Knowledge Base, which provides technical and semantic interoperability to connect data, data sources, tools and, most important, knowledge, is described.
The NanoCommons was started as part of the EU research infrastructure project (NanoCommons). The NanoCommons project was funded as a “starting community” with the aim to develop solutions to overcome the challenges of data fragmentation and inaccessibility in the nanosafety arena. It also aimed to provide a set of tools and services to organise and visualise nanosafety data and data relationships, make the data accessible, and integrate computational tools for risk assessment and decision support. In its 2016–2017 work programme the European Commission defined a “starting community” as “a community that has not been supported for the integration of its infrastructures … shows a limited degree of coordination and networking … focus on networking, standardisation and establishing common access procedures to the specific tools and/or services offered.” Central to the NanoCommons vision, therefore, was the establishment of a community-owned and co-developed nanosafety informatics research infrastructure, in collaboration with the EU Nanosafety Cluster and internationally via the EU-US Communities of Research and other ongoing partnerships. To this end, a multi-project collaboration was established to develop tools and standards for nanosafety data management and integration of modelling tools to enhance their interoperability and sustainability. The ultimate goal was the establishment of an ecosystem to serve and connect the community in all aspects of human and ecosystem risk assessment, risk governance and safe and sustainable design of nanoscale materials, nano-enabled products and advanced materials.
The need for a NMs and nanosafety specific research infrastructure was predicated by the well-known challenges of regulating NMs (e.g., [1–3]), given their dynamic nature, their properties as both particles and chemicals, and their multitude of applications across traditional regulatory sector boundaries (e.g., silver NMs have applications in cosmetics, textiles, electronics, and as biocides and pesticides ([1]; [4]). Indeed, in 2022 the European Chemicals Agency issued an Appendix to the chemicals legislation REACH to address the additional reporting and data requirements required for nanoforms, defined as “a form of a … substance containing particles … size distribution of one or more external dimensions is in the size range 1 nm–100 nm” where variation of the characteristics (size distribution, shape and other morphological characterisation, surface treatment and functionalisation and specific surface area of the particles) results in a different nanoform [5].
The EU-US Nanoinformatics Roadmap [6] identified two of the main needs and barriers to achieving the promise of Nanoinformatics as the lack of sufficiently accessible data for model development and the lack of a much-needed community infrastructure to support data and model accessibility through training and the development of community consensus regarding standards for metadata reporting and interoperability. The term “infrastructure” in this case is referring to “a collection of connectable tools to perform a (set of specific) purpose(s)” whether these are the network of power stations, cables and transformers needed to keep cities powered, or the software standards, regulatory frameworks, and information technology environment necessary to perform exposure, hazard, sustainability, and risk assessment of chemicals and NMs. Community infrastructures, in the EU Research infrastructures conception, are “facilities that provide resources and services for the research communities to conduct research and foster innovation in their fields”. These are designed to underpin multiple technological approaches and provide tailorable solutions for a multitude of users with different needs. This general purpose approach is an important distinction from project-level platforms (e.g., GuideNano, SUNDS, NanoinformatTIX, SbD4nano) built for a very specific purpose, which are often closed systems with limited potential for onward integration or development. Limiting factors include restrictive intellectual property ownership models, limited investment in graphical user interfaces, lack of time as the project ends before the interface can be implemented and tested, and many other challenges not the least a lack of sustainability (e.g., [7, 8]). Indeed, even well-established freely-accessible Knowledge Bases such as UniProt struggle for long term sustainability [8, 9], further emphasising the important role of community research infrastructures and the collaborative development approach. A common theme for all infrastructures is the requirement for interoperability of the individual parts. For nanosafety and nanoinformatics these include, for example, standards for harmonising data upload and download, processes to ensure that the data outputs from models and workflows are in the correct format to be upload to the Knowledge Base, and APIs for two-way exchange of data between data warehouses and modelling workflows. All these require a common understanding of what to exchange, in what format and how to exchange it (e.g., increasingly moving towards concepts of data visiting rather than duplication of datasets as described in [10]). These functionalities support actionability of the data and ensure their continued utility for secondary exploitation.
The “how to exchange” concerns the interfaces to be established between software (similar to the plugs and sockets of an electricity infrastructure). The format concerns questions of syntax (in the electricity example this might concern the 110 V US and 220 V European electricity formats) for data management. Furthermore, it concerns differences between generally computationally readable ASCII text such as comma delimited or extendedly marked up (XML) files compared to binary file formats like .docx or .xlsx, which require proprietary software to read. However, most vexing is the issue of “what to exchange” which in data and knowledge management concerns the semantics or meaning of each bit of exchanged information. Traditionally, informatics infrastructure has been concerned primarily with the exchange of raw data. For instance, the world wide web facilitates transfer of, and access to, files that communicate structured or unstructured information, such as images or natural language text. The infrastructure is typically concerned solely with transfer of the data, and it is only by external consensus that meaning can be derived from/assigned to them. In the case of natural language text, it is our shared faculty for language and agreement upon the meaning of a particular language that allows us to derive meaning. In the case of files that describe information in a more structured way, such as a spreadsheet, the semantics may again be derived from human interpretation, or encoded in any software that interacts with it. In order to provide these functionalities, in a way that data becomes machine actionable, implementation of a computable semantics is required.
For example, a dataset may describe a series of cell viability measurements pertaining to cells that have been exposed to a particular nanomaterial. The dataset may take the form of a spreadsheet, and include assays such as with/without nucleus stored as strings, as well as measurements of cell size in numerical form. Critically, however, none of these data points by themselves inherently mean anything - they are fundamentally ambiguous and their meaning must be inferred by the person or software that interacts with the dataset (similar to “110” having no meaning unless one knows it concerns 110 V for the aforementioned power supplies). This presents a problem for continued access to, use and integration of this dataset with others, because its semantics are sequestered in the humans and software that originally created and interacted with the dataset. Standard external free-text documentation only partially solves this problem, because it too requires manual human interpretation and action.
In order to make data machine-actionable, and thereby to support uses such as integrative analysis, the semantics must instead be available with the data and in a standardised, structured way. This is the basis of the linked data paradigm: ensuring that raw data are described with, or linked to, additional information required to make them actionable. For example, measurements of cell size in a spreadsheet column may be linked to a unique identifier that links to a consensus definition of cell size, and another with the unit being used. When these files are then uploaded to a supportive infrastructure, they contain the means by which cell size measurements can be aligned across datasets or into a common data model, with necessary steps taken for their integration (such as translation of units). When they are subsequently downloaded, the data can be interpreted and interacted with in the sense that it was intended.
Semantic description of data also builds meaning through the construction of a knowledge graph wherein linked data defines and provides access to background knowledge. For example, the phenotypic changes of cells in a toxicity test may be semantically described by linking them to an ontology of phenotypes, which provide static identifiers This phenotype ontology is also used to describe data in many other contexts, and therefore this dataset is now also linked to a vast source of background knowledge and context concerning phenotypic effects of toxic substances or genetic changes, which can be leveraged to enhance understanding and analysis of the dataset. By semantically describing data, access to a font of machine-actionable knowledge is achieved, but the users are also engaged in building it - it is from this reciprocal relationship that the greatest benefit of computational semantics arises.
In addition to the described technical issues for data accessibility and exchange much of the “Access” provision during the NanoCommons project related to data management up-skilling. A new data related functionality, termed “data shepherding”, was defined. Furthermore, NanoCommons pushed for data management to be considered much earlier in the data life cycle, indeed at the point of experimental planning and design, as a means to identify at the outset the metadata and data needs. This could help develop meta (data) capture tools to support the researchers in their day-to-day data generation and processing [11]. Thus, based on the experiences from data shepherding, the nanosafety community needs and requirements guided and informed the design and implementation of the technical solutions developed and integrated into the NanoCommons Knowledge Base. These acted as the means to overcome the various barriers that limit data sharing, data usefulness and thus data re-use (e.g., disparate data, lack of rich descriptive metadata, limited accessibility to data, unclear re-use conditions/licensing conditions). The infrastructure solutions provided by NanoCommons thus combine the technical aspects and the processes, persons and funding needed to “shepherd” the data along its entire life-cycle [12].
In the following, a key part of the NanoCommons infrastructure, the NanoCommons Knowledge Base, designed to provide interoperability for nanosafety data sources and nanoinformatics tools, both on a semantic and technical level, to connect knowledge and provide a user-friendly interface to access the data and associated modelling tools, is described. In addition, the standards and interfaces used to achieve the required interoperability are presented, including the file templates to enable the research community to contribute their datasets to the NanoCommons Knowledge Base, and the programming interface to enable connection of tools allowing them direct access to the data in the NanoCommons Knowledge Base.
2 General concept of the NanoCommons Knowledge Base
2.1 Software framework reference and brief description
The technical framework of the NanoCommons Knowledge Base, the BioXM™ Knowledge Management Environment, has been described previously [13]. Briefly the system is implemented as a platform-independent Java server—HTML (hypertext markup language) client application. It is a General Data Protection Regulations (GDPR) and ISO 27001 compliant resource providing user management and auditing which allow detailed control and monitoring of users rights and activities to view or modify certain data. The application backend integrates No-SQL and relational database management and employs a Hibernate layer for object-relation modelling. Therefore, all administrative activities occur on the level of an object-oriented graph model which is automatically transformed into a generic database scheme. Actual information, including ontologies and data, mapped to the object-oriented model form a dense knowledge-graph for search, retrieval and analysis. A restful (REST) web service application programming interface (API) provides technical interoperability for transparent tool integration. On the one hand this translates external resources into native reports or analyses delivered by the user interface (UI), on the other hand it facilitates access to data by external tools. A range of connections to community standards such as KNIME nodes, Jupyter notebooks as well as the various Jaqpot and Enalos nanoinformatics applications are described in further detail below, to demonstrate the openness of the Knowledge Base and its role in ensuring ease of access to nanosafety data to third-party tools.
2.2 Semantic model
The NanoCommons Knowledge Base semantic model and standard REST API provide an open infrastructure which allows any data source or tool to be connected. To this end the data source concepts (e.g., nanomaterial, endpoint) or the tool input/output parameters are mapped to the NanoCommons Knowledge Base semantic model (see Figure 1). A semantic model is a method of organizing data that reflects the basic meaning of data items and the relationships between them, which ensures consistency of uploaded data.
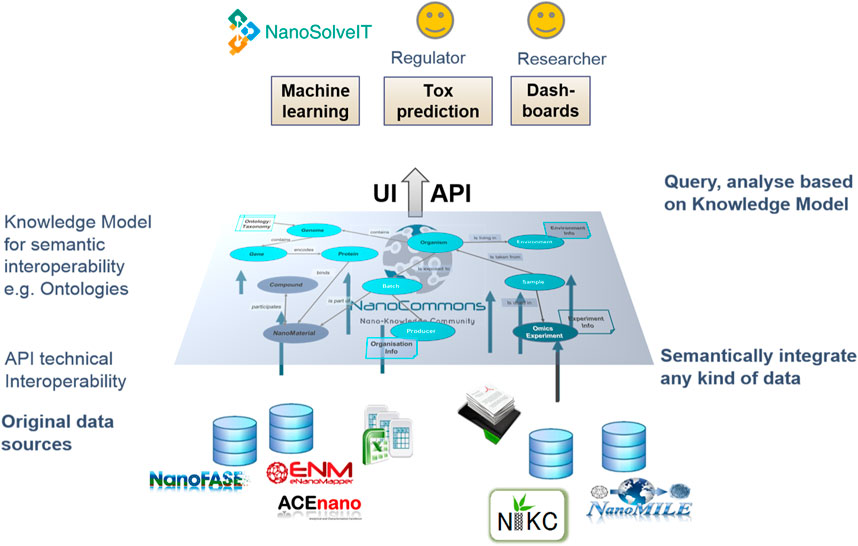
FIGURE 1. The semantic model integrates standard Ontologies with data source concepts or tool input/output parameters. The API provides technical interoperability.
While data from EU-funded projects such as NanoFASE or NanoMILE is managed directly in the NanoCommons Knowledge Base data warehouse, other data sources such as the “Nanosafety Data Interface” based project data [14] are integrated virtually through semantic mapping and use of the APIs of both the NanoCommons Knowledge Base and the integrated resource (see Figure 2).
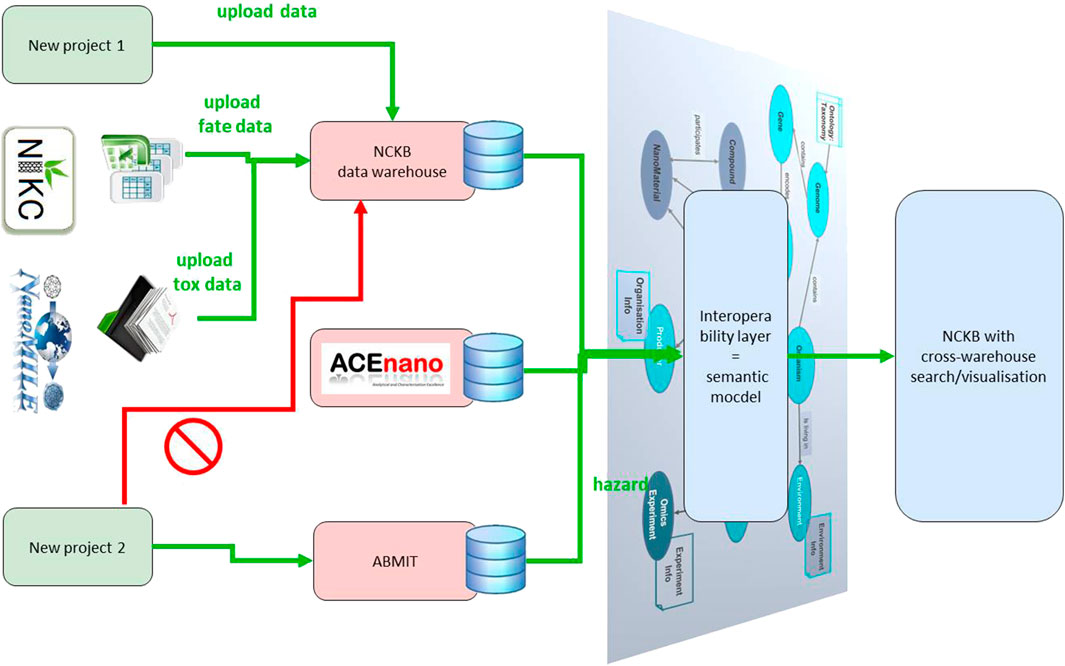
FIGURE 2. Illustration of the “virtual integration” of external data resources into the NanoCommons Knowledge Base. “Virtual integration” Indicates both the technical and semantic concepts of virtual data integration.
A detailed description of the mapping process has been provided previously [13]. Briefly, the NanoCommons Knowledge Base employs existing ontologies such as the eNanoMapper ontology [14], the environmental ontology [15], or the phenotype and trait ontology [16]. Whenever required concepts could not be mapped to an existing ontology, the gap was filled using a consensus concept and a request submitted to the appropriate domain ontology for extension. To unambiguously map data, information and knowledge into the common data model, unique entity references from reference databases such as PubChem [17] for chemicals or the European Registry of Materials (ERM) [18] for NMs, are employed.
3 NanoCommons Knowledge Base functionality and use
3.1 Data uploads
The NanoCommons Knowledge Base enables users to manage their own data as public or private within a Nanoinformatics data repository. To this end, a file-template based data upload functionality is provided. Based on user feedback from the multiple groups collaborating with The NanoCommons, a mechanism and associated templates were established, which combine flexibility and ease of use by separating the upload into three steps: 1. Batch import of nanomaterials 2. Upload of measurement parameters (“endpoints”) 3. Import of actual measurement data.
Due to the breadth of potential assays and the fast technological and scientific development, a decision was taken to abstain from defining a fixed set of measurement parameters and instead allow users to extend the set of covered endpoints. At the same time, the integrative NanoCommons infrastructure needs to go beyond simple data archives with semantically undefined measurement parameters. Therefore, an upload and mapping mechanism has been developed, which allows users to dynamically extend the measurement parameters accommodated by the system while at the same time ensuring semantic mapping of new parameters via ontologies. The semantic model underlying the NanoCommons Knowledge Base allows mapping of a diverse variety of information to each other and to external sources such as the European Registry of Materials [18] and in due course to the NMs InChI extension ([19]; [20]) (Figure 3).
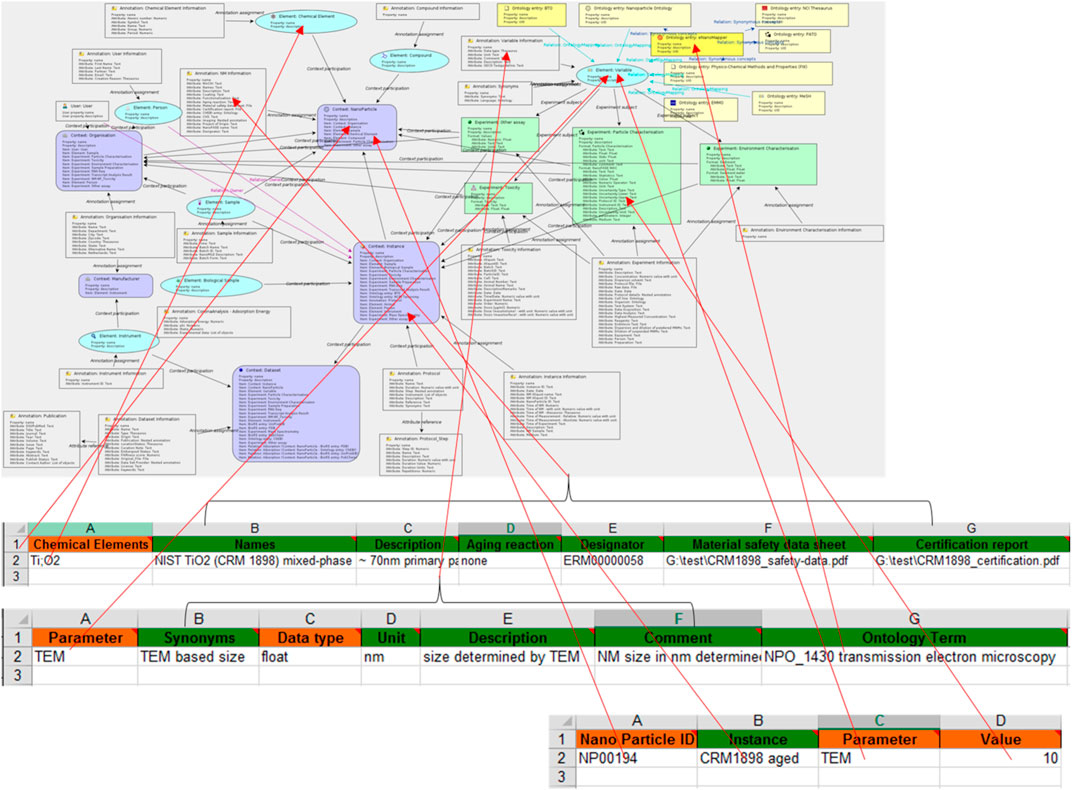
FIGURE 3. The NanoCommons Knowledge Base semantic model includes concepts such as “Nanomaterial”, “Instance”, “Parameter” and “Experiment:Particle Characterisation” which can be associated with each other, for example, “Nanomaterial has Instance”. During data upload the information from the upload templates is extracted and mapped to the semantic model (red arrows) which thereby connects data from different sources and types, e.g., physico-chemical characterisation and toxicology, and allows retrieval of related data from different sources.
3.2 Search and retrieve
For datasets and tools integrated directly into the NanoCommons Knowledge Base, metadata made public by their providers is made searchable in public search engines. The access to actual data/tools then requests a registration to enable authentication and authorisation. The NanoCommons Knowledge Base, therefore, is fully compliant with the GDPR. Providing options for tailored solutions and support for individual users and registration also helps to support future sustainability by enabling anonymised monitoring of stakeholder-group-based usage statistics.
A sliding menu provides support features such as News, a Help Desk with manuals and FAQs, “My Profile” to manage personal contact data and login information, and a link to The NanoCommons User Guidance Handbook with training materials, video tutorials and in-depth descriptions to The NanoCommons infrastructure (for further details see the NanoCommons Knowledge Base User Manual in the Supplementary Material SI).
As of writing, information for over 1,400 NMs with physico-chemical and/or toxicological/ecotoxicological characterisation are available via the NanoCommons Knowledge Base. Importantly, all information is organised, as far as possible, around individual NMs (or environmental samples of unknown NM composition) rather than on the level of datasets bringing together diverse information from different experiments and even institutions to provide a full picture of existing, specific NM related information. As noted above, metadata and data from a range of public projects is directly managed within the NanoCommons Knowledge Base (nanoMILE, NanoFASE, SmartNanoTox, NanoSolveIT, NanoPAT) while other projects using different technical primary data management solutions are integrated on the level of metadata only (via the Nanosafety Data Interface, metadata from eNanoMapper, NanoReg and NanoReg2 projects).
Users can browse through/search in the following NM related information.
• General Data such as supplier information including designator (provider code/ID), ERM ID, and NInChI [19] (in experimental alpha version), project-specific names (if relevant), synthesis date (if available) and/or opening date of bottle (for commercial samples) as part of the provenance information [21] storage conditions for the samples to minimise ageing during storage [22] (alternatively aging reaction and transformations if relevant [23], general description, composition information (including coating/capping);
• Physico-chemical characterisation data such as size, shape or crystal phase (if relevant);
• Omics data from in vitro exposure assays;
• Toxicity/ecotoxicity data, release, exposure and environmental fate data and computational descriptors calculated for a variety of simulated and existing NMs. As far as available protocols and methods for all experimental procedures are included and linked to the corresponding data.
For physico-chemical characteristics, typical information available are primary NM size determined by electron microscopy (TEM or STEM, including number of NMs analysed), size distribution (polydispersity index), hydrated/aggregated size by dynamic light scattering (DLS), shape (as controlled vocabulary such as “particle”, “fibre”), crystallinity, coating composition and how the coating is attached to the NM, the form in which the NM was supplied (e.g., powder, aqueous dispersion, etc.), dispersion liquid, redox state (where relevant), Zeta potential (as a measure of surface charge, which should be accompanied by the pH at which it was measured, and the ionic strength of the measurement liquid), electrophoretic mobility from which the zeta potential value is derived and energy band gap calculated from the UV-Vis spectrum using Tauc plots [24]. In addition, NMs are associated with the TEM/STEM images to enable further analysis. Available information for a given NM depends on the contributions from the community and whether a given property is deemed relevant for the given NM (such as redox state deemed relevant for some metal oxides (e.g., Fe, Ce) but not relevant for others (e.g., Ti, Si); some elements can exist in multiple crystal phases (e.g., Ti can exist as anatase, rutile, brookite and amorphous forms) while other elements have only one lattice structure).
• Currently all omics data available via the NanoCommons Knowledge Base are RNA-seq based gene expression data from in vitro cell culture assays. Additional data sets recently curated from the literature are made available as part of The NanoCommons infrastructure via the NanoPharos database [25].
The user interface provides exports in tab-delimited text format as well as .xlsx (Excel) for all available information; the API in addition provides .xml and .json format options.
3.3 Use of ontologies
In addition to forming part of the semantic model, ontologies including the Cell Ontology (CO) [26] inform the knowledge graph by populating concepts such as “cells” with relevant information like, for example, which cell types exist in humans or a list of cell-lines derived from human cell types. Further use is the application as controlled vocabulary to provide metadata for information mapped into the knowledge graph. For example, a toxicological assay may provide data on cell viability (as number reflecting the percentage of cells alive) while the CO may be mapped with the item CO_000001 A549 to the dataset, indicating that A549 cells (a human cell line derived from lung epithelial cells) where used in the assay.
Another example is the use of the eNanoMapper Ontology (eNMO) to describe NMs in a standardised way (Figure 4).
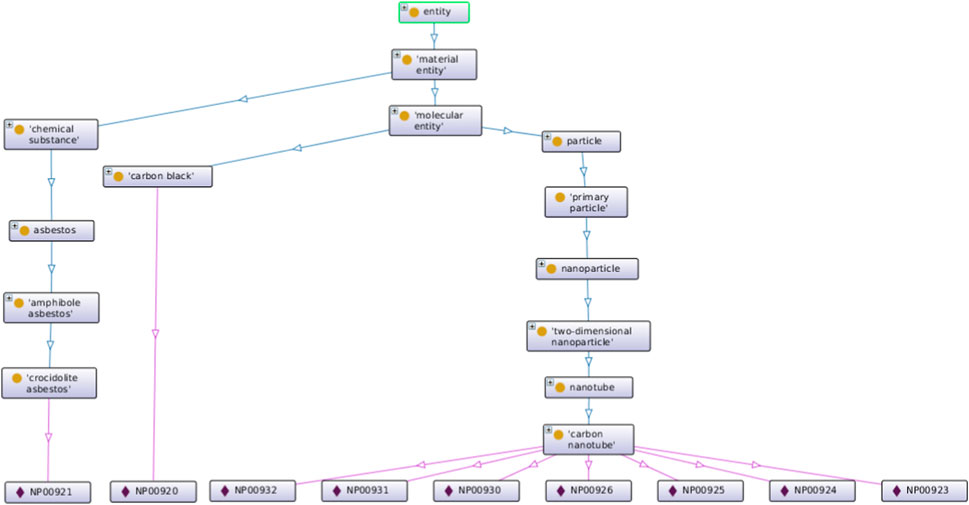
FIGURE 4. “Protégé visualization of the class hierarchy for the eNMO classes used in the NC KNOWLEDGE BASE to annotate different NMs identified by IDs NP0000X.
The eNMO terms used to annotate NMs in the NanoCommons Knowledge Base are sourced from the upstream ontologies NPO (carbon nanotube) and ChEBI (crocidolite asbestos and carbon black). The division into chemical substance and molecular entity results from the different ontological definitions in the imported ontologies: a chemical substance, defined by ChEBI as a portion of matter of constant composition, against the NPO approach of addressing the individual molecular entities themselves.
Mapping of appropriate ontology terms to metadata is a manual process requiring expert domain knowledge. In the NanoCommons Knowledge Base it is supported by an ontology search which provides keyword-to-multi-ontology-mapping features to enable fast identification of most appropriate terms.
3.4 Ontology search tool
A user interface is available within the NanoCommons Knowledge Base for data owners to quickly and easily search for ontological terms and identifiers that match the terms used in the metadata or the properties measured in their data. By annotating the identified ontology term to their experimental term in the data upload template, the NanoCommons Knowledge Base “knows” exactly how to semantically map, i.e., where to add the term and the associated data into the knowledge graph, to make it easily searchable and retrievable, and enable combining disparate data. A range of relevant ontologies have been incorporated (see Supplementary Material SVI with the list of ontologies and their references) and, as part of The NanoCommons, these are being extended where needed (e.g., via the GRACIOUS Terminology Harmonizer [27]).
3.5 Data FAIRification
The NanoCommons Knowledge Base is registered with r3data.org to make it visible as a searchable data source. Datasets with agreed public visibility are disseminated by making their metadata visible in schema.org, which is indexed by search engines. In addition the H2020 project SbD4Nano provides and indexes the metadata in their landscape at github.com/h2020-sbd4nano/sbd-data-nanocommons. Listing the datasets in Google allows tracking of citations of the datasets as well, which can be higher than the citations to the original papers. Standard formats, controlled vocabularies and the API make data in the NanoCommons Knowledge Base accessible and interoperable with other datasets deposited in other repositories. Re-usability, beyond providing a clear licence stating who may re-use the data under which conditions (as requested in the data/tool registration template and most typically fulfilled by adding a Creative Commons licence), is a use-case dependent feature. This requires data and tool providers to submit appropriate information as part of the metadata that accompanies the data. An automatic visual feedback, presented as a “completeness check”, empowers information providers to understand the level of re-usability achieved and to get insight into opportunities to further increase the re-usability of their data (see section 5.2.2 “Data Completeness indicator” below for further details).
3.6 Private and embargoed areas
By default, all information uploaded to the NanoCommons Knowledge Base is immediately publicly available. Quality and completeness of data is ensured by the users providing the corresponding information. However, the NanoCommons Knowledge Base offers upon request the alternative option to keep data private, shared only within a certain group or consortium, or to keep it embargoed for a certain period of time. The corresponding rights and restrictions are role based and currently need to be manually assigned upon user request by the maintenance team.
3.7 Overall usage
The NanoCommons Knowledge Base requires a simple do-it-yourself automatic registration to enable monitoring of usage distribution for sustainability. This allows gathering its stakeholder distribution to some extent (Figure 5). With almost 250 registrations, academic users prevail (>40%) followed by Research Organisations (10%–20%), Government Agencies (5%–15%), and SMEs (15%). Other important stakeholders are Regulators (3%–6%), Policymakers (1%–3%), Large Industry (2%–4%), Consultancies and NGOs (1%–3%). Since it became publicly available in 2019 the NanoCommons Knowledge Base has been accessed more than 10,000 times by its registered users with at maximum, so far, of 32 concurrently active users (during one of the NanoCommons Knowledge Base training sessions).
4 Integrated data sources
4.1 Nanosafety data interface (NSD)
For interoperability with the Nanosafety Data Interface (NSD, [28, 29]) the NanoCommons Knowledge Base API is used to issue calls against the NSD API (as an example of the process), and the NSD objects and concepts were mapped into the NanoCommons Knowledge Base semantic model (see Figure 6).
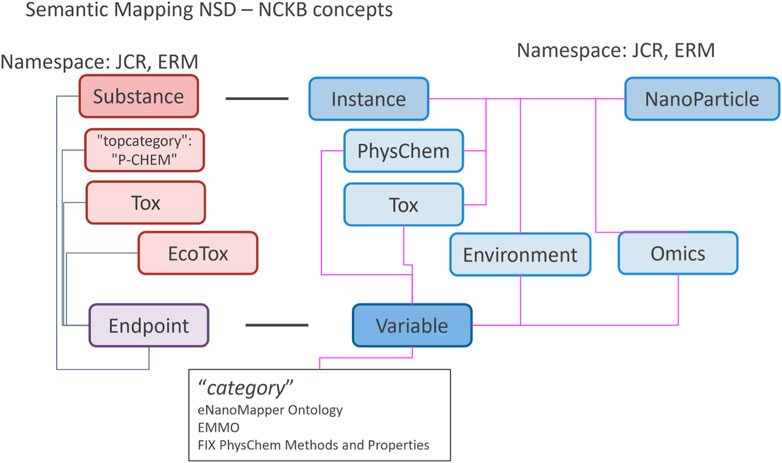
FIGURE 6. NSD concepts such as “Substance” or “Endpoint” where mapped to ontological terms, if possible, or directly to NanoCommons Knowledge Base concepts such as “Instance” or “Variable”.
The integration is metadata based and works across multiple individual NSD based data warehouses such as eNanoMapper or NANoREG. This can jointly be searched for:
1. List of available NMs
2. List of available endpoints (measurements - phys-chem and toxicological including omics)
3. List of available NMs with specific endpoints (e.g., Phys-chem characterisation)
4. Specific NMs by chemical identifier (EC, CAS registry number, IUPAC name, PubChem ID, InChI, SMILES, name).
Once a specific set of queries of interest combining NMs and available endpoints has been identified, the user is referred to the actual AMBIT data warehouse for data retrieval.
4.2 Institutional repositories providing highly structured data
More and more universities and research institutes make it optional or even mandatory to provide data in their institutional repositories, even in cases where a suitable community-agreed repository for the specific data type exists. However, these repositories are not domain specific and, thus, do not provide guidance on (meta) data schemas or harmonised templates. To showcase how data published on these repositories can still be integrated into the nanosafety data ecosystem, a prototype workflow was designed. The first step to achieve this is to provide the (meta) data in a machine-readable format, i.e., highly structured and, in the best case, fully semantically (ontologically) annotated. The example used in the prototype was results from a study on nanometer-sized fly ash particles identified through single particle inductively coupled plasma time of flight mass spectrometry (spICP-TOF-MS) fingerprinting performed by the University of Vienna [30]. Metadata files with information on the NMs, media and instruments used and protocol steps for a study on fly ash were combined with the data files as produced by the lab equipment into a complete data package according to the frictionless data specification (https://frictionlessdata.io/introduction/) and represents the primary data resource for this study to be uploaded to the institutional data repository of the University of Vienna (see also [31] in this special issue). To be able to index the data in the [32] Knowledge Base, an automated procedure was created optimised for on-the-fly data curation (Figure 7). The process translated the primary data format into the NIKC-NanoFASE format representing one of the standard data transfer formats accepted by the NanoCommons Knowledge Base. The procedure addressed the following tasks: 1) downloading the data from the institutional repository, 2) mapping of the customised (meta) data schema to the NanoCommons data model, 3) writing of the new NanoFASE file, and 4) uploading of the transformed (meta) data to the NanoCommons Knowledge Base. Task (1) and (4) are performed by utilising the APIs of the institutional repository and of the NanoCommons Knowledge Base, respectively. Since full semantic annotation of the data was out of scope of the prototype development, the mapping of the data models in step (2) had to be hard-coded limiting the applicability of the prototype to data generated in exactly the same way as done for the test data. Thus, further work is needed to automate this process and make it more generally applicable beyond this proof of concept.
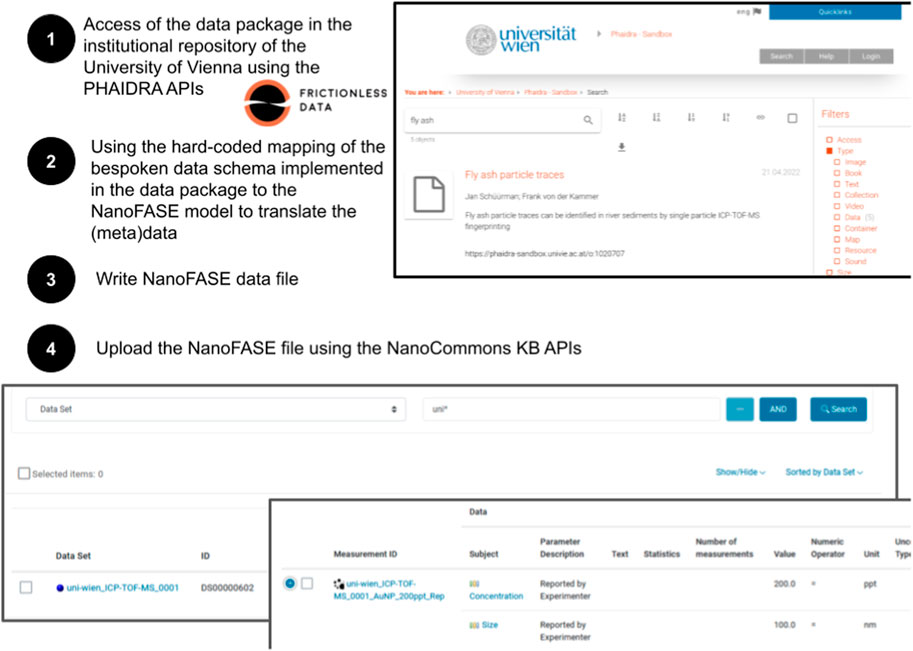
FIGURE 7. Steps of the automatic process starting with data packages stored in the institutional repository and resulting in the data indexed in the NanoCommons KNOWLEDGE BASE for improved findability and accessibility.
4.3 General life science databases
The BioXM framework technically underlying the NC Knowledge Base provides a No-SQL application which currently offers access to 141 public databases for integration into domain specific semantic models [13, 33]. Of these, the NC Knowledge Base currently makes available AlphaFold, Ensembl, Medline, PDB, PubChem, and UniProt as part of specific use cases such as the protein corona prediction model or the gene function enrichment analysis (see further details of these use cases below).
5 Software architecture for interoperability
5.1 Application programming interface
The NanoCommons Knowledge Base software framework provides a two-level access REST API. The first level remains stable regardless of any future extensions to content, functionality or connected tools. It also provides the authentication mechanism and the calls to discover available endpoints for search, retrieval or import of information. The second level is the ever increasing list of specific endpoints to be assessed. To access these named endpoints, any arbitrary request (conforming to the data model specification) can be posted as XML. An API call to the NanoCommons Knowledge Base therefore consists of several steps.
1. Authentication - establish session ID for re-use in next steps;
2. Generic call e.g., “search”, “report” to specific endpoint e.g., “list of nanomaterials”;
3. Closing of session.
To get a full list of available specific endpoints execute the generic call “Search_and_retrieve” to the endpoint “REST Webservice” (see the Supplementary Material SIII - for a full description of the API and an example Jupyter notebook).
In addition to enabling search, retrieval and data-import functions, the API forms the basis for integration of existing data sources into a “NanoCommons” by providing on-the-fly integrative search and reporting.
5.2 Integrated tools
5.2.1 RNA-seq
The tools for omics analysis integrated directly into the user interface of the NanoCommons Knowledge Base target non-expert users and therefore rely on pre-defined analysis methods and parameters without requiring in-depth knowledge of data analysis approaches. The analyses are based on the integration of R-based workflows and default parameters from published standards [6].
The basic workflow enables users to.
1. Select experimental data (transcription, protein, metabolome) in the portal;
2. Submit the selected data into a selected analysis tool such as “differential expression”. Only analysis methods suitable to the data type and dataset size are available for selection by users;
3. Run the analysis in background;
4. Access the results and store them locally and/or in the NanoCommons Knowledge Base;
5. Visualise them in a graph;
6. Re-use the results in further analysis, queries and overviews;
7. Make the results available to other users.
The following methods are currently available (and are described in detail in Supplementary Material SII).
1. Evaluation of data quality;
2. Normalisation;
3. Differential Expression Analysis;
4. Functional Enrichment Analysis;
5. Network reconstruction.
5.2.2 Data completeness indicator
To aid data re-use for a set of different use cases, a mechanism for automatic data completeness check and visualisation in the NanoCommons Knowledge Base was developed. To this end, it is necessary to first specify which data is actually needed for each of these cases. For example, developing a QSAR-model to predict toxicity will require a certain set of physico-chemical as well as toxicity endpoints, while differentiating nanoforms according to the REACH definition may require data for additional physico-chemical but no toxicity endpoints.
Based on reviews published by GRACIOUS [34] and the ToxRTool quality measures [35], as well as the NInChI concept [19] and the ECHA guideline on QSAR information requirements and read-across [36], use cases and endpoints that are relevant to these were collected. Based on these requirements, mappings between endpoints and use-cases were developed in order to be able to assess how “complete” data for a given NM is regarding a specific use case (see Supplementary Material SV - Data Completeness).
The completeness test concept based on these mappings provides a general framework, which can easily be adapted to additional or changing use cases and information requirements. The basic semantic components of the concept are 1) the “use case” and 2) a list of endpoints and metadata needed to be able to execute this use case. As the NanoCommons Knowledge Base treats every NM and endpoint measurements as an atomistic, connected concept, the completeness check occurs on the level of available information for a specific NM rather than the historic legacy level of individual datasets. In this way, completeness is defined by the full set of available information independent of the history of different datasets providing parts of information.
Conceptually the following query is presented to the system:
For a given set of user-selected NMs (e.g., all TiO2 anatase below diameter 10 nm) show the completeness of the sum of available data for a certain use case (e.g., nanoform identification).
The system then queries which endpoints have been defined for the selected use case (here: core compound, coating compound, functionalisation, crystallinity, surface area and shape, see Supplementary Material SV). For each of those endpoints it queries for each of the selected NMs whether at least one measurement is available. The result of such a completeness check can be reported graphically as well as numerically (Figure 8).
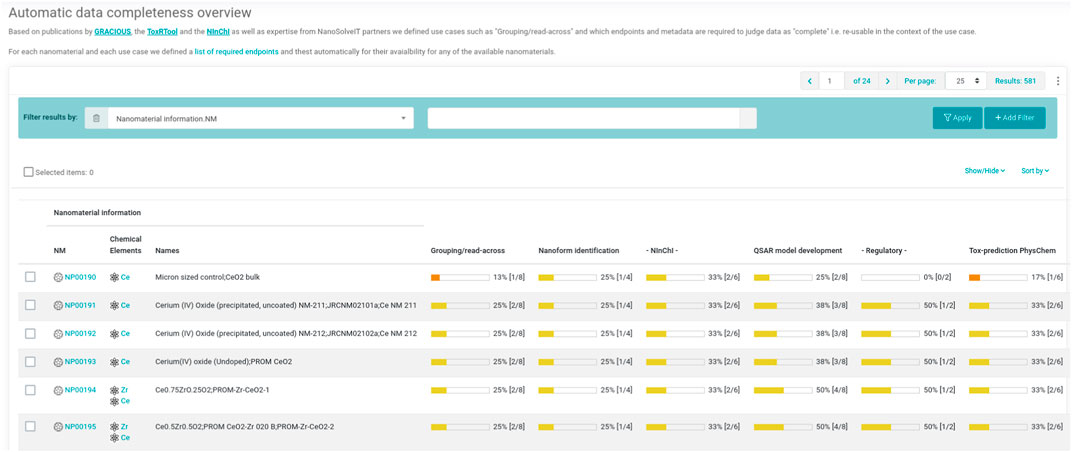
FIGURE 8. Visualisation of data completeness for given NMs and a set of different use cases (in columns). Length of bar indicates fraction of completeness, colour indicates quartile (red almost incomplete, green complete). Numbers in parentheses provide a fraction of the required endpoints with measurement data.
5.2.3 NanoXtract - image analysis
NanoXtract is an advanced online tool that has been specifically designed to analyse TEM images of NMs [37]. NanoXtract has been complemented with an API, which has been linked to the NanoCommons Knowledge base. In this way, it is possible for images uploaded to the Knowledge Base to be automatically uploaded to the tool, analysed, and retrieve the results. The tool enables researchers to determine a variety of nanomaterial’s properties, such as their size, shape, and distribution within a given image. These are calculated as image descriptors, i.e., numerical values assigned to various specific characteristics of a NMs’ TEM image. In the context of nanoscience, understanding properties such as size and shape is of paramount importance. NMs behave in ways that are largely governed by their physical characteristics. Therefore, by using the descriptors generated by NanoXtract, researchers can gain insights into the potential behaviour or effects of these NMs. NanoXtract operates on the Enalos Cloud Platform, a platform that provides a host of chemoinformatics and nanoinformatics services. Additionally, NanoXtract is integrated into the NanoCommons Knowledge Base, thereby enabling direct integration of its output with other information on the concerned NMs and other tools relevant to nanoinformatics. One of the key features of NanoXtract is its user-friendly interface. It allows users to upload a TEM image of a nanomaterial, refine the analysis parameters and receive a set of 18 image descriptors. This straightforward process makes the tool accessible to users of varying levels of expertise, and it simplifies the process of deriving valuable information from TEM images of NMs.
The zeta potential model [37], in conjunction with the NanoXtract nanodescriptors, provides an innovative approach to predicting the zeta potential of NMs. It is a read-across model, which is a method used in various fields of science and technology for making predictions about certain properties based on existing knowledge about similar substances or materials. The zeta potential is an essential parameter, which is based on the surface charge and potential surface coating or functionalisation of NMs. Zeta potential influences the NMs electrostatic stability, their tendency to agglomerate, and their interaction with cellular membranes. These factors play a crucial role in determining the overall behaviour and potential toxicity of NMs within a biological system. Τhe read-across model leverages the data provided by NanoXtract to align NMs by their core similarities, offering a comparative basis to extrapolate data from known NMs to unknown ones. The key technique utilised by the read-across model is the nearest neighbours approach, an algorithm that groups objects based on their similarity in certain properties. In this case, it groups NMs according to their similarity in specific image descriptors (i.e., the main elongation as extracted via NanoXtract), core composition, as well as the pH of the solution in which the NMs are dispersed. Subsequently, it predicts the zeta potential of a target NM by taking the average zeta potential of its “neighbours”, i.e., other NMs that are similar in terms of the image descriptors and core composition for a given pH. This model was developed and validated using a dataset comprising 63 different NMs. These NMs varied in their core compositions, shapes, and sizes, thereby offering a diverse dataset for the development and testing of the model. The model’s predictions were found to correlate closely with the actual measured zeta potentials of the NMs in the dataset, validating its accuracy and reliability.
5.2.4 NanoCommons Enalos KNIME nodes
The NanoCommons Enalos KNIME nodes offer unprecedented access to nanoinformatics tools and resources (developed within NanoCommons and related projects), providing researchers with the capabilities to handle the challenges of big data in nanotechnology. The integration into user-friendly workflows opens avenues for streamlining the research process, enhancing data reproducibility, and promoting open science in the nanotechnology community. The continuous development and refinement of such tools will be crucial in harnessing the full potential of nanoinformatics, driving scientific discovery and innovation in nanotechnology in collaboration with other EU projects (Figure 9).
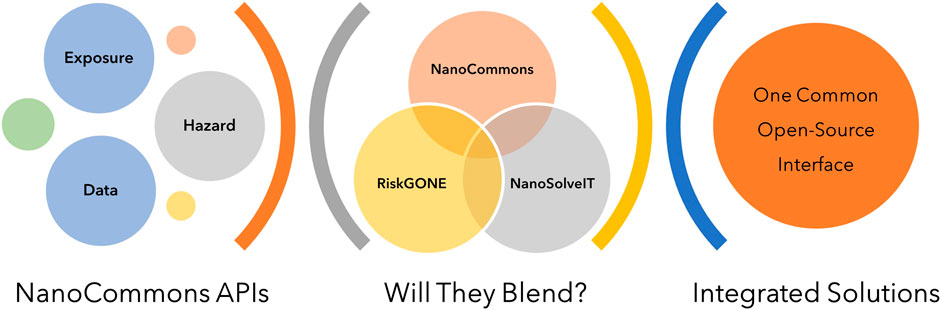
FIGURE 9. Integration of NanoCommons tools with nanoinformatics tools developed nodes by other H2020 projects, which will be maintained and further extended with tools developed in Horizon Europe projects.
The integration of NanoCommons tools with nanoinformatics tools developed by other H2020 projects, such as NanoSolveIT and RiskGONE, demonstrates the power of collaborative efforts to advance the field of nanotechnology. This integrative effort is facilitated by the Enalos KNIME nodes, which serve as the central connecting point between the different project tools. NanoCommons provides a wealth of resources, tools, and services related to nanoinformatics. Its tools are designed to facilitate data management, processing, analysis, and mining, as well as modelling and safe-by-design procedures. The goal is to enhance the reproducibility and reusability of NMs data for the wider scientific community. NanoSolveIT, on the other hand, offers an innovative in silico Integrated Approach to Testing and Assessment (IATA) for NMs. NanoSolveIT focuses on predictive modelling to support nanomaterial grouping and risk assessment. RiskGONE aims to develop a robust framework for risk governance for engineered NMs. Its focus is on evaluating, optimising, and pre-validating standard operating procedures and test guidelines, which are integrated into a risk governance framework. The Enalos KNIME nodes provide the technical means to link these distinct yet complementary tools together, promoting a holistic approach to nanoinformatics. The nodes serve as connectors, enabling users to create complex, automated workflows for data management, processing, and modelling purposes. Through these KNIME nodes, users can access and utilise the unique tools provided by each of these projects in a seamless, integrated manner. This synergy brings together the strengths of each individual project and presents them in a unified platform, offering unprecedented capabilities for nanotechnology research and development.
NanoCommons KNIME nodes (Table 1) represent a comprehensive toolkit for nanoinformatics, offering capabilities for image analysis, data retrieval, and predictive modelling of NMs. The integration of these nodes enables a seamless workflow from data acquisition to data application, thereby promoting efficiency and productivity in nanotechnology research. NanoCommons KNIME nodes are a fundamental part of the NanoCommons infrastructure providing a bridge to connect the different data management, analysis, and modelling tools developed by NanoCommons and related projects. They encompass three main categories: Image Analysis Nodes, Knowledge Base Nodes, and Model Nodes, each with its unique role in nanoinformatics.
1. Image Analysis Nodes: These nodes are primarily designed to analyse NMs’ TEM images. One of the main tools in this category is the NanoXtract tool, which allows users to calculate up to 18 image descriptors encoding geometrical characteristics of the depicted NMs (see Supplement “NanoXtract Knime node”). These descriptors include the shape, size, distribution, and other properties of the NMs, thereby facilitating in-depth exploration of the NMs’ characteristics and their potential effects. The user-friendly nature of the Image Analysis Nodes, combined with their capacity to generate essential data about NMs from TEM images, makes them a valuable asset for nanotechnology researchers.
2. Knowledge Base Nodes: These nodes provide a direct connection to the NanoCommons Knowledge Base, an extensive repository of nanoinformatics data and tools. They allow users to access and retrieve various types of data for NMs, including microscopy images, physicochemical data, toxicity data, and more. Some nodes enable users to query data for specific NMs, while others return a list of NMs that meet certain criteria, such as having available toxicity data or images. The Knowledge Base Nodes also provide information about the datasets and organisations contributing to the Knowledge Base. These nodes are crucial for managing and utilising the wealth of nanoinformatics data available in the NanoCommons Knowledge Base.
3. Model Nodes: These nodes provide access to various models developed as part of the NanoCommons project or related projects. For instance, the CNT SMILES and CNT sdf nodes give access to a predictive Quantitative Nanostructure-Activity Relationship (QNAR) model correlating molecular descriptors of decorating molecules on multi-walled carbon nanotubes with their toxicity and biological activity. Another example is the Zeta potential node, which enables the prediction of a nanomaterial’s zeta potential based on its core type, main elongation, and the medium’s pH value. Model Nodes, therefore, serve a vital role in applying the data obtained from Image Analysis Nodes and Knowledge Base Nodes to predict specific properties of NMs.
5.2.5 Protein adsorption model
The adsorption energy between proteins and NPs is expected to be significantly useful for a wide variety of use cases, but is difficult to calculate purely from first principles. A model based on the UnitedAtom methodology has been previously developed which allows for the prediction of the binding affinity of proteins to nanoparticles given an input protein structure and a set of interaction potentials for the NM surface to the set of twenty standard amino acids [38]. The NanoCommons Knowledge Base implements an interface to this model which allows for calculating these binding energies given a protein specified by their UniProtID or PDB accession number and an NP of specified material, radius and zeta potential. The generated output is the orientation-specific binding energies of the protein to the NM, given in both tabular form and a graphical heat-map representation of this data.
5.2.6 Integrative use of data and tools - evaluation of model predictions
The computational model predicting the adsorption strength and preferred orientation of a protein of interest to a NM of interest has been evaluated experimentally [39]. To this end, users uploaded NMs including TEM images, used the connected NanoXtract tool to determine NM size and shape (which are automatically stored in the NanoCommons Knowledge Base) and submitted the corresponding information to the protein adsorption model (selecting available 3D protein data from the integrated PDB database). The resulting adsorption strength predictions were then matched to extended experimental data and metadata. For this use case extended experimental data and metadata upload functions were implemented which are deemed highly specific and therefore currently available upon request only).
6 Discussion and conclusion
As hopefully substantiated in the results section, the original aim of The NanoCommons, to make available and connect as much existing NM specific information as possible and to integrate this information with tools for analysis and modelling, has been achieved. The NanoCommons Knowledge Base provides a semantic and technical interoperability framework, which enables data source providers to gain visibility while avoiding the need for data transfer and synchronisation. Users searching for data get an integrated view centred around individual NMs and can quickly judge which data sources to access.
To enable diverse user groups, from wet-lab experimentalists to data scientists and nanoinformaticians, to access the data, the NanoCommons Knowledge Base provides a graphical user interface as well as an application programming interface. Overall usage and breadth of active stakeholder groups seem to confirm the success of this bimodal access strategy.
Real-world usefulness of the NanoCommons Knowledge Base was also confirmed by enabling the successful execution of an important experimental validation of the nanomaterial protein-corona prediction model without the need for dedicated nanoinformatician support. This use-case especially emphasises the benefit of combining API and graphical user interfaces to bring specialist tools to a broad applier user group. The analysis of experimental data (images) is based on an image analysis tool integrated by API with the data management part of the NanoCommons Knowledge Base, as is the computational model predicting the NM protein corona which also uses the API-integrated public database of protein 3D-structures. At the same time users can access these analysis results and predictions, add data and edit information interactively via the graphical user interface. This ecosystem of virtual data, tool and interactive use integration exemplifies the envisioned infrastructure of The NanoCommons and its relevance and usefulness.
Overall, the NanoCommons Knowledge Base successfully addresses two of the main barriers and gaps identified in the EU-US Nanoinformatics roadmap, namely, overcoming limited data sets by combining and therefore completing multi-source data on the level of NMs, and addresses limited data access by providing open access, easy to use graphical user interfaces as well as a technical application programming interface. By integrating data from different projects, databases/data warehouses and providing visualisation tools to query and compare the data from disparate sources, the NanoCommons Knowledge Base provides a one-stop shop and the basis on which to continue building the democratisation of access to nanosafety data and nanoinformatics tools, via a “Commons” approach that is agnostic to the underpinning hardware.
Despite these successes in providing a resource as broadly useful as possible for an as broad as possible stakeholder base and despite the large amount of effort contributed to the NanoCommons Knowledge Base from many sides, this can only be the beginning of a European nanoinformatics infrastructure and it can only be a small (but hopefully important) part of it. While a large number of datasets and some data management systems have been integrated these still only represent a fraction of the over 60 EU nanomaterial safety projects executed in recent years. In addition the integrated tools and analysis methods only represent examples out of an incredibly broad tools environment (e.g., Enalos, Jaqpot, as described in [40–42]).
Due to the ongoing support by existing projects such as NanoSolveIT or PANORAMIX maintenance and some further extensions of the NanoCommons Knowledge Base are possible. Currently, plans are in place to extend the existing tools, for example, adding additional use cases such as the GRACIOUS IATA [43] to the completeness check or extending the protein adsorption model to a full protein corona prediction model, integrate with further tools such as the GRACIOUS grouping [44] and add convenience features such as SAML/OAuth2 based authentication. In addition, contributions from other projects regarding data, data management systems or tools can still be supported. As part of ongoing developments in the community for a common nanoinformatics infrastructure the NanoCommons Knowledge Base will need to continue to integrate with other and newly developing infrastructures such as the RiskGone portal. Evaluation and consideration of the potential for transfer of the tools and services to the EOSC infrastructure were also undertaken.
6.1 Sustainability as a community infrastructure
The NanoCommons approach will first and foremost need the acceptance and the support by the community it aims to serve. Only if ongoing and new projects are prepared to contribute with tools, resources and support for maintenance, will it be possible to take the next step towards an EU research community infrastructure for NMs safety and sustainability by design. While initiatives such as EOSC do provide the hardware/cloud to run the infrastructure on, they neither contribute dedicated Nanoinformatics tools nor manpower and expertise. On the bright side, new research projects making use of the developing nanoinformatics infrastructure, rather than having to assign an infrastructure WP or task in each new project, would be able to focus on their vested tools and set aside some budget to contribute to the maintenance of the overall NanoCommons. Consideration is needed in the long-term to understand whether continued contribution from projects, potentially organised in bottom-up networks such as the [45, 46]), some sort of non-for-profit foundation with industry contribution, a European Research Infrastructure Consortium, a mixture of all or some of these, or indeed another option that has not yet emerged is the optimal approach to sustain the community infrastructure. In any case, the acceptance, interest and willingness to use and support of the Research community will be the key for any of these options.
Data availability statement
The original contributions presented in the study are included in the article/Supplementary Material, further inquiries can be directed to the corresponding authors.
Author contributions
DM: Conceptualization, Formal Analysis, Investigation, Methodology, Resources, Software, Supervision, Visualization, Writing–original draft. TE: Conceptualization, Formal Analysis, Writing–original draft, Writing–review and editing, Methodology. AP: Data curation, Formal Analysis, Methodology, Writing–review and editing. AAm: Formal Analysis, Writing–review and editing. AT: Methodology, Writing–review and editing, Resources, Software. PD: Methodology, Resources, Software, Formal Analysis, Writing–review and editing. IR: Methodology, Resources, Software, Writing–review and editing. LS: Methodology, Writing–original draft. GG: Methodology, Writing–review and editing. NJ: Data curation, Writing–review and editing, Methodology. HI: Writing–review and editing, Methodology, Resources. MZ: Methodology, Data curation, Writing–review and editing, Investigation. BG: Data curation, Methodology, Writing–review and editing. SK: Data curation, Methodology, Writing–review and editing. DJ: Data curation, Writing–review and editing. LW: Data curation, Writing–review and editing. CS: Supervision, Writing–review and editing. HS: Methodology, Writing–original draft, Software. VL: Data curation, Methodology, Writing–review and editing. MH: Data curation, Visualization, Writing–review and editing. JR: Writing–review and editing, Resources. LW: Methodology, Writing–review and editing. JM: Software, Resources, Writing–review and editing. EW: Methodology, Software, Supervision, Writing–original draft. GM: Data curation, Investigation, Methodology, Writing–review and editing. AAf: Conceptualization, Data curation, Formal Analysis, Funding acquisition, Project administration, Supervision, Validation, Writing–original draft, Writing–review and editing. IL: Conceptualization, Data curation, Funding acquisition, Methodology, Resources, Software, Supervision, Writing–original draft.
Funding
The author(s) declare financial support was received for the research, authorship, and/or publication of this article. European Commission and UKRI Guarantee Fund for Horizon Europe. Funding from the following Grants enabled the presented results: EU H2020 research infrastructure for nanosafety project NanoCommons (Grant Agreement No. 731032), EU H2020 nanoinformatics project NanoSolveIT (Grant Agreement No. 814572), EU H2020 NMs safety project NanoFASE (Grant Agreement No. 646002), EU FP7 NMs safety project NanoMILE (Grant Agreement No. 310451), EU H2020 nanomaterial safety project SmartNanoTox (Grant Agreement No. 686098), EU H2020 nanomaterial characterisation project NanoPAT (Grant Agreement No. 862583) and EU H2020 GreenDeal chemical mixture safety project PANORAMIX (Grant Agreement No. 101036631). Additional support was provided by the Horizon Europe project WorldFAIR (Grant Agreement No. 101058393) and the Innovate UK support for UoB participation in WorldFAIR (Grant No. 1831977).
Acknowledgments
We thank Denise Slenter for her contributions to the eNMO project.
Conflict of interest
Authors DM, HI, MZ, BG, and SK are employed by Labvantage - Biomax GmbH, Author TE is employed by Seven Past Nine GmbH, Authors AT, AP, and AAf are employed by NovaMechanics Ltd., Authors LS, GG, NJ is employed by Ideaconsult Ltd. and Authors DJ, GG is employed by TEMAS Solutions GmbH.
The remaining authors declare that the research was conducted in the absence of any commercial or financial relationships that could be construed as a potential conflict of interest.
Publisher’s note
All claims expressed in this article are solely those of the authors and do not necessarily represent those of their affiliated organizations, or those of the publisher, the editors and the reviewers. Any product that may be evaluated in this article, or claim that may be made by its manufacturer, is not guaranteed or endorsed by the publisher.
Supplementary material
The Supplementary Material for this article can be found online at: https://www.frontiersin.org/articles/10.3389/fphy.2023.1271842/full#supplementary-material
References
1. Reidy B, Haase A, Luch A, Dawson KA, Lynch I. Mechanisms of silver nanoparticle release, transformation and toxicity: a critical review of current knowledge and recommendations for future studies and applications. Materials (Basel) (2013) 6(6):2295–350. doi:10.3390/ma6062295
2. Miernicki M, Hofmann T, Eisenberger I, von der Kammer F, Praetorius A. Legal and practical challenges in classifying nanomaterials according to regulatory definitions. Nat Nanotechnol (2019) 14:208–16. doi:10.1038/s41565-019-0396-z
3. Isigonis P, Afantitis A, Antunes D, Bartonova A, Beitollahi A, Bohmer N, et al. Risk governance of emerging technologies demonstrated in terms of its applicability to nanomaterials. Small (2020) 16(36):e2003303. doi:10.1002/smll.202003303
4. Miettinen M “By Design” and risk regulation: insights from nanotechnologies. European Journal of Risk Regulation (2021):1–17. doi:10.1017/err.2020.58
5. ECHA. Appendix for nanoforms applicable to the guidance on registration and substance identification. Version 2.0 (2022). Available at: https://echa.europa.eu/documents/10162/13655/how_to_register_nano_en.pdf/f8c046ec-f60b-4349-492b-e915fd9e3ca0 (Accessed May 02, 2023).
6. Haase and Klaessig. EU US roadmap nanoinformatics 2030 (2018). Available at: https://zenodo.org/record/1486012 (Accessed October 26, 2023).
7. Karcher S, Willighagen EL, Rumble J, Ehrhart F, Evelo CT, Fritts M, et al. Integration among databases and data sets to support productive nanotechnology: challenges and recommendations. NanoImpact (2018) 9:85–101. doi:10.1016/j.impact.2017.11.002
8. Serrano BA, Gheorghe LC, Exner TE, Resch S, Wolf C, Himly M, et al. The role of FAIR nanosafety data and nanoinformatics in achieving the UN Sustainable Development Goals: the NanoCommons experience. Submitted to RSC Sustainability (2023).
9. Bastow R, Leonell S. Sustainable digital infrastructure. Although databases and other online resources have become a central tool for biological research, their long-term support and maintenance is far from secure. EMBO Rep (2010) 11(10):730–4. doi:10.1038/embor.2010.145
10. Gabella C, Durinx C, Appel R. Funding knowledgebases: towards a sustainable funding model for the UniProt use case. F1000Res (2017) 6:ELIXIR–2051. doi:10.12688/f1000research.12989.2
11. Weise M, Kovacevic F, Popper N, Rauber A. OSSDIP: open source secure data infrastructure and processes supporting data visiting. Data Sci J (2022) 21(1):4. doi:10.5334/dsj-2022-004
12. Papadiamantis AG, Klaessig FC, Exner TE, Hofer S, Hofstaetter N, Himly M, et al. Metadata stewardship in nanosafety research: community-driven organisation of metadata schemas to support FAIR nanoscience data. Nanomaterials (2020) 10:2033. doi:10.3390/nano10102033
13. Maier D, Kalus W, Wolff M, Kalko SG, Roca J, de Mas IM, et al. Knowledge management for systems biology a general and visually driven framework applied to translational medicine. BMC Syst Biol (2011) 5:38. doi:10.1186/1752-0509-5-38
14. Chang JK, Willighagen E, Winckers L, Uana F, Tancheva G, Ehrhart F, et al. eNanoMapper/ontologies: release 7 of the eNanoMapper ontology (2021). Available at: https://zenodo.org/record/4600986/export/hx (Accessed February 4, 2021).
15. Buttigieg PL, Morrison N, Smith B, Mungall CJ, Lewis SEENVO Consortium The environment ontology: contextualising biological and biomedical entities (2013) 4:43. doi:10.1186/2041-1480-4-43
16. Gkoutos GV, Schofield PN, Robert Hoehndorf R. The anatomy of phenotype ontologies: principles, properties and applications. Brief Bioinform (2018) 19:1008–21. doi:10.1093/bib/bbx035
17. Bolton EE, Wang Y, Thiessen PA, Bryant SH. Chapter 12 PubChem: integrated platform of small molecules and biological activities. Elsevier (2008). p. 217–41. doi:10.1016/S1574-1400(08)00012-1
18. van Rijn J, Afantitis A, Culha M, Dusinska M, Exner TE, Jeliazkova N, et al. European Registry of Materials: global, unique identifiers for (undisclosed) nanomaterials J Cheminformatics (2022) 14:57. doi:10.1186/s13321-022-00614-7
19. Lynch I, Afantitis A, Exner T, Himly M, Lobaskin V, Doganis P, et al. Can an InChI for nano address the need for a simplified representation of complex nanomaterials across experimental and nanoinformatics studies? Nanomaterials (2020) 10:2493. doi:10.3390/nano10122493
20. NanoInChI Working group. Nanomaterials. Avaialable at: https://www.inchi-trust.org/inchi-working-groups/#nano (Accessed November 01, 2023).
21. Baer DR, Munusamy P, Thrall BD. Provenance information as a tool for addressing engineered nanoparticle reproducibility challenges. Biointerphases (2016) 11:04B401. doi:10.1116/1.4964867
22. Izak-Nau E, Huk A, Reidy B, Uggerud H, Vadset M, Eiden S, et al. Impact of storage conditions and storage time on silver nanoparticles’ physicochemical properties and implications for their biological effects. RSC Adv (2015) 5:84172–84185. doi:10.1039/C5RA10187E
23. Baer DR. The chameleon effect: characterization challenges due to the variability of nanoparticles and their surfaces. Front Chem (2018) 6:145. doi:10.3389/fchem.2018.00145
24. Tauc J. Optical properties and electronic structure of amorphous Ge and Si. Mater Res Bull (1968) 3:37–46. doi:10.1016/0025-5408(68)90023-8
25. Saarimäki LA, Federico A, Lynch I, Papadiamantis AG, Tsoumanis A, Melagraki G, et al. Manually curated transcriptomics data collection for toxicogenomic assessment of engineered nanomaterials. Sci Data (2021) 8:49. doi:10.1038/s41597-021-00808-y
26. Bard J, Seung Y, Rhee Y, Ashburner M. An ontology for cell types. Genome Biol (2005) 6:R21. doi:10.1186/gb-2005-6-2-r21
27. Terminology Harmonizer. Terminology harmonizer. Available at: https://terminology-harmonizer.greendecision.eu/login (Accessed October 26, 2023).
28. Jeliazkova N, Apostolova MD, Andreoli C, Barone F, Barrick A, Bossa C, et al. Towards FAIR nanosafety data. Nat Nanotechnol (2021) 16:644–54. doi:10.1038/s41565-021-00911-6
29. Jeliazkova N, Chomenidis C, Doganis P, Fadeel B, Grafström R, Hardy B, et al. The eNanoMapper database for nanomaterial safety information. Beilstein J Nanotechnol (2015) 6:1609–34. doi:10.3762/bjnano.6.165
30. Schüürman J, Micić V, von der Kammer F, Hofmann T. Fly ash releases from surface impoundments can be identified through spICP-TOF-MS fingerprinting. Vienna, Austria: EGU General Assembly 2022 (2022). EGU22-826. doi:10.5194/egusphere-egu22-826
31. Exner TE, Papadiamantis AG, Melagraki G, Amos JD, Bossa N, Gakis G, et al. Metadata stewardship in nanosafety research: learning from the past, preparing for an “on-the-fly” FAIR future. Front. Phys. (2023) 11. doi:10.3389/fphy.2023.1233879
32. NanoCommons User Guidance Handbook. NanoCommons user guidance Handbook. Available at: https://nanocommons.github.io/user-handbook/((continuously developed) and April 2022 static release on Zenodo: https://zenodo.org/record/6504273.
33. Cano I, Tényi A, Schueller C, Wolff M, Migueláñez MMH, Gomez-Cabrero D, et al. The COPD Knowledge Base: enabling data analysis and computational simulation in translational COPD research. J Translational Med (2014) 12:S6. doi:10.1186/1479-5876-12-S2-S6
34. Comandella D, Gottardo S, Rio-Echevarria IM, Hubert Rauscher H. Quality of physicochemical data on nanomaterials: an assessment of data completeness and variability. Nanoscale (2020) 12:4695–708. doi:10.1039/C9NR08323E
35. Schneider K, Schwarz M, Burkholder I, Kopp-Schneider A, Edler L, Kinsner-Ovaskainen A, et al. ‘ToxRTool’, a new tool to assess the reliability of toxicological data. Toxicol Lett (2009) 189:138–44. doi:10.1016/j.toxlet.2009.05.013
36. ECHA. ECHA guideline on QSAR information requirements and read-across (2017). https://echa.europa.eu/documents/10162/13628/raaf_en.pdf/614e5d61-891d-4154-8a47-87efebd1851a (Accessed October 26, 2023).
37. Varsou D-D, Afantitis A, Tsoumanis A, Papadiamantis A, Valsami-Jones E, Lynch I, et al. Zeta-potential read-across model utilizing nanodescriptors extracted via the NanoXtract image analysis tool available on the enalos nanoinformatics cloud platform. Small (2020) 16:1906588. doi:10.1002/smll.201906588
38. Power D, Rouse I, Poggio S, Brandt E, Lopez H, Lyubartsev A, et al. A mulsticale model of protein adsorption on a nanoparticle surface. Model Simulation Mater Sci Eng (2019) 27:8. doi:10.1088/1361-651X/ab3b6e
39. Hasenkopf I, Mills-Goodlet R, Johnson L, Rouse I, Geppert M, Duschl A, et al. Computational prediction and experimental analysis of the nanoparticle-protein corona: showcasing an in vitro-in silico workflow providing FAIR data. Nano Today (2022) 46:101561. doi:10.1016/j.nantod.2022.101561
40. Chomenidis C, Drakakis G, Tsiliki G, Anagnostopoulou E, Valsamis A, Doganis P, et al. Jaqpot quattro: a novel computational web platform for modeling and analysis in nanoinformatics. J Chem Inf Model (2017) 57(9):2161–72. doi:10.1021/acs.jcim.7b00223
41. Afantitis A, Tsoumanis A, Melagraki G. Enalos suite of tools: enhancing cheminformatics and nanoinformatics through KNIME. Curr Med Chem (2020) 27(38):6523–35. doi:10.2174/0929867327666200727114410
42. Papadiamantis AG, Afantitis A, Tsoumanis A, Valsami-Jones E, Lynch I, Melagraki G. Computational enrichment of physicochemical data for the development of a ζ-potential read-across predictive model with Isalos Analytics Platform. NanoImpact (2021) 22:100308. doi:10.1016/j.impact.2021.100308
43. Braakhuis HM, Murphy H, Ma-Hock L, Dekkers S, Keller J, Oomen AG, et al. An integrated approach to testing and assessment to support grouping and read-across of nanomaterials after inhalation exposure. Appl Vitro Toxicol (2021) 7:112–28. doi:10.1089/aivt.2021.0009
44. Stone V, Gottardo S, Bleeker EAJ, Braakhuis H, Dekkers S, Fernandes T, et al. A framework for grouping and read-across of nanomaterials-supporting innovation and risk assessment. Nano Today (2020) 35:100941. doi:10.1016/j.nantod.2020.100941
45. AdvancedNano GO FAIR Implementation network. AdvancedNano GO FAIR implementation network. Avaialable at: https://www.go-fair.org/implementation-networks/overview/advancednano/ (Accessed October 26, 2023).
Keywords: nanosafety, materials modelling, nanoinformatics, safe and sustainable by design, FAIR data, semantic knowledge graphs, data infrastructure, data democratisation
Citation: Maier D, Exner TE, Papadiamantis AG, Ammar A, Tsoumanis A, Doganis P, Rouse I, Slater LT, Gkoutos GV, Jeliazkova N, Ilgenfritz H, Ziegler M, Gerhard B, Kopetsky S, Joshi D, Walker L, Svendsen C, Sarimveis H, Lobaskin V, Himly M, van Rijn J, Winckers L, Millán Acosta J, Willighagen E, Melagraki G, Afantitis A and Lynch I (2023) Harmonising knowledge for safer materials via the “NanoCommons” Knowledge Base. Front. Phys. 11:1271842. doi: 10.3389/fphy.2023.1271842
Received: 03 August 2023; Accepted: 19 October 2023;
Published: 13 November 2023.
Edited by:
Surya K. Ghosh, National Institute of Technology Warangal, IndiaReviewed by:
Paola Italiani, National Research Council (CNR), ItalyPritam Mandal, Michigan Technological University, United States
Copyright © 2023 Maier, Exner, Papadiamantis, Ammar, Tsoumanis, Doganis, Rouse, Slater, Gkoutos, Jeliazkova, Ilgenfritz, Ziegler, Gerhard, Kopetsky, Joshi, Walker, Svendsen, Sarimveis, Lobaskin, Himly, van Rijn, Winckers, Millán Acosta, Willighagen, Melagraki, Afantitis and Lynch. This is an open-access article distributed under the terms of the Creative Commons Attribution License (CC BY). The use, distribution or reproduction in other forums is permitted, provided the original author(s) and the copyright owner(s) are credited and that the original publication in this journal is cited, in accordance with accepted academic practice. No use, distribution or reproduction is permitted which does not comply with these terms.
*Correspondence: Dieter Maier, cmVzZWFyY2hAaW5zdGl0dXQtbWFpZXIuZGU=; Iseult Lynch, aS5seW5jaEBiaGFtLmFjLnVr