- 1Department of Mathematics, Faculty of Science, Al Balqa Applied University, As-Salt, Jordan
- 2Department of Mathematics, Faculty of Science, The University of Jordan, Amman, Jordan
This article proposes a new approach to solving fuzzy M-fractional integrodifferential models under strongly generalized differentiability using an innovative formulation of the characterization principle. The study presents theoretical effects on the existence-uniqueness of fuzzy two M-solutions and, thus, showcases the solvability of the fuzzy Volterra models. Moreover, the study offers numerical solutions using the Hilbert reproducing kernel algorithm in a new fuzzy look, utilizing two fitting Hilbert spaces. The proposed models and algorithms are under scrutiny, with particular attention given to the analysis of the series solution, the assessment of convergence, and the evaluation of error. The debated Hilbert approach is shown to be effective in solving several fractional Volterra problems under uncertainty, and the numerical impacts manifest the accuracy and competence of the algorithm. Overall, our work contributes to the advancement of mathematical tools for solving complex fractional Volterra problems under uncertainty and shows potential to impact various fields of science and engineering, as depicted in the utilized figures, tables, and comparative analysis. The findings of the study are evaluated based on the analysis conducted, and a numerical algorithm is presented in the final section, along with several suggestions for future research directions.
1 Introduction
In recent years, fuzzy calculus and fuzzy integrodifferential models have gained significant attention due to their ability to model complex real-world problems that are inherently imprecise or uncertain [1–3]. Fuzzy calculus extends traditional calculus by incorporating the concept of fuzzy sets, which allows for the representation of uncertain or vague data in a mathematical framework. Fuzzy integrodifferential models combine the concepts of fuzzy sets and integrodifferential equations to model systems that involve both memory and uncertainty. In contrast, fractional calculus has emerged as a mighty mathematical tool for the formation of complex systems in various fields of science and engineering [4–6]. Recently, there has been a growing fascination with extending the concepts of fractional calculus to fuzzy environments, where the parameters of the system are not precisely defined but are instead represented by fuzzy numbers. This has led to the development of fuzzy fractional calculus, which has shown promising results in modeling and analyzing complex systems under uncertainty [7–9]. In this article, we will explore the concept of fuzzy fractional calculus and its application to FM-FIDMs. We will discuss the fundamental concepts of fuzzy calculus and fractional M-calculus, and then show how the two concepts can be combined to form a powerful tool for modeling and analyzing fuzzy systems represented by Volterra patterns. We will also present some examples of FM-FIDMs and their solutions utilizing HRKA, showcasing the productivity of our approach.
HRKA is a powerful mathematical tool that has found numerous products in assorted areas of stochastics and nonlinear phenomena [10–12]. The algorithm is based on the theory of Hilbert spaces, which provides a framework for the study of functions and their properties. HRKA is particularly useful for solving problems involving function approximation, interpolation, and regression, and has been used in applications such as machine learning, signal processing, and control theory [13–22]. One of the key characteristics of HRKA is its ability to represent functions in terms of inner products, which allows for efficient computation of function values and derivatives. This property is closely related to the concept of reproducing kernels, which are positive definite functions that satisfy certain properties. The construction of the reproducing kernel is an important aspect of HRKA and involves finding a function that satisfies the reproducing property and other properties that ensure its suitability for the problem at hand.
HRKA is a powerful mathematical framework used in applied mathematics, applied physics, machine learning, and other fields [10–12]. It offers several advantages over other approaches when it comes to solving NLDMs. Some of the distinguishing features of HRKA are as follows:
1. Nonlinear modeling: HRKA can capture nonlinear relationships between variables, making it a powerful tool for modeling NLDMs.
2. Flexibility: HRKA is very flexible and can be used to model a wide range of NLDMs, including those with complex constraint conditions.
3. High-dimensional feature space: HRKA maps data points into a high-dimensional feature space, where linear methods can be used to perform nonlinear tasks. This makes it possible to solve complex NLDMs using simple successive techniques.
4. Reproducing property: HRKA has a unique property called the reproducing property, which allows the evaluation of functions in the reproducing Hilbert space at any point in the input space. This means that it can be used to interpolate solutions to NLDMs and make predictions at any point in the input space.
5. Regularization: HRKA uses regularization to control the complexity of the model and prevent overfitting. This is performed by introducing a penalty term in the objective function that penalizes large coefficients in the model.
Indeed, when using HRKA to solve NLDMs, these advantages translate into several distinct benefits.
1. Accuracy: The flexibility of HRKA allows it to accurately model complex NLDMs, producing solutions with high accuracy.
2. Efficiency: The use of high-dimensional feature space and simple successive techniques can make HRKA more computationally efficient than other methods for solving NLDMs.
3. Interpolation: The reproducing property of HRKA allows it to interpolate solutions to NLDMs, making it possible to accurately predict values at any point in the input space.
4. Regularization: The use of regularization helps prevent overfitting, producing more reliable solutions to NLDMs.
Generally, HRKA offers several advantages over other approaches when it comes to solving NLDMs. Its ability to model nonlinear relationships, flexibility, use of high-dimensional feature space, reproducing property, and regularization all contribute to its accuracy, efficiency, and ability to interpolate solutions.
This article delves into two important mathematical concepts: the existence-uniqueness and characterization theorems, and the simulated HRKA. In the first part, we explore the theorem’s significance in proving the existence-uniqueness of fuzzy two M-solutions, and we will discuss how the characterization theorem helps us provide a framework for understanding the proof. In the second part, we delve into HRKA, which is a powerful tool for analyzing numerical approximations and their properties. Through this, we will explore our requirements for the following general model:
Here,
FM-D is a relatively new concept in the field of fractional calculus. It was introduced as an innovative class of fractional derivatives that has some advantages over other fractional approaches, such as Riemann or Caputo derivatives. One of the main advantages of FM-D is that it preserves the chain rule of differentiation. This means that if we apply FM-D to a composite function, we can use the chain rule to simplify the result. This property is not shared by other fractional derivatives, which can make it difficult to apply them in practice. Another advantage of FM-D is that it is more closely related to the ordinary derivative than other fractional derivatives. In particular, it satisfies a version of the Leibniz rule, which allows us to differentiate products of functions naturally. This makes it easier to use FM-D in applications where we need to differentiate products of functions, such as in physics and engineering [23–28]. It also has some interesting mathematical properties that make it an attractive tool for studying fractional models. For example, it has been shown that FM-D can be used to obtain exact solutions for certain types of fractional models. This could be useful in applications where we need to solve NLDMs that involve fractional derivatives. Other theoretical and application results concerning fractional calculus patterns with several constraints and types can be collected from [29–35]. Overall, FM-D is a promising new tool in the branch of calculus, and it shows potential to be useful in a wide range of applications.
After the preliminary stage and the problem formulation phase, the study is structured as follows: Section 2 provides an overview of fuzzy calculus and FM-D. Section 3 introduces fuzzy FM-D as differentiation and continuity, followed by fuzzy FM-I as integration and inversion. In Section 4, we examine FM-FIDM as structures, tools, and steps, while Section 5 presents a new characterization theorem. Section 6 introduces HRKA in terms of structures and tools, and Section 7 implements HRKA as structures and tools. Section 8 showcases the numerical implementations and computed results. Finally, Section 9 presents the key points and summary of the study.
2 Outline of fuzzy calculus and FM-D
Herein, we will delve into the concept of fuzzy numbers, which are an essential tool in the fuzzy set theory. Fuzzy numbers are a generalization of traditional real numbers, allowing for uncertainty and imprecision to be incorporated into numerical values. We will explore the
Specifically, substitute
Theorem 1. [36] Presume that
For a more in-depth explanation, let
A metric
Using a pair of fuzzy functions and the
Theorem 2. [37] If
In the following paragraphs, we will explore the concept of FM-D, which is a memorization of conformable scaling derivative. We will start by defining FM-D and discussing its mathematical properties, including its relationship to classical derivatives, and its applications in various fields of study. Additionally, we will examine several related results that shed light on the behavior of FM-D and its significance in understanding the complexity of real-world phenomena.
FM-D has several tools in engineering and applied sciences [23–28]. For example, it can be used to model non-Newtonian fluids, which exhibit complex and nonlinear behaviors that cannot be described by ordinary derivatives. In addition, it can be used to simulate fractional-order systems, like electrical circuits and control systems, which exhibit memory effects and other non-ideal behaviors. However, FM-D is a generalization of the classical derivative to noninteger values of the differentiation of order
Definition 1. [23] Let
Herein,
Using the FM-D definition as our foundation, we can showcase the linearity of FM-D, as well as its adherence to fundamental rules, such as the product, composition, quotient, and chain rules for two
Definition 2. [23] Let
Next, theoretical results are employed to elucidate the relationship between FM-D and FM-I behaviors. Specifically, the inversion formula and the fundamental theorem of calculus are utilized in the sense of fractional M-calculus.
Theorem 3. [23] For
i. If
ii. If
For additional information on the FM-D, FM-I, and Mittag–Leffler parameter, including further results, historical notes, characteristics, applications, and methods, please refer to [23–28].
3 Fuzzy FM-D: differentiation and continuity
Foremost, we present the fuzzy FM-D concept, its definitions, and its properties. We utilize a new strongly generalized fuzzy FM-D delineation for a
Definition 3. Let
i.
ii.
Definition 4. Let
i.
ii.
Undoubtedly, the fuzzy FM-Ds of
Theorem 4. Let
i. If
ii. If
Proof. Here, our attention will be directed toward (i), while a comparable proof can be utilized for (ii). Assuming that
Multiplying by
Passing to the limit, we get
Theorem 5. Let
i. If
ii. If
Proof: Here, our attention will be directed toward (i), while a comparable proof can be utilized for (ii). Assuming that
Since
Take
Thus, one can formulate
Theorem 6. Let
i. If
ii. If
Proof. Here, our attention will be directed toward (i), while a comparable proof can be utilized for (ii). Assuming that
Catch the limits on both sides of Eq. 18 to obtain
Utilizing (14), one obtains
It becomes apparent that
4 Fuzzy FM-I: integration and inversion
After utilizing several fuzzy FM-D results, a new approach for the fuzzy FM-I for
In this section,
Definition 5. Assume
Theorem 7. Let
i.
ii.
Proof. First,
For part (ii) take
The results in [36] produce
Theorem 8. Let
i.
ii.
ProofFor part (i),
Thus,
The rearranging of Eq. 24 gives
Theorem 9. Let
i.
ii.
Proof. For part (i),
Thereafter,
The rearranging of Eq. 26 gives
Thus,
5 FM-FIDM: structures, steps, and tools
This section delves into the examination of existence-uniqueness outcomes for coupled fuzzy solutions associated with
5.1 FM-FIDM formalism
Applying the strongly generalized
The functional framework of FM-FIDM utilizing can be prioritized as
The
Thus, this leads to the determination of the subsequent coupled CM-FIDMs concerning
Definition 6. Let
i. If
ii. If
Algorithm 1.To construct a
Phase I. If
i. Solve
ii. Validate that
iii. Fit a
Phase II. If
i. Solve the
ii. Validate that
iii. Fit a
5.2 Existence-uniqueness of two fuzzy M-solutions
Our focus in this study is to address two main questions. First, we aim to identify the conditions under which solutions for FM-FIDM (28) exist. Second, we aim to determine under what circumstances two unique fuzzy M-solutions exist, with one solution for an individual crosswise fuzzy FM-D.z
Lemma 1. FM-FIDM (28) with
i.
ii.
This depends on
Proof. For part (i), because
Considering
This is tantamount to the format presented in part (ii) of Lemma 1. ■
From Lemma 1, one can consider
Lemma 2. Both
Proof. Since
It is important to note that the presence of a unique fixed point is assured by Lemma 2, which is relevant to the subsequent theorem. This means that a distinct fuzzy M-solution exists for (28) for every type of differentiability.
Theorem 10. Let
Then,
i. FM-FIDM (28) possesses a unique fuzzy M-solution in
ii. FM-FIDM (28) possesses a unique fuzzy M-solution in
Proof. Here, our attention will be directed toward (i), while a comparable proof can be utilized for (ii). However,
First, we want to confirm whether the hypothesis of the Banach theorem is satisfied well by
Utilize Lemma 2 and choose
Furthermore, differentiate (Eq. 38) and substitute
6 New characterization theorem
Herein, the characterization theorem suggests a general approach for solving FM-FIDM—we can convert it into a couple of CM-FIDMs, which have extensively studied solution techniques. By solving the crisp system, we can obtain solutions for the original FM-FIDM. Therefore, there is no need to rewrite the algorithms in a fuzzy setting; instead, they can be directly applied to the acquired coupled crisp equations.
An
Theorem 11. Consider FM-FIDM (33), where
i.
ii.
Then,
i. For
ii. For
Proof. Here, our attention will be directed toward (i), while a comparable proof can be utilized for (ii). However, it is assumed that
Similarly, one obtains
The continuity of
Conversely, presume that
The aim of the following results is not to significantly enhance Theorem 11 but instead to provide alternative criteria that establish the equivalence between FM-FIDM (Eq. 28) and the corresponding coupled CM-FIDMs (Eq. 30) and (Eq. 31).
Corollary 1. Consider FM-FIDM (Eq. 28), where
then,
i. For
ii. For
Proof. Here, our attention will be directed toward (i), while a comparable proof can be utilized for (ii). To achieve this objective, let us presume the hypothesis of Corollary 1. Thus, condition (ii) of Theorem 11 is valid. To prove (i) in Theorem 11, fix
The claim is to show
Since
7 HRKA: structures and tools
Although the tools of HRKA have been widely studied and operated in assorted areas of engineering and sciences [10–22], the principle of reproducing kernels continues to be extensively researched. Nonetheless, HRKA has proven to be a beneficial scheme for solving a broad range of stochastics and nonlinear equations in a fractional sense and provides a generic numerical scheme for handling solution performances.
7.1 Principles and requirements
Given the
the following requirements are essential to apply the HRKA steps:
Fundamentally,
To apply HRKA, we partition
Theorem 12.
Proof. We aim to exhibit
7.2 Illustration of the FM-FIDM solution
The HRKA methodology comprises a variety of essential elements, such as constructing Hilbert spaces that are suitable for the problem at hand, creating kernels, identifying linear operators that are appropriate, and employing Mathematica solvers. During the forthcoming, we expound on how the HRKA approach can be employed to create numerical solutions that are highly efficient for tackling FM-FIDM problems.
In our formalism, we will exclusively focus on
Set
Herein, substitute
Algorithm 2.Generating orthogonalization coefficients
Phase 1: For
Phase 2: For
Theorem 13.
Proof. If
Since
Call the term on the right of Eq. 51 and refer to it henceforth as
Theorem 14. Whenever
Proof. Initially,
Remark 1. To perform numerical computations, we truncated (Eq. 57) and generated an
7.3 Mathematical analysis: error and convergence
To analyze the habits of the HRKA solution, we derive convergence analyses and error estimates in
Theorem 15. Let
Proof. First, we will demonstrate that
where
Afterward, symbolize
Theorem 16. For (61), one obtains
Proof. Clearly,
So,
Thus, for
Because
Theorem 17. For (61),
Proof. Taking
If
Theorem 18. If
Proof. From
Using
8 Numerical implementations and computed results
The analytical formalism we have developed is not only useful for verifying the principles of HRKA but also for comparing
8.1 Steps of HRKA and applications
Promoting software packages is a crucial aspect of computational analysis in fields such as applied stochastics and nonlinear engineering. Herein, we will now discuss two applications that can be used to present our constructions. The first application is related to electrical engineering and focuses on the fuzzy IRCC. The second application incorporates a fuzzy forcing term in its nonhomogeneous part.
In Algorithm 3, we have set the number
Algorithm 3.Steps of HRKA for handling FM-FIDM in the case of
Phase I: Fix
• Set
• Set
• Set
Output:
Phase II: For
Output:
Phase III: Set
Output:
Phase IV: Set
• Set
• Set
• Set
Output:
To elaborate further, let us start by demonstrating that CM-FIDM can be naturally modeled as FM-FIDM. As evidence, we consider the crisp IRCC
By considering the ambiance fuzzy setting, we can obtain more realistic results and better detect unknown conditions in circuit analysis, as utilized in Application 1.
Application 1. We examine the fuzzy IRCC circuit concerning an AC creator:
concerning precise
Herein,
Phase 1. The coupled equation concerning
The exact
Herein,
Phase 2. The coupled equation concerning
The exact
Application 2. We examine how FM-FIDM incorporates a fuzzy forcing term in its nonhomogeneous component:
concerning precise
Herein,
Phase 1 The coupled equation concerning
The exact
Phase 2 The coupled equation concerning
The exact series
8.2 Findings and analysis
For computations concerning
The key goal is to exemplify the uncertain behaviors of the HRKA
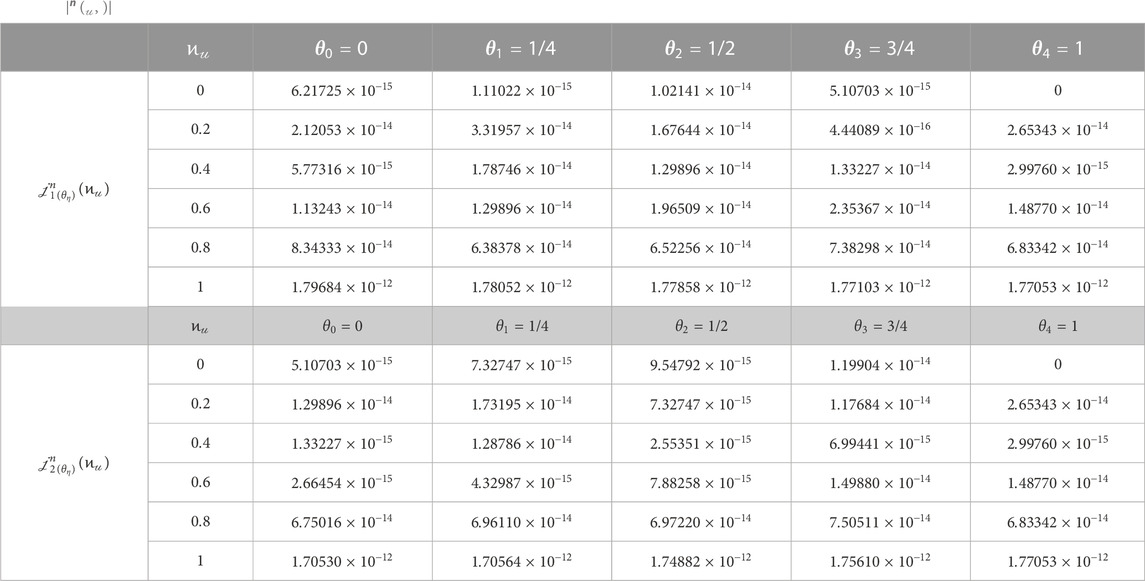
TABLE 1.
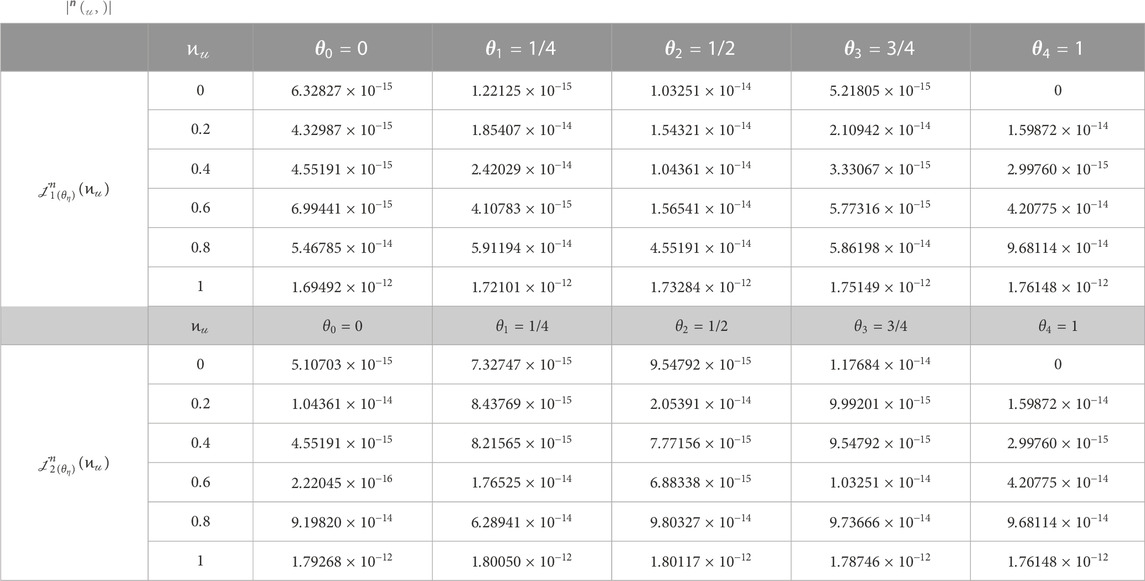
TABLE 2.
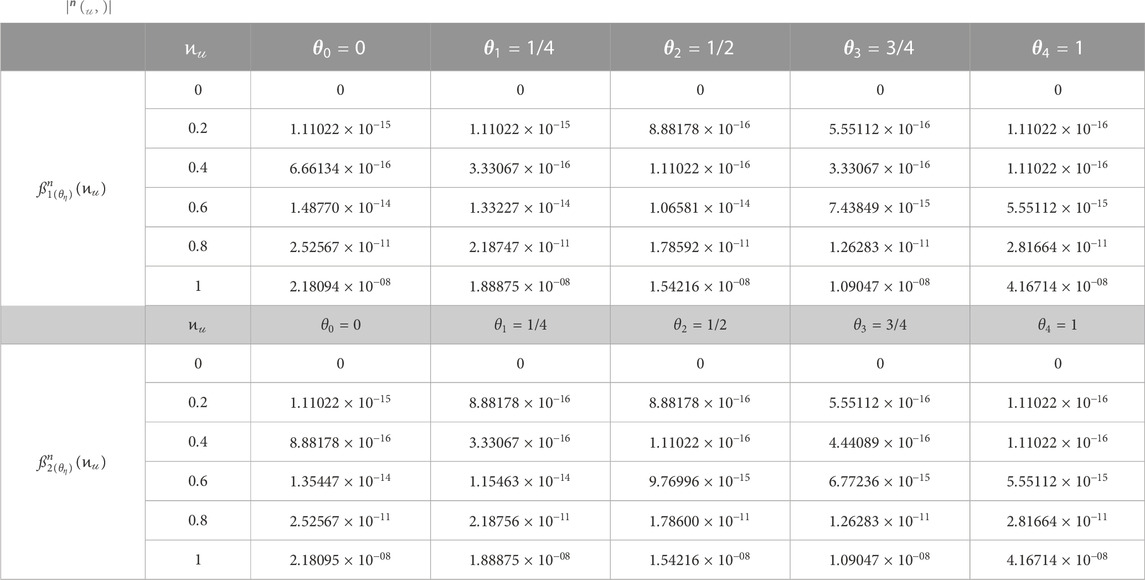
TABLE 3.
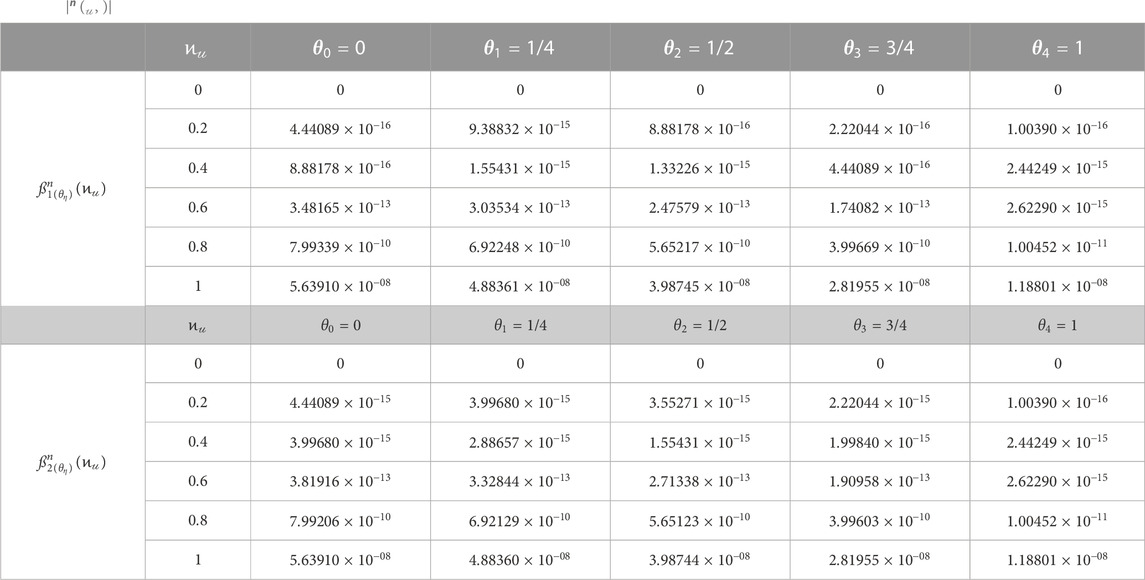
TABLE 4.
As is evident from the tabulated digits in Tables 1–4,
Our research focuses on exploring the HRKA’s vibrant and structural characteristics, and remembrance and heritage features. In pursuit of this, we provide geometric certifications for
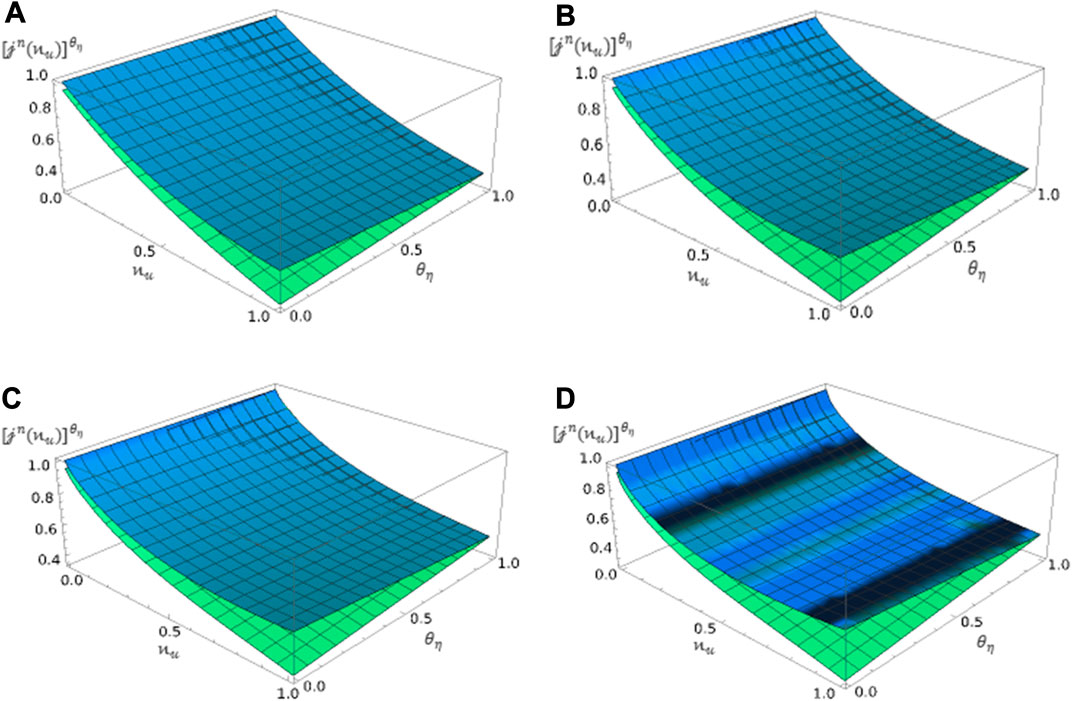
FIGURE 2. Plot of HRKA
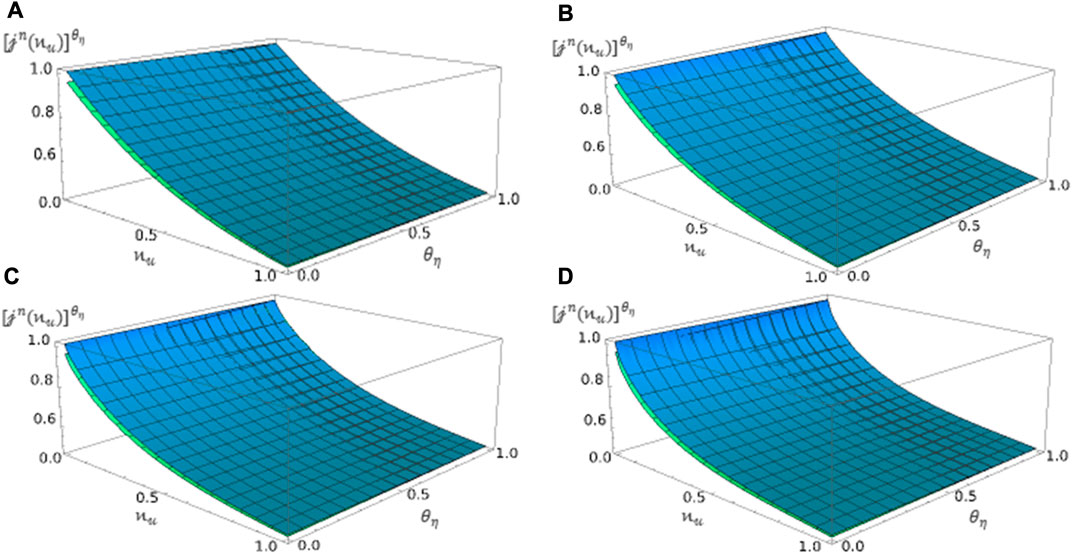
FIGURE 3. Plot of HRKA
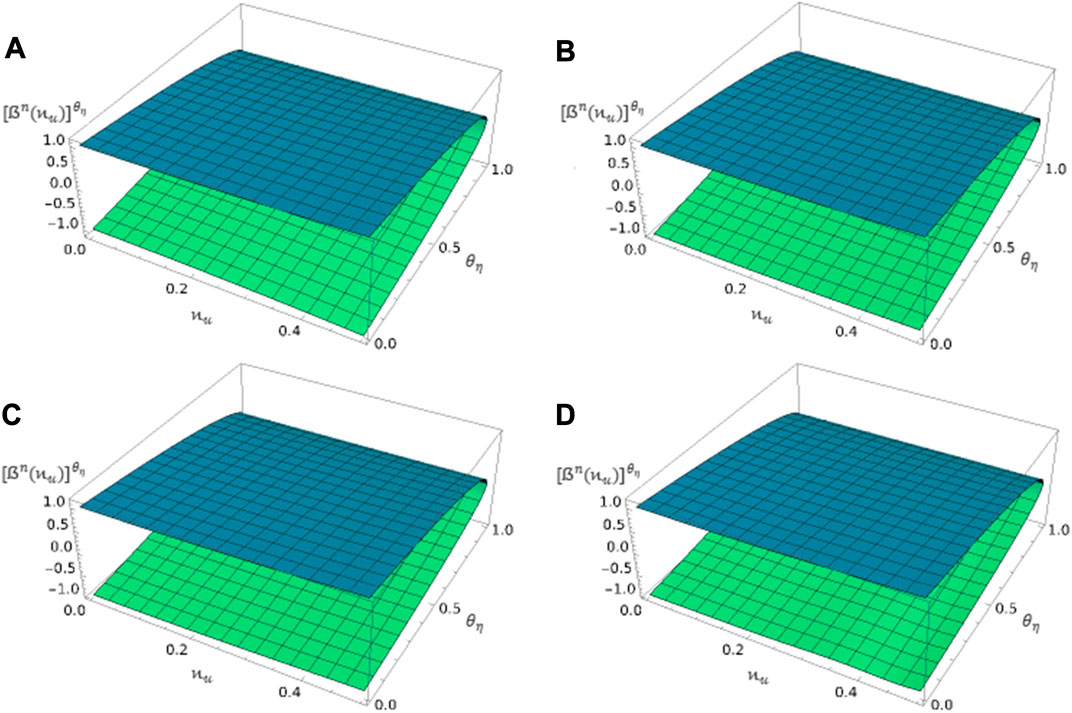
FIGURE 4. Plot of HRKA
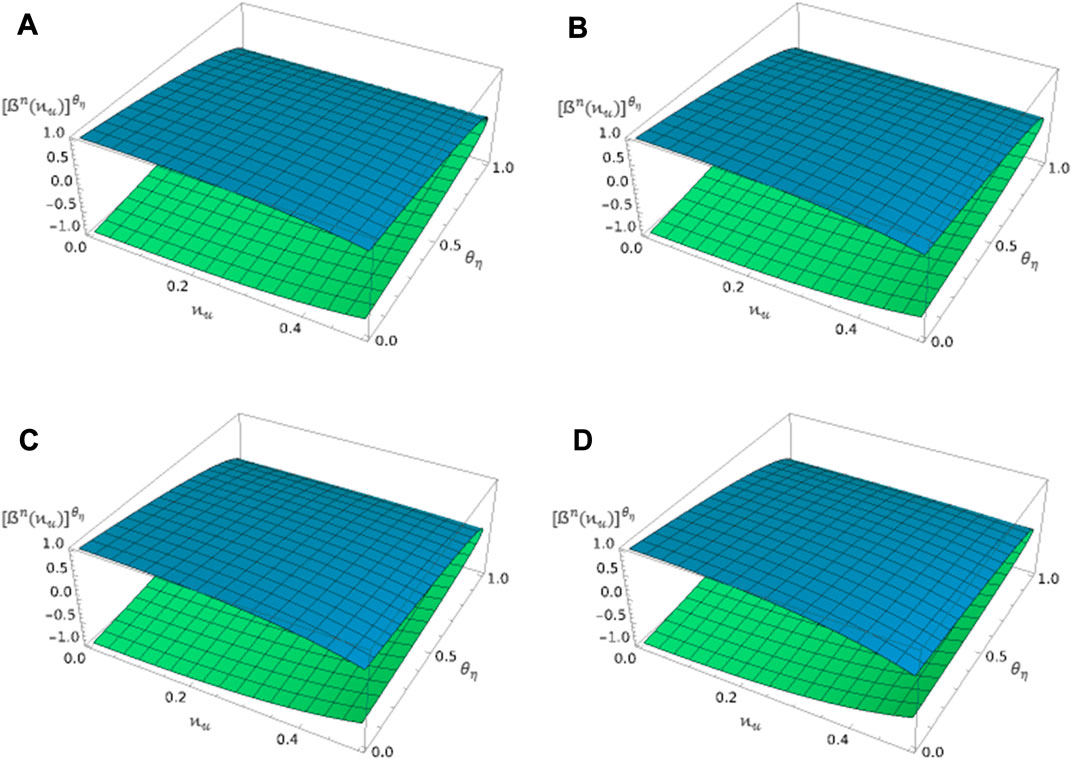
FIGURE 5. Plot of HRKA
Ultimately, we provide
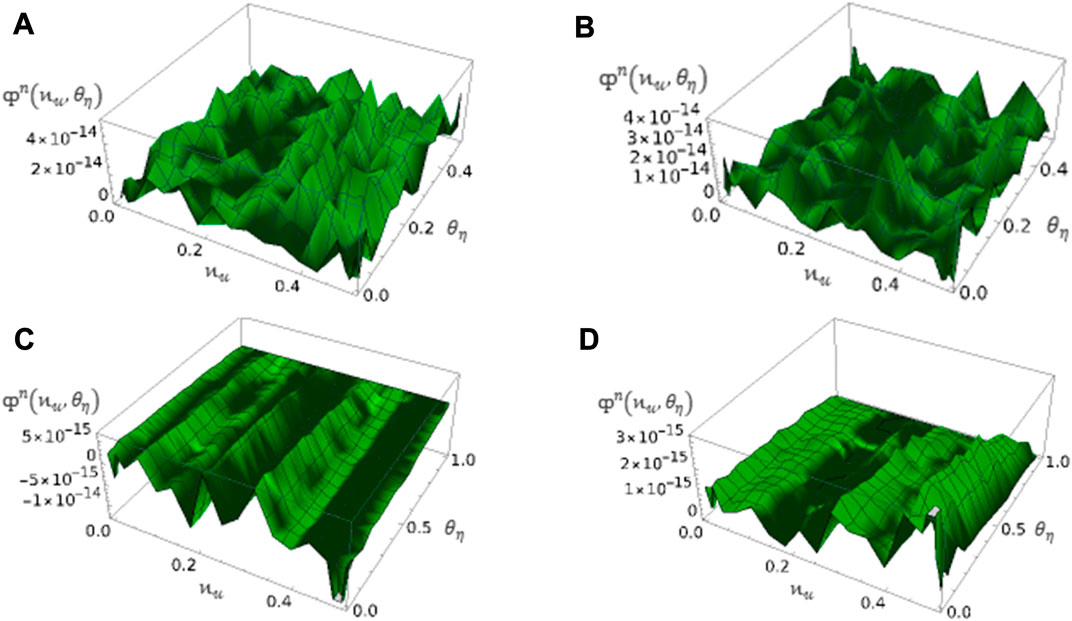
FIGURE 6. Plot of
Based on the obtained plots, it is evident that the graphs demonstrate close agreement and similar behaviors, especially when analyzing the classical derivative. It is important to take note that the model profiles can exhibit unusual behaviors when the value of
9 Key points and summary
In this exploration research, FM-D, FM-I, and FM-FIDM are examined and analyzed for the first time. Alongside, the existence-uniqueness of fuzzy two M-solutions jointly with the characterization theorem is employed as pioneering results as well. Indeed, triplet-simulated pseudocodes related to characterizing
Data availability statement
The original contributions presented in the study are included in the article/Supplementary Material; further inquiries can be directed to the corresponding author.
Author contributions
OA: data curation, investigation, software, methodology, validation, writing–original draft, and writing–review and editing. RM: funding acquisition, investigation, resources, supervision, visualization, and writing–original draft. BM: conceptualization, formal analysis, investigation, project administration, software, and writing–review and editing. All authors contributed to the article and approved the submitted version.
Acknowledgments
The authors would like to express their gratitude to the unknown reviewers for carefully reading the paper and for their helpful comments.
Conflict of interest
The authors declare that the research was conducted in the absence of any commercial or financial relationships that could be construed as a potential conflict of interest.
Publisher’s note
All claims expressed in this article are solely those of the authors and do not necessarily represent those of their affiliated organizations, or those of the publisher, the editors, and the reviewers. Any product that may be evaluated in this article, or claim that may be made by its manufacturer, is not guaranteed or endorsed by the publisher.
Abbreviations
FM-FIDM, fuzzy M-fractional integrodifferential model; CM-FIDM, crisp M-fractional integrodifferential model; HRKA, Hilbert reproducing kernel algorithm; FM-D, fractional M-derivative; FM-I, fractional M-integral; NLDM, nonlinear differential model; IRCC, inductance–resistance–capacitance circuit.
References
1. Chakraverty S, Tapaswini S, Behera D. Fuzzy differential equations and applications for engineers and scientists. USA: CRC Press (2016).
2. Gomes LT, de Barros LC, Bede B. Fuzzy differential equations in various approache. Germany: Springer (2015).
3. Rajab N, Ahmed AM, Al-Faour OM. On fuzzy linear integro-deffrential equations of Volterra type: Approximated solutions of fuzzy linear Volterra integro-differential equations. Moldova: LAP LAMBERT Academic Publishing (2013).
4. Herrmann R. Fractional calculus: An introduction for physicists. Singapore: World Scientific (2014).
5. Tarasov VE. Fractional dynamics: Applications of fractional calculus to dynamics of particles, fields and media. Germany: Springer (2011).
6. West BJ. Fractional calculus view of complexity: Tomorrow’s science. UK: Taylor & Francis (2015).
7. Shabestari MR, Ezzati R, Allahviranloo T. Numerical solution of fuzzy fractional integro-differential equation via two-dimensional Legendre wavelet method. J Intell Fuzzy Syst (2018) 34:2453–65. doi:10.3233/jifs-171707
8. Alikhani R, Bahrami F. Global solutions for nonlinear fuzzy fractional integral and integrodifferential equations. Commun Nonlinear Sci Numer Simulation (2013) 18:2007–17. doi:10.1016/j.cnsns.2012.12.026
9. Salahshour S, Allahviranloo T, Abbasbandy S. Solving fuzzy fractional differential equations by fuzzy Laplace transforms. Commun Nonlinear Sci Numer Simulation (2012) 17:1372–81. doi:10.1016/j.cnsns.2011.07.005
10. Cui M, Lin Y. Nonlinear numerical analysis in the reproducing kernel space. USA: Nova Science (2009).
11. Berlinet A, Agnan CT. Reproducing kernel Hilbert space in probability and statistics. USA: Kluwer Academic Publishers (2004).
13. Jiang W, Chen Z. A collocation method based on reproducing kernel for a modified anomalous subdiffusion equation. Numer Methods Partial Differential Equations (2014) 30:289–300. doi:10.1002/num.21809
14. Geng FZ, Qian SP, Li S. A numerical method for singularly perturbed turning point problems with an interior layer. J Comput Appl Math (2014) 255:97–105. doi:10.1016/j.cam.2013.04.040
15. Akram G, Rehman HU. Solution of fifth order boundary value problem in the reproducing kernel space. Middle East J Scientific Res (2011) 10:191–5.
16. Akgül A, Bonyah E. Reproducing kernel Hilbert space method for the solutions of generalized Kuramoto–Sivashinsky equation. J Taibah Univ Sci (2019) 13:661–9. doi:10.1080/16583655.2019.1618547
17. Akgül A. A novel method for a fractional derivative with non-local and non-singular kernel. Chaos, Solitons and Fractals (2018) 114:478–82. doi:10.1016/j.chaos.2018.07.032
18. Akram G, Rehman HU. Numerical solution of eighth order boundary value problems in reproducing Kernel space. Numers (2013) 62:527–40. doi:10.1007/s11075-012-9608-4
19. Akram G, Rehman HU. Solutions of a class of sixth order boundary value problems using the reproducing kernel space,. Abstract Appl Anal (2013) 2013:560590. doi:10.1155/2013/560590
20. Attia N, Akgül A, Seba D, Nour A, Riaz MB. Reproducing kernel Hilbert space method for solving fractal fractional differential equations. Results Phys (2022) 35:105225. doi:10.1016/j.rinp.2022.105225
21. Abu Arqub O. Adaptation of reproducing kernel algorithm for solving fuzzy Fredholm-Volterra integrodifferential equations. Neural Comput Appl (2017) 28:1591–610. doi:10.1007/s00521-015-2110-x
22. Hasan S, Maayah B, Bushnaq S, Momani S. A modified reproducing kernel Hilbert space method for solving fuzzy fractional integro-differential equations. Boletim da Sociedade Paranaense de Matemática (2023) 41:1–16. doi:10.5269/bspm.52289
23. Yépez-Martínez H, Gómez-Aguilar JF. M-derivative applied to the dispersive optical solitons for the Schrödinger-Hirota equation. The Eur Phys J Plus (2019) 134:93. doi:10.1140/epjp/i2019-12459-7
24. Sousa JVDC, Oliveira ECD. On the local M-derivative. Prog Fractional Differ Appl (2018) 4:479–92. doi:10.18576/pfda/040403
25. Yépez-Martínez H, Gómez-Aguilar JF. Local M-derivative of order $$\alpha$$ α and the modified expansion function method applied to the longitudinal wave equation in a magneto electro-elastic circular rod. Opt Quan Elect (2018) 50:375. doi:10.1007/s11082-018-1643-5
26. Yusuf A, Inc M, Baleanu D. Optical solitons with M-truncated and beta derivatives in nonlinear optics. Front Phys (2019) 7:126. doi:10.3389/fphy.2019.00126
27. Sousa JVDC, Oliveira ECD. A new truncated M-fractional derivative type unifying some fractional derivative types with classical properties. Int J Anal Appl (2018) 16:83–96. doi:10.28924/2291-8639-16-2018-83
28. Mohammed WW, El-Morshedy M, Moumen A, Ali EE, Benaissa M, Abouelregal AE. Effects of M-truncated derivative and multiplicative noise on the exact solutions of the breaking soliton equation. Symmetry (2023) 15:288. doi:10.3390/sym15020288
29. Li C, Dao X, Guo P. Fractional derivatives in complex planes. Nonlinear Anal Theor Methods Appl (2009) 71:1857–69. doi:10.1016/j.na.2009.01.021
30. Guariglia E. Fractional calculus, zeta functions and Shannon entropy. Open Access (2021) 19:87–100. doi:10.1515/math-2021-0010
31. Ortigueira MD, Rodríguez-Germá L, Trujillo JJ. Complex grünwald–letnikov, liouville, riemann–liouville, and Caputo derivatives for analytic functions. Commun Nonlinear Sci Numer Simulation (2011) 16:4174–82. doi:10.1016/j.cnsns.2011.02.022
32. Guariglia E. Riemann zeta fractional derivative-functional equation and link with primes. Adv Difference Equations (2019) 2019:261. doi:10.1186/s13662-019-2202-5
33. Závada P. Operator of fractional derivative in the complex plane. Commun Math Phys (1998) 192:261–85. doi:10.1007/s002200050299
34. Lin SD, Srivastava HM. Some families of the Hurwitz–Lerch Zeta functions and associated fractional derivative and other integral representations. Appl Math Comput (2004) 154:725–33. doi:10.1016/s0096-3003(03)00746-x
35. Podlubny I. Geometric and physical interpretation of fractional integration and fractional differentiation. Fractional Calculus Appl Anal (2004) 5:367–86.
36. Goetschel R, Voxman W. Elementary fuzzy calculus. Fuzzy Sets Syst (1986) 18:31–43. doi:10.1016/0165-0114(86)90026-6
Keywords: fuzzy M-fractional integrodifferential model, fractional M-derivative, fractional M-integral, Hilbert reproducing kernel algorithm, fuzzy existence and uniqueness, characterization theorem
Citation: Abu Arqub O, Mezghiche R and Maayah B (2023) Fuzzy M-fractional integrodifferential models: theoretical existence and uniqueness results, and approximate solutions utilizing the Hilbert reproducing kernel algorithm. Front. Phys. 11:1252919. doi: 10.3389/fphy.2023.1252919
Received: 04 July 2023; Accepted: 31 August 2023;
Published: 02 October 2023.
Edited by:
Emanuel Guariglia, São Paulo State University, BrazilReviewed by:
Firdous A. Shah, University of Kashmir, IndiaHamood Ur Rehman, University of Okara, Pakistan
Tamilvanan Kandhasamy, Kalasalingam University, India
Copyright © 2023 Abu Arqub, Mezghiche and Maayah. This is an open-access article distributed under the terms of the Creative Commons Attribution License (CC BY). The use, distribution or reproduction in other forums is permitted, provided the original author(s) and the copyright owner(s) are credited and that the original publication in this journal is cited, in accordance with accepted academic practice. No use, distribution or reproduction is permitted which does not comply with these terms.
*Correspondence: Omar Abu Arqub, by5hYnVhcnF1YkBiYXUuZWR1Lmpv