- 1Department of Orthopedic Surgery, University of Texas Southwestern Medical Center, Dallas, TX, United States
- 2Department of Physical Medicine and Rehabilitation, University of Texas Southwestern Medical Center, Dallas, TX, United States
- 3Department of Bioengineering, University of Texas at Dallas, Dallas, TX, United States
- 4Department of Physical Therapy and Human Movement Science, Northwestern University, Chicago, IL, United States
- 5Department of Biomedical Engineering, Shanghai Jiao Tong University, Shanghai, China
Background: Shoulder ultrasound is a well-established point-of-care diagnostic modality in orthopaedic and sports medicine. Despite offering measurements of high-quality morphology, this methodology has faced several challenges, including variability in ultrasound systems, operator dependency, and lack of reliable and objective quantitative measures to track disease progression and responses to therapeutic interventions. Computer-aided quantitative ultrasound algorithm (CAQUSA) is an emerging novelty that automates the detection of normal and abnormal structures. Although CAQUSA has been shown to improve detections and diagnoses of soft tissue lesions, the proof-of-concept of utilizing CAQUSA to measure subacromial space width and its encroachment to the rotator cuff tendon have not been tested to assist in clinical decision-making for subacromial pain syndrome.
Objective: This study aimed to develop a CAQUSA that measured the acromiohumeral distance (AHD) and test the algorithm’s reliability and agreement with manual measurements in wheelchair users with spinal cord injury (SCI).
Methods: 116 ultrasound video clips recorded from 10 manual wheelchair users with SCI were evaluated manually by an experienced examiner with expertise in AHD examination and by the CAQUSA, which was developed for bone segmentation with probability mapping. The reliability and agreement of the diagnostic performance between the examiner and the CAQUSA were calculated and compared in both groups of AHD measurements.
Results: The CAQUSA achieved a satisfactory agreement between computer-aided (11.95 ± 2.29 mm) and manual (11.33 ± 2.48 mm) measurements. The intraclass correlation coefficient between the two measures was excellent (ICC = 0.95). The sensitivity was 0.2 mm, with 95% true positives and 5% false positives at the fixed threshold of CAQUSA.
Conclusion: AHD measurements made by the clinical observer were similar to that of the CAQUSA for assessing the clinical metrics related to subacromial pain syndrome. However, the CAQUSA showed greater reliability in its unclear or misleading image analysis. Based on the findings of this proof-of-concept study, the CAQUSA has promise for clinical utilization and interchangeability to minimize examiner-dependent errors and potentially reduce the cost of care.
Introduction
Subacromial pain syndrome (SAPS) is one of the most frequent causes of shoulder pain and dysfunction among individuals with spinal cord injury and disease (SCI/D) [1]. SAPS is reported in 40%–60% of manual wheelchair users with SCI who have had longer wheelchair uses. These individuals have an approximately 10-fold higher risk for rotator cuff tears than able-bodied individuals [2,3]. Rotator cuff injuries resulting from aging and degenerative changes within the subacromial space affect the quality of life among these individuals and inhibit their functional independence [4].
The subacromial space width has been widely used as a clinical index, representing the occupation ratio of the supraspinatus tendon’s thickness within the subacromial space [5]. The manual measurement of the subacromial space width, defined as acromiohumeral distance (AHD), has been discussed in a recently published systematic review to be one of the contributing factors of shoulder pain and dysfunction [6]. Conventional grey-scale ultrasound that examines AHD is a well-established point-of-care diagnostic modality in musculoskeletal and sports medicine rehabilitation [7–10]. The ultrasound system provides portability, no radiation exposure, relatively low cost, and capacity for AHD imaging in dynamic functional positions, making this modality valuable in the clinical care of patients with shoulder disorders. Ultrasonographic AHD measurements identify the bone morphology based on the echo intensity of brightness-mode images [11,12]. Despite offering measurements of high-quality morphology, this modality has faced several challenges, including variability in ultrasound systems, operator fatigue, and lack of reliable and objective quantitative measures to not only identify bone morphologies but also screen for the stage or progression of the AHD, as well as assess responses to therapeutic interventions [13]. Computer-aided quantitative ultrasound algorithm (CAQUSA) is an emerging novelty that automates the detection of normal and abnormal structures [14–16]. Although CAQUSA has been shown to improve detections and diagnoses of soft tissue lesions, there is very little research that explores the segmentation of bone surfaces from echogenicity-based ultrasound images and tests the proof-of-concept and reliability of utilizing CAQUSA to measure AHD in high-risk populations, such as manual wheelchair users with spinal cord injury (SCI). In this study, we developed a CAQUSA to measure the AHD and tested the reliability and agreement with manual measurements in manual wheelchair users with SCI.
Methods
This was a cross-sectional study conducted at two universities’ laboratory settings. Before participating in this study, all participants read and signed the informed consent forms, which were approved by both universities’ Institution Review Board.
Participants
The inclusion criteria for participants were between 18 and 65 years old, having SCI that occurred over a year prior to the start of the study and using a manual wheelchair as the primary means of mobility (self-propel at least 40 h per week). Participants were excluded from this study if they had a history of fractures or dislocations in the shoulder, from which they had not fully recovered; upper limb dysthetic pain because of a syrinx or complex regional pain syndrome; and a history of cardiovascular and cardiopulmonary diseases. Participants were excluded if they had pain in an upper extremity that would interfere with normal functioning and daily activities.
Study protocol
Quantitative ultrasound protocol has strong reliability and validity to determine acute changes in tendon appearance and subacromial space with repetitive wheelchair activity [9,10,17–19]. The rater reliability of AHD measures has been reported good to excellent in SCI population [20]. As recommended to improve reliability of the measures, a single examiner (YSL) with experiences in clinical ultrasound shoulder examinations among wheelchair users scanned and recorded all ultrasound images in a randomized order [9,20]. Image field depth was set at 5 cm, and gain was set at 75 dB in each ultrasound system to minimize the variations of echogenicity of raw images that were optimized based on the relative contrasts to differentiate bone surfaces from other surrounding tissues [17]. Shoulder ultrasound images were captured from the Philips HD11 1.0.6 ultrasound machine with a 5–12 MHz linear transducer or the Supersonic Imagine Aixplorer Ultrasound Machine with an SL14-5 transducer placed on the skin above the deltoid muscles of the non-dominant arm. Each video clip was recorded at 10 Hz for approximately 10 s. Operators recorded two shoulder ultrasound clips to ensure that each frame included scapular and humeral structures. AHD measurements were recorded on the non-dominant shoulder in four different positions: shoulder in a neutral resting position without weight, neutral position with a 3-lb (1.4 kg) weight, 45° and 90° arm elevation in the scapular plane with humeral internal rotation (IR) while holding a 3-lb weight, similar to the methods in previous research protocols [20]. AHD ultrasonographic images were recorded at 10 Hz for each scan using an external video recorder. All collected video clips were converted into a series of ultrasound (US) snapshots and processed in the MATLAB software (Mathworks, Natick, United States). The first and the last 2 seconds of each clip were excluded from the analysis due to the transducer’s preparation and separation from the region of interest. A licensed physical therapist (AS), experienced in imaging AHD and blinded from the data collection and experimental setup, performed manual identification of bony landmarks for calculating the AHD.
Image processing
Our computer-aided approach included two sections. The first section demonstrated the pipeline of echogenicity-based extraction from snapshots of pre-recorded ultrasound video clips. The second section described the CAQUSA based on the probability map used to extract the superior surfaces of the acromion and humeral head for AHD measurement within each ultrasound snapshot. The flowchart of the proposed CAQUSA method for processing an ultrasound video clip to measure AHD is illustrated in Figure 1.
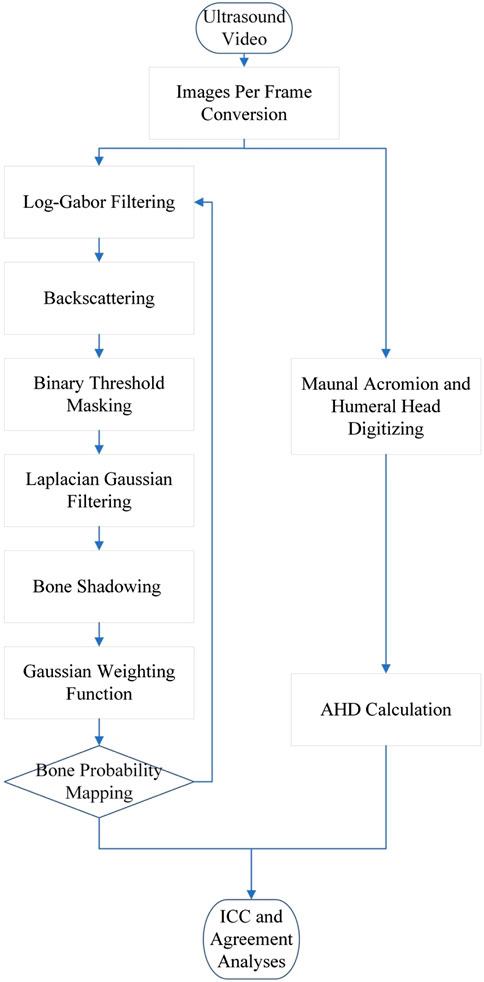
FIGURE 1. Flowchart of the proposed computer-aided quantitative ultrasound algorithm (CAQUSA) for processing an ultrasound video clip to quantify the acromiohumeral stance in wheelchair users with spinal cord injury.
Datasets
This dataset comprised a total of 116 video clips obtained from 10 manual wheelchair users with SCI.
Segmentation method with bone probability mapping
As a significant proportion of the acoustic energy was reflected toward the transducer when comparing the reflected signal from the bone relative to surrounding structures, the acromion and humeral head surfaces were more hyperechoic than the deltoid and supraspinatus tendon in ultrasound images (Figure 2A). The greater pixel values above the shaded area were identified as a bone surface with augmented features through our proposed development of post-processing techniques. Figure 2B represented the inadequate image quality of bone surface to proceed the analyses. Boundaries of bone surfaces were effectively isolated by a local phase extraction procedure, as the echo signal beam reflection was enhanced on bone surfaces and attenuated in other regions. Bone surfaces in ultrasounds exhibit ridge-like responses that were well captured by local image phase symmetry features [14]. We implemented the phase symmetry Log-Gabor filter using the monogenic signal to compute the odd and even responses from band-pass quadrature features [21]. This filter could construct the arbitrary bandwidth to characterize the shadowing effect below the bone surface in ultrasound images (Figure 2C). We applied a binary threshold mask that filtered the neighboring pixels and intensity values lower than two standard deviations from the average neighborhood echogenicity to exclude shallow soft tissue structures above the bone surfaces, thereby reducing the processing time and efficiency (Figure 2D). The Laplacian Gaussian filter detected reflections from US images and carried zero filter responses to a boundary of bright, ridge-like structures. US waves did not penetrate the bone structures, showing higher intensity from soft tissues above and lower intensity from those below the bone structures. This filter resorted to dichotomizing positive responses to low pixel intensity and negative responses to high pixel intensity, resulting in brighter surface areas on the acromion and humeral head and other structures The Laplacian of Gaussian filter was then applied to blur images from the Gaussian kernel (Figure 2E). The shadowing effect of the image was used to eliminate unwanted ridge-like structures above a bone area (Figure 2F). We then multiplied the Gaussian kernel using Gaussian weighting function to calculate the pixel intensities below the shadowiness and the weighted sum of the pixels used to smooth images and remove details and noises as described previously [22,23]. To minimize false-positive and false-negative detections from structures bearing similar echogenicity to bone features in US images, we further processed our images with a backscattering to reduce the interference from soft tissues above the bone structure (Figure 2G). The scan lines of ultrasound backscattering energy were considered to enhance the probability of detecting bone areas, as most of the ultrasound energy was higher in areas of the bone structure. The integrated backscattering energy was calculated by the sum of squared intensities above a given pixel on a scan line to reduce the number of incorrect probability responses [14]. The bone-probability map was constructed by the multiplication of normalized products from the Log-Gabor filter with the band-pass quadrature filter to integrate backscattering and bone shadowing features [24] (Figure 2H).
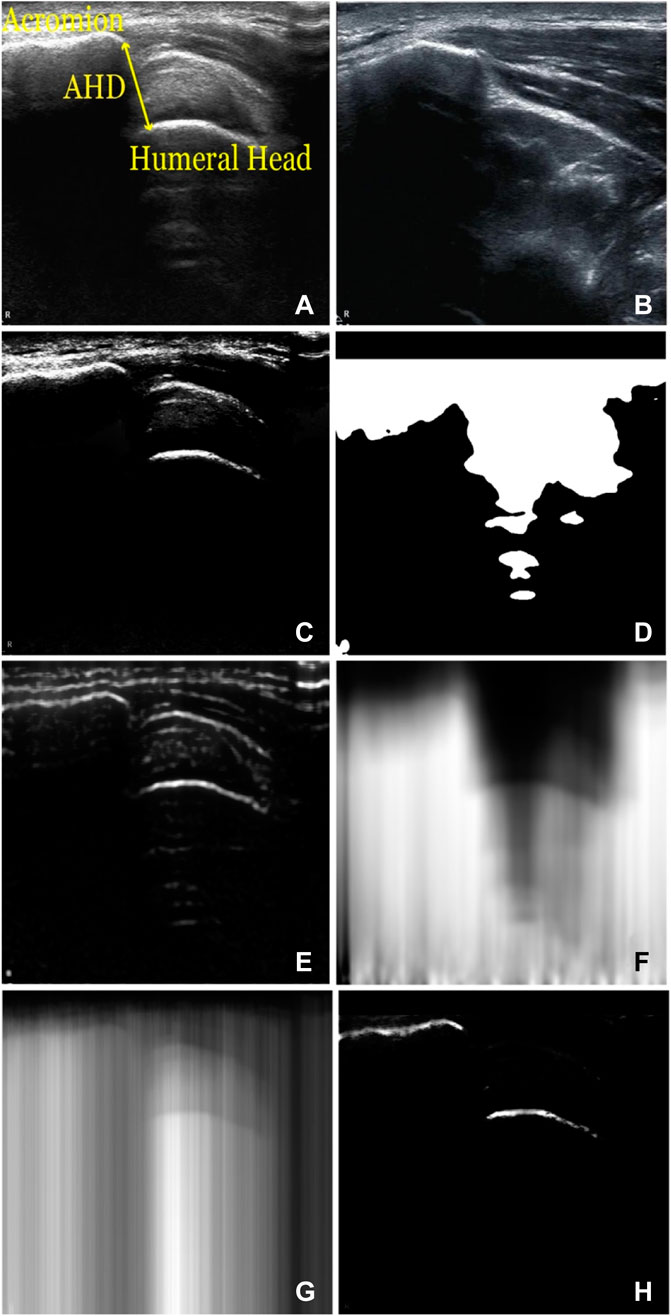
FIGURE 2. CAQUSA Pipeline-post imaging process. (A) example US image with AHD measurement (B) example of inadequate US image (C) US image with Log-Gabor filter applied. (D) Binary features (E) Laplacian of Gaussian with Gaussian Kernel (F) shadowed US image (G) integrated backscattering (H) example probability map.
We identified the acromion and humeral head based on the degree of discontinuity acquired from each vertical (column) scan line in US images using a threshold value of 0.5, as described in previous literature, to separate bone surfaces from other unwanted structures [14,21]. We then optimized a bone probability map for a bone-detection process [22]. The Cost (i, j) function (Eq. 1) represents the continuity of pixels to enhance the segmentation of bone structures and eliminate unwanted hyperechogenic or hypoechogenic pixels of segmented bone structures.
In Eq. 1, i and j are rows and columns, respectively, and Pix indicates pixel coordinates in a 2D.
US image. Cost (i, j) represents the distance between pixels in the jth scan line and the adjacent scan line. A higher referred to adjacent pixels being sparser; therefore, it carried more degrees of discontinuity. Detected bone-like surfaces in US images were not always firm. Thus, we enhanced those features by filling in gaps between pixels with a degree of discontinuity lower than a certain number of pixels, n, in Eq. 2.
Specific conditions for Eq. 2 are shown above, where j is the column of a US image, and n is a constant that is determined by the user based on the specs of the US machine, including resolution and scale. In a user-specified range of j scan lines, the algorithm found two specific pixels that carried Cost (i, j). If Cost (i, j) was less than or equal to the user-specified constant n, the two pixels were assumed to have originated from homogeneous bone-like features. The algorithm connected the two pixels by the creation of a path. If Cost (i, j) was higher than n, the two pixels were assumed to have originated from two heterogeneous bone-like features. Therefore, the algorithm continued to the following scan lines to find other pixels in the following conditions. When a path was created, other pixels between the two specified pixels were assumed to be soft tissue and, therefore, eliminated. According to the principle of ultrasound bean reflection, only one pixel per column on the bone probability map is recognized to initiate the segmentation (Eq. 3). The recognition was performed as follows:
where j is the column index, R is the number of rows in the US image, and B is the bone probability map described in previous fast automatic bone surface segmentation [25]. After generating the bone probability map, the segmentation can then be obtained as follows:
Detected pixels were then segmented based on a Cost function that was recognized as a “jump” in Eq. 5.
In Eq. 5, J(i) indicates a specific column in a US image; it carries a higher Cost (i, j) than the user-determined value of n and is considered as a “jump.” Meanwhile, S(i) is the number of segments determined by the number of “jumps.” Our study used 3.4 mm, the minimum AHD reported previously [26], as a standard to assess the value n. Therefore, spaces between two pixels that were higher than this number indicated the existence of a jump between two heterogeneous segments. On the other hand, the algorithm did not detect bone surfaces if Cost (i, j) was consistently lower than value n, which occurred primarily in cases without hypoechogenic bone features in the US images. A “jump” defined two segments that were located anterior and superior to the “jump.” When multiple “jumps” were detected, additional features could be determined between jump points in addition to the two segments. Assuming that bone surfaces carried a more significant number of pixels, the algorithm excluded segments composed of lower numbers of pixels. It retained two details bearing the first and second highest number of pixels. These two remaining segments were considered as the acromion and humeral head. Once all unwanted components were eliminated, AHD was calculated by selecting two points, the pixel’s most inferior in the acromion and the most superior in the humeral head. After selecting points in each segment, the point-to-point distance was defined as the AHD.
Data analysis
Sensitivity analyses were conducted by excluding low-quality snapshots containing indistinguishable acromion or humeral head in the US images, examined by the experienced examiner. The agreement and reliability were calculated again. If there were no significant changes, the outcome of the computer-aided algorithm was reliable and insensitive to the lower-quality images. For the validation of the proposed computer-aided segmentation method, we compared the AHD measured by our algorithm to that from manual measurement. We compared the processing time between both methods to determine the quantitative performance evaluation. A licensed physical therapist (AS), blinded to the study participants and their shoulder positions, reviewed handpicked sets of images (n = 116) and manually analyzed the AHD. The intra-measurement reliability between the gold standard and computer-aided analyses of AHD was examined using the intraclass correlation coefficient (ICC), a two-way random method with an average of two measurements. The following ICC scale was used for the evaluation: almost perfect (0.81–1.00), excellent (0.61–0.80), moderate (0.41–0.60), and poor to fair (below 0.40) [27]. Correlations between the two methods of measurement were assessed using a Bland-Altman plot. The confidence interval within 95% of the mean differences between the two methods was evaluated. We further validated our segmentation method by evaluating the percentage of a surface fitting between computer-aided and manually segmented bone pixels. To elaborate clinical tolerance (0.5 mm), we extended the surface of manually segmented bone areas by ± 7 pixels.
Results
Acromion and humeral head surface segmentation between CAQUSA and manual measurement
The characteristics of manual wheelchair users are summarized in Table 1. A total of 116 measurements were included in the analysis of AHD measurements. The developed CAQUSA demonstrated 95% true positive with 5% false positive when fixed parameters of the threshold and the 4th power of shadowiness were used to achieve the detection rate. Figure 3 demonstrates the bone surface segmentation determined by manual selection and CAQUSA, visualizing the acromion and humeral head morphology in US images from one of the SCI participants.
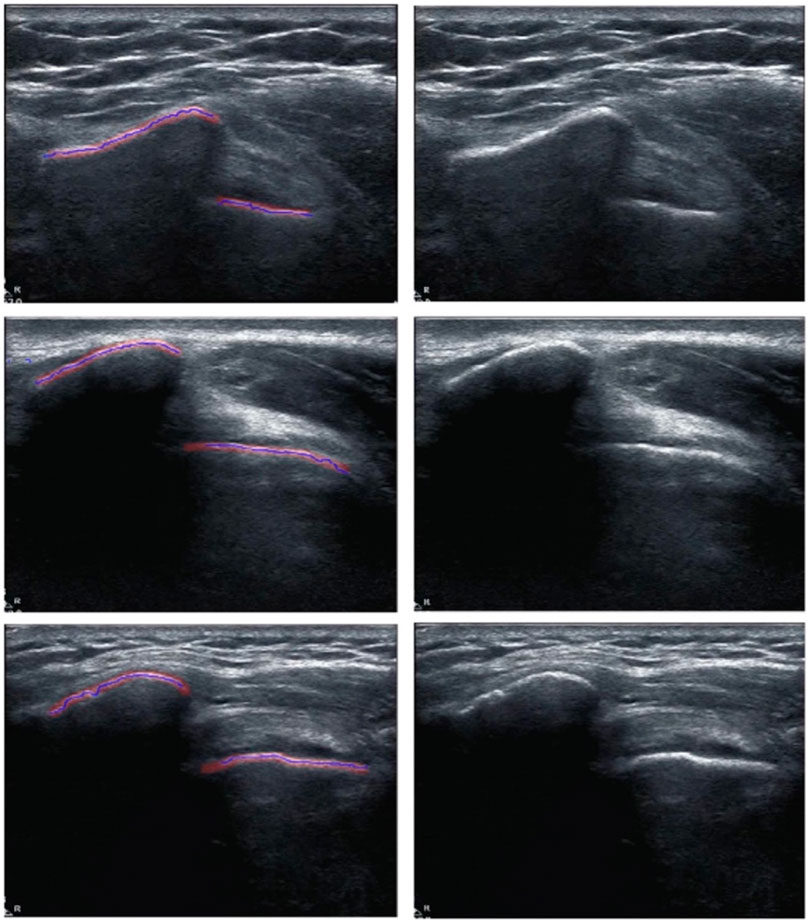
FIGURE 3. Acromion and humeral head surfaces determined by CAQUSA and manual measurement. Pictures in 1st column show bone surface detected by CAQUSA (blue) and manual section (red). Pictures in 2nd column are original images.
In Figure 3, we compared bone surfaces determined by two methods. Our evaluation indicated that both the humeral head and acromion showed great approximation errors of 95.9% ± 5.81% and 96.1% ± 5.81%, respectively.
AHD measurements between CAQUSA and manual measurement
The mean and standard deviation of manual and CAQUSA measurements were 11.95 ± 2.29 mm and 11.33 ± 2.48 mm, respectively. The ICC between the two measures showed excellent intra-rater reliability (ICC = 0.953). In Figure 4A, AHD determined by CAQUSA showed a positive linear association with AHD analyzed by manual detection (r2 = 0.83). Bland-Altman plots were analyzed with reasonable limits of agreement and a mean difference close to zero, indicating similar measurements (Figure 4B). However, one size was over 0.27 mm, and five were below −0.14 mm between the CAQUSA and manual measurements, which suggests that the CAQUSA method achieved excellent sensitivity. Compared to manual measurements, the CAQUSA outside of a confidence interval showed a tendency to underestimate due to the heterogeneity of bone surface reflection in US images that were more hyperechogenic than a bony surface during humeral active elevation.
Discussion
The use of ultrasound to measure AHD has been widely described in SCI populations [7,17,20,28]. Since this is a safe procedure easily to maneuver in the clinics, the US is well-known and regarded as the optimal point-of-care modality to image the shoulders. The US allows for the visualization of the subacromial space, including the bone surface and soft tissues. In addition, US offers real-time examination, no radiation, lower cost, and portability makes the US imaging modality a suitable alternative for imaging bones in functional postures with a higher risk of shoulder injuries and/or pathologies, such as SCI populations. Our study described a computer-aided algorithm utilizing probability mapping for measuring AHD in manual wheelchair users with SCI. The CAQUSA achieved satisfactory reliability and validity compared to manual measurements, demonstrating potential application not only in detecting signs of SAPS, but also becoming an alternative for evaluating the shoulder anatomical morphology within the subacromial space. The study enabled the analytical capacity for addressing labor-intensive challenge to broaden the application with a larger sample size and assist in the grading of AHD. The CAQUSA shows the potential to improve the efficiency and objectiveness of diagnosis and reduce the healthcare cost for assessing SAPS that are increasingly recognized as a popular topic in the field of orthopaedic medicine.
While a growing body of literatures utilized a computer-aided approach to segment anatomical regions of interest from US images, the computer-assisted detection of shoulder structures in US images is underdeveloped and still considered as a research tool in clinical settings [29–31].
Pandey and the colleagues reviewed the common techniques for 2D bone segmentation and reported a lack of standardized validation metric or data set to compare which methods or benchmarking is recommended [23]. In addition, the comparison between using AHD measurements via CAQUSA and independent manual AHD measurements among individuals relying on shoulders as primary means of mobility has not been previously reported. Several studies have employed computer-aided algorithms to segment anatomical features using local phase feature-based methods to automate the analyses in US images [7,32]. Jia and his colleagues developed a computer-aided tracking system with an automatic bone segmentation algorithm based on the US intensity information, including backscattering, acoustic shadows, and local phase features [14]. As the proposed automatic bone detection using a motion analysis system showed the feasibility of quantifying the three-dimensional hip joint rotation angles, the ability to whether this technique is clinically translatable to examine the shoulders of individuals with SCI is unknown. Hacihaliloglu and his colleagues developed a tool that automatically extracted bone surface information from the B-mode US approach to calcualte local phase-based features on the phantom, in vivo and clinical data of bone fractures. Although this technique showed promising results in capturing bone features, the cross-validation with an experienced clinical specialist to confirm the proposed computer-aided method is undetermined [21]. In addition to bone detection, Wang and his colleagues developed a quantitative method to segment the supraspinatus tendons using a self-shrinking snake technique based on a multilayer segmentation framework [31]. The technique required radiologists or US operators to define the rough region of interest before automatically detecting the supraspinatus tendon. Although their approach demonstrated superior performance, the ability to detect the bone surfaces reliably and accurately is unknown for individuals with SCI if the images of tendon boundaries are unclear. Achieving great accuracy in automatic bone segmentation in the clinical setting is challenging due to the variability and heterogeneity while imaging bone surfaces. To mitigate these constraints, specific procedures that filter the noises and misleading artifacts are necessary to amplify the desired features. A low-noise bone surface probability mapping is an ideal method to bypass the constraints. Probability mapping incorporates the results of independent post-image processing methods, and its product indicates the likelihood that the actual bone surface exists within that voxel. The CAQUSA minimized the independent noise-associated features in US images and therefore enhanced the entire bone surfaces while suppressing the shortcomings associated with high levels of artifacts, speckle noises, and unclear boundaries of bone surfaces in US images.
Our study had several limitations. Our dataset involved a small sample size, which only allowed for the development the feasibility of CAQUSA. Clinical data will be needed to verify the external validity of the CAQUSA technique. In addition, this study only included one experienced clinician as the evaluator, disallowing the evaluation of the interobserver agreement among clinicians. Moreover, machine learning-based network architecture has shown great segmentation accuracy with recall and precision rates [33,34]. For example, US image processing using Convolutional Neural Networks has shown great sensitivity, specificity, and accuracy in detecting musculoskeletal structures of the shoulder [35]. We expect further refinements of the CAQUSA method tracking two-bone features will be essential for the future application of an advanced machine learning architectures.
Conclusion
To the best of our knowledge, this was the first study to compare manual and automated techniques for measuring subacromial space with B-mode ultrasound images among individuals with high risk of SAPS. More importantly, this study showed the proof-of-concept of utilizing CAQUSA to measure AHD, which can further assist clinical decision-making among high-risk populations with subacromial pain syndrome. Quantitative measurements using CAQUSA may help identify AHD and enable tailored treatment strategies. Future works are warranted to improve the generalizability of CAQUSA in other non-SCI population such as elders or athletes prone to develop the SAPS. In addition, the receiver operating characteristic curve will also be included to test the sensitivity and specificity of computer-aided approaches with machine learning architecture, as well as explore quasi-real-time measurements in the clinical environment. Our approaches may also assist the computer-aided screening, diagnosis, and evaluation, promoting the generalizability of quantitative ultrasound techniques in the clinical setting.
Data availability statement
The original contributions presented in the study are included in the article/supplementary materials, further inquiries can be directed to the corresponding author.
Ethics statement
The studies involving human participants were reviewed and approved by The University of Texas Southwestern Medical Center Northwestern University, University of Pittsburgh. The patients/participants provided their written informed consent to participate in this study.
Author contributions
Y-SL conceived, designed, and led the study; Y-SL performed the imaging data acquisition; Y-SL and HK performed the imaging algorithm development, processing, analysis, and interpretation. AS, T-YT, and NJ performed analysis and data interpretation. All authors 1) made substantial contributions to the conception and design, or acquisition of data, or analysis and interpretation of data, 2) were involved in drafting the manuscript or revising it critically for important intellectual content, 3) gave final approval of the version to be published, and 4) agreed to be accountable for all aspects of the work in ensuring that questions related to the accuracy or integrity of any part of the work are appropriately investigated and resolved.
Funding
Y-SL was funded for this work by the Craig H. Neilsen Foundation. (Grant number: 295107) and supported, in part, by funding from the National Institute of Health (NIH TL1TR0014230). The funding body had no role in the design of the study collection, analysis, and interpretation of data and in writing the manuscript.
Conflict of interest
The authors declare that the research was conducted in the absence of any commercial or financial relationships that could be construed as a potential conflict of interest.
Publisher’s note
All claims expressed in this article are solely those of the authors and do not necessarily represent those of their affiliated organizations, or those of the publisher, the editors and the reviewers. Any product that may be evaluated in this article, or claim that may be made by its manufacturer, is not guaranteed or endorsed by the publisher.
References
1. Mulroy SJ, Hafdahl L, Dyson-Hudson T. A primary care provider’s guide to shoulder pain after spinal cord injury. Top Spinal Cord Inj Rehabil (2020) 26:186–96. doi:10.46292/SCI2603-186
2. Akbar BM, Balean G, Brunner M, Seyler TM, Bruckner T, Munzinger J, et al. Prevalence of rotator cuff tear in paraplegic patients compared with controls. The J Bone Jt Surgery-American Volume (2010) 92:23–30. doi:10.2106/JBJS.H.01373
3. Pepke W, Brunner M, Abel R, Almansour H, Gerner HJ, Hug A, et al. Risk factors for the development of rotator cuff tears in individuals with paraplegia: A cross-sectional study. Orthopade (2018) 47:561–6. doi:10.1007/s00132-018-3546-3
4. Prescher A. Anatomical basics, variations, and degenerative changes of the shoulder joint and shoulder girdle. Eur J Radiol (2000) 35:88–102. doi:10.1016/s0720-048x(00)00225-4
5. Michener L, Subasi Yesilyaprak SS, Seitz AL, Timmons MK, Walsworth MK. Supraspinatus tendon and subacromial space parameters measured on ultrasonographic imaging in subacromial impingement syndrome. Knee Surg Sports Traumatol Arthrosc (2013) 23:363–9. cited 7 Jan 2014. doi:10.1007/s00167-013-2542-8
6. Walker-Bone K, van der Windt DA. Shoulder pain — where are we now? Curr Treat Options Rheumatol (2021) 7:285–306. doi:10.1007/s40674-021-00184-z
7. Fournier Belley A, Gagnon DH, Routhier F, Roy JS. Ultrasonographic measures of the acromiohumeral distance and supraspinatus tendon thickness in manual wheelchair users with spinal cord injury. Arch Phys Med Rehabil (2017) 98:517–24. doi:10.1016/j.apmr.2016.06.018
8. McCreesh KM, Crotty JM, Lewis JS. Acromiohumeral distance measurement in rotator cuff tendinopathy: Is there a reliable, clinically applicable method? A systematic review. Br J Sports Med (2013) 49:298–305. cited 6 Jan 2014. doi:10.1136/bjsports-2012-092063
9. Lin Y-S, Boninger M, Worobey L, Farrokhi S, Koontz A. Effects of repetitive shoulder activity on the subacromial space in manual wheelchair users. Biomed Res Int (2014) 2014:583951–9. doi:10.1155/2014/583951
10. Soo Hoo JA, Kim H, Fram J, Lin YS, Page C, Easthausen I, et al. Shoulder pain and ultrasound findings: A comparison study of wheelchair athletes, nonathletic wheelchair users, and nonwheelchair users. PM R (2021) 14:551–60. doi:10.1002/pmrj.12648
11. Klich S, Pietraszewski B, Zago M, Galli M, Lovecchio N, Kawczyński A. Ultrasonographic and myotonometric evaluation of the shoulder girdle after an isokinetic muscle fatigue protocol. J Sport Rehabil (2019) 29:1047–52. doi:10.1123/jsr.2019-0117
12. Seitz AL, Michener L. Ultrasonographic measures of subacromial space in patients with rotator cuff disease: A systematic review. J Clin Ultrasound (2010) 39:146–54. doi:10.1002/jcu.20783
13. Seitz AL, McClure PW, Finucane S, Ketchum JM, Walsworth MK, Boardman ND, et al. The scapular assistance test results in changes in scapular position and subacromial space but not rotator cuff strength in subacromial impingement. J Orthop Sports Phys Ther (2012) 42:400–12. doi:10.2519/jospt.2012.3579
14. Jia R, Mellon S, Monk P, Murray D, Noble JA. A computer-aided tracking and motion analysis with ultrasound (CAT & MAUS) system for the description of hip joint kinematics. Int J Comput Assist Radiol Surg (2016) 11:1965–77. doi:10.1007/s11548-016-1443-y
15. Chang RF, Lee CC, Lo CM. Quantitative diagnosis of rotator cuff tears based on sonographic pattern recognition. PLoS One (2019) 14:e0212741–13. doi:10.1371/journal.pone.0212741
16. Hacihaliloglu I, Abugharbieh R, Hodgson A, Rohling R. Bone segmentation and fracture detection in ultrasound using 3D local phase features. Med Image Comput Comput Assist Interv (2008) 11:287–95. doi:10.1007/978-3-540-85988-8_35
17. Bossuyt FM, Boninger ML, Cools A, Hogaboom N, Eriks-Hoogland I, Arnet U. Changes in supraspinatus and biceps tendon thickness: Influence of fatiguing propulsion in wheelchair users with spinal cord injury. Spinal Cord (2020) 58:324–33. doi:10.1038/s41393-019-0376-z
18. Bossuyt FM, Arnet U, Cools A, Rigot S, De Vries W, Eriks-Hoogland I, et al. Compensation strategies in response to fatiguing propulsion in wheelchair users: Implications for shoulder injury risk. Am J Phys Med Rehabil (2020) 99:91–8. doi:10.1097/PHM.0000000000001267
19. Bossuyt FM, Hogaboom NS, Worobey LA, Koontz AM, Arnet U, Boninger ML. Start-up propulsion biomechanics changes with fatiguing activity in persons with spinal cord injury. J Spinal Cord Med (2020) 43:476–84. doi:10.1080/10790268.2019.1582603
20. Lin Y, Boninger M, Kevin A. Ultrasonographic measurement of the acromiohumeral distance in spinal cord injury: Reliability and effects of shoulder positioning. J Spinal Cord Med (2014) 0:1–9. doi:10.1179/2045772314Y.0000000205
21. Hacihaliloglu I, Abugharbieh R, Hodgson AJ, Rohling RN. Automatic adaptive parameterization in local phase feature-based bone segmentation in ultrasound. Ultrasound Med Biol (2011) 37:1689–703. doi:10.1016/j.ultrasmedbio.2011.06.006
22. Foroughi P, Boctor E, Swartz MJ, Taylor RH, Fichtinger G. Ultrasound bone segmentation using dynamic programming. In: Proceeding of the 2007 IEEE Ultrason Symp Proc (2007). p. 2523–6. doi:10.1109/ULTSYM.2007.635
23. Pandey PU, Quader N, Guy P, Garbi R, Hodgson AJ. Ultrasound bone segmentation: A scoping review of techniques and validation practices. Ultrasound Med Biol (2020) 46:921–35. doi:10.1016/j.ultrasmedbio.2019.12.014
24. Rajpoot K, Vicente VV, Noble JA. Local-phase based 3d boundary detection using monogenic signal and its application to real-time 3-d echocardiography images. Proc - 2009 IEEE Int Symp Biomed Imaging From Nano to Macro (2009) (Boston, MA: ISBI). 783–6. doi:10.1109/ISBI.2009.5193166
25. Tu SJ, Morel J, Chen M, Mellon SJ. Fast automatic bone surface segmentation in ultrasound images without machine learning. Lect Notes Comput Sci (Including Subser Lect Notes Artif Intell Lect Notes Bioinformatics (2021) 12722:250–64. LNCS: 250–264. doi:10.1007/978-3-030-80432-9_20
26. Goutallier D, Guilloux P, Postel J, Radier C, Bernageau J, Zilber S. Acromio humeral distance less than six millimeter: Its meaning in full-thickness rotator cuff tear. Orthop Traumatol Surg Res (2011) 97:246–51. doi:10.1016/j.otsr.2011.01.010
27. Landis JR, Koch GG. The measurement of observer agreement for categorical data. Biometrics (1977) 33:159–74. doi:10.2307/2529310
28. Arnet U, Boninger ML, Cools A, Bossuyt FM. Effect of fatiguing wheelchair propulsion and weight relief lifts on subacromial space in wheelchair users. Front Rehabil Sci (2022) 3:849629–11. doi:10.3389/fresc.2022.849629
29. Gupta R, Elamvazuthi I, Dass SC, Faye I, Vasant P, George J, et al. Curvelet based automatic segmentation of supraspinatus tendon from ultrasound image: A focused assistive diagnostic method. Biomed Eng Online (2014) 13:157–18. doi:10.1186/1475-925X-13-157
30. Jahanifar M, Tajeddin NZ, Hasani M, Shekarchi B, Azema K. Automatic recognition of the supraspinatus tendinopathy from ultrasound images using convolutional neural networks (2020). Available: http://arxiv.org/abs/2011.11777 (Accessed January 31, 2023).
31. Wang YW, Lee CC, Lo CM. Supraspinatus segmentation from shoulder ultrasound images using a multilayer self-shrinking snake. IEEE Access (2019) 7:146724–31. doi:10.1109/ACCESS.2018.2885709
32. Hacihaliloglu I, Abugharbieh R, Hodgson AJ, Rohling RN, Guy P. Automatic bone localization and fracture detection from volumetric ultrasound images using 3-D local phase features. Ultrasound Med Biol (2012) 38:128–44. doi:10.1016/j.ultrasmedbio.2011.10.009
33. Baka N, Leenstra S, Van Walsum T. Ultrasound aided vertebral level localization for lumbar surgery. IEEE Trans Med Imaging (2017) 36:2138–47. doi:10.1109/TMI.2017.2738612
34. Ronneberger O, Fischer P, Brox T. U-net: Convolutional networks for biomedical image segmentation. In: Lecture Notes in Computer Science (including subseries Lecture Notes in Artificial Intelligence and Lecture Notes in Bioinformatics) (2015). doi:10.1007/978-3-319-24574-4_28
Keywords: spinal cord injury, acromiohumeral distance, ultrasound, computer-aided quantification algorithm, shoulder, CAQUSA
Citation: Lin Y-S, Kim H, Seitz AL, Tsai T-Y and Jain N (2023) Computer-aided quantitative ultrasound algorithm of acromiohumeral distance among individuals with spinal cord injury. Front. Phys. 11:1075753. doi: 10.3389/fphy.2023.1075753
Received: 03 February 2023; Accepted: 14 March 2023;
Published: 30 March 2023.
Edited by:
Tadashi Yamaguchi, Chiba University, JapanCopyright © 2023 Lin, Kim, Seitz, Tsai and Jain. This is an open-access article distributed under the terms of the Creative Commons Attribution License (CC BY). The use, distribution or reproduction in other forums is permitted, provided the original author(s) and the copyright owner(s) are credited and that the original publication in this journal is cited, in accordance with accepted academic practice. No use, distribution or reproduction is permitted which does not comply with these terms.
*Correspondence: Yen-Sheng Lin, eWVuLXNoZW5nLmxpbkB1dHNvdXRod2VzdGVybi5lZHU=