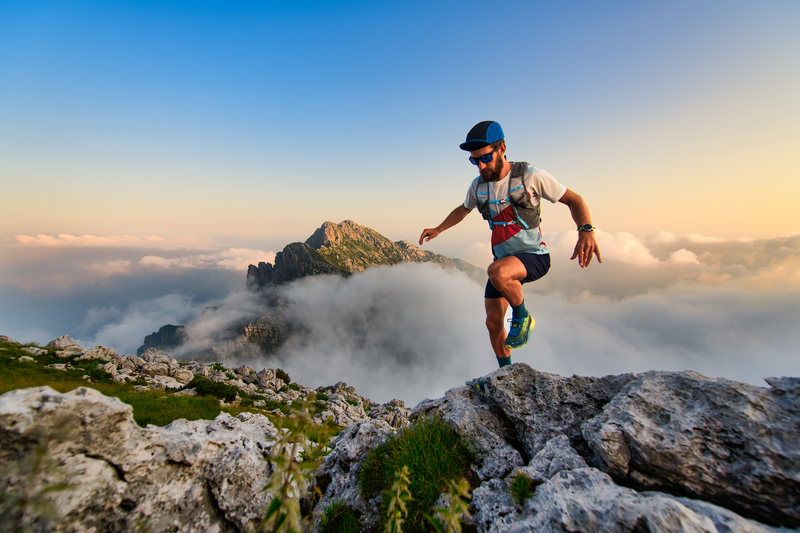
94% of researchers rate our articles as excellent or good
Learn more about the work of our research integrity team to safeguard the quality of each article we publish.
Find out more
PERSPECTIVE article
Front. Phys. , 23 November 2022
Sec. Interdisciplinary Physics
Volume 10 - 2022 | https://doi.org/10.3389/fphy.2022.942896
This article is part of the Research Topic Interdisciplinary Approaches to the Structure and Performance of Interdependent Autonomous Human Machine Teams and Systems (A-HMT-S) View all 16 articles
Intelligent highly-automated systems (HASs) are increasingly being created and deployed at scale with a broad range of purposes and operational environments. In uncertain or safety-critical environments, HASs are frequently designed to seamlessly co-operate with humans, thus, forming human-machine teams (HMTs) to achieve collective goals. Trust plays an important role in this dynamic: humans need to be able to develop an appropriate level of trust in their HAS teammate(s) to form an HMT capable of safely and effectively working towards goal completion. Using Autonomous Ground Vehicles (AGVs) as an example of an HAS used in dynamic social contexts, we explore interdependent teaming and communication between humans and AGVs in different contexts and examine the role of trust and communication in these teams. Drawing on lessons from the AGV example for the design of an HAS used for an HMT more broadly, we argue that trust is experienced and built differently in different contexts, necessitating context-specific approaches to designing for trust in such systems.
Automation is defined as “technology that actively selects data, transforms information, makes decisions, or controls processes” [1]. These technologies are typically designed to help humans achieve their goals more efficiently, and can be classified according to purpose: information acquisition, information analysis, decision selection, action implementation, and automated systems monitoring [2, 3]. A highly-automated system (HAS) may incorporate one or more automation types, and is designed to pursue specific goals with some independence [4]. An HAS designed to operate in uncertain environments is often required to form a dynamic relationship with one or more humans to achieve a goal, forming a human-machine team (HMT). In this perspective, we explore the role of trust in HMTs with a focus on contextual factors shaping trust dynamics in an HMT, as a means of guiding “trustworthy” HMT systems design for diverse and uncertain contexts - an unsolved problem [5].
Human-machine teaming refers to the relationship between a human and machine (typically a HAS) that encompasses the shared pursuit of a common goal [6] as set by humans. The nature of this relationship varies depending on the distribution of decision-making power and roles among the teammates. For example, an HAS may have little influence over the team’s collective actions if it only helps the human make decisions or only acts as instructed by the human. Alternatively, an HAS with the capacity to independently act on its environment in alignment with its team’s goal, with or without human oversight, could have a significant influence over the team’s actions [4]. In some HMTs, the distribution of decision-making and agency between human and HAS teammates is dynamic—it changes with time and circumstance. This distribution can be beneficial: both human and HAS teammates have different strengths and response timescales; dynamically allocating agency can allow for collaborations that optimise the teammates’ contributions. As with any teamwork, achieving these benefits depends heavily on the establishment of an effective relationship between human and HAS teammates.
Designing for effective relationships between human and HAS teammates can prove challenging [4]—particularly when an HAS incorporates artificial intelligence (AI) capabilities. AI capabilities are often used in HASs to enable intelligent, dynamic actions. Essentially, AI imbues HASs with the ability to learn and evolve over time from experience [7]. This learning ability is typically probabilistic, which can yield unpredictable behaviour. This unpredictability is intensified when the HAS is used in real-world contexts characterised by dynamic interactions. One example of such contexts is road traffic: a setting consisting of multiple heterogenous autonomous actors acting in the same environment towards their individual goals, with their interactions often guided by shared rules and understandings. For HMTs operating in such environments, there may be unpredictable aspects of teammate interactions that emerge as a function of the HAS capabilities, the human teammate, the team dynamics, and the complexity and unpredictability of the contexts they operate in. This makes the HMTs adoption in dynamic contexts risky and potentially costly for humans involved—both within the HMT, and in their environments [8–11].
Trust in automation is a key enabler of HMT collaborations and automation adoption. Research shows that trust is key in the successful teaming of dissimilar heterogenous agents involving humans [12]. Trust reflects the degree of confidence a person may have in another actor and can shape human-automation interactions [2]. As noted in [2], trust’s importance in a technology’s adoption correlates with the complexity of the automation and its roles, how critical their deployed environment is, and perceived risks (e.g. [13]). Trust is generally important and useful in:
1. Guiding the design of automation that facilitates productive HMT collaboration and appropriate interactions [2]; and
2. Designing automation with the goal of mitigating the potential negative consequences of their use [2].
In the remainder of this perspective, we focus our exploration of trust in HMTs on HASs designed for large-scale deployment in social settings characterised by dynamic interactions, risks, and uncertainties requiring contextual considerations. To facilitate this argument, we will use the example of an AGV on the road. AGV driving automation systems are HASs that demonstrate all five categories of automation identified in [2, 3]; form part of a HMT; can be designed to dynamically shift roles between a human operator and itself; and operate in diverse, complex, and safety-critical social environments. AGVs deployed in road traffic environments are therefore useful for exploring trust’s role in HMTs operating in social contexts, and demonstrating the need to consider their potential contexts of use in HAS design. To facilitate this exploration, we begin by defining AGVs and exploring some of their properties, considering AGVs as individual agents and exploring AGVs in autonomous teams.
AGVs include driving HAS that, depending on their design, may have the capacity to achieve partial to full autonomy, meaning that the system’s actions can range from providing advice to a human driver to taking full control of driving operations. Their intelligent driving capabilities are often enabled by AI. In the case of AGVs, the HMT consists of a driving HAS and the human driver.
To describe the nature of HMT dynamics between a human operator and an HAS during driving, we draw on the Society of Automotive Engineers (SAE) taxonomy [14] for driving automation systems. The SAE levels describe the capabilities and roles of driving automation and humans at different automation levels. According to the SAE standard, Level 0 vehicles offer no driving automation, while vehicles at level 1 and beyond incorporate driving automation that provides varying levels of support and control when engaged. The human and HAS have joint control of either longitudinal or lateral vehicle motion in level 1, while for level 2, the human actively supervises the system. Level 3–5 vehicles incorporate an Automated Driving System (ADS)—in-vehicle HAS that provides automated driving capabilities that allow for partial to full driverless operation of AGVs. Level 3 vehicles can perform driving conditionally and require humans to serve as a “fallback-ready user”—a human teammate that can take over driving in the vehicle or remotely as appropriate. Level 4 and 5 vehicles perform driving autonomously (albeit in limited circumstances for level 4) and do not need a fallback ready user during operation [14].
Driving HASs from levels 1–4 are increasingly being integrated in vehicles because they promise to improve road safety. This promise can only be achieved if people are receptive of AGVs, use them, and if AGVs operate safely, in a socially acceptable manner when in use. We are already witnessing the trialling and roll out of level 1–4 AGVs in societies—for example, Tesla’s Autopilot features, or China’s first fully driverless taxis—the Baidu self-driving taxis.
These AGVs operate in societies with humans, including a human co-driver on roads with other human and autonomous road agents. They use road resources and infrastructure alongside other road users in diverse socioeconomic contexts. Consider, for example, the operation of Level 3 AGVs on the road. When engaged, the ADS and human driver complement each other as co-drivers, playing interdependent dynamic roles in ensuring the safe navigation of AGVs to their destinations. This requires the human driver and the ADS to continuously communicate with each other and their environments through sensing, monitoring, and team acting. This example demonstrates an HMT in which the human and machine share decision-making and action implementation control. In such an HMT, there are two interdependent dynamical aspects to consider: that of the environment the HMT acts, and the team itself.
Within the HMT, team dynamics are shaped by the capabilities of each teammate, as well as the roles they are expected to play in achieving the team’s goals set by the human teammate. In AGVs, increased automation made possible by increased cognitive capability and dynamic adaptability of the ADS comes at a price: adapting in real-time to the surrounding environment. This can lead to the ADS exhibiting unpredictable behavior, particularly in situations they have not been designed for nor are familiar with, impacting trust.
The potential for unpredictability in AGVs has been demonstrated multiple times—e.g., a Tesla in automated driving mode nearly hitting an individual [15], or the Uber self-driving car crash resulting in the death of a jaywalking pedestrian in Arizona [16]. In the case of the Uber crash, the AGV was struggling to classify the jaywalking pedestrian, while its human operator was paying attention to her tablet. Both were operating independently—unaware of each other’s activities until too late [17].
Both examples illustrate the challenge AGVs and their human teammates face in operating on the road that needs to be considered and designed for in ADS: the diverse and dynamic nature of road transport environments. While transport infrastructure facilitates some predictability through traffic lights and stop signs, the inclusion of human agency—within and outside vehicles—creates an inherently unpredictable environment, one that has been found to vary significantly depending on infrastructure and social norms [18, 19].
An unpredictable environment combined with increased dependency on the ADS by the human creates the opportunity for unpredictable reactions by the human or the AGV teammate to that environment. To achieve AGV use at scale, HMTs will need to demonstrate the ability to act and react appropriately to achieve their collective goals safely and responsively in any environmental context. This requirement poses a significant design challenge in which the HMT and its environments are dynamic and inherently unpredictable.
Trust—within an HMT and within societies where HMTs may operate—is an important factor that affects the adoption and safe use of HASs. It is a dynamic construct that can help us to understand HASs, HMTs and their environments, and to design for their interactions. Trust definitions are subjective and contextual, and one’s understanding may be shaped by experiences in different research fields, cultures or contexts [1, 12, 20]. With this in mind, we explore and define trust, first broadly, in the context of HASs, and then specifically for AGVs.
Trust is widely researched across disciplines ranging from engineering to psychology, economics, etc. Trust as a social concept is interpersonal, and is researched as existing within relationships [2, 12]. We adopt this trust definition: the “willingness of a party to be vulnerable to the actions of another party based on the expectation that the other will perform a particular action important to the trustor, irrespective of the ability to monitor or control that other party” [12]. By this definition, HMT teamwork is easily understood as mutual dependence from a shared awareness (e.g. [21]).
Over the past century, efforts towards researching and developing trustworthy AI and human-automation trust have increased, as have the complexity and deployment rate of HASs. With regards to automation, human trust can be defined as “the attitude that an agent will help achieve an individual’s goals in a situation characterized by uncertainty and vulnerability” [1]. In this definition, agent can refer to humans or automation systems. Specific to AI systems, the High-Level Expert Group on Artificial Intelligence defined trustworthy AI systems as systems where trust is established in their design, development, deployment, and use [22]. Trustworthy AI refers to AI systems that are assured to act in the trusting party’s interest [23], and society at large.
Trust in automation varies depending on the automation, their context of use, and the human operator [2]. All these need to be considered holistically in trustworthy automation design. In the context of an HMT, trust is usually one-sided: humans need to trust their automated teammates to collaborate effectively, but an automation agent within the team has no inherent knowledge of “trust” in the human sense. Humans tend to evaluate the trustworthiness of other agents—HASs included—based on their perceived abilities, integrity, and benevolence [12]. An automation’s association with trust relates specifically to its design: its actions and communication must foster an appropriate trust level with the humans it interacts with.
In AGVs, as with many other safety-critical HAS, trust is necessary for a human driver to willingly collaborate with the driving automation [24]. Hence, it is useful in understanding how they might interact with ADS. Trust development is dynamic. In HMTs, human and HAS teammates develop mutual expectations and an understanding of one another over time [1] as they interact in a given context. One way human teammates express the level of trust they have in an automation is through reliance or compliance, which may vary in different use cases [1, 2]. For AGVs, for example, a human operator may rely on an ADS to take the lead after it safely navigates a familiar well-marked road, while opting to take full control when navigating an unfamiliar school crossing.
To ensure an appropriate level of reliance on an ADS in uncertain and risky situations, humans need to develop and maintain appropriate trust with the ADS. To achieve this, appropriate communication of the automation’s capabilities, intentions, decisions, and actions is important. But appropriate communication is also contextual and dynamic: the nature of the automation, the human operator, and the context or environment the HMT operate in all inform the potential risks involved in navigating a given situation as well as the appropriate communication methods within and outside the HMT [2]. In the next section, we explore the importance of communication in trust development and maintenance in HMTs with a continued focus on AGVs.
An AGV HMT operates in safety-critical situations where lack of cooperation can result in fatal accidents, as observed from the aforementioned Uber accident in Arizona [16]. In general, analyses show that accidents can stem from inappropriate trust. Inappropriate trust in AGVs can include overtrust, where a human operator trusts an ADS too much, leading to human inaction at crucial moments, or undertrust, where humans do not trust the ADS enough, resulting in a human overtaking ADS duties inappropriately [1]. Inappropriate trust can be caused by inappropriate communication of information between automation and its teammate [1, 2, 25, 26].
Hoff and Bashir [2] summarized the design recommendations for trustworthy automation as: increasing anthropomorphism with consideration of user preferences, simplifying user interfaces, ensuring an automation’s communication style appears trustworthy, providing users with accurate and continuous feedback on its reliability, explaining their behaviours, and increasing automation feedback and transparency. The Chartered Institute for Ergonomics and Human Factors similarly proposed nine principles to address key human factor challenges in ADS design [4]. The principles revolve around the HAS, their users and environments, and their interactions and communication. All these design recommendations highlight appropriate communication as a means of shaping trust dynamics for humans interacting with automation.
Specific to AGVs, trust can be influenced by the driving scenario [27], the ADS communication style, the interface design [28], the appropriateness of the level of detail in explanations provided to the human operator [27], and so on (see [29]). These findings, too, highlight the importance of appropriate communication and interface design in shaping trust dynamics for a successful AGV HMT. Because the context informs the risks involved, the definitions of appropriate communication and the ways appropriate communication are achieved will vary depending on the human and machine teammates and their operational context. This highlights the importance of understanding context to designing appropriately for successful teaming.
However, implementing these recommendations in an HAS used in diverse environments globally may prove challenging. For AGVs, driving culture and norms may vary in different nations, driving environments, and communities; these are usually tacitly and explicitly taught to—and understood by—human drivers; shape how human drivers operate on the road; and have been found to influence the risks involved [18]. Success for AGVs and any HAS used for HMT deployed at scale will involve responsively accounting for local cultures, norms, and communication expectations, lending support to the idea that contextually appropriate communication will play an important role in enabling effective HMTs. Some provide guidance for carrying out contextually-sensitive work for specific contexts—see, e.g., Smith [30]. But such guidance is difficult to carry out at scale.
To properly design for the diverse contexts HASs may operate in, it is important to understand these contexts and how road agents interact and communicate with one another in them. Some of the approaches used for this are: ethnographic observations, cultural probes, interviews, modelling and simulations, surveys, etc. [31–34] The choice of method is in itself shaped by context; therefore, there is currently no one systematic way for determining the appropriateness of the methods to contextual design problems.
In this perspective, we used AGVs to explore how the contextual nature of trust can play a significant role in whether HMTs can operate at scale and how, particularly in uncertain or safety-critical scenarios. As we saw with HMTs involving AGVs, dynamic changes in the teammates’ roles can combine with contextual factors (environments, communication expectations, social norms, trust definitions, etc.) to make designing for successful HMTs a significant challenge.
As a result, we see a need to change how designers think about designing for trust in HMTs. It is not enough to design HASs that are trusted by humans—we must instead aspire to design HASs that are worthy of trust in the contexts and dynamic environments in which they will operate. Central to this conclusion is the need to facilitate appropriate trust through appropriate communication and performance—both of which are context dependent.
We therefore propose questions that could guide future work on HASs that are likely to form part of HMTs in diverse contexts:
• How can we help designers create trustworthy HASs for HMTs, where “trustworthy” is defined appropriately for the contexts HMTs will operate in?
• How can we help designers (those who play a significant role in shaping HAS) understand how their own trust perception shapes the design process? And how can they design for trust as others (drivers, pedestrians, regulators, etc.) understand it?
• What approaches and frameworks can be used to systematically support these?
Most HASs—if successful—are now deployed globally. These questions suggest the need to create new frameworks for creating trustworthy HMTs—ones where the definition of “trustworthy” is dynamic, contextual, and representative of the many voices whose lives are likely to be impacted when such a system is deployed [5].
The original contributions presented in the study are included in the article/Supplementary material, further inquiries can be directed to the corresponding author.
MI conducted the literature review underpinning this work, wrote the first draft, and took the lead in revisions leading to the submitted paper. ZA and EW contributed ideas to the structure of the paper, provided additional literature on human-machine teaming and communication aspects, provided editorial feedback, and contributed writing to sections of the paper.
MI is funded by scholarships including: ANU HDR Fee Merit Scholarship, Florence McKenzie Supplementary Scholarship in a New Branch of Engineering, and the University Research Scholarship.
We thank Amir Asadi, a fellow PhD student, for sharing relevant literature with the authors.
The authors declare that the research was conducted in the absence of any commercial or financial relationships that could be construed as a potential conflict of interest.
All claims expressed in this article are solely those of the authors and do not necessarily represent those of their affiliated organizations, or those of the publisher, the editors and the reviewers. Any product that may be evaluated in this article, or claim that may be made by its manufacturer, is not guaranteed or endorsed by the publisher.
1. Lee JD, See KA. Trust in automation: Designing for appropriate reliance. hfes (2004) 46(1):50–80. doi:10.1518/hfes.46.1.50.30392
2. Hoff KA, Bashir M. Trust in automation: Integrating empirical evidence on factors that influence trust. Hum Factors (2015) 57(3):407–34. doi:10.1177/0018720814547570
3. Parasuraman R, Sheridan TB, Wickens CD. A model for types and levels of human interaction with automation. IEEE Trans Syst Man Cybern A (2000) 30(3):286–97. doi:10.1109/3468.844354
4.CIEHF. Human factors in highly automated systems (2022). Available from: https://ergonomics.org.uk/resource/human-factors-in-highly-automated-systems-white-paper.html (Accessed on Sep 19, 2022).
5. National Academies of Sciences E. Human-AI teaming: State-of-the-Art and research needs (2021). Available from: https://nap.nationalacademies.org/catalog/26355/human-ai-teaming-state-of-the-art-and-research-needs (Accessed on Sep 28, 2022).
6. Walliser JC, de Visser EJ, Shaw TH. Application of a system-wide trust strategy when supervising multiple autonomous agents. Proc Hum Factors Ergon Soc Annu Meet (2016) 60(1):133–7. doi:10.1177/1541931213601031
7. Wing JM. Trustworthy AI (2020). ArXiv200206276 Cs [Internet]Available from: http://arxiv.org/abs/2002.06276 (Accessed on Nov 23, 2021).
8. Lima A, Rocha F, Völp M, Esteves-Veríssimo P. Towards safe and secure autonomous and cooperative vehicle ecosystems. In: Proceedings of the 2nd ACM workshop on cyber-physical systems security and privacy [internet]. New York, NY, USA: Association for Computing Machinery (2016). p. 59
9. Liu P, Ma Y, Zuo Y. Self-driving vehicles: Are people willing to trade risks for environmental benefits? Transportation Res A: Pol Pract (2019) 125:139–49. doi:10.1016/j.tra.2019.05.014
10. Ramchurn SD, Stein S, Jennings NR. Trustworthy human-AI partnerships. iScience (2021) 24(8):102891. doi:10.1016/j.isci.2021.102891
11. Hussain R, Zeadally S. Autonomous cars: Research results, issues, and future challenges. IEEE Commun Surv Tutorials (2019) 21(2):1275–313. doi:10.1109/comst.2018.2869360
12. Mayer RC, Davis JH, Schoorman FD. An integrative model of organizational trust. Acad Manage Rev (1995) 20(3):709–34. doi:10.5465/amr.1995.9508080335
13. Williams ET, Nabavi E, Bell G, Bentley CM, Daniell KA, Derwort N, et al. Chapter 17 - begin with the human: Designing for safety and trustworthiness in cyber-physical systems. In: WF Lawless, R Mittu, and DA Sofge, editors. Human-machine shared contexts. Academic Press (2020). Available from: https://www.sciencedirect.com/science/article/pii/B9780128205433000171 (Accessed on Jul 4, 2022).
14.On-Road Automated Driving (ORAD). Taxonomy and definitions for terms related to driving automation systems for on-road motor vehicles [Internet] (2021). Available at: https://www.sae.org/content/j3016_202104 (Accessed Oct 15, 2021).
15. Hill B. This shocking video of Tesla fsd Autopilot almost hitting A pedestrian is being covered-up [internet]. HotHardware (2021). Available from: https://hothardware.com/news/tesla-fsd-autopilot-crosswalk-dmca-video-takedown (Accessed on Apr 7, 2022).
16.Uber self-driving test car involved in accident resulting in pedestrian death. TechCrunch. Available from: https://social.techcrunch.com/2018/03/19/uber-self-driving-test-car-involved-in-accident-resulting-in-pedestrian-death/(Accessed on Apr 7, 2022).
17. Lawless W. Toward a Physics of interdependence for autonomous human-machine systems: The case of the uber fatal AccidentFront phys (2018). Available from: https://www.frontiersin.org/articles/10.3389/fphy.2022.879171 (Accessed on Sep 23, 2022).
18. Nordfjærn T, Şimşekoğlu Ö, Rundmo T. Culture related to road traffic safety: A comparison of eight countries using two conceptualizations of culture. Accid Anal Prev (2014) 62:319–28. doi:10.1016/j.aap.2013.10.018
19. Müller L, Risto M, Emmenegger C. The social behavior of autonomous vehicles. In: Proceedings of the 2016 ACM international joint conference on pervasive and ubiquitous computing: Adjunct. Heidelberg Germany: ACM (2016). Available from: https://dl.acm.org/doi/10.1145/2968219.2968561 (Accessed on Dec 7, 2022).
20. Idemudia ES, Olawa BD. Once bitten, twice shy: Trust and trustworthiness from an african perspective. In: CT Kwantes, and BCH Kuo, editors. Trust and trustworthiness across cultures: Implications for societies and workplaces [internet]. Cham: Springer International Publishing (2021). doi:10.1007/978-3-030-56718-7_3
21. Sliwa J. Toward collective animal neuroscience. Science (2021) 374(6566):397–8. doi:10.1126/science.abm3060
22.Ethics guidelines for trustworthy AI. Shaping Europe’s digital future (2022). Available from: https://digital-strategy.ec.europa.eu/en/library/ethics-guidelines-trustworthy-ai (Accessed on Apr 7, 2022).
23.The IEEE Global Initiative on Ethics of Autonomous and Intelligent Systems. Ethically aligned design: A vision for prioritizing human well-being with autonomous and intelligent systems, version 2 [Internet]. New York, USA: IEEE. Available from: https://standards.ieee.org/industry-connections/ec/ead-v1/ (Accessed on Apr 7, 2022).
24. Walker F, Wang J, Martens MH, Verwey WB. Gaze behaviour and electrodermal activity: Objective measures of drivers’ trust in automated vehicles. Transportation Res F: Traffic Psychol Behav (2019) 64:401–12. doi:10.1016/j.trf.2019.05.021
25. Ekman F. Designing for appropriate trust in automated vehicles: A tentative model of trust information exchange and gestalt [Internet]. Gothenburg, Sweden: Chalmers University of Technology. Available from: https://research.chalmers.se/publication/517220.
26. Niculescu AI, Dix A, Yeo KH. Are you ready for a drive? User perspectives on autonomous vehicles. In: Proceedings of the 2017 CHI Conference Extended Abstracts on Human Factors in Computing Systems [Internet]. (Denver, Colorado: Association for Computing Machinery (2017). p. 2810–2817. doi:10.1145/3027063.3053182
27. Ma RHY, Morris A, Herriotts P, Birrell S. Investigating what level of visual information inspires trust in a user of a highly automated vehicle. Appl Ergon (2021) 90:103272. doi:10.1016/j.apergo.2020.103272
28. Oliveira L, Burns C, Luton J, Iyer S, Birrell S. The influence of system transparency on trust: Evaluating interfaces in a highly automated vehicle. Transportation Res Part F: Traffic Psychol Behav (2020) 72:280–96. doi:10.1016/j.trf.2020.06.001
29. Merat N, Madigan R, Nordhoff S. Human factors, user requirements, and user acceptance of ride-sharing in automated vehicles. International Transport Forum Discussion Papers (2017). Report No.: 2017/10. Available from: https://www.oecd-ilibrary.org/transport/human-factors-user-requirements-and-user-acceptance-of-ride-sharing-in-automated-vehicles_0d3ed522-en.
30. Smith CJ. Designing trustworthy AI: A human-machine teaming framework to guide development (2019). ArXiv191003515 Cs Available from: http://arxiv.org/abs/1910.03515
31. Vereschak O, Bailly G, Caramiaux B. How to evaluate trust in AI-assisted decision making? A survey of empirical methodologies. Proc ACM Hum Comput Interact (2021) 5(CSCW2):1–39. doi:10.1145/3476068
32. Nathan LP. Sustainable information practice: An ethnographic investigation. J Am Soc Inf Sci Technol (2012) 63(11):2254–68. doi:10.1002/asi.22726
33. Balfe N, Sharples S, Wilson JR. Understanding is key: An analysis of factors pertaining to trust in a real-world automation system. Hum Factors (2018) 60(4):477–95. doi:10.1177/0018720818761256
Keywords: trust, autonomous vehicle, human-machine teaming, communication, context
Citation: Ibrahim MA, Assaad Z and Williams E (2022) Trust and communication in human-machine teaming. Front. Phys. 10:942896. doi: 10.3389/fphy.2022.942896
Received: 13 May 2022; Accepted: 10 November 2022;
Published: 23 November 2022.
Edited by:
William Frere Lawless, Paine College, United StatesReviewed by:
Katie Parnell, University of Southampton, United KingdomCopyright © 2022 Ibrahim, Assaad and Williams. This is an open-access article distributed under the terms of the Creative Commons Attribution License (CC BY). The use, distribution or reproduction in other forums is permitted, provided the original author(s) and the copyright owner(s) are credited and that the original publication in this journal is cited, in accordance with accepted academic practice. No use, distribution or reproduction is permitted which does not comply with these terms.
*Correspondence: Memunat A. Ibrahim, bWVtdW5hdC5pYnJhaGltQGFudS5lZHUuYXU=
Disclaimer: All claims expressed in this article are solely those of the authors and do not necessarily represent those of their affiliated organizations, or those of the publisher, the editors and the reviewers. Any product that may be evaluated in this article or claim that may be made by its manufacturer is not guaranteed or endorsed by the publisher.
Research integrity at Frontiers
Learn more about the work of our research integrity team to safeguard the quality of each article we publish.