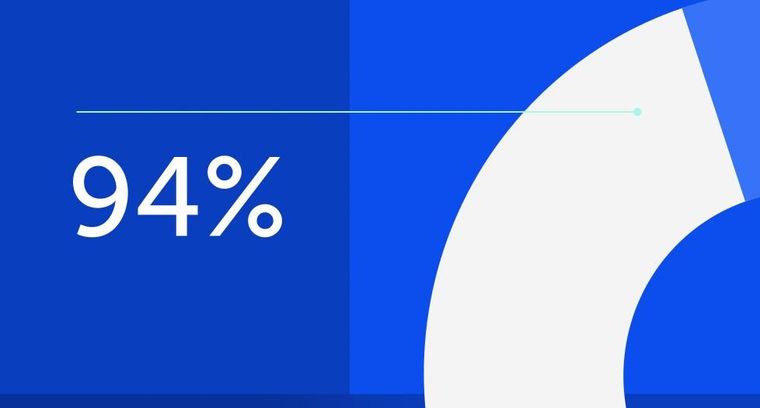
94% of researchers rate our articles as excellent or good
Learn more about the work of our research integrity team to safeguard the quality of each article we publish.
Find out more
ORIGINAL RESEARCH article
Front. Phys., 16 June 2022
Sec. Interdisciplinary Physics
Volume 10 - 2022 | https://doi.org/10.3389/fphy.2022.919951
El Niño is the long-lasting anomalous warming of sea surface temperature (SST) and surface air temperature (SAT) over the tropical Pacific. Each El Niño event has its unique impact on the overlaying atmosphere, where the warming exhibits diversity in spatiotemporal patterns. It still remains an open question for discriminating the El Niño diversity, since the single area-averaging SST index often fails to distinguish the impact of the event diversity, which is partially due to the nonlinear and non-uniform variations of the warming patterns. Here, we introduced the Dynamical Systems metrics (DSMs) to measure instantaneous dimensions and persistence of the SAT warming patterns over the tropical Pacific. Our results show that different SAT warming patterns can be discriminated by their corresponding values of dimension and persistence, then the central Pacific and eastern Pacific El Niño events can be discriminated by DSM. Particularly, through the analyses of El Niño events, we can interpret the physical meaning of DSM parameters applied to the space-time SAT field: an instantaneous dimension reflects whether the sub-regions of the SAT field are consistently varying and to what degree the spatial pattern of anomalies is homogeneous, while the instantaneous persistence indicates how long an anomalous SAT pattern can be maintained. This work analyzes the spatiotemporal variability of El Niño from a dynamical system perspective, and DSM may also serve as a useful tool to study extreme events related to SST anomalies.
1. The instantaneous persistence and dimensions of SAT anomalies over the tropical Pacific are highly sensitive to El Niño states
2. The central Pacific and eastern Pacific El Niño events can be discriminated by DSM parameters
3. The physical meaning of DSM parameters in meteorological space-time fields is interpreted.
El Niño is a typical extreme event causing a strong influence on the worldwide weather and climate [1–3]. Each El Niño event can last for several months, in which sea surface temperature (SST) over the tropical Pacific gets anomalously warm compared to that in the normal periods. The spatial patterns of SST anomaly (SSTA) are found to have the wide diversity in different types of El Niño events [4–6], i.e., the central Pacific (CP) El Niño which has strong warm SSTAs only located in the central Pacific and the eastern Pacific (EP) El Niño whose warm SSTAs distribute over both central and eastern Pacific. The difference in the SSTA spatial patterns can initiate the anomalous atmospheric convections with different strengths and locations [7,8], further causing different global climate effects via atmospheric waves [3,9]. Therefore, the El Niño diversity regarding the spatiotemporal patterns and the impact on atmospheric states has been an important topic in the climate study (e.g., [2,10–13]).
El Niño is usually quantified by the area-averaged SSTAs over four given regions (Figure 1A), i.e., Niño1+2, Niño3, Niño4, and Niño3.4 indices [4,14]. These four Niño indices essentially denote the temporal variations of the sea surface temperature over the four given spatial regions, respectively (Figure 1A). However, these SST-based Niño indices are obscure in terms of dynamical states of atmospheric fields and then they cannot always accurately infer the El Niño impact on the atmosphere [3,8,13,15]. On the other hand, the dynamical evolution features of a meteorological field have been encoded into both of its spatial and temporal structures, whereas the area-averaged procedure misses this spatial information of meteorological fields [13,16]. Thus, the dynamical evolution features of different El Niño events cannot be adequately reflected by Niño indices [8,12,13,15]. Accordingly, further focusing on atmospheric variables (e.g., surface air temperature) and spatiotemporal variability of the meteorological fields can be favorable to an in-depth understanding of the El Niño diversity and its impact on the dynamical states of the atmosphere.
FIGURE 1. Illustration of the dynamical system analysis applied to the equatorial Pacific SATA. (A) Example of a real-time SATA map over the equatorial Pacific on 19 February 2003. The Niño1+2, Niño3, Niño4, and Niño3.4 regions are shown by red, blue, purple, and green boxes, respectively. The black box denotes the SATA field selected to construct the dynamical state space. (B) Idealized diagram of a trajectory in the dynamical state space of the SATA. A point
In this study, we will utilize the Dynamical Systems metrics (DSMs, [17]) to analyze the spatiotemporal variability of the surface air temperature (SAT) over the tropical Pacific during El Niño episodes. DSM was proposed to quantify the instantaneous dynamical states of the climate subsystem over a given region, by incorporating both the spatial and temporal structures of the interested meteorological field. Different from the empirical orthogonal function (EOF), which relies on the linear covariance of the data, the DSM algorithm regards spatiotemporal data as the dynamical trajectory in the state space, and thus both linear and nonlinear statistical features can be characterized by DSM [13,17]. In recent studies, DSM has been applied to various issues in the climate study (e.g., [13,18–20]), where the instantaneous dynamical properties of the interested meteorological field can be effectively measured, and DSM is considered to be a promising tool to analyze the extreme events in the ocean and atmosphere.
Accordingly, two motivations of this study are to identify the dynamical properties of the SAT anomalies within different El Niño states and to interpret the physical meaning of DSM through analysis of the SAT over the tropical Pacific. On the one hand, the DSM applied to analyze SAT over tropical Pacific may address the aforesaid deficiency of Niño indices in the El Niño study. On the other hand, the interpretation of DSM could contribute further to a better understanding of this newly developed approach and provide a technical reference for future related climate studies.
The rest of this article is arranged as follow: Section 2 will describe the used data and DSM. Section 3 is divided into three parts: Section 3.1 will compare the Niño indices with the instantaneous dynamical parameters quantified by DSM, thus understanding how the dynamical states of SATA are influenced by the extreme events. Based on DSM, Section 3.2 will focus on the SAT anomaly (SATA) over the tropical Pacific during El Niño episodes, with regard to the diversity in spatial and temporal patterns of the SATA. Particularly, through analysis of the spatiotemporal patterns of SATA, the physical meaning of the DSM parameters in terms of the meteorological field will be demonstrated. Then, Section 3.3 will utilize the DSM parameters to compare different El Niño events. Further discussions and conclusions will be made in Section 4.
This study uses the daily mean 2-m temperature data (i.e., SAT) from the European Centre for Medium-Range Weather Forecasts’ ERA-Interim reanalysis [21], with a temporal range from 1979-01-01 to 2019-08-31. To focus on the anomalous variations of SAT, here, we subtract the long-term climatological average for each calendar day over the whole span from the 2-m temperature data. Additionally, the daily time series of Niño1+2, Niño3, Niño4, and Niño3.4 indices are obtained from the KNMI Climate Explorer website: http://climexp.knmi.nl. The spatial domains of Niño1+2, Niño3, Niño4, and Niño3.4 regions are, respectively, illustrated in Figure 1A. To cover these regions and measure the SATA dynamical states over the tropical Pacific, the spatial domain for the DSM computation is set at 160°E–80°W and 10°N–10°S (Figure 1A). Noting that DSM has been demonstrated to be not sensitive when the spatial resolution is set as 0.75°, 1.5°, and 2.5° [19], here, we select a horizontal resolution of 0.75° for the SATA data.
In the methodology of DSM [17], the evolution of a given meteorological field is acting as a dynamical system, in which the temporal evolutions of states of this dynamical system are described by an observed state-space trajectory X(t) (i.e., a matrix embedded with the spatiotemporal data). In this study, X(t) is a succession of daily SATA latitude–longitude maps within the selected spatial domain (see black box lines in Figures 1A), and a certain point
Aiming at
As reported in the previous studies (19,22,23), the two parameters in Eq. 1 can be used to indicate the instantaneous dynamical properties of the system.
The aforementioned interpretations for the DSM parameters are in the sense of dynamical systems theory [18,23]; then, the recent studies have demonstrated that the values of instantaneous persistence and dimension are closely related to the intrinsic predictability of a certain climate subsystem ([19,20]). In this study, we will use
We first analyze how the extreme SSTA states over the tropical Pacific influence the dynamical properties of SATA. The extreme SSTA states are usually inferred by referring to Niño indices. Though the four Niño indices are highly correlated to one another [5,14], extreme events from four different Niño indices are not completely consistent. For example, when the Niño1+2 index exceeds its 90% quantile, all of Niño3, Niño3.4, and Niño4 indices do not reach their own 90% quantiles (Figures 2A,B). This also means that extreme SSTA events can emerge from different sub-regions of the tropical Pacific [13]. Then, we observe how the dynamical properties of SATA vary with these extreme SSTA states defined by different Niño indices (Figures 2C,D). Niño1+2 and Niño4 indices denote the SSTA states in the eastern and western tropical Pacific, respectively (Figure 1A), and the comparison in Figure 2 shows that when either Niño1+2 index or Niño4 index reaches an extreme state, both
FIGURE 2. Comparisons among the four Niño indices and the dynamical properties of SATA. (A) Scatter plots of Niño1+2 index versus Niño4 index, and the color map of the Niño3 index. The dash lines denote the 90% quantiles of the Niño1+2 and Niño4 indices, respectively. (B) Same as (A), but the color map is for the Niño3.4 index. (C) Same as (A), but the color map is for
Taking a segment of the SATA temporal evolution as an example (Figure 3), the covariation between DSM parameters and SATA spatiotemporal pattern can be observed. During the first 200 days of this segment, the zonal distribution of SATA is inhomogeneous and the SATA signals over different sub-regions are weak, at which
FIGURE 3. The SATA temporal evolution from 1996-08-21 to 1997-12-09. (A) SATA is averaged over 5°S–5°N as a function of time and longitude. (B) and (C) show the time series of
To further understand the association between the SATA dynamical properties and the different El Niño states, we inspect how
FIGURE 4. Analyses of the dynamical system parameters during El Niño episodes. (A) Time series of Niño34 index,
Focusing on the SATA dynamical properties during El Niño episodes (Figure 4B),
To show the spatiotemporal patterns of the SATA field corresponding to different values of
FIGURE 5. Comparison of the SATA Hovmöller diagrams between high and low persistence (during El Niño episodes). (A) SATA is averaged over 5°S–5°N as a function of the lag time and longitude, with compositing the days with the
Toward the analysis on
FIGURE 6. Comparison of the SATA patterns between high and low dimensions (during El Niño episodes). (A) Composite SATA patterns with (top panel) the
The aforementioned results interpret the physical meaning of
The aforementioned section demonstrates that
FIGURE 7. Comparison of different El Niño events. (A) Scatter plots of
The previous studies utilized the scatter plot of Niño3 and Niño4 indices to classify the types of El Niño [4,5,30]. For comparison, in Figure 7B, we present the mean values of Niño3 and Niño4 indices within the ten El Niño events. The diagonal line in this scatter plot can divide the typical EP and CP El Niño events into two divisions, whereas the events in 1986/87, 1991/92, 2006/07, and 2015/16 are close to the diagonal line. A similar phenomenon can be observed in the scatter plot of
In this study, we employ the DSM parameters (i.e.,
Additionally, in Section 3.2, we systematically discuss the physical meaning of
In addition to El Niño, the extreme SSTA events over the tropical Pacific can be La Niña events, the coastal warming events, and the Pacific meridional mode events, etc. [4,13,32–34], but they are not analyzed in this study. From the results of DSM, it is shown that La Niña and the coastal warming events have also reduced the values of
The original contributions presented in the study are included in the article/Supplementary Material; further inquiries can be directed to the corresponding author.
YH and ZF designed the study. YH accomplished the computations. YH wrote the manuscript. All authors discussed and interpreted the results and edited the manuscript.
This work was supported by the National Natural Science Foundation of China (Nos. 42175065 and 41975059).
The authors declare that the research was conducted in the absence of any commercial or financial relationships that could be construed as a potential conflict of interest.
All claims expressed in this article are solely those of the authors and do not necessarily represent those of their affiliated organizations, or those of the publisher, the editors, and the reviewers. Any product that may be evaluated in this article, or claim that may be made by its manufacturer, is not guaranteed or endorsed by the publisher.
The authors thank the constructive suggestions of the reviewers.
The Supplementary Material for this article can be found online at: https://www.frontiersin.org/articles/10.3389/fphy.2022.919951/full#supplementary-material
1. Timmermann A, An S-I, Kug J-S, Jin F-F, Cai W, Capotondi A, et al. El Niño-Southern Oscillation Complexity. Nature (2018) 559(7715):535–45. doi:10.1038/s41586-018-0252-6
2. Cai W, Wu L, Lengaigne M, Li T, McGregor S, Kug JS, et al. Pantropical Climate Interactions. Science (2019) 363(6430):944. doi:10.1126/science.aav4236
3. Hu ZZ, McPhaden MJ, Kumar A, Yu JY, Johnson NC. Uncoupled El Niño Warming. Geophys Res Lett (2020) 47(7):e2020GL087621. doi:10.1029/2020gl087621
4. Kug J-S, Jin F-F, An S-I. Two Types of El Niño Events: Cold Tongue El Niño and Warm Pool El Niño. J Clim (2009) 22(6):1499–515. doi:10.1175/2008jcli2624.1
5. Ren HL, Jin FF. Niño Indices for Two Types of ENSO. Geophys Res Lett (2011) 38:L04704. doi:10.1029/2010gl046031
6. Capotondi A, Wittenberg AT, Newman M, Di Lorenzo E, Yu J-Y, Braconnot P, et al. Understanding ENSO Diversity. Bull Am Meteorol Soc (2015) 96(6):921–38. doi:10.1175/bams-d-13-00117.1
7. Gill AE. Some Simple Solutions for Heat-Induced Tropical Circulation. Q.J R Met. Soc. (1980) 106(449):447–62. doi:10.1002/qj.49710644905
8. Williams IN, Patricola CM. Diversity of ENSO Events Unified by Convective Threshold Sea Surface Temperature: a Nonlinear ENSO index. Geophys Res Lett (2018) 45(17):9236–44. doi:10.1029/2018gl079203
9. Horel JD, Wallace JM. Planetary-scale Atmospheric Phenomena Associated with the Southern Oscillation. Mon Wea Rev (1981) 109(4):813–29. doi:10.1175/1520-0493(1981)109<0813:psapaw>2.0.co;2
10. Meng J, Fan J, Ashkenazy Y, Havlin S. Percolation Framework to Describe El Niño Conditions. Chaos (2017) 27(3):035807. doi:10.1063/1.4975766
11. Hou Z, Li J, Ding R, Karamperidou C, Duan W, Liu T, et al. Asymmetry of the Predictability Limit of the Warm ENSO Phase. Geophys Res Lett (2018) 45(15):7646–53. doi:10.1029/2018gl077880
12. Lu ZH, Yuan NM, Chen L, Gong Z. On the Impacts of El Niño Events: a New Monitoring Approach Using Complex Network Analysis. Geophys Res Lett (2020) 47(6):e2019GL086533. doi:10.1029/2019gl086533
13. Huang Y, Yuan N, Shi M, Lu Z, Fu Z. On the Air-Sea Couplings over Tropical Pacific: An Instantaneous Coupling index Using Dynamical Systems Metrics. Geophys Res Lett (2022) 49(2):e2021GL097049. doi:10.1029/2021gl097049
14. Trenberth KE, Stepaniak DP. Indices of El Niño Evolution. J Clim (2001) 14(8):1697–701. doi:10.1175/1520-0442(2001)014<1697:lioeno>2.0.co;2
15. Song W-J, Yu J-Y. Identifying the Complex Types of Atmosphere-Ocean Interactions in El Niño. Environ Res Lett (2019) 14(11):114030. doi:10.1088/1748-9326/ab4968
16. Ren HL, Wang R. Distinct Growth Rates of the Two ENSO Types. Geophys Res Lett (2020) 47(16):e2020GL088179. doi:10.1029/2020gl088179
17. Faranda D, Messori G, Yiou P. Diagnosing Concurrent Drivers of Weather Extremes: Application to Warm and Cold Days in North America. Clim Dyn (2020) 54(3-4):2187–201. doi:10.1007/s00382-019-05106-3
18. Messori G, Caballero R, Faranda D. A Dynamical Systems Approach to Studying Midlatitude Weather Extremes. Geophys Res Lett (2017) 44(7):3346–54. doi:10.1002/2017gl072879
21. Dee DP, Uppala SM, Simmons AJ, Berrisford P, Poli P, Kobayashi S, et al. The ERA-Interim Reanalysis: Configuration and Performance of the Data Assimilation System. Q.J.R Meteorol Soc (2011) 137(656):553–97. doi:10.1002/qj.828
19. Faranda D, Messori G, Yiou P. Dynamical Proxies of North Atlantic Predictability and Extremes. Sci Rep (2017) 7:41278. doi:10.1038/srep41278
22. Faranda D, Masato G, Moloney N, Sato Y, Daviaud F, Dubrulle B, et al. The Switching between Zonal and Blocked Mid-latitude Atmospheric Circulation: a Dynamical System Perspective. Clim Dyn (2016) 47(5):1587–99. doi:10.1007/s00382-015-2921-6
23. Faranda D, Vaienti S. Correlation Dimension and Phase Space Contraction via Extreme Value Theory. Chaos (2018) 28(4):041103. doi:10.1063/1.5027386
20. Faranda D, Alvarez-Castro MC, Messori G, Rodrigues D, Yiou P. The Hammam Effect or How a Warm Ocean Enhances Large Scale Atmospheric Predictability. Nat Commun (2019) 10(1):1316. doi:10.1038/s41467-019-09305-8
25. Zheng F, Fang X-H, Yu J-Y, Zhu J. Asymmetry of the Bjerknes Positive Feedback between the Two Types of El Niño. Geophys Res Lett (2014) 41(21):7651–7. doi:10.1002/2014gl062125
26. Bach E, Motesharrei S, Kalnay E, Ruiz-Barradas A. Local Atmosphere-Ocean Predictability: Dynamical Origins, lead Times, and Seasonality. J Clim (2019) 32(21):7507–19. doi:10.1175/jcli-d-18-0817.1
27. Reynolds RW, Smith TM, Liu C, Chelton DB, Casey KS, Schlax MG. Daily High-Resolution-Blended Analyses for Sea Surface Temperature. J Clim (2007) 20(22):5473–96. doi:10.1175/2007jcli1824.1
28. Paek H, Yu JY, Qian CC. Why Were the 2015/2016 and 1997/1998 Extreme El Niños Different? Geophys Res Lett (2017) 44(4):1848–56.
29. Hu S, Fedorov AV. Cross-equatorial Winds Control El Niño Diversity and Change. Nat Clim Change (2018) 8(9):798–802. doi:10.1038/s41558-018-0248-0
30. Yeh S-W, Kug J-S, Dewitte B, Kwon M-H, Kirtman BP, Jin F-F. El Niño in a Changing Climate. Nature (2009) 461(7263):511–4. doi:10.1038/nature08316
31. Okumura YM. ENSO Diversity from an Atmospheric Perspective. Curr Clim Change Rep (2019) 5(3):245–57. doi:10.1007/s40641-019-00138-7
32. Zhao J, Kug JS, Park JH, An SI. Diversity of North Pacific Meridional Mode and its Distinct Impacts on El Niño-Southern Oscillation. Geophys Res Lett (2020) 47(19):e2020GL088993. doi:10.1029/2020gl088993
33. Pietri A, Colas F, Mogollon R, Tam J, Gutierrez D. Marine Heatwaves in the Humboldt Current System: from 5-day Localized Warming to Year-Long El Niños. Sci Rep (2021) 11(1):21172. doi:10.1038/s41598-021-00340-4
34. Shi M, Huang Y, Fu Z. Dynamical Systems Persistence Parameter of Sea Surface Temperature and its Associations with Regional Averaged index over the Tropical Pacific. Intl J Climatology (2022). doi:10.1002/joc.7664
Keywords: dynamical systems, instantaneous persistence, instantaneous dimension, El Niño, surface air temperature
Citation: Huang Y, Shi M and Fu Z (2022) A Dynamical Systems Perspective to Characterize the El Niño Diversity in Spatiotemporal Patterns. Front. Phys. 10:919951. doi: 10.3389/fphy.2022.919951
Received: 14 April 2022; Accepted: 09 May 2022;
Published: 16 June 2022.
Edited by:
Jingfang Fan, Beijing Normal University, ChinaReviewed by:
Yongwen Zhang, Kunming University of Science and Technology, ChinaCopyright © 2022 Huang, Shi and Fu. This is an open-access article distributed under the terms of the Creative Commons Attribution License (CC BY). The use, distribution or reproduction in other forums is permitted, provided the original author(s) and the copyright owner(s) are credited and that the original publication in this journal is cited, in accordance with accepted academic practice. No use, distribution or reproduction is permitted which does not comply with these terms.
*Correspondence: Zuntao Fu, ZnV6dEBwa3UuZWR1LmNu
†ORCID: Yu Huang, https://orcid.org/0000-0002-7930-9056; Zuntao Fu, https://orcid.org/0000-0001-9256-8514
Disclaimer: All claims expressed in this article are solely those of the authors and do not necessarily represent those of their affiliated organizations, or those of the publisher, the editors and the reviewers. Any product that may be evaluated in this article or claim that may be made by its manufacturer is not guaranteed or endorsed by the publisher.
Research integrity at Frontiers
Learn more about the work of our research integrity team to safeguard the quality of each article we publish.