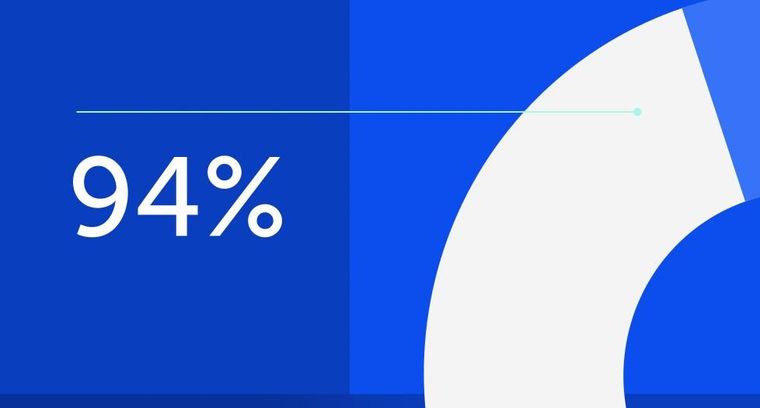
94% of researchers rate our articles as excellent or good
Learn more about the work of our research integrity team to safeguard the quality of each article we publish.
Find out more
ORIGINAL RESEARCH article
Front. Phys., 06 April 2022
Sec. Social Physics
Volume 10 - 2022 | https://doi.org/10.3389/fphy.2022.855380
Polycentric city-regional development has become a buzzword for urban scholars and planners around the world. Inspired by the two critical characteristics of the polycentric urban region (PUR) as incubators and hinges of knowledge production, this study revealed the evolution of the polycentric structure and driving mechanism of PUR from the perspective of innovation network by using unique inter-city patent cooperation data. The results show that: 1) The innovation networks exhibit hierarchical and uneven characteristics, with the economic core cities becoming incubators and hinges of innovation and dominating the development of innovation networks. 2) There is compatibility between morphological polycentricity and functional polycentricity in PURs, but there are also differences. Although the morphological polycentricity of three PURs has improved, functional polycentricity is underdeveloped. 3) The geographic, institutional, institutional, and cultural proximity play the facilitating role for inter-city knowledge flows and their polycentric enhancement, but city attributes such as population size have a lower impact. Our study is believed to renew the knowledge polycentricity conceptualization and enlighten the evolution of innovation network.
Since the 21st century, most economically developed polycentric urban region (PUR) have shown polycentric development trends (Steven et al., 2015) and have attracted extensive attention from economics, geography, and planning disciplines [1]. In spatial planning, polycentric development is also regarded as a vital strategy to alleviate the over-concentration of resources in large cities and improve the overall regional competitiveness and social cohesion [2]. Due to the different geographical scales and development stages, polycentricity has different meanings [3], which leads to considerable ambiguity in its understanding, and no consensus has been formed [4]. However, the measurement of morphological polycentricity relies mainly on that of socio-economic statistics, which not only lacks uniform criteria for center classification and threshold delineation[5], but is also often restricted by administrative divisions, making it difficult to accurately reflect the complex and diverse functional linkages and measure functional polycentricity [6].
With the rise of the network society, PURs are no longer considered morphological entities with clear administrative boundaries but functional regions with close linkages between multiple centers [7]. [8] reformulated relational polycentricity as functional polycentricity, which was widely recognized by other scholars. In this context, regional polycentricity refers to the balanced distribution of PUR and emphasizes the year of close functional linkages between centers [9]. However, although scholars have interpreted polycentricity in multiple scales and dimensions [10], there are still some shortcomings for polycentricity measurement due to the difficulties of data acquisition (Veneri and Paolo, 2013). Therefore, the empirical study of polycentricity is still challenging [11].
In recent years, the rapid development of big data technology has provided powerful support for obtaining complex relational data among cities [12,13], thus becoming a new perspective and method for measuring the spatial structure of cities and regions [14]. However, previous studies have analyzed the socio-economic patterns of PURs and their polycentric structures using enterprise networks or transportation networks [15], while ignoring the crucial functions of PURs as innovation incubators and hinges, especially the lack of analysis on the evolution process of knowledge polycentricity in PURs [11]. With the knowledge economy development, inter-city knowledge flows characterize higher-energy innovation linkages and become the determinant of the global competitiveness of PURs [12]. Exploring the morphological and functional polycentricity of PURs based on the perspective of innovation networks is the way to fully understand innovation space formation and promote balanced and sustainable polycentric development of PUR. Therefore, this paper examines the evolution pattern of knowledge polycentricity in PUR from three dimensions: Network pattern, morphological polycentricity, and functional polycentricity to increase the understanding of PUR development, which could propose policy implications for collaborative innovation development of PUR.
This paper answers three main questions: 1) What are the characteristics of region science system innovation network pattern evolution, and which cities function as the innovation incubators and hinges? 2) How to measure the PUR structure, and is there consistency between morphological and functional polycentricity? 3) What are the driving mechanisms for the evolution of knowledge polycentricity? The innovation points of this study mainly lie in the following three aspects. Firstly, this study uses big data technology to obtain long-term inter-city patent data series to focus on neglected innovation functions (incubators and hinges) and analyze the evolution of their innovation network from a dynamic perspective. In particular, the performance of PUR is interpreted with full consideration of the strength and direction of inter-city innovation linkages, providing sufficient information and insights for future urban regions planning. Secondly, this study establishes a systematic analytical framework for understanding the structure of regional polycentricity in three dimensions: Pattern, morphology, and function, which helps to compensate for the limitations of the one-sided focus on morphological polycentricity in current studies and is well adapted to other studies of urban regional polycentricity. Finally, this study draws on multi-dimensional proximity theory and constructs an econometric model to analyze the driving mechanisms of innovation linkages and their polycentric evolution of knowledge, extending recent theoretical and empirical studies.
Polycentricity includes both morphological and functional dimensions. Morphological polycentricity is centered on nodal characteristics and refers to the spatially balanced distribution of centers [16]. In terms of measurement, scholars have mainly used indicators such as the economic output of cities and population size (Mcmillen and Mcdonald, 2010). In practice, the clearest indicator of polycentricity is the slope of the best-fit regression line using the rank-size rule. In addition, scholars have also compared the observed polycentricity with ideal polycentricity models and searched for gaps to initially identify polycentric structures (Wu et al., 2021). For example, [17] used a gravity model, and [2] proposed a connectivity model to estimate the ideal polycentricity scenario. In general, morphological polycentricity focuses on the relative size of nodes and their importance [18]. However, the essence of PUR is the free flow of resources such as people and other resources among different centers and the formation of close functional links [19], and the city size and its scale distribution are not enough to accurately portray the polycentric characteristics. In recent years, scholars have analyzed the polycentric structure of urban areas mainly from functional polycentricity [20].
Functional polycentricity emphasizes linkages between nodes and argues that more balanced and multi-pointing relationships represent a higher degree of polycentricity [21]. state that a polycentric system “can be defined as a network of node cities and their interactions.” Scholars have mainly used the social network analysis proposed to measure it. Currently, scholars use multivariate relational data such as population flow [22], traffic flow [15], and enterprise flow [23] to analyze the functional polycentricity of PUR. In addition, some scholars have also studied the economic and environmental performance of polycentric structures in PUR (Xu et al., 2021). However, different methods of measuring morphological and functional polycentricity often produce differentiated results and affect the accuracy of conclusion (Zhang and Derudder, 2019). Scholars argue that morphological and functional polycentricity are not mutually exclusive but complementary [24]. Since polycentric is by urban systems with close inter-regional linkages, their polycentric structure can be more accurately assessed from a network perspective [6]. As scholars propose, morphological polycentricity could be understood from a network perspective, and city importance can be measured in terms of the strength of their total linkages in the network [25]. In other words, from the network perspective, the morphological polycentricity measurement is based on an analysis of the relative balance of the overall linkage intensity of cities [15], while the functional polycentricity measurement is an analysis that considers the balance of linkage intensity and directional diversity. PUR can truly achieve balanced and sustainable development only when they satisfy morphological and functional polycentricity, avoid agglomeration negative externalities, and improve regional competitiveness [26].
Due to the complex and diverse functional linkages among cities, multiple networks are gradually formed [27,28]. The geographic scope and spatial structure of urban networks vary under different types of linkages [6], and localities assume diverse political, economic, and innovative roles. For one function, cities are at the center, but not in comparison to other functions [29]. Although scholars have explored the polycentric structure of PUR using multiple types of functional linkages [18], there is a relative lack of research on the polycentricity of inter-city knowledge cooperation and its innovation networks [30]. In the knowledge economy era, the comprehensive competitiveness of PUR depends more on the level of innovation [6], and knowledge flows and their formation of innovation links are increasingly becoming the core of inter-city relations [31] and affecting the evolution of the spatial structure [32]. Drawing on [33] idea of PUR as innovation incubator and hinge [34], propose the concept of knowledge polycentricity and analyze the knowledge flows within and outside city clusters and their impact on the evolution of spatial structure in the Yangtze River Delta. In addition, [35] used the Gini coefficient approach to measure the evolutionary characteristics of the polycentric science system in the GBA and analyzed its driving mechanism. With the increasing intercity knowledge cooperation, the flow of innovation factors within and outside the PUR is becoming more frequent and agglomerated in some cities. Then, these cities gradually become important incubators for knowledge production and innovation, which promote the development of morphological polycentricity. In addition, under the role of priority linkage mechanism, some core cities become important contacts for other cities and gather a large number of high-intensity innovation linkages, which become the hubs of innovation networks and play an important hinge function in internal and external knowledge cooperation in PUR. These studies have opened up new horizons for research related to innovation linkages of PURs and their network structures but still lack the analysis of the evolution pattern of innovation network morphology and functional polycentricity.
Although scholars have conducted more studies on polycentricity, there are some shortcomings in the following aspects. Firstly, polycentricity is a dynamic process of spatial integration, and the degree of polycentricity of PUR will increase with the growth of city size and the strengthening of inter-regional ties. However, existing studies mainly measure a single time to analyze regional polycentricity while lacking a dynamic evolutionary perspective to analyze its evolutionary process. Secondly, although scholars have used relational data such as traffic flow and information flow to measure the functional polycentricity of PUR, the impact of knowledge system polycentricity and its collaborative innovation development in PUR has been less measured at the level of knowledge cooperation. Finally, the current studies focus on analyzing the global PUR structure and it is planning practices in developed countries but lack analysis of the mechanisms driving the polycentric structure of knowledge systems in PUR in developing countries, especially the impact of multi-dimensional proximity on the formation and evolution of polycentric network structures in conjunction with proximity theory.
In this paper, we constructed the inter-city innovation network through the following steps. Firstly, the data of joint patent applications were extracted. The data of joint patent applications published by the China Intellectual Property Office (CNIPA) were extracted through big data technology, including the basic information such as inventors’ names, units, and regions. In this study, big data technology is used to crawl and download the patent data stored in the Patent Information Service Platform website based by writing a programming due to the difficulty of downloading the huge amount of website data directly. Secondly, geographic information was matched. The python software is a programming design language library that provides efficient high-level data structures for simple and effective object-oriented programming and has been widely used in web development, artificial intelligence, spatial statistics, and other applications. In this study, we use the python software geocoding function to write a programming language which matches the address information of each patent to each city, thus transforming inter-patent cooperation linkages into inter-city patent cooperation. Therefore, this paper constructs a weighted and directed OD matrix from the patent cooperation data between city i and city j to generate the innovation network (Eq. 1). The final step is innovation network construction and spatial visualization. The strength of inter-city innovation linkage was used as the weight of network edge, and the total amount of city innovation linkage was used as the weight of nodes to construct the OD linkage network and visualize it spatially through GIS software.
The degree of polycentricity is often measured by the rank-size analysis, which describes the distribution pattern of city size to reveal morphological polycentricity. The social network analysis method focuses on measuring the network topology to reveal functional polycentricity. To comprehensively analyze the spatial and temporal evolution of knowledge polycentricity in the three major PURs, we measured the morphological and functional polycentricity of the PURs by means of rank-size analysis and social network analysis.
1) Morphological polycentricity: Scholars have used various methods to characterize the distribution of urban hierarchical scale and measure the morphological polycentricity of PURs by comparing them to monocentric or polycentric structures in an ideal state. [1] suggested that there is a balanced distribution of large and small cities in the PUR system, rather than dominated by a single large city. Therefore, the size distribution of large and small cities in the PUR is long and more planar. The morphological polycentricity of PUR could be identified by means of rank-size analysis. If the absolute value of the slope of the fitted line q (polycentric index) is greater than 1, it indicates that the cities are more decentralized in size and have the polycentric characteristics. If the absolute value of the slope of the fitted line is less than 1, it indicates that large cities dominate the development of the urban system and have the monocentric character. We characterized and ranked the size in terms of the number of city patents and measured the degree of polycentricity within PURs by applying the rank-size (Zipf) analysis.
In Eq. (2),
2) Functional polycentricity: The functional polycentricity of the innovation network of PURs was measured by drawing on Green’s modified social network analysis with the following equation.
Network density (D): In the complex network, network density reflects the closeness of the overall connection between nodes, which is equal to the proportion of the actual number of connections and the theoretical maximum possible number of connections.
In Eq. 4, D denotes network density,
In Eq. (5), PF is the network (functional) polycentricity of the PUR, σF is the standard deviation of the innovation linkage level of each node in the measured PUR, σFmax is the standard deviation of the highest value in the assumed two-node network where one node is 0, and the other node is the highest value in the network; D denotes the network density.
As the research on “multi-dimensional proximity and innovation” has gradually intensified, scholars have defined various forms of proximity and explored their roles in the evolution of innovation networks. Drawing on [36], the role of geographic proximity, institutional proximity, social proximity, and cultural proximity in the evolution of the polycentric structures of innovation networks were analyzed, with consideration of the specificity of inter-city technological cooperation.
1) Geographical Proximity: The geographical distance between cities was calculated based on the latitude and longitude of the cities. Since the administrative center of cities tend to reside in the center of the district in China, and the latitude and longitude data of each administrative center are officially published. Therefore, we use the administrative center of cities as the starting and ending points, and calculate the geographical distance between cities using latitude and longitude data. Since the geographic distances between some cities are large (e.g., more than 4,000 km), regression was performed using logarithm in order to reduce the gap between the data and to eliminate the effects of covariance and heteroskedasticity in the model. In addition, taking logarithms would not change the nature of the data and the relative relationship between the variables. With smaller values of geographic distance, the cities are closer to each other, and the geographic proximity is relatively better. The geographical proximity can be expressed by the following equation, where
2) Institutional Proximity: Cities in the same provinces often have a natural advantage in administrative coordination and regional division of labor, as well as better institutional proximity [18]. Therefore, provincial administrative boundaries were defined by setting dummy variables to characterize inter-city institutional proximity.
3) Social Proximity: As an important representation of kinship relations between actors, social proximity also has a profound impact on the formation and evolution of urban networks. Drawing on [37], the jaccard index was constructed to measure social proximity.
In Eq. 7,
4) Cultural Proximity: In this paper, we chose language affinity as an important indicator of cultural proximity and set a dummy variable (0 or 1) to describe whether a common dialect is spoken between two cities based on the 2010 Atlas of Chinese Languages [38].
Due to the number of zeros and the over dispersion characteristic of the variables in the data, it is difficult to obtain valid unbiased estimates using the OLS. Based on the panel data of inter-city patent cooperation from 2005 to 2015, this paper constructs a negative binomial regression model (NBR) to explore the factors influencing the evolution of knowledge polycentricity. The formula is as follows.
In Eq. 8, the dependent variable
In this paper, three well-represented and typical major PURs, Beijing-Tianjin-Hebei (BTH), Yangtze River Delta (YRD), and Pearl River Delta (PRD), were selected as the study areas. Three major PURs are selected as the study regions based on the following considerations. Firstly, three major PURs are the most urbanized and industrialized regions in China, and have formed the more mature regional spatial organization of economic integration, which is qualified to perform polycentric network analysis. However, other urban regions have the low level of urbanization and industrialization and have not yet formed a more complete spatial organization, making it difficult to perform polycentric measurements. Secondly, three major PURs account for more than 40% of the economy and are the core growth poles of China’s economic development. Their development has been elevated to a national strategy by the Chinese government and represents the future direction and trend of urban regions. Although other urban agglomerations exist in China, their economic output are far less representative than the three urban agglomerations. In addition, although there are only three urban agglomerations as the study area, BTH includes 13 cities, YRD includes 41 cities, and PRD includes 9 cities, and the number of all cities has reached 63. Finally, three major PURs have rich scientific and educational resources, developed high-tech industries and many innovative talents, and the innovation level is highly developed and a better science and technology collaboration system has been formed, which is suitable for performing knowledge polycentric analysis. Therefore, the selection of three major PURs as the study regions is representative and typical, which is not only good for understanding the evolution pattern of PUR knowledge polycentric pattern, but also has good reference significance for the formulation of other PUR collaborative innovation development strategies.
According to the spatial scope of the PUR plan developed by the Chinese government, BTH includes 13 cities, PRD includes 9 cities, and the YRD includes 41 cities. Since patent cooperation and social statistics are difficult to obtain for Hong Kong and Macau, nine cities in the PRD were mainly selected. As the region with the strongest economic development and innovation capacity in China, three major PURs have rapid urbanization and a relatively high level of regional integration. These regions have become important growth poles leading economic development and the stage to connect with the world economy, making them the most international and representative PURs in China (Figure 1).
As the world’s largest technology source, patent data comprehensively reflects the research output of each region and records the cooperation parties and their basic information in detail. These characteristics make the patent data an important data source for describing the flow of technological knowledge between cities. In this paper, we used joint patent applications to characterize inter-city patent cooperation, and these data were obtained from the “Patent Information Service Platform” in the database of SIPO. In addition, we used the geocoding function of Python software to identify and match the spatial attribute information of each patent cooperation information, and the data of 2005 and 2015 were extracted by cross-checking and manual verification. The inter-city patent cooperation data of the three major PURs were filtered out, including 22,460 patent cooperation data in BTH, 34,251 patent cooperation data in YRD, and 12,374 patent cooperation data in PRD.
Based on the inter-city patent cooperation data, the innovation linkage matrix of the PURs for 2005, 2010 ,and 2015 was constructed, and spatial characterization analysis was performed using ArcGIS software (Table 1). The main findings are as follows.
1) The inter-city innovation linkages in BTH have been increasing, but a clear core-edge pattern emerges. Firstly, Beijing-Tianjin has always been the strongest linkage pair in the innovation network, becoming the backbone of technological knowledge flows. This phenomenon reflects that the economically developed core cities are the incubators and linkage hubs for knowledge production and external diffusion. Secondly, the innovation linkages between the edge cities in Hebei Province, such as Qinhuangdao, and other cities are always at the lowest level, making it difficult to obtain knowledge spillover, indicating that inter-city knowledge spillover is still limited by geographical distance. Moreover, Beijing’s external innovation linkages are greater than those of other cities such as Tianjin, making the PUR form an obvious monocentric spatial pattern. Finally, with the implementation of the BTH cooperative development strategy, BTH innovation linkages gradually expanded to cities in Hebei province in 2015. The innovation linkages in BTH gradually became intensive, and a networked pattern initially emerged. However, these linkages were still weakly connected, and the innovation linkages of the PUR showed a core-edge pattern.
2) The innovation linkages in YRD have formed a more stable polycentric spatial pattern. Firstly, as the innovation carriers such as scientific research institutions and high-tech enterprises are mainly distributed between the eastern cities such as Shanghai and Hangzhou, innovation linkages are highly concentrated among economically developed core cities. This type of linkage distribution reflects the level of economic development and the concentration of innovative enterprises, which greatly facilitates inter-city knowledge production and flow. Secondly, two provincial capitals, Nanjing and Hangzhou, have also formed high-intensity innovation linkages with other cities in the province, reflecting that inter-city knowledge flows are still influenced by administrative divisions. Finally, in 2005, only Shanghai and Hangzhou formed the highest intensity of innovation linkages, and the development of the polycentric structure of the PUR was limited. The development of sub-center cities such as Nanjing and Hangzhou has also become important hubs for the concentration of innovation resources, promoting the rapid development of the polycentric spatial pattern of the PUR. This result indicates that government planning has also contributed to the formation and development of the PUR polycentric (Figure 2).
3) The innovation linkages in PRD mainly revolve around the two core cities of Guangzhou and Shenzhen, forming a dual-center network pattern. Firstly, Guangzhou and Shenzhen have much more total linkages than other cities in the network and have become important knowledge producers and incubators. However, there are differences in the basis of their innovation. Shenzhen has become the innovation network highland with many high-tech enterprises (e.g., Huawei), while Guangzhou relies mainly on universities and other research institutions to become an important innovation center. These differences reflect that both high-tech enterprises and research institutions play a significant role in forming innovation centers. Secondly, with the development of innovation networks, other cities have more innovation linkages with Guangzhou and Shenzhen, indicating that inter-city innovation linkages have a strong merit-based linking mechanism. In addition, innovation factors such as technology and talent have stronger clustering characteristics than traditional production factors. Finally, Dongguan and Foshan also formed high-grade innovation linkages with Guangzhou and Shenzhen in 2015, respectively. The total number of external linkages was also increasing, reflecting the evolutionary trend of the dual-center pattern towards polycentricity in PRD.
TABLE 1. Top five innovation linkages of Chinese three PURs in 2015. Morphological polycentricity and functional polycentricity of three PURs.
Drawing on [1], we ranked the number of patents in the cities to characterize the morphological polycentricity in PURs (Figure 3).
Firstly, in terms of evolutionary trends, the absolute values of the three major PURs’ morphological polycentricity show a generally decreasing trend, indicating that the morphological polycentricity of innovation networks is strengthening. With the rapid urbanization, the increasing knowledge cooperation in the three PURs has led to the accelerated flow and agglomeration of innovation factors in some big cities. This makes these cities become incubators for knowledge production and innovation R&D, which in turn promotes the improvement of urban innovation capacity and the development morphological polycentricity of PUR. With the rapid urbanization and increasing technological cooperation. Secondly, BTH polycentric index has the largest absolute value, followed by PRD polycentric index, and YRD polycentric index has the lowest absolute value, indicating that YRD polycentric structure is the most obvious in terms of the knowledge size distribution while BTH mainly presents a monocentric structure. In 2010, the degree of polycentricity of BTH significantly declined and was only improved in 2015, indicating that the development of PUR polycentricity is a cyclical and gradual process and needs to be guided by long-term observation and planning. Finally, in terms of the magnitude of the change, the absolute value change of the polycentricity index of YRD is the largest, while that of the polycentricity index of the BTH is the lowest, further indicating that the innovation resources of the BTH are always highly concentrated in Beijing. Due to the siphoning effect of the first city on other cities, the knowledge production and innovation R&D capacity of Beijing, Tianjin and Hebei Province is characterized by “one city alone”, while other cities lack innovation resources to become innovation centers and limit the development of morphological polycentricity. In recent years, inter-city knowledge cooperation in the YRD has been increasing and forming the certain functional division of labor. This has led to a concentration of innovation resources not only in the top city but also in other big cities. In this background, Hangzhou, Nanjing, and Suzhou have become incubators and innovation centers for knowledge production and innovation, improving the morphological polycentricity of PUR. These factors cause the size of Shanghai to decline in the scientific knowledge system of the PUR, while more other cities improve their knowledge production capacity and increase their size. However, the slope of the fitted curve mainly satisfies the size distribution trend of most cities. Therefore, in the fitted curve of the YRD rank order scale analysis, most of the medium-sized cities dominated the magnitude and trend of the slope, while the scale distribution of Shanghai could not reach the desired height and was below the highest point of the fitted curve.
Although the rank-size analysis reveals the scale distribution characteristics of PUR linkages and their morphological polycentricity, it does not explore the balance of functional linkages distribution and functional polycentricity. Therefore, this paper adopts the social network analysis proposed by [8] to measure the evolution characteristics of functional polycentricity in three major PURs (Figure 4).
Firstly, in the evolutionary trend aspect, the functional polycentricity indexes of the three major PURs all increased, reflecting that the direction becomes relatively more diversified and intensity becomes more balanced of innovation linkage in the PURs. In addition, core cities become hubs for internal and external knowledge cooperation and play the function of hinges in the innovation network. Since 2010, the functional polycentricity of the three major PURs has increased substantially, and the inter-city innovation linkages have developed the trend of networking and polycentricity. Secondly, there are regional differences in the polycentricity among PURs, the YRD having the highest functional polycentricity and the BTH having the lowest degree of functional polycentricity, reflecting that the innovation linkages of the YRD are more balanced. In the YRD, several core cities such as Shanghai, Nanjing and Hangzhou gather more high-level innovation linkages and become important hubs for knowledge cooperation not only within the PUR but also at the national scale, thus playing an important hinge role in innovation networks. However, BTH is underdeveloped in terms of functional polycentricity due to the fact that the primary city dominates the development of the innovation network and performs the hinge function of bridging internal and external knowledge cooperation, while the other cities are less interlinked and play a lesser hinge role. Finally, previous studies measured the degree of functional polycentricity of PURs using traffic flow, enterprise flow, and information flow, and this paper uses similar information. As socio-economic linkages between cities intensify, innovation factors such as capital, technology and people flow in a more diversified and balanced direction, resulting in core cities playing a hinge role in internal and external knowledge collaboration and driving the functional polycentric evolution of PURs.
Morphological and functional polycentricity reflect the evolution of the spatial structure of the PUR. Table 1 summarizes the evolution characteristics of morphological polycentricity and functional polycentricity in the three major PURs (Table 1).
Firstly, morphological polycentric and functional polycentric are coupled but have certain differences. In terms of the coupling, the morphological polycentricity and functional polycentricity of technological knowledge are relatively higher in the YRD and PRD than in the BTH. In terms of the differences, the number and scale of linkages of sub-center cities in the innovation networks of the three major PURs have been gained. However, the directionality and balance of linkages are relatively insufficient, leading to functional polycentricity lower than morphological polycentricity. Secondly, with the strengthening of intercity knowledge cooperation, the innovation factors accelerate flow and agglomeration, on one hand promoting the improvement of urban and regional knowledge production capacity and the morphological polycentric evolution, and on the other hand facilitating the enhancement of intercity innovation linkages and the functional polycentric development. Finally, the level of technological integration has a deep impact on the function of incubators and hinges in PUR, and the stronger the intercity knowledge cooperation linkages, the better the functions of knowledge production (incubators) and knowledge collaboration (hinges). The YRD has the best intercity innovation linkages and the highest level of technological integration, with several large cities not only becoming centers of innovation factor aggregation and knowledge production, but also hubs and gateways for internal and external knowledge collaboration. However, the BTH has the low level of innovation linkage and relatively low level of technological integration, which leads to the knowledge production and knowledge collaboration functions are still mainly concentrated in the primary city. There is not yet a good form of technological division of labor and limits the rapid development of incubator and hinge functions in PUR.
In the background of economic globalization, the cities are not isolated, but formed complex and various socio-economic linkages with each other, which driving the economic development and the polycentric structure evolution of PUR through factor flow and agglomeration. In particularly, inter-city knowledge cooperation promotes the free flow of innovation factors and shapes knowledge polycentricity of PUR through agglomeration and diffusion effects (Yingcheng and Nicholas, 2018). In addition, previous studies have shown that the strengthening of inter-city knowledge interactions and innovation linkages underlies the evolution of knowledge polycentricity at different geographical scales [39]. Some medium and small cities could achieve agglomeration economies through the innovation linkages that develop with large cities, thus driving the rise of sub-core cities and innovation networks, which in turn drive the development morphology and functional polycentricity of PUR. However, economic geographers argue that inter-city knowledge flows are not only influenced by geographic, organizational, institutional, social, and cultural proximity but are also conditioned by the socio-economic environment of cities. Therefore, this paper analyzes the driving mechanism of the evolution of knowledge polycentricity in PURs at two levels: city attributes and inter-city proximity. Inter-city proximity mainly includes four dimensions: Geographic, institutional, institutional, and cultural dimensions. City attributes mainly include economic development level, population size, number of R&D personnel, R&D capital investment, and number of patents granted (Table 2).
To verify the reasonableness of the model, the baseline regression model was tested for multiple covariance, heteroskedasticity, and serial autocorrelation. The results show that the maximum values of both are less than 10, indicating that there is no multicollinearity. The p-value of the BG test is 0.005, indicating that there is no serial autocorrelation. In terms of inter-city proximity, geographic, institutional, social and cultural proximity all significantly affect knowledge flows, driving the formation of innovation networks and knowledge polycentric development (Table 3). Firstly, since geographic proximity is a negative indicator, the intensity of inter-city patent cooperation increases with the decrease of the geographic distance, which expands the scope of knowledge spillover from the core city and increases the innovation level of surrounding cities. In recent years, the construction of expressways and high-speed railroads in PURs in China has contributed to the “spatial-temporal convergence” effect, enhanced geographical proximity of cities along the route, and facilitated knowledge exchange and cross-regional patent cooperation. Secondly, institutional proximity has a significant positive impact on inter-city patent cooperation since it can reduce the cooperation cost and increase the possibility of success. For example, the YRD cities with high institutional proximity have engaged in a lot of bottom-up governmental cooperation, contributing to the tightening of inter-city innovation linkages and the development of knowledge polycentricity. However, the BTH cities have poor inter-city institutional proximity since they belong to three different provinces, and there is less inter-governmental cooperation, which constrains the development of knowledge polycentricity. Finally, cultural and social proximity also have a significant positive impact on inter-city patent cooperation, reflecting that good social relationships and cultural affinity are conducive to enhancing trust in inter-city patent cooperation, creating a social environment for knowledge spillover, and promoting inter-city innovation linkages.
In terms of city attributes, the level of economic development, the number of R&D personnel, and physical capital investment significantly promote inter-city patent cooperation, but the effect of city population size is not significant. High-intensity patent cooperation is more likely to be formed among economically developed cities since less economically developed regions lack the demand and motivation for patent cooperation. In recent years, the rapid economic development of the YRD and PRD have led to the formation of several economically developed sub-center cities, which have led to a more diversified direction of innovation resource flow between cities and increased functional polycentricity by radiation effect. In particularly, the YRD has formed several cities with GDP over trillion such as Shanghai, Hangzhou and Nanjing, and the PRD has formed three cities with GDP over trillion such as Shenzhen, Guangzhou and Foshan. In addition, the number of R&D personnel and physical capital are also important conditions for innovation activities, and inter-city patent cooperation is mainly directed to large cities with strong scientific research strength, such as Shanghai, Beijing and Shenzhen, which have become the main targets of high-intensity innovation links in different city clusters. However, although Beijing has a large number of higher education institutions and research institutes and a large amount of physical capital investment, other cities in the PUR have a serious lack of scientific research strength, which leads to the development trend of knowledge monocentricity by siphon effect. In addition, the number of R&D personnel and physical capital are also important factors for innovation activities, and inter-city patent cooperation is mainly directed to large cities with strong scientific research strength. Moreover, the population size of cities does not have a significant impact on inter-city patent cooperation, suggesting to a certain extent that inter-city innovation linkages are not dependent on population size but are more influenced by inter-city proximity and innovation investment of cities.
Based on the unique patent cooperation data, this paper establishes a systematic framework for identifying the polycentric of PUR by using the rank-size analysis and social network analysis. The main conclusions of this paper are as follows.
1) In terms of morphological polycentricity, the BTH mainly form a monocentric structure, the PRD forms a bicentric structure while the YRD forms a polycentric structure. The core cities of Beijing, Shanghai and Shenzhen become the hubs of innovation resources and create a large number of invention patents through knowledge collaboration, thus playing the function of incubators of knowledge production and innovation outcomes in PUR. In terms of functional polycentricity, the three major PURs have been increasing their functional polycentricity, but show a differentiated development trend. The YRD has the highest degree of functional polycentricity, while BHT has the lowest degree of functional polycentricity. For example, Shanghai, Nanjing and Hangzhou all form higher-level innovation links with other cities, and become important linkage hubs for regional knowledge collaboration, which also plays the function of hinge in internal and external knowledge integration.
2) The morphological polycentricity and functional polycentricity of PUR are not only coupled, but also differentiated. In terms of collinearity, the innovation networks of the YRD have formed a more stable morphology and a higher degree of functional polycentricity than those of BTH. In terms of difference, the number and scale of the innovation networks have obtained the increase, but the direction and balance of the linkages are relatively insufficient. In addition, the functional polycentricity is generally higher than the morphological polycentricity in three PUR.
3) In terms of the driving mechanism, the increased innovation linkages and the improved knowledge polycentricity in PURs are attributed to a combination of geographic, institutional, social, and cultural proximity. In the evolution of knowledge polycentricity, geographic, institutional and social multidimensional proximity promotes the free flow of talent and knowledge elements between cities, and makes large cities becoming the important clusters of innovation elements and incubators of knowledge production, which promotes the development of morphological polycentricity. In addition, multi-dimensional proximity also promotes inter-city knowledge collaboration and enhances the overall strength of innovation networks, and makes core cities become important hubs for internal and external knowledge integration, driving the evolution of functional polycentricity in PUR.
In the background of economic globalization, PUR have gained new importance as the basic unit for national participation in global trade and external exchanges. Although previous studies have used traffic flow, enterprise flow and information flow to measure the polycentricity of PUR, and have argued that PUR become the places of economic agglomeration and hinges of internal and external linkages [6,23,40]. However, the knowledge cooperation perspective is lacking to directly analyze the incubator and hinge functions of PUR. Based on the perspective of innovation network, this paper found that the core cities of PURs become the agglomeration of innovation resources and the incubator of knowledge production and innovation outcomes through internal and external knowledge integration. Moreover, the core cities have formed high-intensity innovation linkages with other cities and become the gateway cities with the strongest knowledge collaboration linkages, playing an important hinge function. Therefore, the perspective of innovation network helps to understand the process of knowledge polycentric evolution and explains the incubator and hinge function of core cities in knowledge production and knowledge collaboration at the theory level, providing evidence for the view of Gottman about the PUR as incubator and hinge of knowledge production and hinge of innovation networks. This paper also proves that PURs show polycentric development characteristics from a dynamic perspective in China, which making the PURs develop toward more balanced and sustainable polycentricity. Last but not least, this paper finds not only coupling but also differences between morphological and functional polycentricity in PURs. However, other scholars generally agreed that morphological polycentricity differs from functional polycentricity [4,19,35], providing new evidence for understanding the evolutionary pattern of urban regionalization and polycentric structure. Therefore, the identification of PUR polycentric structure should be measured from morphological and functional perspectives separately to obtain more accurate results and to formulate regional spatial development strategies.
Due to the lack of data, this paper mainly uses patent cooperation data to analyze the evolution of knowledge polycentricity. However, high-tech enterprises and firms are also important carriers of knowledge and have an important impact on inter-city knowledge flows. In addition, this paper mainly understands the evolution pattern of knowledge polycentricity and its driving mechanism from the regional scale, but the knowledge production (incubator) and knowledge collaboration (hinge) functions of core cities may show different characteristics at the national or global scale. Such as Beijing is not only a knowledge production center and innovation linkage hub of BTH, but also a national or global knowledge production center or internal and external innovation linkage hub and gateway. Therefore, future research could further focus on the influence of the multiplicity of city networks at different scales on the formation and evolution of polycentric structures to provide a more comprehensive understanding of the evolution of polycentric structures and spatial effects [41].
Our study can enlighten future urban and regional planning from three aspects. Firstly, when formulating city-regional plans, extensive attention should be paid to the spatial and temporal evolution of innovation network linkages of PURs. The scope of PURs should be delineated, and central cities should be identified based on actual functional linkages to guide a more balanced distribution of innovation resources among different central cities to increase inter-city technology cooperation. Secondly, the building of infrastructure of the central cities should be strengthened, and the social-institutional environment should be improved to attract high-tech enterprises and enhance the leading role of the central cities in the knowledge flow within and outside the PUR. Thus, the knowledge spillover effect of the central cities can be fully utilized to drive the coordinated development of the neighboring cities. Finally, when actively integrating into the innovation network of the PUR, other cities in the cluster should find their positions and give full play to their comparative advantages, which will encourage the diversification of inter-city technological cooperation and form a functional complementary technological innovation system. In this way, the efficiency of technological output will be improved, and the overall innovation level of the PUR can be promoted.
The original contributions presented in the study are included in the article/Supplementary Material, further inquiries can be directed to the corresponding author.
CT: Conceptualization, Methodology, Writing-Original. JD: Methodology, Writing-Reviewing and Editing, Validation, Resources.
This study was funded by the National Natural Science Foundation of China (No. 71974120) and Social Science Planning Project of Shanghai (No. 2018BJL005).
The authors declare that the research was conducted in the absence of any commercial or financial relationships that could be construed as a potential conflict of interest.
All claims expressed in this article are solely those of the authors and do not necessarily represent those of their affiliated organizations, or those of the publisher, the editors and the reviewers. Any product that may be evaluated in this article, or claim that may be made by its manufacturer, is not guaranteed or endorsed by the publisher.
The authors would like to thank the editors and reviewers for their helpful comments and suggestions.
1. Meijers EJ, Burger MJ. Spatial Structure and Productivity in. Environ Plann A (2010) 42(06):1383–402. US Metropolitan Areas. doi:10.1068/a42151
2. Vasanen A. Functional Polycentricity: Examining Metropolitan Spatial Structure through the Connectivity of Urban Sub-centres. Urban Stud (2012) 49(16):3627–44. doi:10.1177/0042098012447000
3. Goei BD, Burger MJ, Oort FV, Kitson M. Functional Polycentrism and Urban Network Development in the Greater South East UK: Evidence from Commuting Patterns, 1981-2001. Reg Stud (2010) 44(09):1149–70. doi:10.1080/00343400903365102
4. Taubenböck HSIW. Measuring Morphological Polycentricity - A Comparative Analysis of Urban Mass Concentrations Using Remote Sensing Data. Comput Environ Urban Syst (2017) 64(07):42–56.
5. Camille R, Kang SM, Michael B, Marc B, Matjaz P. Structure of Urban Movements: Polycentric Activity and Entangled Hierarchical Flows. PLoS ONE (2011) 6(01):e15923.
6. Wang T, Yue W, Ye X, Liu Y, Lu D. Re-evaluating Polycentric Urban Structure: A Functional Linkage Perspective. Cities (2020) 101(06):102672. doi:10.1016/j.cities.2020.102672
7. Taylor PJ, Evans DM, Pain K. Application of the Interlocking Network Model to Mega-City-Regions: Measuring Polycentricity within and beyond City-Regions. Reg Stud (2008) 42(08):1079–93. doi:10.1080/00343400701874214
8. Green N. Functional Polycentricity: A Formal Definition in Terms of Social Network Analysis. Urban Stud (2007) 44(11):2077–103. doi:10.1080/00420980701518941
9. Cai J, Huang B, Song Y. Using Multi-Source Geospatial Big Data to Identify the Structure of Polycentric Cities. Remote Sensing Environ (2017) 202(12):210–21. doi:10.1016/j.rse.2017.06.039
10. Dadashpoor H, Shirvan SS. Measuring Functional Polycentricity Developments Using the Flow of Goods in Iran: a Novel Method at a Regional Scale. Int J Urban Sci (2019) 23(04):551–67. doi:10.1080/12265934.2018.1556114
11. Giffinger R, Suitner J. Polycentric Metropolitan Development: From Structural Assessment to Processual Dimensions. Eur Plann Stud (2015) 23(06):1169–86. doi:10.1080/09654313.2014.905007
12. Schweitzer F, Zhang Y, Casiraghi G. Intervention Scenarios to Enhance Knowledge Transfer in a Network of Firms. Front Phys (2020) 8:382. doi:10.3389/fphy.2020.00382
13. Dong G, Duan D, Xia Y. A Briefing Survey on Advances of Coupled Networks with Various Patterns. Front Phys (2021) 9:795279. doi:10.3389/fphy.2021.795279
14. Sarkar S, Wu H, Levinson DM. Measuring Polycentricity via Network Flows, Spatial Interaction and Percolation. Urban Stud (2020) 57(12):2402–22. doi:10.1177/0042098019832517
15. Liu X, Derudder B, Wu K. Measuring Polycentric Urban Development in China: An Intercity Transportation Network Perspective. Reg Stud (2016) 50(08):1302–15. doi:10.1080/00343404.2015.1004535
16. Davoudi S. Polycentricity in European Spatial Planning: from an Analytical Tool to a Normative Agenda. Eur Plann Stud (2003) 11(08):979–99. doi:10.1080/0965431032000146169
17. Hanssens H, Derudder B, Van Aelst S, Witlox F. Assessing the Functional Polycentricity of the Mega-City-Region of Central Belgium Based on Advanced Producer Service Transaction Links. Reg Stud (2014) 48(12):1939–53. doi:10.1080/00343404.2012.759650
18. Zhang W, Derudder B, Wang J, Witlox F. An Analysis of the Determinants of the Multiplex Urban Networks in the Yangtze River Delta. Tijdschrift voor Economische en Sociale Geografie (2020) 111(02):117–33. doi:10.1111/tesg.12361
19. Zhao M, Derudder B, Huang J. Examining the Transition Processes in the Pearl River Delta Polycentric Mega-City Region through the Lens of Corporate Networks. Cities (2017) 60(02):147–55. doi:10.1016/j.cities.2016.08.015
20. Natalia VV, Heinrichs D. Identifying Polycentricism: a Review of Concepts and Research Challenges. Eur Plann Stud (2020) 28(04):713–31. doi:10.1080/09654313.2019.1662773
21. Dadashpoor H, Alidadi M. Towards Decentralization: Spatial Changes of Employment and Population in Tehran Metropolitan Region, Iran. Appl Geogr (2017) 85(08):51–61. doi:10.1016/j.apgeog.2017.05.004
22. Zhen F, Cao Y, Qin X, Wang B. Delineation of an Urban Agglomeration Boundary Based on Sina Weibo Microblog 'check-In' Data: A Case Study of the Yangtze River Delta. Cities (2017) 60(08):180–91. doi:10.1016/j.cities.2016.08.014
23. Sokol M, Van Egeraat C, Williams B. Revisiting the ‘Informational City’: Space of Flows, Polycentricity and the Geography of Knowledge-Intensive Business Services in the Emerging Global City-Region of Dublin. Reg Stud (2008) 42(08):1133–46. doi:10.1080/00343400801932284
24. Hoyler M, Freytag T, Mager C. Connecting Rhine-Main: The Production of Multi-Scalar Polycentricities through Knowledge-Intensive Business Services. Reg Stud (2008) 42(08):1095–111. doi:10.1080/00343400701874230
25. Burger M, Meijers E. Form Follows Function? Linking Morphological and Functional Polycentricity. Urban Stud (2012) 49(05):1127–49. doi:10.1177/0042098011407095
26. Lai W, Yun L, Miao W, Shiliang S, Li B, Hangyu J. Multiscale Identification of Urban Functional Polycentricity for Planning Implications: An Integrated Approach Using Geo-Big Transport Data and Complex Network Modeling-ScienceDirect. Habitat Int (2020) 97(03):102134.
27. Hu X, Wang C, Wu J, Stanley HE. Understanding Interurban Networks from a Multiplexity Perspective. Cities (2020) 99(4):102625. doi:10.1016/j.cities.2020.102625
28. Yuan Z, Lin H, Tang S, Guo R. Geographically Explicit Network Analysis of Urban Living and Working Interaction Pattern in Shenzhen City, South China. Front Phys (2021) 9:795641. doi:10.3389/fphy.2021.795641
29. BurgerMartijn J, van, der K, Bert W, Ronald S. Polycentricity and the Multiplexity of Urban Networks. Eur Plann Stud (2014) 22(04):816–40. doi:10.1080/09654313.2013.771619
30. Cao Z, Derudder B, Dai L, Peng Z. ‘Buzz-and-pipeline’ Dynamics in Chinese Science: the Impact of Interurban Collaboration Linkages on Cities’ Innovation Capacity. Reg Stud (2021) 62(04):1–17.
31. Xie Q, Su J. The Spatial-Temporal Complexity and Dynamics of Research Collaboration: Evidence from 297 Cities in China (1985-2016). Technol Forecast Soc Change (2021) 162(01):120390. doi:10.1016/j.techfore.2020.120390
32. Li P, Miao Q, Meng Y, Ning J, Long J, Huang J. A Study on the Attention of Yoga and its Development Based on Complex Network Theory. Front Phys (2021) 9:719012. doi:10.3389/fphy.2021.719012
34. Li Y, Wu F. Understanding City-Regionalism in China: Regional Cooperation in the Yangtze River Delta. Reg Stud (2018) 52(03):313–24. doi:10.1080/00343404.2017.1307953
35. Ma H, Li Y, Huang X. Proximity and the Evolving Knowledge Polycentricity of Megalopolitan Science: Evidence from China's Guangdong-Hong Kong-Macao Greater Bay Area, 1990–2016. Urban Stud (2020) 58(02):1–19. doi:10.1177/0042098020942665
36. Boschma R. Proximity and Innovation: A Critical Assessment. Reg Stud (2005) 39(01):61–74. doi:10.1080/0034340052000320887
37. Scherngell T, Hu Y. Collaborative Knowledge Production in China: Regional Evidence from a Gravity Model Approach. Reg Stud (2011) 45(06):755–72. doi:10.1080/00343401003713373
39. Liu X, Derudder B, Taylor P. Mapping the Evolution of Hierarchical and Regional Tendencies in the World City Network, 2000-2010. Comput Environ Urban Syst (2014) 43(01):200051–1066. doi:10.1016/j.compenvurbsys.2013.10.004
40. Liu C, Niu C, Han J. Spatial Dynamics of Intercity Technology Transfer Networks in China's Three Urban Agglomerations: A Patent Transaction Perspective. Sustainability (2019) 11(6):1647. SSCI/SCI. doi:10.3390/su11061647
41. Song W, Sheng W, Li D, WuandMa CJ, Ma J. Modeling Complex Networks Based on Deep Reinforcement Learning. Front Phys (2022) 9:822581. doi:10.3389/fphy.2021.822581
42. Roca Cladera J, Marmolejo Duarte CR, Moix M. Urban Structure and Polycentrism: Towards a Redefinition of the Sub-centre Concept. Urban Stud (2009) 46(13):2841–68. doi:10.1177/0042098009346329
43. Hall P, Pain K. The Polycentric metropolis: Learning from Mega-City Regions in Europe. London: Routledge (2006).
44. Meijers E. Measuring Polycentricity and its Promises. Eur Plann Stud (2008) 16(09):1313–23. doi:10.1080/09654310802401805
Keywords: polycentric urban region, innovation linkages, functional polycentricity, urban network, collaborative innovation
Citation: Tang C and Dou J (2022) Exploring the Polycentric Structure and Driving Mechanism of Urban Regions From the Perspective of Innovation Network. Front. Phys. 10:855380. doi: 10.3389/fphy.2022.855380
Received: 15 January 2022; Accepted: 17 March 2022;
Published: 06 April 2022.
Edited by:
Takayuki Mizuno, National Institute of Informatics, JapanReviewed by:
Woo-Sung Jung, Pohang University of Science and Technology, South KoreaCopyright © 2022 Tang and Dou. This is an open-access article distributed under the terms of the Creative Commons Attribution License (CC BY). The use, distribution or reproduction in other forums is permitted, provided the original author(s) and the copyright owner(s) are credited and that the original publication in this journal is cited, in accordance with accepted academic practice. No use, distribution or reproduction is permitted which does not comply with these terms.
*Correspondence: Jianmin Dou, am1kb3VAbWFpbC5zaHVmZS5lZHUuY24=
Disclaimer: All claims expressed in this article are solely those of the authors and do not necessarily represent those of their affiliated organizations, or those of the publisher, the editors and the reviewers. Any product that may be evaluated in this article or claim that may be made by its manufacturer is not guaranteed or endorsed by the publisher.
Research integrity at Frontiers
Learn more about the work of our research integrity team to safeguard the quality of each article we publish.